Considerable effects of imaging sequences, feature extraction, feature selection, and classifiers on radiomics-based prediction of microvascular invasion in hepatocellular carcinoma using magnetic resonance imaging
Introduction
Hepatocellular carcinoma (HCC) has high incidence and mortality rates. It is ranked fifth among all malignancies in terms of incidence and is the third most common cause of cancer-related death (1). Partial hepatectomy and liver transplantation are the most effective treatments for HCC (2); however, the respective 5-year recurrence rates are as high as 70% and 35% (3-5). Occult metastatic lesions produced by hepatoma cells that invade microvessels are a major cause of postoperative recurrence of HCC. Numerous studies have shown that microvascular invasion (MVI) is one of the independent risk factors for the prognosis of primary HCC (5-9).
Accurate identification of MVI in patients with HCC is key to formulating treatment strategies and predicting prognosis. However, MVI cannot be reliably diagnosed by biopsy, which makes its preoperative diagnosis challenging. Although several radiologic features of magnetic resonance imaging (MRI) and computed tomography (CT) images, such as tumor margin, internal arteries, and hypodense halos, are known to be predictors of MVI status, a consensus on the best predictive features of MVI in HCC has not been reached (10-12).
The rapid development of medical imaging technology has allowed for more information to be obtained for HCC evaluation. Radiomics, a method that involves the high-throughput extraction of high-dimensional quantitative features from medical images, has received widespread attention in recent years (13-15), due to the additional information it provides for disease analysis. The key steps of radiomics analysis methods include image acquisition, tumor segmentation, feature extraction, and classifier modeling (13,14); all of these steps may have an effect on the final performances of radiomics models for HCC MVI. Studies have investigated the variability of radiomics features with respect to different imaging scanners (16,17), image acquisition parameters (18), tumor delineation methods (19), reconstruction methods (20-22), feature selection methods (23,24), and discretization (25), as well as the variability of model performance using different classifiers (23,24).
To date, several studies have shown that radiomics features based on CT (26-31) or MRI (32-34) perform well for the prediction of HCC MVI, outperforming radiographic features (26,27,30,32). It is worth noting that those studies mainly explored the predictive performance of different radiomics features; although, as mentioned previously, each step of the radiomics analysis can affect the final predictive performance. Ni et al. (31) showed that different feature selection methods and different classifiers had a significant effect on the prediction of HCC MVI based on CT radiomics features. Yang et al. (33) reported that radiomics features performed differently in the prediction of HCC MVI with different MRI sequences. However, almost all studies have neglected the effect of different radiomics feature extraction parameters on the performances of radiomics models for HCC MVI. In addition, some studies have failed to mention the detailed parameters of radiomics feature extraction. Therefore, we aimed to explore the effects of imaging sequences, feature extraction, feature selection and classifiers on the predictive performance of a radiomics biomarker for HCC MVI, and to provide standardized recommendations for subsequent radiomics research related to the prediction of HCC MVI.
Methods
Patients
The study was approved by the Institutional Review Board and Ethical Committee of The First Affiliated Hospital, Sun Yat-sen University, and conformed to the provisions of the Helsinki Declaration. The requirement for informed consent was waived. Between August 2016 and March 2019, we reviewed the medical records and imaging data of all HCC patients diagnosed at the First Affiliated Hospital, Sun Yat-sen University. The inclusion criteria were: (I) a solitary lesion; (II) hepatectomy; (III) no prior surgical or medical anticancer treatment before the MRI scan; (IV) MRI acquired within the 1 month prior to treatment; (V) HCC and MVI confirmed by pathologic examination; (VI) no known distant metastasis; and (VII) images with the same spatial resolution for all sequences. Patients with mixed cell tumors (HCC and cholangiocarcinoma) or with extensive tumor invasion were excluded. Details of the patient recruitment process are shown in Figure 1. Sixty-nine HCC patients (66 males, 3 females; mean age: 52.7 years, range: 18–75 years) met the inclusion criteria; of them, 29 were confirmed with MVI by postoperative histopathology.
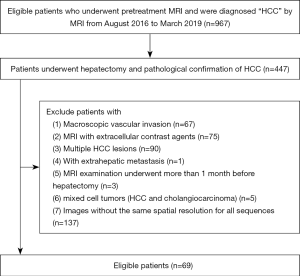
MRI acquisition
Gadoxetic acid-enhanced MRI examinations were performed for all patients (body coil, supine position), using a 3.0T MR system (Siemens Veiro, Germany). The MRI scan sequence included: T1-weighted imaging (T1WI) in/out of phase sequence imaging, pre-enhanced T1-weighted imaging (pre-T1WI) sequence axial imaging, and T2-weighted imaging (T2WI). Following the injection of gadoxetic acid (Primovist®, 0.1 mL/kg body weight) into the cubital vein at a flow rate of 1 mL/s, post-contrast dynamic three-dimensional (3D) T1-weighted volumetric-interpolated breath-hold examination (VIBE) was conducted during the arterial phase (AP; 20–30 seconds), portal venous phase (PVP; 60–70 seconds), and hepatobiliary phase (HBP; 20 minutes). The detailed scanning parameters of the pre-T1WI, AP, PVP, and HBP are shown in Table 1. Figure 2 shows examples of pre-T1WI, AP, PVP, and HBP images of a 55-year-old man.
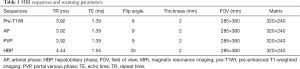
Full table
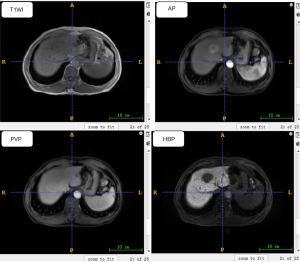
Radiomics feature extraction
The tumor lesions on each axial slice were contoured carefully by a board-certified abdominal radiologist using ITK-SNAP software (www.itksnap.org) and then checked by an experienced radiologist (both with >10 years of experience in abdominal imaging). To increase the reliability of the MRI texture measurements, the voxels within the tumor region with intensities outside the range of µ ± 3σ (µ and σ represent the mean and standard deviation of the image intensities within the tumor region, respectively) were rejected and not considered in subsequent texture computations, as suggested by Collewet et al. (35). Next, all images were resampled to an isotropic voxel size using an interpolation method. Except that by Zhang et al. (34), all previous radiomics studies on the prediction of HCC MVI resampled the images to a voxel size of 1.00×1.00×1.00 mm3. Vallières et al. (36) suggested an isotropic voxel size that was equal to the initial in-plane resolution (1.19×1.19×1.19 mm3 in our study). Considering that the slice thickness was 2 mm, in our study we resampled the images to different sizes including 1.00×1.00×1.00, 1.19×1.19×1.19, 1.50×1.50×1.50, and 2.00×2.00×2.00 mm3. Because high interpolation accuracy can reduce the influence of image distortion, we chose the commonly used 3D cubic interpolation with the Matrix Laboratory (MATLAB, Version R2019a) software.
The quantitative image feature toolkit, which was developed in-house using MATLAB, was used to calculate the radiomics features. Using an image of a single tumor lesion as input, we calculated 167 quantitative features, including shape, intensity, and texture features, with the toolkit. In total, 24 shape features described the 3D geometric properties of the tumor. A further 37 intensity features estimated the first-order statistics of the intensity histogram. Moreover, the intra-tumor heterogeneity was quantified by 106 textural features derived from the gray-level co-occurrence matrix (GLCM), gray-level run-length matrix (GLRLM), gray-level size zone matrix (GLSZM), spatial gray-level dependence matrix (SGLDM), neighborhood gray-tone difference matrix (NGTDM), and neighborhood gray-level difference statistics (NGLDS).
Prior to the computation of the textural features, the full intensity range of the tumor region was quantified to a smaller number of gray levels (Ng). The pixel value in a gray-scale image is usually an integer between 0 and 255. If Ng =8 is chosen, the pixel value is quantitatively mapped to an integer between 0 and 7, which can greatly reduce the burden of calculation in feature extraction. The Uniform, equal-probability, and Lloyd-Max quantization algorithms were tested using our quantitative image feature kit. The calculation formula of the Uniform quantization algorithm was:
[1]
where ‘round’ represents the rounding function, ‘x’ refers to the original tumor region voxel value, xmax and xmax are the maximum and minimum voxel values of the original tumor region, respectively, and y is the result (1–Ng) after quantization. The equal-probability and Lloyd-Max quantization algorithms were calculated using the histeq and lloyds functions of MATLAB, respectively. Ng can theoretically be set to any integer value in all three quantization algorithms. All previous radiomics studies related to the prediction of HCC MVI, only Zhang et al. (34), reported the value of Ng is 64 and selected the Uniform quantization algorithm as the quantization algorithm, while other studies (26-33) failed to specify the value of Ng or the quantization algorithm. In our study, we tested the three quantization algorithms and commonly used Ng values, including 8, 16, 32, 64, and 128.
Radiomics feature selection
There are three main types of feature selection methods: filter, wrapper, and embedded (37). Minimum redundancy maximum relevance (mRMR) (38), support vector machine (SVM) recursive feature elimination (RFE) (39), and least absolute shrinkage and selection operator (LASSO) (40) algorithms are common filter, wrapper, and embedded methods for feature selection, respectively. Of the radiomics studies related to HCC MVI prediction, we found that only two used SVM-RFE or mRMR as the feature selection method (29,34), and the others used LASSO (26,28,30,32,33). Our study tested the performance of the feature selection methods, including SVM-RFE, mRMR, and LASSO, in HCC MVI prediction. In addition, we present a two-stage feature selection method combining LASSO and the gradient boosting decision tree RFE (GBDT-RFE) (LASSO-RFE). The workflow of the LASSO-RFE is shown in Figure 3. With a 10-fold cross-validation strategy, LASSO was applied to obtain the feature subsets in the training set; next, the GBDT-RFE was used to sort the feature subsets. A backward search algorithm was used to verify the performance of different numbers of feature combinations in the validation set, from which the feature subset with the best performance was selected as the optimal feature subset. Finally, the maximal information coefficient (MIC) was used to calculate the correlation in the optimal feature subset for 10 folds and remove the redundant features that had a strong correlation (MIC >0.5) with the features with higher frequency.
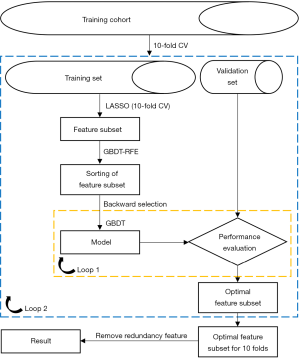
Classifiers and radiomics model building
Almost all of the previous radiomics studies about HCC MVI prediction used logistic regression (LR) as a classifier (29-30,32-34). Moreover, as SVM, random forest (RF), and GBDT are machine-learning classifiers commonly used in similar radiomics research, we explored the differences in performance between these four classifiers for the prediction of HCC MVI. In our study, LR, SVM, RF, and GBDT were implemented in the scikit-learn Python (version 3.7) library.
To train and test the model, we used the leave-one-out cross-validation (LOOCV) strategy, with one subject for testing and the remaining subjects for training. The data were divided into a training cohort and a testing cohort using the LOOCV strategy. In the training cohort, the best feature subset was first obtained by feature selection, and then the feature values of the best feature subset were standardized:
[2]
where x is the original feature value, µ and σ are the mean and standard deviation values of the original eigenvectors, respectively, and y is the standardized feature value. In the testing cohort, the corresponding features were standardized in the same way; however, it should be noted that µ and σ referred to the mean and standard deviation values of the original eigenvectors in the training cohort. Finally, the model was trained and tested using the training and testing cohorts, respectively. The above steps were repeated until each sample had been tested once as the testing cohort.
Study design and performance evaluation
The workflow of the study is shown in Figure 4. We chose 1.19×1.19×1.19 mm3, 64, Uniform, LASSO, and LR as the initial settings for the resampled voxel size, Ng, the quantization algorithm, feature selection method, and classifier, respectively, because they have been the most commonly used in other radiomics studies. In experiment 1, we aimed to explore the prediction performance differences between the pre-T1WI, AP, PVP, and HBP sequences; the experiment was carried out with the initial settings. In subsequent experiments, we used the control variable strategy, and each experiment setting was based on the variables that obtained the best predictive performance from the previous experiment. Furthermore, we explored the effects of resampled voxel size, Ng, quantization algorithm, feature selection method, and classifiers on the performance of predictive models in experiments 2, 3, 4, 5 and 6, respectively.
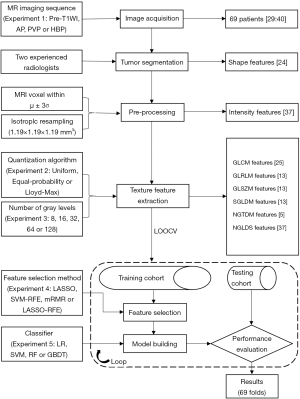
The performance of the predictive models was evaluated using the receiver operating characteristic (ROC) curve. The area under the ROC curve (AUC) with 95% confidence intervals (95% CI) was calculated, because it denotes the classification performance across all decision thresholds. From the ROC curve, the accuracy, sensitivity, and specificity were calculated according to the decision threshold with the highest accuracy. In our study, the stability of the feature selection method using the cross-validation strategy was defined as:
[3]
where Fi and Fj are the feature selection results of the i-fold and j-fold, respectively, and K represents the total number of folds. The stability value ranged between 0 and 1. If the feature selection results of each fold were consistent, the stability value would be 1.
Statistical analysis
The Mann-Whitney U test was used to compare the clinical characteristics between MVI-present and MVI-absent cohorts for continuous variables, and the Chi-square test was used for categorical variables. To evaluate the differences in the predictive performance of the different models, the ROC curves of all models were compared using MedCalc software (version 12.7.0). Other statistical analyses were performed in the scipy-stats Python (version 3.7) library. Two-tailed P values <0.05 were considered statistically significant.
Results
Demographic and clinical characteristics
The demographic and clinical characteristics of the patients are listed in Table 2. All patients had hepatitis, and 28 had accompanying cirrhosis.
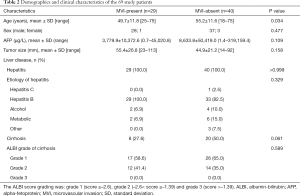
Full table
Prediction performance of the models
Experiment 1
With the settings of resampled voxel size, quantization algorithm, Ng, feature selection method, and classifier as 1.19×1.19×1.19 mm3, Uniform, 64, LASSO, and LR, respectively, the performance of the predictive models built using the AP, PVP, pre-T1WI, and HBP images are listed in Table 3. The ROC curves are shown in Figure 5. The model based on the radiomics features of HBP images displayed a better predictive performance for HCC MVI than the radiomics features of AP, PVP, and pre-T1WI (AUC =0.792 vs. 0.641/0.634/0.620, respectively). The P values of the differences between the HBP ROC curve and the AP, PVP, and pre-T1WI curves were 0.041, 0.021, and 0.010, respectively.
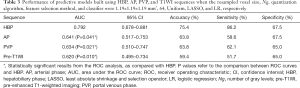
Full table
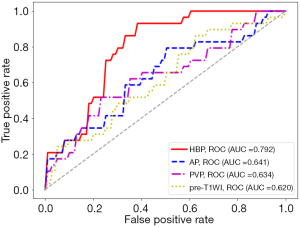
Experiment 2
With the settings of Ng, quantization algorithm, feature selection method, and classifier as 64, Uniform, LASSO, and LR, respectively, details of the performance of the predictive models built using the HBP radiomics features obtained with different resampled voxel sizes are listed in Table 4. The ROC curves are shown in Figure 6. Compared with the images of 1.50×1.50×1.50 and 2.00×2.00×2.00 mm3, the radiomics features obtained with images of 1.00×1.00×1.00 or 1.19×1.19×1.19 mm3 displayed a better performance (AUC =0.792/0.792 vs. 0.639/0.613, P=0.010 and 0.001, respectively). The radiomics features obtained using the 1.193 mm3 resampled voxel size had a higher sensitivity than those obtained using 1.003 mm3 (sensitivity =86.2% vs. 75.9%).
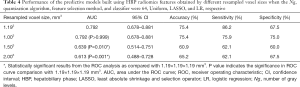
Full table
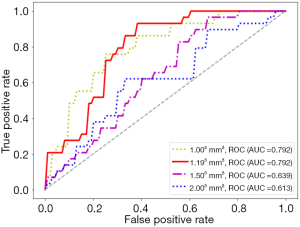
Experiment 3
With the settings of resampled voxel size, Ng, feature selection method, and classifier as 1.19×1.19×1.19 mm3, 64, LASSO, and LR, respectively, details of the performance of the predictive models built using the HBP radiomics features obtained with the Uniform, Lloyd-Max, and equal-pro quantization algorithms are listed in Table 5. The ROC curves are shown in Figure 7. Compared with the equal-probability and Lloyd-Max algorithms, the radiomics features obtained using the Uniform quantization algorithm had a better performance (AUC =0.792 vs. 0.643/0.666, respectively). The P values for the differences between the Uniform ROC curves and the Lloyd-Max and equal-pro curves were 0.002 and 0.003, respectively.
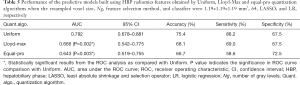
Full table
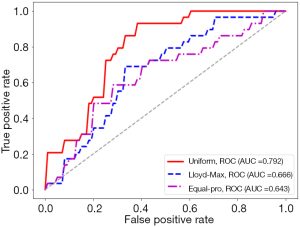
Experiment 4
With the settings of resampled voxel size, quantization algorithm, feature selection method, and classifier as 1.19×1.19×1.19 mm3, Uniform, LASSO, and LR, respectively, details of the performance of the predictive models built using the HBP radiomics features obtained with different Ngs, including 8, 16, 32, 64, and 128, are listed in Table 6. The ROC curves are shown in Figure 8. Among the values of 8, 16, 32, 64, and 128, the best HCC MVI predictive performance was obtained when Ng was 64 (AUC =0.792 vs. 0.584/0.697/0.677/0.734, respectively). The P values of the differences between the ROC curves with a Ng of 64 and the curves with a Ng of 8, 16, 32, and 128 were <0.001, 0.039, 0.001, and 0.137, respectively.
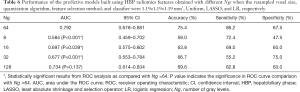
Full table
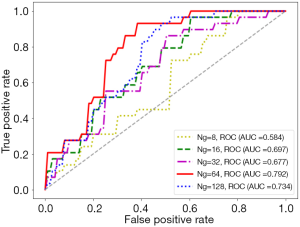
Experiment 5
With the settings of resampled voxel size, Ng, quantization algorithm, and the classifier as 1.19×1.19×1.19 mm3, 64, Uniform, and LR, respectively, details of the performance of the predictive models built using the HBP radiomics features obtained by different feature selection methods, including LASSO-RFE, LASSO, mRMR, and SVM-RFE, are listed in Table 7. Among these feature selection methods, the radiomics features selected by LASSO-RFE showed better stability and predictive performance than LASSO, mRMR, and SVM-RFE (stability =0.967 vs. 0.837/0.623/0.390, respectively; AUC =0.850 vs. 0.792/0.713/0.699, respectively) (Figure 9). The P values of the differences between the LASSO-RFE ROC curves and the LASSO, mRMR, and SVM-RFE curves were 0.142, 0.007, and 0.003, respectively.
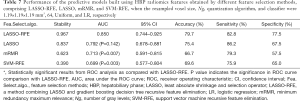
Full table
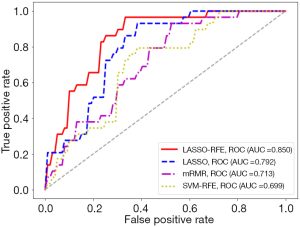
Experiment 6
With the settings of resampled voxel size, Ng, quantization algorithm, and feature selection method as 1.19×1.19×1.19 mm3, 64, Uniform, and LASSO-RFE, respectively, details of the performance of the predictive models established with SVM, LR, RF, and GBDT based on the HBP images are shown in Figure 10. The model based on the radiomics features of the HBP images using GBDT displayed a better performance than LR, SVM, and RF for the preoperative prediction of MVI (AUC =0.895 vs. 0.850/0.834/0.884, respectively). The P values of the differences between the GBDT ROC curves and the RF, LR, and SVM curves were 0.558, 0.229, and 0.058, respectively (Table 8).
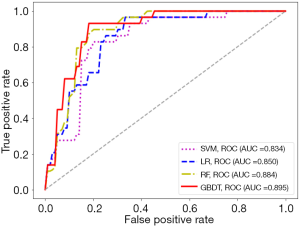
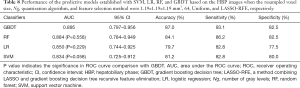
Full table
Feature selection results
With the LOOCV strategy, LASSO-RFE was used to select features in the training cohort. The selected features of 68 folds were solidity, range, high gray-level zone emphasis (HGLZE), and gray-level variance of GLSZM (GLSZM_GLV), while those of the remaining 1 fold were solidity, range, HGLZE, GLSZM_GLV, and maximum NGLDS (NGLDS_Max); the mean, standard deviation, and range (minimum–maximum) of each of these five features are listed in Table 9.
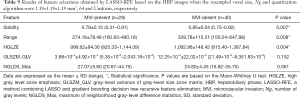
Full table
Discussion
In this study, the model based on the radiomics features of HBP images displayed a better predictive performance for HCC MVI than those based on the radiomics features of AP, PVP, and pre-T1WI images; moreover, the differences in performance were significant (P values =0.010–0.041). Our finding is in accordance with the conclusion of Yang et al. (33). Notably, the predictive model based on HBP had higher sensitivity than the other sequences. Gadoxetic acid is a liver-specific intracellular MRI contrast agent that provides enhanced hepatocellular parenchymal contrast. Other studies have reported that gadoxetic acid-enhanced MRI may aid in the diagnosis of HCC MVI (41,42), which has been confirmed by our research, and may occur because, in the presence of MVI, tumor thrombi obstruct the minute portal branches that supply the hepatocytes. Thus, the uptake of gadoxetic acid by hepatocytes will decrease, resulting in hemodynamic changes that are visible on imaging (43).
Our results showed that the feature extraction parameters (resampled voxel size, Ng, and discretization method) had a considerable effect on the predictive performance of models for HCC MVI. The best predictive performance was obtained when the resampled voxel size was 1.19×1.19×1.19 mm3, equivalent to the transverse plane resolution. A possible explanation is that compared with 1.00×1.00×1.00, 1.50×1.50×1.50, and 2.00×2.00×2.00, the resampled voxel size of 1.19×1.19×1.19 mm3 does not require interpolation in the transverse plane of MR images, which can reduce the image distortion caused by interpolation and is thus conducive to extracting the features correlated with MVI. Moreover, the greater the difference between the resampled voxel size and 1.19×1.19×1.19 mm3, the worse the performance. Compared with equal-probability and Lloyd-Max, the radiomics features obtained using the Uniform quantization algorithm had better performance. Furthermore, the performance differences were mainly reflected in sensitivity, which may be because the equal-pro and Lloyd-Max quantization algorithms change the dimensionless range of intensity, thereby discarding the gadoxetic acid uptake information (i.e., metabolic activity) that plays an important role in the diagnosis of MVI, as discussed earlier. Among the values of 8, 16, 32, 64, and 128, the best HCC MVI predictive performance was obtained when Ng was 64. There were significant differences (P<0.001–0.039) in predictive performance compared with Ng =8 or 16. However, when Ng was 128 (>64), a better predictive performance was not achieved, even if the difference of predictive performance between 64 and 128 was not significant (P=0.137). PET (44), CT (45), and MRI (46) studies have reported that radiomics features are extremely sensitive to the specific choice of Ng, which is similar to the findings of our study. Compared with previous studies, we focused on the change in the final performance rather than the variability of the radiomics feature values. Ng values of 8, 16, and 32 are likely too small, especially a Ng of 8, which has previously been criticized as being inadequate for textural analysis (44). However, using a Ng of 128 will lead to large and empty matrices (including GLCM, GLRLM, GLSZM, NGTDM, and SGLDM) that may influence results. Thus, the most robust choice for Ng is 64; previous studies have also shown that this value is more suitable for the extraction of radiomics features than 8, 16, 32, and 128 (44).
High-throughput radiomics features can easily lead to over-fitting (47), especially in small datasets such as ours. In this case, what the classifier learns may be data-dependent noise rather than MVI biomarkers, which greatly reduces the generalizability of the HCC MVI predictive model. In our study, to avoid the disadvantages of dimensionality, feature selection was used, and we used the LOOCV strategy to determine if over-fitting had occurred. Among the three commonly used feature selection methods (mRMR, SVM-RFE, and LASSO), the radiomics features selected by LASSO displayed the best stability and predictive performance, which may be because LASSO retains the good features of both the subset selection and ridge regression. LASSO is suitable for analyzing large sets of radiomics features with a relatively small sample size and is designed to avoid over-fitting, which helps to reduce the interference of data noise (irrelevant features) (48,49). We also presented a two-stage feature selection LASSO-RFE, which achieved better stability and performance than LASSO. After using LASSO to select a feature subset, RFE used a verification set to perform a secondary selection of feature subsets, which further eliminated irrelevant features. Similar methods using two-stage feature selection have been used to achieve better performance in previous radiomics studies (50,51).
In the preoperative prediction of MVI, the model based on the radiomics features of HBP images using GBDT displayed a better performance than those using LR, SVM, and RF; this is consistent with the findings of Ni et al. (31), who explored the best model for MVI prediction based on CT images (31). In fact, some radiomics studies have also reported that GBDT has a better performance than other classifiers, including LR, SVM, and RF (52,53). This may be because GBDT is an iterative algorithm based on a decision tree, which can improve performance by reducing model deviation in the iterative process. Nevertheless, no significant differences were found among the four classifiers (P values =0.058–0.558), which indicates that the predictive ability of the radiomics features found in our study for MVI does not depend largely on the classifier.
Using the LOOCV strategy, the solidity, range, HGLZE, and GLSZM_GLV were selected stably in all 69 folds, and achieved a good average predictive performance using classifiers including LR, SVM, RF, and GBDT (AUC =0.834–0.895). This may demonstrate the stability and robustness of the extracted radiomics features. Specifically, we found that the presence of HCC MVI resulted in lower values of solidity, range, HGLZE, and GLSZM_GLV. The possible reasons may be as follows. Solidity is derived by calculating the proportion of pixels of the tumor region to the largest possible convex hull polygon structure of the tumor region, and the convex hull is the best-fitting polygon that encloses all of the pixels in the tumor region. Solidity reflects the degree of irregularity of the tumor; the more irregular the tumor, the smaller the isolation value. Moreover, according to histologic examination, MVI-positive HCC has a tendency to aggressively invade the tumor capsule and protrude into the noncancerous parenchyma, thus often showing an irregular shape (54). HGLZE indicates the distribution of the high gray-level region; a smaller HGLZE value indicates that the high gray-level region occupies a lower proportion of the image. A previous study reported that lower signal intensities are more frequent in MVI-positive HCC than in MVI-negative HCC on HBP images using gadoxetic acid-enhanced MRI (55). Therefore, the lower values of HGLZE in MVI-positive patients are most likely caused by an uneven occurrence of MVI in the tumor region. GLSZM_GLV indicates the dispersion degree of gray levels in the tumor region, and the range indicates the difference between the maximum and minimum values of tumor signal intensity. The lower values of range and GLSZM_GLV in MVI-positive patients may be caused by intratumoral heterogeneity (e.g., micro-necrosis and inflammation), accompanying MVI (56).
To date, radiomics studies of MVI prediction have reported AUCs of 0.727–0.88 and 0.833–0.861 with CT (26-31) and MRI (32-34), respectively. By optimizing the combination of various impact factors that affect the radiomics process, the final MVI predictive model in our study achieved an AUC of 0.895, accuracy of 87.0%, sensitivity of 93.1%, and specificity of 82.5%. Despite our predictive model of HCC MVI showing a better performance than those in previous studies, it should be noted that because of the lack of standardization in radiomics and the diversity in research methods, it is difficult to compare the results of different studies. The majority of previous studies used a single method for their radiomics research; thus, their models may not achieve the maximum prediction performance, because many aspects of the radiomics process, including imaging sequence, feature extraction parameters, feature selection method, and classifiers, have a great effect on MVI predictive performance. Moreover, previous studies have given only limited consideration to the effect of these factors on MVI predictive performance. When the quantization algorithm, Ng, feature selection method, and classifier were Uniform, 64, LASSO-RFE, and GBDT, respectively, the performance of the predictive models built on HBP images achieved the best performance in our study. This combination of factors is not quite the same as the method that has been commonly used in previous studies on MVI prediction. The lack of standardization and unification is also a major problem facing radiomics (57), which limits its large-scale application for the diagnosis of diseases. Therefore, we also hope that our work can provide standardized references for subsequent radiomics research related to MVI prediction.
This study may have several limitations. Firstly, with regard to accurate tumor segmentation, radiomics features might provide a more complete characterization of the tumor; however, there is no gold standard for tumor contouring. Thus, we failed to explore the effect of tumor segmentation on MVI prediction. However, in our study, tumor lesion contouring was verified by experienced radiologists, so we believe that any variation in tumor lesion contouring had limited effects in this study. Secondly, the exact value of the best Ng for MVI prediction is not given in our study. There are currently no specific guidelines for choosing the optimal Ng; however, we strongly recommend a Ng of 64. Thirdly, we only tested the most commonly used method of feature extraction, in which the Ng is a single value, but the optimal feature sets may consist of different features produced by multiple Ngs. Finally, because we used the LOOCV strategy on a small number of samples from a single center, further verification with a larger, external cohort is warranted to obtain reliable results.
In summary, we showed that imaging sequence, feature extraction parameters, feature selection method, and classifiers all affect the performance of radiomics models in predicting MVI. When the imaging sequence, quantization algorithm, Ng, feature selection method, and classifier were HBP, Uniform, 64, LASSO-RFE, and GBDT, respectively, the performance of the predictive models was better than those based on other combinations. The effect of different factors should be considered in future similar radiomics studies.
Acknowledgments
Funding: This work was funded by the National Natural Science Foundation of China (No. 81971684, No. 81771908, No. 61973220), Guangdong Basic and Applied Basic Research Foundation (No. 2020A1515010571), Jiangxi provincial leading scholar program (No. 20204BCJ22011), Guangzhou Science and Technology Planning Project (No. 201903010073), Shenzhen University Top Ranking Project (No. 860/000002100108).
Footnote
Conflicts of Interest: All authors have completed the ICMJE uniform disclosure form (available at http://dx.doi.org/10.21037/qims-20-218). The authors have no conflicts of interest to declare.
Ethical Statement: The study was conducted in accordance with the Declaration of Helsinki (as revised in 2013). The study was approved by the Institutional Review Board and Ethical Committee of The First Affiliated Hospital, Sun Yat-sen University {no. [2017]258} and individual consent for this retrospective analysis was waived.
Open Access Statement: This is an Open Access article distributed in accordance with the Creative Commons Attribution-NonCommercial-NoDerivs 4.0 International License (CC BY-NC-ND 4.0), which permits the non-commercial replication and distribution of the article with the strict proviso that no changes or edits are made and the original work is properly cited (including links to both the formal publication through the relevant DOI and the license). See: https://creativecommons.org/licenses/by-nc-nd/4.0/.
References
- Torre LA, Bray F, Siegel RL, Ferlay J, Lortet-Tieulent J, Jemal A. Global cancer statistics, 2012. CA Cancer J Clin 2015;65:87-108. [Crossref] [PubMed]
- Earl TM, Chapman WC. Hepatocellular carcinoma: resection versus transplantation. Semin Liver Dis 2013;33:282-92. [Crossref] [PubMed]
- Fong Y, Sun RL, Jarnagin W, Blumgart LH. An analysis of 412 cases of hepatocellular carcinoma at a Western center. Ann Surg 1999;229:790-9; discussion 799-800. [Crossref] [PubMed]
- Grazi GL, Ercolani G, Pierangeli F, Del Gaudio M, Cescon M, Cavallari A, Mazziotti A. Improved results of liver resection for hepatocellular carcinoma on cirrhosis give the procedure added value. Ann Surg 2001;234:71-8. [Crossref] [PubMed]
- Lim KC, Chow PK, Allen JC, Chia GS, Lim M, Cheow PC, Chung AY, Ooi LL, Tan SB. Microvascular invasion is a better predictor of tumor recurrence and overall survival following surgical resection for hepatocellular carcinoma compared to the Milan criteria. Ann Surg 2011;254:108-13. [Crossref] [PubMed]
- Gouw AS, Balabaud C, Kusano H, Todo S, Ichida T, Kojiro M. Markers for microvascular invasion in hepatocellular carcinoma: where do we stand? Liver Transpl 2011;17 Suppl 2:S72-80. [Crossref] [PubMed]
- Sumie S, Kuromatsu R, Okuda K, Ando E, Takata A, Fukushima N, Watanabe Y, Kojiro M, Sata M. Microvascular invasion in patients with hepatocellular carcinoma and its predictable clinicopathological factors. Ann Surg Oncol 2008;15:1375-82. [Crossref] [PubMed]
- Kim H, Park MS, Park YN, Kim H, Kim KS, Choi JS, Ahn SH, Han KH, Kim MJ, Kim KW. Preoperative radiologic and postoperative pathologic risk factors for early intra-hepatic recurrence in hepatocellular carcinoma patients who underwent curative resection. Yonsei Med J 2009;50:789-95. [Crossref] [PubMed]
- Shah SA, Greig PD, Gallinger S, Cattral MS, Dixon E, Kim RD, Taylor BR, Grant DR, Vollmer CM. Factors associated with early recurrence after resection for hepatocellular carcinoma and outcomes. J Am Coll Surg 2006;202:275-83. [Crossref] [PubMed]
- Banerjee S, Wang DS, Kim HJ, Sirlin CB, Chan MG, Korn RL, Rutman AM, Siripongsakun S, Lu D, Imanbayev G, Kuo MD. A computed tomography radiogenomic biomarker predicts microvascular invasion and clinical outcomes in hepatocellular carcinoma. Hepatology 2015;62:792-800. [Crossref] [PubMed]
- Renzulli M, Brocchi S, Cucchetti A, Mazzotti F, Mosconi C, Sportoletti C, Brandi G, Pinna AD, Golfieri R. Can current preoperative imaging be used to detect microvascular invasion of hepatocellular carcinoma? Radiology 2016;279:432-42. [Crossref] [PubMed]
- Lee S, Kim SH, Lee JE, Sinn DH, Park CK. Preoperative gadoxetic acid-enhanced MRI for predicting microvascular invasion in patients with single hepatocellular carcinoma. J Hepatol 2017;67:526-34. [Crossref] [PubMed]
- Lambin P, Rios-Velazquez E, Leijenaar R, Carvalho S, van Stiphout RG, Granton P, Zegers CM, Gillies R, Boellard R, Dekker A, Aerts HJ. Radiomics: extracting more information from medical images using advanced feature analysis. Eur J Cancer 2012;48:441-6. [Crossref] [PubMed]
- Kumar V, Gu Y, Basu S, Berglund A, Eschrich SA, Schabath MB, Forster K, Aerts HJ, Dekker A, Fenstermacher D, Goldgof DB, Hall LO, Lambin P, Balagurunathan Y, Gatenby RA, Gillies RJ. Radiomics: the process and the challenges. Magn Reson Imaging 2012;30:1234-48. [Crossref] [PubMed]
- Gillies RJ, Kinahan PE, Hricak H. Radiomics: images are more than pictures, they are data. Radiology 2016;278:563-77. [Crossref] [PubMed]
- Mackin D, Fave X, Zhang L, Fried D, Yang J, Taylor B, Rodriguez-Rivera E, Dodge C, Jones AK, Court L. Measuring computed tomography scanner variability of radiomics features. Invest Radiol 2015;50:757-65. [Crossref] [PubMed]
- Zhao B, Tan Y, Tsai WY, Schwartz LH, Lu L. Exploring variability in ct characterization of tumors: a preliminary phantom study. Transl Oncol 2014;7:88-93. [Crossref] [PubMed]
- He L, Huang Y, Ma Z, Liang C, Liang C, Liu Z. Effects of contrast-enhancement, reconstruction slice thickness and convolution kernel on the diagnostic performance of radiomics signature in solitary pulmonary nodule. Sci Rep 2016;6:34921. [Crossref] [PubMed]
- Galavis PE, Hollensen C, Jallow N, Paliwal B, Jeraj R. Variability of textural features in FDG PET images due to different acquisition modes and reconstruction parameters. Acta Oncol 2010;49:1012-6. [Crossref] [PubMed]
- Parmar C, Rios Velazquez E, Leijenaar R, Jermoumi M, Carvalho S, Mak RH, Mitra S, Shankar BU, Kikinis R, Haibe-Kains B, Lambin P, Aerts HJ. Robust Radiomics feature quantification using semiautomatic volumetric segmentation. PLoS One 2014;9:e102107. [Crossref] [PubMed]
- Velazquez ER, Parmar C, Jermoumi M, Mak RH, van Baardwijk A, Fennessy FM, Lewis JH, De Ruysscher D, Kikinis R, Lambin P, Aerts HJ. Volumetric CT-based segmentation of NSCLC using 3D-Slicer. Sci Rep 2013;3:3529. [Crossref] [PubMed]
- Echegaray S, Gevaert O, Shah R, Kamaya A, Louie J, Kothary N, Napel S. Core samples for radiomics features that are insensitive to tumor segmentation: method and pilot study using CT images of hepatocellular carcinoma. J Med Imaging (Bellingham) 2015;2:041011. [Crossref] [PubMed]
- Parmar C, Grossmann P, Bussink J, Lambin P, Aerts HJWL. Machine learning methods for quantitative radiomic biomarkers. Sci Rep 2015;5:13087. [Crossref] [PubMed]
- Parmar C, Grossmann P, Rietveld D, Rietbergen MM, Lambin P, Aerts HJ. Radiomic machine-learning classifiers for prognostic biomarkers of head and neck cancer. Front Oncol 2015;5:272. [Crossref] [PubMed]
- Leijenaar RT, Nalbantov G, Carvalho S, van Elmpt WJ, Troost EG, Boellaard R, Aerts HJ, Gillies RJ, Lambin P. The effect of SUV discretization in quantitative FDG-PET Radiomics: the need for standardized methodology in tumor texture analysis. Sci Rep 2015;5:11075. [Crossref] [PubMed]
- Bakr S, Echegaray S, Shah R, Kamaya A, Louie J, Napel S, Kothary N, Gevaert O. Noninvasive radiomics signature based on quantitative analysis of computed tomography images as a surrogate for microvascular invasion in hepatocellular carcinoma: a pilot study. J Med Imaging (Bellingham) 2017;4:041303. [Crossref] [PubMed]
- Zheng J, Chakraborty J, Chapman WC, Gerst S, Gonen M, Pak LM, Jarnagin WR, DeMatteo RP, Do RKG, Simpson AL. Hepatopancreatobiliary Service in the Department of Surgery of the Memorial Sloan Kettering Cancer Center. Research Staff in the Department of Surgery at Washington University School of Medicine. Preoperative Prediction of Microvascular Invasion in Hepatocellular Carcinoma Using Quantitative Image Analysis. J Am Coll Surg 2017;225:778-88.e1. [Crossref] [PubMed]
- Peng J, Zhang J, Zhang Q, Xu Y, Zhou J, Liu L. A radiomics nomogram for preoperative prediction of microvascular invasion risk in hepatitis B virus-related hepatocellular carcinoma. Diagn Interv Radiol 2018;24:121-7. [Crossref] [PubMed]
- Xu X, Zhang HL, Liu QP, Sun SW, Zhang J, Zhu FP, Yang G, Yan X, Zhang YD, Liu XS. Radiomic analysis of contrast-enhanced CT predicts microvascular invasion and outcome in hepatocellular carcinoma. J Hepatol 2019;70:1133-44. [Crossref] [PubMed]
- Ma X, Wei J, Gu D, Zhu Y, Feng B, Liang M, Wang S, Zhao X, Tian J. Preoperative radiomics nomogram for microvascular invasion prediction in hepatocellular carcinoma using contrast-enhanced CT. Eur Radiol 2019;29:3595-605. [Crossref] [PubMed]
- Ni M, Zhou X, Lv Q, Li Z, Gao Y, Tan Y, Liu J, Liu F, Yu H, Jiao L, Wang G. Radiomics models for diagnosing microvascular invasion in hepatocellular carcinoma: which model is the best model? Cancer Imaging 2019;19:60. [Crossref] [PubMed]
- Feng ST, Jia Y, Liao B, Huang B, Zhou Q, Li X, Wei K, Chen L, Li B, Wang W, Chen S, He X, Wang H, Peng S, Chen ZB, Tang M, Chen Z, Hou Y, Peng Z, Kuang M. Preoperative prediction of microvascular invasion in hepatocellular cancer: a radiomics model using Gd-EOB-DTPA-enhanced MRI. Eur Radiol 2019;29:4648-59. [Crossref] [PubMed]
- Yang L, Gu D, Wei J, Yang C, Rao S, Wang W, Chen C, Ding Y, Tian J, Zeng M. A radiomics nomogram for preoperative prediction of microvascular invasion in hepatocellular carcinoma. Liver Cancer 2019;8:373-86. [Crossref] [PubMed]
- Zhang R, Xu L, Wen X, Zhang J, Yang P, Zhang L, Xue X, Wang X, Huang Q, Guo C, Shi Y, Niu T, Chen F. A nomogram based on bi-regional radiomics features from multimodal magnetic resonance imaging for preoperative prediction of microvascular invasion in hepatocellular carcinoma. Quant Imaging Med Surg 2019;9:1503-15. [Crossref] [PubMed]
- Collewet G, Strzelecki M, Mariette F. Influence of MRI acquisition protocols and image intensity normalization methods on texture classification. Magn Reson Imaging 2004;22:81-91. [Crossref] [PubMed]
- Vallières M, Freeman CR, Skamene SR, El Naqa I. A radiomics model from joint FDG-PET and MRI texture features for the prediction of lung metastases in soft-tissue sarcomas of the extremities. Phys Med Biol 2015;60:5471-96. [Crossref] [PubMed]
- Haury AC, Gestraud P, Vert JP. The influence of feature selection methods on accuracy, stability and interpretability of molecular signatures. PLoS One 2011;6:e28210. [Crossref] [PubMed]
- Peng H, Long F, Ding C. Feature selection based on mutual information: criteria of max-dependency, max-relevance, and min-redundancy. IEEE Trans Pattern Anal Mach Intell 2005;27:1226-38. [Crossref] [PubMed]
- Guyon I, Weston J, Barnhill S, Vapnik V. Gene selection for cancer classification using support vector machines. Machine Learning 2002;46:389-422. [Crossref]
- Tibshirani R. Regression shrinkage and selection via the lasso. J R Stat Soc Series B Stat Methodol 1996;58:267-88.
- Kim MJ, Lee M, Choi JY, Park YN. Imaging features of small hepatocellular carcinomas with microvascular invasion on gadoxetic acid-enhanced MR imaging. Eur J Radiol 2012;81:2507-12. [Crossref] [PubMed]
- Huang M, Liao B, Xu P, Cai H, Huang K, Dong Z, Xu L, Peng Z, Luo Y, Zheng K, Peng B, Li ZP, Feng ST. Prediction of microvascular invasion in hepatocellular carcinoma: preoperative Gd-EOB-DTPA-dynamic enhanced MRI and histopathological correlation. Contrast Media Mol Imaging 2018;2018:9674565. [Crossref] [PubMed]
- Kim KA, Kim MJ, Jeon HM, Kim KS, Choi JS, Ahn SH, Cha SJ, Chung YE. Prediction of microvascular invasion of hepatocellular carcinoma: usefulness of peritumoral hypointensity seen on gadoxetate disodium-enhanced hepatobiliary phase images. J Magn Reson Imaging 2012;35:629-34. [Crossref] [PubMed]
- Tixier F, Hatt M, Le Rest CC, Le Pogam A, Corcos L, Visvikis D. Reproducibility of tumor uptake heterogeneity characterization through textural feature analysis in 18F-FDG PET. J Nucl Med 2012;53:693-700. [Crossref] [PubMed]
- Shafiq-Ul-Hassan M, Zhang GG, Latifi K, Ullah G, Hunt DC, Balagurunathan Y, Abdalah MA, Schabath MB, Goldgof DG, Mackin D, Court LE, Gillies RJ, Moros EG. Intrinsic dependencies of CT radiomic features on voxel size and number of gray levels. Med Phys 2017;44:1050-62. [Crossref] [PubMed]
- Duron L, Balvay D, Vande Perre S, Bouchouicha A, Savatovsky J, Sadik JC, Thomassin-Naggara I, Fournier L, Lecler A. Gray-level discretization impacts reproducible MRI radiomics texture features. PLoS One 2019;14:e0213459. [Crossref] [PubMed]
- Spruyt V. The Curse of Dimensionality in classification. Computer Vision for Dummies 2014;21:35-40.
- Gui J, Li H. Penalized Cox regression analysis in the high-dimensional and low-sample size settings, with applications to microarray gene expression data. Bioinformatics 2005;21:3001-8. [Crossref] [PubMed]
- Hepp T, Schmid M, Gefeller O, Waldmann E, Mayr A. Approaches to regularized regression - a comparison between gradient boosting and the lasso. Methods Inf Med 2016;55:422-30. [Crossref] [PubMed]
- Zhang Y, Ding C, Li T. Gene selection algorithm by combining reliefF and mRMR. BMC Genomics 2008;9 Suppl 2:S27. [Crossref] [PubMed]
- Wu W, Parmar C, Grossmann P, Quackenbush J, Lambin P, Bussink J, Mak R, Aerts HJ. Exploratory study to identify radiomics classifiers for lung cancer histology. Front Oncol 2016;6:71. [Crossref] [PubMed]
- Ypsilantis PP, Siddique M, Sohn HM, Davies A, Cook G, Goh V, Montana G. Predicting response to neoadjuvant chemotherapy with PET imaging using convolutional neural networks. PLoS One 2015;10:e0137036. [Crossref] [PubMed]
- Liao X, Cai B, Tian B, Luo Y, Song W, Li Y. Machine-learning based radiogenomics analysis of MRI features and metagenes in glioblastoma multiforme patients with different survival time. J Cell Mol Med 2019;23:4375-85. [Crossref] [PubMed]
- Hu H, Zheng Q, Huang Y, Huang XW, Lai ZC, Liu J, Xie X, Feng ST, Wang W, Lu M. A non-smooth tumor margin on preoperative imaging assesses microvascular invasion of hepatocellular carcinoma: a systematic review and meta-analysis. Sci Rep 2017;7:15375. [Crossref] [PubMed]
- Kim JY, Kim MJ, Kim KA, Jeong HT, Park YN. Hyperintense HCC on hepatobiliary phase images of gadoxetic acid-enhanced MRI: correlation with clinical and pathological features. Eur J Radiol 2012;81:3877-82. [Crossref] [PubMed]
- Wang WT, Yang L, Yang ZX, Hu XX, Ding Y, Yan X, Fu CX, Grimm R, Zeng MS, Rao SX. Assessment of microvascular invasion of hepatocellular carcinoma with diffusion kurtosis imaging. Radiology 2018;286:571-80. [Crossref] [PubMed]
- Yip SS, Aerts HJ. Applications and limitations of radiomics. Phys Med Biol 2016;61:R150-66. [PubMed]