Quantitative assessment of acquisition imaging parameters on MRI radiomics features: a prospective anthropomorphic phantom study using a 3D-T2W-TSE sequence for MR-guided-radiotherapy
Introduction
Radiomics (1-5) holds great promises in clinical oncology to aid better diagnosis, prognosis, and personalized clinical decision making, as witnessed by a huge number of research studies published in recent years (6-13). However, the broad validity and generality of radiomics are much hindered by the concerns on its reliability (14-20). Besides disease, tissue, and physiological characteristics in the patients, variability and uncertainty of radiomics can be introduced in many procedures of the complicated workflow. These procedures include but not limited to imaging hardware configuration, patient setup, image acquisition, reconstruction, image post-processing (filtering, segmentation and registration etc.), radiomics feature computation, and radiomics modeling.
Image acquisition is one of the key procedures that could substantially influence clinical image characteristics and thus radiomics reliability, no matter for computerized tomography (CT), magnetic resonance imaging (MRI), ultrasound or positron emission tomography (PET). Different from the quantitative nature of CT and PET, anatomical MRI has no exact physical meaning in its voxel value. Rather, MRI voxel value (or intensity) is comprehensively influenced by a number of endogenous and exogenous factors. The endogenous factors include the tissue properties such as proton density, T1/T2 relaxation times, fat-water composition, tissue susceptibility, and physiological motion. The exogenous factors include but not limited to MRI scanner field strength and homogeneity, radiofrequency (RF) coil, scan setup, MRI pulse sequences and imaging parameters, MRI reconstruction algorithms, as well as contrast agent administration. Among all clinical imaging modalities, MRI has the most flexible and superior soft tissue image contrasts. However, on the other hand, flexibility and superiority of MRI image contrast also impose even greater challenges on radiomics reliability in MRI than in CT and PET, in that MRI image intensity subjects much more to the greater variability in imaging acquisition by various MRI pulse sequences and imaging parameters.
Phantom study is a valuable and useful means to gauge the radiomics reliability, in which the radiomics feature reference could be well defined, and the contributing factors could be well controlled (4). Compared to CT and PET, dependence of radiomics features on MRI acquisition parameters has been much less investigated. Collewet et al. (21) was the first to investigate the effects of two MRI acquisition protocols and intensity normalization on texture classification using different-aged cheeses at 0.2 Tesla (T). Mayerhoefer et al. (22) studied the influence of varying TE, TR, number-of-average, and receiver bandwidth (RBW) on a number of texture features and pattern discrimination between two phantoms consisting of polystyrene spheres and an agar gel solution using a 2D multi-echo spin-echo sequence at 3T. In the era of radiomics, to the best of our knowledge, Buch et al. (23) was the only one to evaluate the influence of MRI scanning parameters on radiomics feature values. Based on publicly available phantom data, they retrospectively evaluated the effects of magnet strength, flip‐angle, number of excitations (NEX), and scanner platform in a gradient echo pulse sequence, on texture radiomics features. All these studies utilized non-anatomical phantoms and investigated a limited number of texture features and imaging parameters. Meanwhile, the utilization of post-acquisition image processing, such as grayscale normalization and spatial-resolution resampling, might obscure the effects of image acquisition on radiomics features.
This study aims to prospectively and quantitatively investigate the impact of various MRI imaging parameters on Imaging Biomarkers Standardization Initiative (IBSI) (24,25) compliant radiomics features using an anthropomorphic phantom. In addition, this study is particularly aiming for MRI radiomics in MR-guided radiotherapy (MRgRT) applications (26-31). Thus, a 3D T2-weighted (T2W) turbo-spin-echo (TSE) sequence standardized on a product 1.5 Tesla MRI-integrated linear accelerator (MR-LINAC) platform (32-34) is utilized to demonstrate the variation of radiomics feature values influenced by acquisition imaging parameters.
Methods
Phantom and MRI acquisition
No ethical approval or written informed consent was required for this phantom study. Imaging was conducted on a 70 cm-bored 1.5 T clinical MRI scanner (Ingenia MR-RT, Philips Healthcare, Best, Netherlands) with the maximum gradient strength of 45 mT/m and the switching rate of 200 T/m/s. A CIRS triple-modality (CT, MRI, and ultrasound) 3D Abdominal Phantom (model 057A, CIRS, Norfolk, Virginia, USA) with the size of 26 cm (LR) × 19 cm (AP) × 12.5 cm (SI) was used (https://www.cirsinc.com/products/ultrasound/zerdine-hydrogel/triple-modality-3d-abdominal-phantom/). The phantom consisted of several anatomical structures, including the liver with six lesions inside, the portal vein, two kidneys, partial lung, the abdominal aorta, the vena cava, a vertebra, and six ribs, to mimic human abdomen.
The phantom was aligned on the MRI flat couch-top using an external 3D laser (DORADOnova MR3T, LAP GmbH Laser Applikationen, Luneburg, Germany). A 16-channel FlexCoverage Anterior Coil held on a coil frame with a fixed distance to the phantom was used for MRI signal reception, along with the posterior 12-channel TotalSpine coil. The scanner integrated quadrature body coil (QBC) was used for signal excitation.
T1 and T2 relaxation times of the phantom were first measured using the vendor-provided T1 and T2 mapping protocols in the clinical sequence library. T1 mapping protocol was based on a dual flip angle (DFA) method using a 3D spoiled gradient echo sequence (FOV =30 cm; matrix size =256×256×40; voxel size =1.17×1.17×3 mm3; TE/TR =1.46/15 ms; flip angles =5°/26°; receiver bandwidth =434 Hz/pixel) (35,36). T2 mapping protocol was based on the conventional exponential decay model fitting using a 2D multi-echo spin-echo sequence (FOV =30 cm; matrix size =256×256; slices =40; slice thickness =3 mm; TEs =13.8/27.6/41.4/55.2/69.0 ms; flip angle =90°; refocusing angle =180° receiver bandwidth =227 Hz/pixel) (37,38). Voxel-wise T1 and T2 maps were automatically generated on the MRI console after imaging acquisition.
A 3D-T2W-TSE sequence was used for image acquisition for radiomics analysis. The baseline imaging protocol was adopted from the abdominal T2W scan protocol on a hybrid 1.5T MR-LINAC (Elekta Unity, Elekta Instrument AB, Stockholm, Sweden) (32-34). The imaging parameters, as listed in Table 1, were identical to that were standardized and clinically used on Elekta Unity for daily MRI scan of abdominal MRgRT for on-line treatment adaptation. But the major difference was the different hardware configuration, such as gradient coil and RF coils, between the Ingenia MR-RT and the Elekta Unity. A number of acquisition imaging parameters that could theoretically affect image intensity and contrast were adjusted on the basis of the baseline imaging protocol within a reasonably large range to assess their quantitative influence on radiomics feature values. These imaging parameters included echo time (TE), repetition time (TR), echo train length (ETL, or turbo factor), phase encoding (PE) direction, turbo direction, number of startup RF pulse, driven equilibrium, RF train flip angle pattern, and partial Fourier factor. They were generally independent of each other. Only one parameter was adjusted each time while other parameters were kept identical unless specified, such that the influence of each parameter on the radiomics features could be individually evaluated. Signal-to-noise ratio (SNR) normalization by increasing the number of signal average (NSA) was conducted to compensate for the SNR reduction introduced by partial Fourier acquisition, according to the well-known proportional relationship of image SNR with the square root of NSA, and with the square root of partial Fourier factor. The main purpose of SNR normalization was to ensure that the change of radiomics feature value was resulted from the image intensity or contrast change, instead of the varying noise level. The information on image parameters and their adjustment ranges were summarized in Table 2.
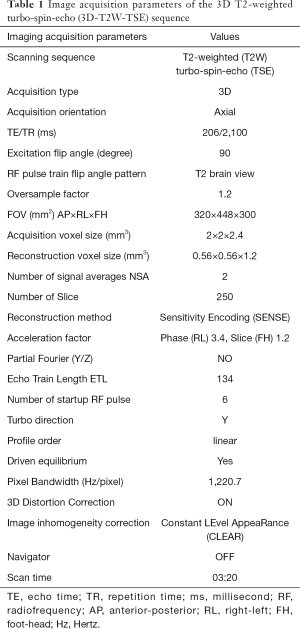
Full table
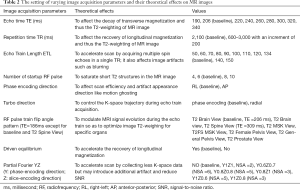
Full table
Feature extraction, image analysis, and statistical analysis
The DICOM-formatted MRI images were imported and converted into NRRD (nearly raw raster data) format in the 3D Slicer v 4.10.2 (39) without any other post-acquisition image processing. Ten volumes-of-interest (VOIs), including the liver, three liver lesions, left and right kidneys, vena cava, aorta, spinal cord and muscle, were segmented by an MRI physicist on the baseline MRI dataset (Figure 1), using the semi-automated “level tracing” function in the 3D slicer “Segment Editor”, or placing a spherical VOI within the tissue (including the liver and muscle VOIs). The segmented VOIs were straightforwardly propagated to all image datasets without needing image co-registration.
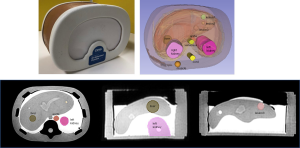
Radiomics features were extracted using PyRadiomics (v.2.2.0) (40), in which most original radiomics features were compliant with the standardized IBSI definition (24,25). A fixed bin size of 25 was used for image intensity discretization. Since shape radiomics features were not changed by imaging parameters, so were excluded for analysis. 93 first-order and texture radiomics features in six categories (first-order n=18; texture_GLCM n=24; texture_GLDM n=14; texture_GLRLM n=16; texture_GLSZM n=16; texture_NGTDM n=5) were extracted in the original images. In addition, the extended first-order and texture radiomics features were extracted in the images transformed from the original images. These transformed images were Laplacian-of-Gaussian filtering (LoG) with the sigma levels of 1 [LoG(1)] and 3 [LoG(3)], 1 level of wavelet decompositions yielding 8 derived images (HHH, HHL, HLH, HLL, LHH, LHL, LLH, LLL) and the images derived using Square, Square Root, Logarithm and Exponential filters. In total, 1,395 (93×15) radiomics features in the original images and 14 transformed images were included. The complete list and definition of these radiomics features could be referred to (https://pyradiomics.readthedocs.io/en/latest/features.html).
For each VOI, the radiomics feature values associated with each imaging parameter adjustment were compared with the baseline values. The feature value difference was then divided by the baseline value to calculate the percent deviation (d%) of the feature. Under a certain imaging parameter adjustment, if the average d% of a feature in all VOIs was < 5%, the feature consistency with the baseline was considered excellent. The consistency was good if 5%≤d%<10%, moderate if 10%≤d%<15%, and poor if d%≥15%.
The intra-class correlation coefficient (ICC, 2-way mixed-effects model, single rater, absolute agreement) was calculated to evaluate the agreement of the radiomics feature values in all 10 VOIs to the baseline (poor: ICC <0.50; moderate: 0.50≤ ICC <0.75; good: 0.75≤ ICC <0.90; excellent: ICC ≥0.90) (41).
If a radiomics feature was in the excellent agreement with the baseline based on both d% and ICC criteria, which was averaged for all imaging parameter settings in all VOIs, it was determined as a robust feature to imaging parameters.
All statistical tests were conducted in R v.1.2.5042 (RStudio, Boston, MA, USA). T-test was conducted to compare the radiomics feature deviation d% due to imaging parameter adjustment. ANOVA with Bonferroni correction as the post-hoc test was conducted to compare the d% among different imaging parameter settings and different feature categories. A P value of less than 0.05 indicated statistical significance.
Results
T1 and T2 relaxation time mapping of the phantom
The measured mean T1 and T2 relaxation times in the 10 VOIs of the phantom were summarized in Table 3.
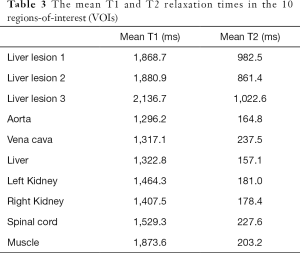
Full table
Deviation of radiomics feature values from the baseline due to imaging parameter change
The variation of original radiomics features with different imaging parameters in all VOIs
The percent deviation d% of the radiomics feature values in the original images averaged in all ten VOIs under each imaging parameter setting from the corresponding baseline feature values is shown in Figure 2. The deviation d% from the baseline values ranged from 0.02% to 321.3%, with a mean of 12.5% averaged for all features in all ten VOIs. The boxplots in Figure 3 illustrate the influences of different types of imaging parameters on the variation of original radiomics feature values. With the adjustment of TE, TR, and ETL, it was found that the larger difference from the default parameter value (defaults: TE/TR =206 ms/2,100 ms; ETL =134), the larger feature deviation d%. The radiomics feature values changed significantly with the varying TE, TR, and ETL (ANOVA, all P<0.001). The number of startup RF pulses notably affected the radiomics features, and the deviation d% was significantly different within the range from 4 to 10 (ANOVA P<0.001). The RF pulse train flip angle pattern also significantly (ANOVA, P<0.001) changed the radiomics feature values. The largest d% occurred (the median d%=18.4%, P<0.001) when the T2 spine view pattern (the longest TE=309ms) was applied, which supposed to have the strongest image T2 weighting among all flip angle patterns. As comparison, the smallest d% was associated with the T2 general pelvis view (median d%=3.5%, T-test P=1) and the T2 female pelvis view (median d%=3.3%, T-test P=1), both insignificant. The use of partial Fourier acquisition also significantly (ANOVA, P<0.001) influenced the radiomics feature values. Since the image SNR had been mostly normalized for partial Fourier acquisitions, the radiomics feature value change might be attributed to the subtle image appearance (or artifact) change rather than the SNR reduction. The absence of driven equilibrium notably but insignificantly changed the feature values with a median d% of 16.4% (T-test P=0.95), which might make the residual transversal magnetization larger at the end of each echo train than in the presence of driven equilibrium. The radial K-space trajectory did not remarkably change the radiomics feature values (the median d%=5.9%, T-test P=0.90) from the default Cartesian (Y) K-space trajectory. The switch of phase encoding direction from RL to AP resulted in a significant median radiomics feature deviation d% of 12.9% (T-test P<0.001).
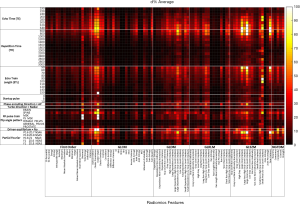
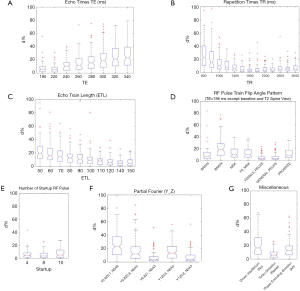
The influences of imaging parameters on different types of original radiomics features in all VOIs
Different types of radiomics features in the original images subjected to the varying imaging parameters to largely different extents, as also demonstrated in the heatmap of Figure 2. Figure 4 selectively illustrates the mean and the standard deviation of the d% associated with each category of radiomics features dependent on the varying TE, TR and ETL, respectively.
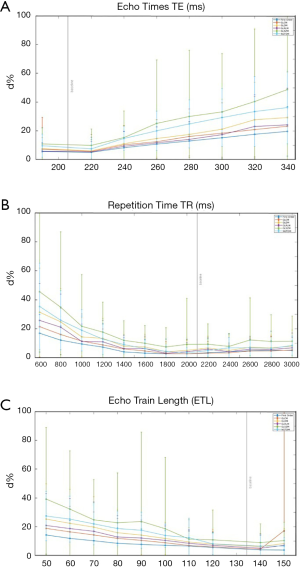
In terms of mean d%, GLSZM features subjected most (mean d%=19.6%) while first-order features subjected least (mean d%=8.0%) to all imaging parameter variations, with NGTDM (mean d%=15.1%), GLDM (mean d%=13.1%), GLRLM (mean d%=10.9%) and GLCM (mean d%=10.1%) in-between in a decreasing order. There were significantly different d% (ANOVA P<0.001) among these six types of radiomics features. The largest to the smallest standard deviation (SD) of d% was associated with GLSZM (SD d%=13.3%), NGTDM (SD d%=10.4%), GLDM (SD d%=8.4%), GLRLM (SD d%=7.9%), GLCM (SD d%=7.0%) and first-order (SD d%=5.0%) features, respectively.
The comparison of original radiomics feature variation with different imaging parameters in different VOIs
Due to the different T1 and T2 values in different VOIs, they subjected to the imaging parameter changes to different degrees. The boxplots in Figure 5 demonstrate the d% in each VOI under the influences of imaging parameter adjustments. The three liver lesions showed similar patterns of d% (ANOVA, P=0.838) with imaging parameter changes, due mainly to their similar T1 and T2 relaxation times. Because of their longer T2 relaxation times than other VOIs, the three lesions subjected more to driven equilibrium and thus induced larger radiomics feature value changes than other VOIs (T-test, P<0.01). A significant correlation between the longer T2 relaxation times and the higher d% of radiomics feature values in each VOI without driven equilibrium was also observed (Pearson correlation coefficient r=0.9; P<0.001). Similarly, left and right kidneys also showed similar patterns of d% (T-test, P=0.47) due to their similar relaxation times. The muscle VOI exhibited quite different and significantly larger (T-test, P<0.001) feature deviation from other VOIs in many imaging parameter changes, mainly attributing to its unique characteristics of long T1 but short T2 relaxation times among the ten VOIs. The percent deviation d% of the radiomics feature values in the original images in each individual VOIs under each imaging parameter setting from the corresponding baseline could be found in the Figure S1.
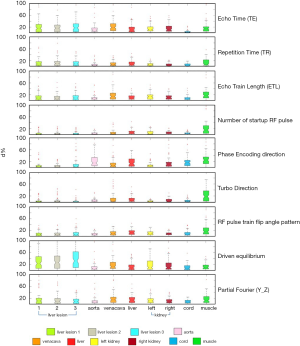
The comparison of feature value variation between the original image and transformed image due to imaging parameter change
Compared to the radiomics features in the original images, the features in the transformed images showed substantial different d% from the baseline values. The boxplots in Figure 6 illustrate the different feature d% patterns of each transformation under all imaging parameter settings averaged in all VOIs for the radiomics features. In general, there were significantly different feature values (ANOVA, P<0.001) between the original and the transformed images. After the transformations, some radiomics features became much more or less sensitive to imaging parameter adjustments. For example, most GLDM and GLRLM features exhibited much smaller d% from the baseline values in the wavelet transformed HHH images than in the original images. On the contrary, many first-order features showed much larger d% in the wavelet transformed HHH images than in the original images. The feature percent deviation d% in the transformed images averaged in all VOIs under each imaging parameter setting from the baseline values found be found in Figure S2.
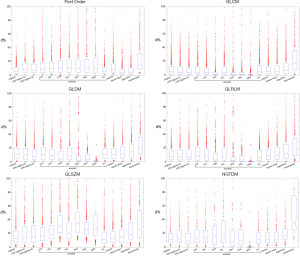
Robust radiomics features to imaging parameter changes
Figure 7 illustrate the agreement of the radiomics features with the baseline values under different imaging parameter settings in all VOIs of the original images on the basis of d% and ICC, respectively. Using the criterion of mean d% averaged for all imaging parameter settings, there were 23 out of 93 radiomics features were in the excellent agreement with the baseline; 25, 10, and 35 features were in the good, moderate and poor agreement with the baseline respectively. If the criterion of mean ICC averaged for all imaging parameter settings was used, there were 85, 7, 1, and 0 features were in the excellent, good, moderate, and poor agreement with the baseline, respectively. The criterion of mean d% seemed to be a much more stringent criterion than mean ICC for feature value robustness evaluation (42).
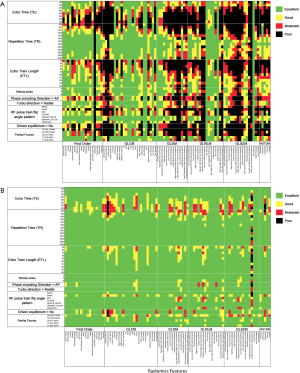
Figure 8 summarizes all robust features in the original and transformed images when both mean d% and mean ICC were taken into account. There were 16.1% (15/93) robust features in the original images. In the transformed images, the wavelet_HHH images presented the most robust features (40.9%, 38/93), while the exponential images had the least robust features (5.4%, 5/93). There were in total 330 out of 1395 features (23.7%) that were robust to imaging parameters in the original and transformed images. In terms of feature types, GLCM features had the largest number of robust features [42.5%, 153/(24×15)], while the GLSZM features had the least number of robust features [3.8%, 9/(16×15)].

Discussion
MRgRT offers a new platform and great opportunity to extend the use of MRI radiomics in a conventional off-line diagnostic setting to an on-line therapeutic setting (43-45). MRgRT is able to acquire daily MRI data to longitudinally and closely monitoring the changes of target tumors and organs-at-risk (OARs) at each treatment fraction. If MRI radiomics were able to truly reveal the changes of tumors and OARs, more sensitively and earlier than the conventional geometric surrogate changes, MRgRT treatment adaptation might be conducted based on the longitudinal MRI radiomics feature changes, named delta-radiomics (45,46). As such, the current MRgRT treatment adaptation practice based on position or shape change might be transformed to the adaptation on radiomics biomarkers in the future, better fulfilling personalized precision radiation oncology (4,47).
This study prospectively and rigorously investigated the quantitative variation of radiomics features, and examined their feature value robustness with respect to the change of imaging parameters in an anthropomorphic phantom, in particular aiming for the use of radiomics in MRgRT by using a standardized 3D-T2W-TSE pulse sequence on a commercial MR-LINAC platform as the baseline.
For MRgRT applications, 3D-T2W-TSE is one of the most important MRI pulse sequences. First, radiotherapy requires continuous volume information of the imaged anatomies without slice gaps and prefers isotropic voxel size for anatomy delineation and dose planning purposes. Moreover, image geometric fidelity is crucial for dose planning. Thus, 3D pulse sequences are advantageous over 2D sequences. In MRgRT, daily MR images are acquired in each treatment fraction for on-line treatment adaptation purposes, based on either patient position change or geometric change of anatomies (33). T2-weighted image contrast becomes preferable to T1-weighted contrast since the use of MRI contrast agent is avoided in this daily MRI acquisition scenario. Meanwhile, gradient echo sequences normally could not produce purely T2-weighted image contrast like spin-echo sequences. In addition, spin echo sequences are much less sensitive to tissue susceptibility and local magnetic field inhomogeneities induced by metal implants, hence, presenting less susceptibility or metal artifacts. Therefore, 3D-T2W-TSE is currently the dominant pulse sequence on the 1.5 T Eleckta Unity to obtain T2-weighted images for most anatomical regions.
The robustness of radiomics features to MRI acquisition imaging parameters is crucial for the reliable use of radiomics in clinics. Therefore, the quantitative variation of radiomics feature values with respect to acquisition pulse sequence and imaging parameters has to be carefully and rigorously gauged. However, this issue has been mostly overlooked in the past. The results of this study showed that acquisition imaging parameters in a 3D-T2W-TSE sequence substantially and significantly impacted many radiomics feature values. Only a fraction of features were robust to imaging parameter changes. The impact of imaging parameters on MRI radiomics features found in this study has some indications for clinical applications. Radiomics feature changes are anticipated to reveal the responses of tumors and organs-at-risk (OARs) to treatment more sensitively and earlier than the conventional geometric surrogates, such as volume and 1D dimension. But, such radiomics feature changes have to be true-positive, in other words, cannot be resulted from or mixed with other influencing factors like imaging parameters and image post-processing. Therefore, the finding in this study underscores the importance of using standardized and consistent imaging parameters in the MRI acquisition protocol for MRgRT radiomics studies. Meanwhile, imaging parameter heterogeneity should be considered as a significant factor and source of MRI radiomics variability and uncertainty, and has to be carefully addressed, well-compensated or harmonized, in particular for cross-vendor multi-center radiomics studies. Furthermore, the results in this study also underpin the necessity of selecting robust features to MR imaging parameters in radiomics modeling. Otherwise, the reliability of radiomics could be greatly compromised, which might even lead to false discovery and wrong decision making (14,48).
This current study complemented and advanced the prior studies (21-23) in several aspects. Our study explored a much larger number of imaging parameters and radiomics features, in which most original features are IBSI-compliant, than other studies. Compared to the study by Buch et al. (23), our study prospectively investigated the impact of imaging parameters on radiomics features in multiple VOIs with the measured T1 and T2 relaxation times in an anthropomorphic phantom, rather than the retrospective analysis on a single large VOI in a non-structural phantom. Better control was conducted in our study to avoid the mixed effect of other factors, like various post-acquisition image processing on the radiomics feature values. Moreover, this study attempted to explore the quantitative dependence of radiomics features on different imaging parameters, but not just to simply exhibit the significant difference of radiomics feature values in the presence of heterogeneous imaging parameters. Finally, robust radiomics features to imaging parameter changes were identified, and an explicit list of such robust features was given.
It should be definitely helpful to exactly figure out how imaging parameters affect radiomics feature values in an explicit and quantitative expression by linking underlying MRI physics and mathematical definition of radiomics features. However, this task could be extremely difficult for some reasons. First, MRI signal convolution in a 3D TSE sequence is much more complicated than in a 2D TSE sequence. In a 3D TSE sequence, specific design strategies and optimization, including but not limited to short non–spatially-selective RF pulses for echo spacing shortening, variable refocusing flip angles for blurring suppression, and optimal K-space trajectory acquisition and undersampling, are conducted to permit single-slab volumetric imaging in clinically acceptable duration (49,50). So its MRI signal cannot be theoretically calculated based on the tissue relaxation times and imaging parameters by using classical MRI physics theory as done in a 2D TSE sequence. On the other hand, the ground truth of the radiomics feature value in the phantom is unknown and cannot be accurately determined simply by imaging itself. Therefore, the impact of imaging parameters on radiomics feature could only be revealed in a comparative manner to the baseline. Radiomics accuracy and its dependence on imaging parameters cannot be assessed in this phantom, but have to rely on dedicatedly designed digital or physical radiomics phantoms (51,52).
Despite these difficulties, this study is still useful to better understand the dependence of radiomics features on different imaging parameters and intrinsic tissue properties, such as relaxation times and tissue heterogeneity. The responses of the MRI signals to the changing imaging parameters could behave so differently that the MR images could exhibit substantially different image intensity changes in different tissues. For example, the long T1 and T2 relaxation time of the lesions in the phantom made their voxel intensity, which is almost hyper-intensive, less sensitive to the change of TE and TR values. In contrast, the intensity change in the muscle of the phantom was much larger than that in the lesions with the same imaging parameter adjustment. The variability of radiomics features in the muscle was thus much larger than in the lesions. Different categories of radiomics features represent different texture characteristics in an image. For example, the GLCM features mainly characterize the texture of an image by calculating the probability of pairs of voxel with specific values and in a specified spatial relationship occur in an image. GLSZM features describe the amount of homogeneous connected areas within the volume, of a certain size and intensity. The results in this study showed GLCM features were more robust than GLSZM features to imaging parameters, indicating that the co-occurrence of voxels with specific intensities might maintain, although the regional intensity homogeneity is much affected in the image induced by the changing imaging parameters. The results also showed that image transformation might be helpful to reduce the sensitivity of some radiomics features to imaging parameters, e.g., wavelet_HHH transformation to many GLDM features, but might make other features more subject to imaging parameters, on the other hand, e.g., wavelet_HHH transformation to many GLSZM features.
There are some limitations in this study. First, it is impractical to exhaustively test all imaging parameters and all possible adjustments even in a single pulse sequence of 3D-T2W-TSE. We only selected some representative imaging parameters that could substantially affect image intensity and contrast. They were adjusted in a reasonably large range for possible clinical use. It is worth noting that other types of imaging parameters in a pulse sequence, such as those that mainly manipulate image geometry and image reconstruction, could also substantially affect MR image characteristics and thus radiomics feature values. They are to be individually and thoroughly investigated to complement this study. Meanwhile, only a subset of first-order and texture radiomics features in the original images and in a limited number of transformed images were included in this study for the same reason. Second, the repeatability of radiomics values under a fixed imaging protocol was not examined in this study. It is postulated that radiomics feature value change by adjusting imaging parameters should be larger than the fluctuation of the radiomics feature value between two repetitive acquisitions with the identical imaging parameters. It is plausible that this postulation might occasionally be violated in certain situations, for instance, in the situation of a very low image signal-to-noise ratio. Such a violation could make acquisition repeatability a greater uncertainty source of radiomics features than imaging parameter adjustment. Test-retest studies are needed for validation in the future. Third, this study was conducted on an MRI-simulator instead of an MR-LINAC. This arrangement had pros and cons. On the MRI-simulator platform, it had larger flexibility in the selection of imaging parameters and their adjustable ranges. On the other hand, due to the modified hardware configurations, such as magnet, gradients, and RF coils, for accommodating MRgRT applications, the MR images acquired on an MR-LINAC might be different from that on an MR-simulator, even with the identical sequence and imaging parameters. As such, further validation on MR-LINAC platform is desirable. Furthermore, cross-vendor inter-scanner reproducibility study is desired in the future to accommodate multi-center radiomics studies (53). Last but not least, this study is inevitably restricted by its phantom nature. The materials in the phantom differed, notably from in vivo tissues in the relaxation times. The structure in the phantom was also much less heterogeneous than in vivo tissues. There was no motion presented in the phantom. To this end, further studies in human subjects and real patients are warranted.
Conclusions
This study rigorously investigated the comprehensive influences of various acquisition imaging parameters on a large number of MRI radiomics features in an anthropomorphic phantom using a standardized 3D-T2W-TSE pulse sequence for MRgRT. It helped to better understand the quantitative dependence of radiomics feature values on different types of acquisition imaging parameters and intrinsic relaxation times. Radiomics features could be substantially affected by imaging parameter changes to different extents, so imaging parameter heterogeneity should be considered as a significant source of radiomics variability and uncertainty, which must be well harmonized to guarantee the radiomics reliability for clinical use. The robust features to imaging parameters identified in this study should be helpful for the pre-selection of radiomics features for reliable radiomics modeling.
Acknowledgments
Funding: This study was supported by Hong Kong Sanatorium and Hospital research project REC-2019-09. Dr. Queenie Chan from Philips Healthcare Hong Kong was acknowledged for the helpful discussion on Philips’ 3D T2 TSE sequence.
Footnote
Conflicts of Interest: All authors have completed the ICMJE uniform disclosure form (available at http://dx.doi.org/10.21037/qims-20-865). Dr. Yuan Jing serves as an associate editor of Quantitative Imaging in Medicine and Surgery. The other authors have no conflicts of interest to declare.
Ethical Statement: No ethical approval or written informed consent was required for this phantom study.
Open Access Statement: This is an Open Access article distributed in accordance with the Creative Commons Attribution-NonCommercial-NoDerivs 4.0 International License (CC BY-NC-ND 4.0), which permits the non-commercial replication and distribution of the article with the strict proviso that no changes or edits are made and the original work is properly cited (including links to both the formal publication through the relevant DOI and the license). See: https://creativecommons.org/licenses/by-nc-nd/4.0/.
References
- Gillies RJ, Kinahan PE, Hricak H. Radiomics: Images Are More than Pictures, They Are Data. Radiology 2016;278:563-77. [Crossref] [PubMed]
- Aerts HJ, Velazquez ER, Leijenaar RT, Parmar C, Grossmann P, Carvalho S, Bussink J, Monshouwer R, Haibe-Kains B, Rietveld D, Hoebers F, Rietbergen MM, Leemans CR, Dekker A, Quackenbush J, Gillies RJ, Lambin P. Decoding tumour phenotype by noninvasive imaging using a quantitative radiomics approach. Nat Commun 2014;5:4006. [Crossref] [PubMed]
- Lambin P, Rios-Velazquez E, Leijenaar R, Carvalho S, van Stiphout RG, Granton P, Zegers CM, Gillies R, Boellard R, Dekker A, Aerts HJ. Radiomics: extracting more information from medical images using advanced feature analysis. Eur J Cancer 2012;48:441-6. [Crossref] [PubMed]
- Lambin P, Leijenaar RTH, Deist TM, Peerlings J, de Jong EEC, van Timmeren J, Sanduleanu S, Larue R, Even AJG, Jochems A, van Wijk Y, Woodruff H, van Soest J, Lustberg T, Roelofs E, van Elmpt W, Dekker A, Mottaghy FM, Wildberger JE, Walsh S. Radiomics: the bridge between medical imaging and personalized medicine. Nat Rev Clin Oncol 2017;14:749-62. [Crossref] [PubMed]
- Aerts HJ. The Potential of Radiomic-Based Phenotyping in Precision Medicine: A Review. JAMA Oncol 2016;2:1636-42. [Crossref] [PubMed]
- Coroller TP, Grossmann P, Hou Y, Rios Velazquez E, Leijenaar RT, Hermann G, Lambin P, Haibe-Kains B, Mak RH, Aerts HJ. CT-based radiomic signature predicts distant metastasis in lung adenocarcinoma. Radiother Oncol 2015;114:345-50. [Crossref] [PubMed]
- Stoyanova R, Takhar M, Tschudi Y, Ford JC, Solorzano G, Erho N, Balagurunathan Y, Punnen S, Davicioni E, Gillies RJ, Pollack A. Prostate cancer radiomics and the promise of radiogenomics. Transl Cancer Res 2016;5:432-47. [Crossref] [PubMed]
- Valdora F, Houssami N, Rossi F, Calabrese M, Tagliafico AS. Rapid review: radiomics and breast cancer. Breast Cancer Res Treat 2018;169:217-29. [Crossref] [PubMed]
- Tagliafico AS, Piana M, Schenone D, Lai R, Massone AM, Houssami N. Overview of radiomics in breast cancer diagnosis and prognostication. Breast 2020;49:74-80. [Crossref] [PubMed]
- Ou D, Blanchard P, Rosellini S, Levy A, Nguyen F, Leijenaar RTH, Garberis I, Gorphe P, Bidault F, Ferte C, Robert C, Casiraghi O, Scoazec JY, Lambin P, Temam S, Deutsch E, Tao Y. Predictive and prognostic value of CT based radiomics signature in locally advanced head and neck cancers patients treated with concurrent chemoradiotherapy or bioradiotherapy and its added value to Human Papillomavirus status. Oral Oncol 2017;71:150-5. [Crossref] [PubMed]
- Chaddad A, Kucharczyk MJ, Daniel P, Sabri S, Jean-Claude BJ, Niazi T, Abdulkarim B. Radiomics in Glioblastoma: Current Status and Challenges Facing Clinical Implementation. Front Oncol 2019;9:374. [Crossref] [PubMed]
- Li M, Chen T, Zhao W, Wei C, Li X, Duan S, Ji L, Lu Z, Shen J. Radiomics prediction model for the improved diagnosis of clinically significant prostate cancer on biparametric MRI. Quant Imaging Med Surg 2020;10:368-79. [Crossref] [PubMed]
- Liu Y, Shi H, Huang S, Chen X, Zhou H, Chang H, Xia Y, Wang G, Yang X. Early prediction of acute xerostomia during radiation therapy for nasopharyngeal cancer based on delta radiomics from CT images. Quant Imaging Med Surg 2019;9:1288-302. [Crossref] [PubMed]
- Vallières M, Zwanenburg A, Badic B, Cheze Le Rest C, Visvikis D, Hatt M. Responsible Radiomics Research for Faster Clinical Translation. J Nucl Med 2018;59:189-93. [Crossref] [PubMed]
- Traverso A, Wee L, Dekker A, Gillies R. Repeatability and Reproducibility of Radiomic Features: A Systematic Review. Int J Radiat Oncol Biol Phys 2018;102:1143-58. [Crossref] [PubMed]
- Reuzé S, Schernberg A, Orlhac F, Sun R, Chargari C, Dercle L, Deutsch E, Buvat I, Robert C. Radiomics in Nuclear Medicine Applied to Radiation Therapy: Methods, Pitfalls, and Challenges. Int J Radiat Oncol Biol Phys 2018;102:1117-42. [Crossref] [PubMed]
- Yip SS, Aerts HJ. Applications and limitations of radiomics. Phys Med Biol 2016;61:R150-66. [Crossref] [PubMed]
- Balagurunathan Y, Kumar V, Gu Y, Kim J, Wang H, Liu Y, Goldgof DB, Hall LO, Korn R, Zhao B, Schwartz LH, Basu S, Eschrich S, Gatenby RA, Gillies RJ. Test-retest reproducibility analysis of lung CT image features. J Digit Imaging 2014;27:805-23. [Crossref] [PubMed]
- Qiu Q, Duan J, Duan Z, Meng X, Ma C, Zhu J, Lu J, Liu T, Yin Y. Reproducibility and non-redundancy of radiomic features extracted from arterial phase CT scans in hepatocellular carcinoma patients: impact of tumor segmentation variability. Quant Imaging Med Surg 2019;9:453-64. [Crossref] [PubMed]
- Li Y, Tan G, Vangel M, Hall J, Cai W. Influence of feature calculating parameters on the reproducibility of CT radiomic features: a thoracic phantom study. Quant Imaging Med Surg 2020;10:1775-85. [Crossref] [PubMed]
- Collewet G, Strzelecki M, Mariette F. Influence of MRI acquisition protocols and image intensity normalization methods on texture classification. Magn Reson Imaging 2004;22:81-91. [Crossref] [PubMed]
- Mayerhoefer ME, Szomolanyi P, Jirak D, Materka A, Trattnig S. Effects of MRI acquisition parameter variations and protocol heterogeneity on the results of texture analysis and pattern discrimination: an application-oriented study. Med Phys 2009;36:1236-43. [Crossref] [PubMed]
- Buch K, Kuno H, Qureshi MM, Li B, Sakai O. Quantitative variations in texture analysis features dependent on MRI scanning parameters: A phantom model. J Appl Clin Med Phys 2018;19:253-64. [Crossref] [PubMed]
- Zwanenburg A, Leger S, Vallières M, Löck S. Image biomarker standardisation initiative. Available online: https://arxivorg/abs/161207003 2019.
- Zwanenburg A, Vallieres M, Abdalah MA, Aerts H, Andrearczyk V, Apte A, Ashrafinia S, Bakas S, Beukinga RJ, Boellaard R, Bogowicz M, Boldrini L, Buvat I, Cook GJR, Davatzikos C, Depeursinge A, Desseroit MC, Dinapoli N, Dinh CV, Echegaray S, El Naqa I, Fedorov AY, Gatta R, Gillies RJ, Goh V, Gotz M, Guckenberger M, Ha SM, Hatt M, Isensee F, Lambin P, Leger S, Leijenaar RTH, Lenkowicz J, Lippert F, Losnegard A, Maier-Hein KH, Morin O, Muller H, Napel S, Nioche C, Orlhac F, Pati S, Pfaehler EAG, Rahmim A, Rao AUK, Scherer J, Siddique MM, Sijtsema NM, Socarras Fernandez J, Spezi E, Steenbakkers R, Tanadini-Lang S, Thorwarth D, Troost EGC, Upadhaya T, Valentini V, van Dijk LV, van Griethuysen J, van Velden FHP, Whybra P, Richter C, Lock S. The Image Biomarker Standardization Initiative: Standardized Quantitative Radiomics for High-Throughput Image-based Phenotyping. Radiology 2020;295:328-38. [Crossref] [PubMed]
- Boldrini L, Cusumano D, Cellini F, Azario L, Mattiucci GC, Valentini V. Online adaptive magnetic resonance guided radiotherapy for pancreatic cancer: state of the art, pearls and pitfalls. Radiat Oncol 2019;14:71. [Crossref] [PubMed]
- van Herk M, McWilliam A, Dubec M, Faivre-Finn C, Choudhury A. Magnetic Resonance Imaging-Guided Radiation Therapy: A Short Strengths, Weaknesses, Opportunities, and Threats Analysis. Int J Radiat Oncol Biol Phys 2018;101:1057-60. [Crossref] [PubMed]
- Hunt A, Hansen VN, Oelfke U, Nill S, Hafeez S. Adaptive Radiotherapy Enabled by MRI Guidance. Clin Oncol (R Coll Radiol) 2018;30:711-9. [Crossref] [PubMed]
- Henke LE, Contreras JA, Green OL, Cai B, Kim H, Roach MC, Olsen JR, Fischer-Valuck B, Mullen DF, Kashani R, Thomas MA, Huang J, Zoberi I, Yang D, Rodriguez V, Bradley JD, Robinson CG, Parikh P, Mutic S, Michalski J. Magnetic Resonance Image-Guided Radiotherapy (MRIgRT): A 4.5-Year Clinical Experience. Clin Oncol (R Coll Radiol) 2018;30:720-7. [Crossref] [PubMed]
- Menten MJ, Wetscherek A, Fast MF. MRI-guided lung SBRT: Present and future developments. Phys Med 2017;44:139-49. [Crossref] [PubMed]
- Lagendijk JJ, Raaymakers BW, Van den Berg CA, Moerland MA, Philippens ME, van Vulpen M. MR guidance in radiotherapy. Phys Med Biol 2014;59:R349-69. [Crossref] [PubMed]
- Woodings SJ, Bluemink JJ, de Vries JHW, Niatsetski Y, van Veelen B, Schillings J, Kok JGM, Wolthaus JWH, Hackett SL, van Asselen B, van Zijp HM, Pencea S, Roberts DA, Lagendijk JJW, Raaymakers BW. Beam characterisation of the 1.5 T MRI-linac. Phys Med Biol 2018;63:085015. [Crossref] [PubMed]
- Raaymakers BW, Jurgenliemk-Schulz IM, Bol GH, Glitzner M, Kotte A, van Asselen B, de Boer JCJ, Bluemink JJ, Hackett SL, Moerland MA, Woodings SJ, Wolthaus JWH, van Zijp HM, Philippens MEP, Tijssen R, Kok JGM, de Groot-van Breugel EN, Kiekebosch I, Meijers LTC, Nomden CN, Sikkes GG, Doornaert PAH, Eppinga WSC, Kasperts N, Kerkmeijer LGW, Tersteeg JHA, Brown KJ, Pais B, Woodhead P, Lagendijk JJW. First patients treated with a 1.5 T MRI-Linac: clinical proof of concept of a high-precision, high-field MRI guided radiotherapy treatment. Phys Med Biol 2017;62:L41-L50. [Crossref] [PubMed]
- Raaymakers BW, Lagendijk JJW, Overweg J, Kok JGM, Raaijmakers AJE, Kerkhof EM, van der Put RW, Meijsing I, Crijns SPM, Benedosso F, van Vulpen M, de Graaff CHW, Allen J, Brown KJ. Integrating a 1.5 T MRI scanner with a 6 MV accelerator: proof of concept. Phys Med Biol 2009;54:N229-37.
- Yuan J, Chow SK, Yeung DK, Ahuja AT, King AD. Quantitative evaluation of dual-flip-angle T1 mapping on DCE-MRI kinetic parameter estimation in head and neck. Quant Imaging Med Surg 2012;2:245-53. [PubMed]
- Wang HZ, Riederer SJ, Lee JN. Optimizing the precision in T1 relaxation estimation using limited flip angles. Magn Reson Med 1987;5:399-416. [Crossref] [PubMed]
- Liney GP, Knowles AJ, Manton DJ, Turnbull LW, Blackband SJ, Horsman A. Comparison of conventional single echo and multi-echo sequences with a fast spin-echo sequence for quantitative T2 mapping: application to the prostate. J Magn Reson Imaging 1996;6:603-7. [Crossref] [PubMed]
- Juras V, Bohndorf K, Heule R, Kronnerwetter C, Szomolanyi P, Hager B, Bieri O, Zbyn S, Trattnig S. A comparison of multi-echo spin-echo and triple-echo steady-state T2 mapping for in vivo evaluation of articular cartilage. Eur Radiol 2016;26:1905-12. [Crossref] [PubMed]
- Fedorov A, Beichel R, Kalpathy-Cramer J, Finet J, Fillion-Robin JC, Pujol S, Bauer C, Jennings D, Fennessy F, Sonka M, Buatti J, Aylward S, Miller JV, Pieper S, Kikinis R. 3D Slicer as an image computing platform for the Quantitative Imaging Network. Magn Reson Imaging 2012;30:1323-41. [Crossref] [PubMed]
- van Griethuysen JJM, Fedorov A, Parmar C, Hosny A, Aucoin N, Narayan V, Beets-Tan RGH, Fillion-Robin JC, Pieper S, Aerts H. Computational Radiomics System to Decode the Radiographic Phenotype. Cancer Res 2017;77:e104-7. [Crossref] [PubMed]
- Koo TK, Li MY. A Guideline of Selecting and Reporting Intraclass Correlation Coefficients for Reliability Research. J Chiropr Med 2016;15:155-63. [Crossref] [PubMed]
- Müller R, Buttner P. A critical discussion of intraclass correlation coefficients. Stat Med 1994;13:2465-76. [Crossref] [PubMed]
- Verma V, Simone CB 2nd, Krishnan S, Lin SH, Yang J, Hahn SM. The Rise of Radiomics and Implications for Oncologic Management. J Natl Cancer Inst 2017.109. [Crossref] [PubMed]
- Peeken JC, Bernhofer M, Wiestler B, Goldberg T, Cremers D, Rost B, Wilkens JJ, Combs SE, Nusslin F. Radiomics in radiooncology - Challenging the medical physicist. Phys Med 2018;48:27-36. [Crossref] [PubMed]
- Boldrini L, Cusumano D, Chiloiro G, Casa C, Masciocchi C, Lenkowicz J, Cellini F, Dinapoli N, Azario L, Teodoli S, Gambacorta MA, De Spirito M, Valentini V. Delta radiomics for rectal cancer response prediction with hybrid 0.35 T magnetic resonance-guided radiotherapy (MRgRT): a hypothesis-generating study for an innovative personalized medicine approach. Radiol Med 2019;124:145-53. [Crossref] [PubMed]
- Carvalho S, Leijenaar R, Troost E, van Elmpt W, Muratet J-P, Denis F, De Ruysscher D, Aerts H, Lambin P. Early variation of FDG-PET radiomics features in NSCLC is related to overall survival-the “delta radiomics” concept. Radiother Oncol 2016;118:S20-1. [Crossref]
- Wu J, Tha KK, Xing L, Li R. Radiomics and radiogenomics for precision radiotherapy. J Radiat Res 2018;59:i25-i31. [Crossref] [PubMed]
- Park JE, Park SY, Kim HJ, Kim HS. Reproducibility and Generalizability in Radiomics Modeling: Possible Strategies in Radiologic and Statistical Perspectives. Korean J Radiol 2019;20:1124-37. [Crossref] [PubMed]
- Mugler JP 3rd. Optimized three-dimensional fast-spin-echo MRI. J Magn Reson Imaging 2014;39:745-67. [Crossref] [PubMed]
- Busse RF, Brau AC, Vu A, Michelich CR, Bayram E, Kijowski R, Reeder SB, Rowley HA. Effects of refocusing flip angle modulation and view ordering in 3D fast spin echo. Magn Reson Med 2008;60:640-9. [Crossref] [PubMed]
- Bianchini L, Botta F, Origgi D, Rizzo S, Mariani M, Summers P, Garcia-Polo P, Cremonesi M, Lascialfari A. PETER PHAN: An MRI phantom for the optimisation of radiomic studies of the female pelvis. Phys Med 2020;71:71-81. [Crossref] [PubMed]
- Jaggi A, Mattonen SA, McNitt-Gray M, Napel S. Stanford DRO Toolkit: Digital Reference Objects for Standardization of Radiomic Features. Tomography 2020;6:111-7. [Crossref] [PubMed]
- Rai R, Holloway LC, Brink C, Field M, Christiansen RL, Sun Y, Barton MB, Liney GP. Multicenter evaluation of MRI-based radiomic features: A phantom study. Med Phys 2020;47:3054-63. [Crossref] [PubMed]