An automated and multiparametric algorithm for objective analysis of meibography images
Introduction
Meibomian glands play an essential role in maintaining ocular surface health and integrity by secreting various lipid components, forming a lipid layer, and preventing excessive tear evaporation (1). Functional disorders of the meibomian glands, known as meibomian gland dysfunction (MGD), are increasingly recognized as a high incidence disease (2-5). MGD is commonly characterized by terminal duct obstruction and/or abnormal glandular secretion, often leading to ocular surface epithelium damage, chronic blepharitis, and dry eye disease, which severely degrade quality of life (6). The reported prevalence of MGD varies widely from 3.6% to 69.3% (7,8), which is largely due to the lack of effective and unified diagnostic criteria (8). Evaluation of the changes to meibomian glands is important for the diagnosis and management of MGD in clinical practice.
Besides traditional clinical evaluation methods that combine clinical manifests for MGD diagnosis (9), imaging techniques such as meibography (10-13), optical coherence tomography (14,15), and in vivo confocal microscopy (16,17) have also been used to image meibomian glands. Meiobiography is a non-contact imaging technique that uses infrared illumination which acquires images of the silhouette of glands from entire human eyelids. Using meiobiography images, morphological changes such as dilation, distortion, shortening, and atrophy can be directly observed and assessed visually and qualitatively (6,9,18), which greatly assists ophthalmologists in developing standardized diagnosis and treatment strategies (9-13).
To aid ophthalmologists in conducting quantitative evaluation of meibomian glands with meibography images, research has been conducted to obtain and evaluate the reliability of various detailed scale parameter grading methods (10-13,19-28). The most common of these is the dropout area or meibomian gland area (10-13,19-23). Using Image J or other image editing software, the dropout area or meibomian gland area can be quantitatively calculated; however, this is mostly conducted in a subjective manner, as gland/gland-loss regions are manually selected (10-13,19-21). Thus, these methods are relatively time-consuming and can lead to obvious intra- and interobserver variability (13,24,25), which limit their use in clinical studies where many images need to be analyzed efficiently. There is thus a strong need for the development of objective analyses using automated algorithms; however, only a few automated approaches have been demonstrated (22,23,25-28) due to difficulties in measuring the eyelid area and meibomian gland segmentation with meibography images.
Meibomian gland dropout mostly occurs in the advanced stage of MGD, and comparison of the histologic sections of normal and obstructed human glands has revealed that obstruction of the orifice in MGD can result in dilation of the gland acinus and central duct, increased gland tortuosity, damage to secretory meibocytes, and eventually the atrophy of glands (29,30). Therefore, in addition to area ratio parameters, there is a need to develop other quantitative parameters that can detect detailed morphological changes to meibomian glands which can potentially be used for MGD diagnosis and severity assessment (9). To date, only a handful of studies have reported the analysis of gland length, width, or regularity in meibography images (12,13,25-28), of which some are subjectively characterized in manual fashion (12,13). A multi-parametric objective analysis would offer doctors comprehensive information in MGD diagnosis and evaluation, especially in discovering subtle changes in preclinical and early-stage cases and monitoring MGD progression.
In this study, we developed an objective meibography image–analyzing algorithm that is capable of automatically performing segmentation of the eyelid area with each single meibomian gland and providing multiple quantitative parameters for gland evaluation. Along with traditional parameters, such as the meibomian gland area ratio, gland length, and gland width, our algorithm introduces new morphological parameters including a gland diameter deformation index and gland tortuosity index. This address concerns in quantifying local gland diameter variation and the degree of curved and winding glands resulting from gland terminal duct obstruction, which mostly exists in the intermediate stages of MGD progression (13,16,25,29,30). Moreover, since changes in the secretion, consistency, and colour of meibum inside meibomian glands (12,29,31) could alter the signal intensity of glands in meibography images, we developed a new parameter, the gland signal index, to quantify the gland signal level, which can potentially be used to evaluate meibum secretion activity. Performance of the automated algorithm was evaluated by analyzing the similarity index and segmentation error between manual and automatic segmentations. We demonstrated the feasibility of our algorithm in processing typical meibograhy images, compared the acquired gland parameters of different images, and selected representative meibomian glands.
Methods
The development of this automated and multiparametric algorithm was based on Labview (Released 2016, National Instruments Corporation, USA) with NI vision Development Module 2016 on a PC running Windows 10. Images processed in this article were acquired using commercialized meibography Keratograph 5M (Oculus, Germany). The meibography acquired red–green–blue (RGB) infrared images and frames of the everted eyelid area with a 1,088×512 pixel resolution. Acquired images were converted to grayscale, and then exported and saved as bitmaps for analysis with our customized software. The algorithm provides fully automatic and repeatable analysis in three steps: (I) segmentation of the tarsal conjunctiva area as the region of interest (ROI), (II) segmentation and identification of meibomian glands within the ROI, and (III) quantitative multiparametric analysis.
ROI segmentation
Figure 1 shows a flow map of the processing steps of the algorithm for ROI segmentation with a sample meibography image (Figure 1, IG). In brief, automatic extraction of the ROI is performed by removing the unwanted signals outside the tarsal conjunctiva area and to fit the boundary of the ROI region. For this, the original grayscale meibography image (Figure 1, IG) is firstly processed with a Prewitt operator to select the unwanted regions with eyelashes and highlight areas that typically appear around the eyelash roots and eyelid margin with large light reflections. The processed image is binarized and dilated to generate a mask image (Figure 1, IM), which is then subtracted from the original grayscale image (Figure 1, IG), resulting in the image in Figure 1, IGM. The resulting image then undergoes median filtering with a circular structural element having a 3-pixel diameter to remove random noises. To enhance the image contrast and visibility of the glands, the Convolution-Highlight Details operator is applied to the image with a structural element size of 25×25 pixels. Gland edges and image details are further sharpened and outlined by a Laplacian filter with a structural element size of 29×29 pixels, as shown in image Figure 1, IHD. The processed image is then binarized and binary inversed to highlight the surrounding background of the glands on the tarsal conjunctiva area (Figure 1, IBI). As the former subtracted regions are inversed to be positive, the mask image is subtracted again from the binary-inversed image Figure 1, IBI, resulting in image Figure 1, IBIM. To eliminate image speckles and small artifacts, the image in Figure 1, IBIM was eroded by a structural element 5×5 pixels in size, which also shatters connected small irregularities. All signal blocks with a size smaller than 190 pixels and which are empirically found, are then cleared to remove small irregularities that do not belong to the everted eyelid. The image is then dilated back by a structural element 5×5 pixels in size, and a border rejecting operator is applied to remove large irregularities connected with the image borders (Figure 1, IRSO).
As most information on the tarsal conjunctiva area is kept, a gradient out operator with a circular structural element 8 pixels in diameter is employed to connect the signal blocks followed by a convex hull operator that uses a Graham scan algorithm to fit a closing shape of the tarsal conjunctiva area (Figure 1, ICH). To remove the remaining surrounding lumps, the image is then eroded, withholding only the largest block, and then dilated back, resulting in a single block fitting the eyelid region (Figure 1, ICF). To define the final ROI, the image, as represented by Figure 1, ICF, is converted into grayscale, and the contour of the final block is used to fit the final boundaries of the ROI. For the upper boundary, longitudinal top-down scans are performed to find the first signal rising points, which are then connected and fitted by a second degree, one-dimensional B-spline of the centripetal parameter (blue line in Figure 1, ICF). For the lower boundary, the optimal fitting with a second degree, one-dimensional B-spline is performed to the line connecting the first signal rising points of the bottom-up longitudinal scans in the image (green line in Figure 1, ICF). The final ROI is acquired with the closing area formed by the fitted upper and lower boundaries (Figure 1, IROI). The original meibography image with the ROI boundary (red line in Figure 1, IGCF), within which the gland segmentation and quantitative analysis would be performed, is also shown in Figure 1, IGCF.
Segmentation and identification of glands within the ROI
The main steps of the algorithm for meibomian gland segmentation are shown in Figure 2. The original grayscale meibography image (Figure 2, IG) is first sequentially processed by a median filter, a Convolution-Highlight Details operator, and a Laplacian filter, as mentioned above in the description of the ROI segmentation process. This dampens the random noises and highlight the glands’ visibility (Figure 2, IGHD). The resulting image is then multiplied by the binary mask of the ROI and binarized (Figure 2, IGE). To remove the remaining image speckles and smooth the shape of the glands, the binarized image is convoluted by a median filter with a circular structural element 5 pixels in diameter. As the ROI segmentation is usually overestimated resulting in some detected objects belonging to the edge of the everted eyelid, an orientation operator is applied to remove those objects with a main angle lower than 45° or higher than 135°. This is determined empirically, as the eyelid edges are typically horizontal while glands mostly have a vertical orientation. The image containing all the detected signals from the glands for gland area ratio analysis is shown as Figure 2, IGL.
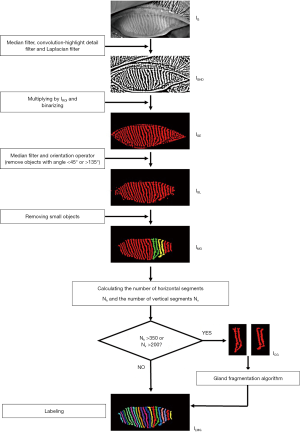
Since the small meibomian glands on the nasal and temporal sides are mostly irregular, usually out of focus, and unevenly illuminated during meibography imaging, these segmented glands are usually broken into pieces (Figure 2, IGL). Thus, to perform further quantitative analysis of each single gland, these broken glands are excluded by eliminating objects containing fewer than 1,400 pixels, as shown in Figure 2, IMG. While in some cases segmented glands can be connected (highlighted in green and yellow in Figure 2, IMG), affecting the subsequent analysis, the connected glands are detected by calculating the number of horizontal segments, Nh, and vertical segments, Nv, of the single objects in Figure 2, IMG and extracting those with Nh >350 or Nv >200 (Figure 2, ICG). The extracted connected glands images (Figure 2, ICG) are then sent to the gland fragmentation algorithm.
As shown in Figure 3, the gland fragmentation algorithm is developed to automatically separate connected glands. The extracted connected gland image (Figure 3, ICG) is continuously eroded with a structural element size of 1×3 pixels until the detecting objects number, N, exceeds 1 (Figure 3, IED). The eroded image is then binary inversed and skeletonized to generate a skeleton image (Figure 3, IIS), which is then subtracted from the connected gland image (Figure 3, ICG), resulting in separation of the connected glands (Figure 3, IGS). However, as seen in the zoom-in area of Figure 3, IGS, although separated, the single glands are disrupted by some skeleton branches. Before sending the separated disrupted glands for repair, the number of horizontal segments, Nh, and vertical segments, Nv, of the single objects depicted in Figure 3, IGS are calculated to ascertain whether objects of connected glands still exist. If they do, the eroding process is repeated to obtain further separation, and if not, the separated single disrupted glands (Figure 3, ISGS) proceed to the repair process. By applying a gradient out operator with a circular structural element with a 3-pixel diameter followed by a fill hole operator, the disrupted single glands are fixed while being expended out by 1 pixel (Figure 3, IGO). A gradient in the operator with a circular structural element 3-pixels in diameter is then applied to form the contours of the single glands (Figure 3, IGC). Gland repair (Figure 3, IRG) is achieved by subtracting the gland contours from the expended glands. The final separated glands are shown in Figure 3, ISG. By combing the final separated glands and the detected single glands (red glands in Figure 2, IMG), all intact glands are identified and labelled for further quantitative analysis.
Quantitative parameter analysis
Using the segmented ROI and labelled intact meibomian glands, quantitative parameters including the gland area ratio (GA), gland length (L), gland width (W), gland diameter deformation index (DI), gland tortuosity index (TI), and gland signal index (SI) are defined and computed.
The gland area ratio, GA is calculated as follows:
[1]
in which Ngl is the number of pixels occupied by all detected signals from the glands (Figure 2, IGL) and NROI is the number of pixels occupied by the segmented ROI area (Figure 1, IROI).
Morphological parameter analysis of the length, width, diameter deformation index, and tortuosity index are performed on each segmented and labelled gland (Figure 2, ILMG). The single gland length, L, is defined as follows:
[2]
in which lMN is the length (in pixels) of the gland central line between endpoints M and N (Figure 4A), and R is the digital resolution of the meibography. By imaging a diffuse reflectance grid distortion target (#62-949, Edmund Optics), as shown in Figure 4B, R was calibrated to be 0.03 mm/pixel in our case with images captured by OCULUS Keratograph 5M. The gland width, D, is defined by the averaged width across a single gland:
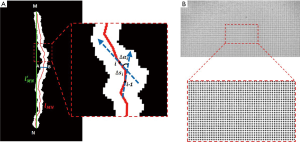
[3]
in which di is the width (in pixels) of the gland at every 3-pixel point on the gland central line and is calculated by the pixel length of the perpendicular line of the local tangent line within the intersection points A, B on the gland edge contour (Figure 4A). To address diameter variations such as the uneven dilation and discontinuous atrophy of a gland, which are related to the status of the meibomian gland duct and acinus (25), the gland diameter deformation index, DI, is introduced by the following:
[4]
which is the standard deviation σd of the local gland widths within a single gland. To quantify the degree of gland curving and hairpin-loop–like winding changes, which mostly result from gland terminal duct obstruction, the gland tortuosity index, TI is defined as follows:
[5]
in which lMN is the length (in pixels) of the gland central line between endpoints M and N,
As meibomian gland functional and structural disorders affect meibum secretion activity, different meibum consistency and colour directly alter the reflection of the illumination light, resulting in changes in the signal intensity of glands in meibography images. To quantify the gland signal level in the image, which can potentially be used to evaluate the meibum secretion, the gland signal index is defined as follows:
[6]
in which GREYi is the averaged image grey value of the segmented intact glands (Figure 2, ILMG) in the raw meibography image (Figure 2, IG), and GREYo is the averaged image grey value of the non-gland area (Inversed area of Figure 2, IGE) within the ROI area in the raw image.
Results
To validate the performance of this automated and multiparametric algorithm, 15 meibography images were acquired with OCULUS Keratograph 5M (Oculus, Germany) from subjects of different meibomian gland health status. Images were evaluated and agreed upon by two professional ophthalmologists. Informed consent was obtained from the subjects. The experimental procedures adhered to the tenets of the Declaration of Helsinki [1983] and were approved by the Institutional Review Board of the Zhongshan Ophthalmic Centre, Sun Yat-sen University, China (protocol number: 2019KYPJ110). The ground truth of the ROI and meibomian glands segmentations were defined by the mean manually delineated ROI and glands’ boundaries by the two ophthalmologists. The meibography images were also automatically and objectively processed by the algorithm for ROI and glands’ segmentation and multiparametric calculation. The manual segmentation of each image took approximately 8 minutes, while the automated processing took approximately 15 seconds.
Segmentation performance validation
To evaluate the segmentation performance of the algorithm, the similarity index (k) and segmentation error (32-35) were calculated between the manually defined ground truth segmentation and the automatic segmentation of both the ROI and meibomian glands. The similarity index (k), also known as the kappa coefficient, measures the similarity of the two segmentations defined by the ratio of the area of their intersection divided by the area of their union {Eq. [7]}. This ranges from 0 for segmentations that have no overlap to 1 for segmentations that are identical. To determine the segmentation error, we calculated the false-positive rate (rP) and the false negative rate (rN). The false-positive rate (rP) was depicted as the probability of the non-ROI/non-gland area included in the automatic segmentation results, and vice versa for the false-negative rate (rN). The false-positive rate (rP) and the false-negative rate (rN) were calculated by dividing the area of the incorrectly segmented non-ROI/non-gland area and ROI/gland area by the manually segmented ROI/gland area, as shown in Equation 8 and 9 respectively.
[7]
[8]
[9]
in which Sm is the manually segmented ROI/gland area and Sα is the automatic segmented ROI/gland area.
Figure 5 illustrates the contours of the segmented ROI (Figure 5A) and meibomian glands (Figure 5B) of a representative meibography image. The contours of the mean manually segmented ROI and meibomian glands are depicted in yellow while the algorithm automatically segmented contours are depicted in red. Table 1 shows the averaged similarity index and segmentation error between the manual and automatic segmentation of the ROI and meibomian glands for the 15 meibography images. For ROI segmentation, the similarity index (k)=0.94±0.02, the false-positive rate (rP)=6.02%±2.41%, and the false-negative rate (rN)=6.43%±1.98%. For meibomian gland segmentation, the similarity index (k)=0.87±0.01, the false-positive rate (rP)=4.35%±1.50%, and the false-negative rate (rN)=18.61±1.54%.
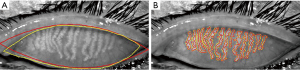
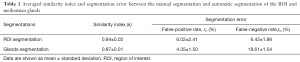
Full table
Automatic segmentation and multiparametric results
The segmentation results of the five representative meibography images acquired from subjects with different gland health status are shown in Figure 6. Images are displayed from Figure 6A,B,C,D,E based on their stage of MGD progression from healthy to severe as evaluated and agreed upon by the two ophthalmologists. The left column shows the original meibography images with the red curves encompassing the automatically segmented ROIs. The right column shows the segmented and labelled single meibomian glands for further quantitative analysis. The automatic calculated quantitative parameters, including the gland area ratio (GA), and gland signal index (SI), in addition to the averaged gland length (
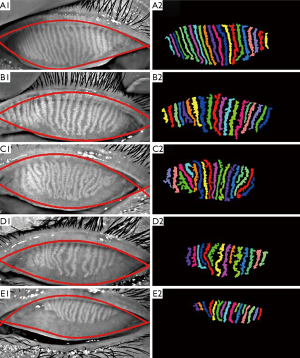
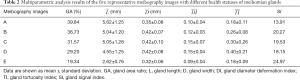
Full table
Comparison of representative glands
To better demonstrate the correspondence of gland morphological parameters to detailed gland characteristics, especially the newly defined DI and TI, we selected several representative glands from the processed meibography images and compared the calculated morphological parameters and their appearance characteristics as shown in Table 3. This showed that the less uniform the gland diameter was along its length, the higher the gland DI was, which is directly related to the uneven dilation and discontinuous atrophy of the meibomian gland duct and acinus (29,30). In addition, the larger the degree of gland curving and winding was, the higher the TI, which reflects the typical macro tortuous appearance of meibomian glands appearing in intermediate MGD (25,29).
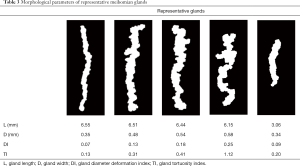
Full table
Discussion
In this study, we reported a fully automated algorithm for the objective segmentation and multiparametric quantitative analysis of meibography images. The automatic segmentations showed high similarity with moderate segmentation errors when compared with manual segmentations performed by professional ophthalmologists. The use of traditional parameters, such as the gland area ratio, gland length, and gland width, along with the newly defined parameters, including gland diameter deformation index, gland tortuosity index, and gland signal index, achieved a more comprehensive analysis of meibography images.
Various researchers have conducted quantitative analysis of meibography images with everted eyelid area and meibomian gland segmentations. However, most of these studies have implemented subjective methods to manually select the eyelid region and/or segmented the gland/gland-loss area with commercialized image editing software such as Image J (12,13,24) or self-developed semiautomatic algorithms (21) which usually have relatively low reliability and efficiency (24,25). Furthermore, while some automated methods have been developed, only a few researchers have tested multiparametric automated analysis methods. Arita et al. proposed image enhancement techniques to isolate meibomian glands but only computed the gland area ratio (22). Celik et al. introduced automated meibomian gland segmentation using Gabor wavelet filtering by extracting the orientation, width, length, and the curvature of segmented glands but used a standardized elliptical area for the ROI segmentation without adapting it to the real shape of each meibography image (23). Koh et al. reported an automated method to extract gland morphological features including gland length and width and trained a linear classifier for image classification but used manual selection for analysis of the eyelid region (26). Koprowski et al. described a fully automated approach that differentiated between gland, intergland, and gland drop-out areas to analyze images by gland area ratio together with detailed parameters quantifying the gland branches (27). Llorens-Quintana et al. developed an algorithm capable of segmenting both gland and tarsal conjunctiva areas automatically, and providing the gland area ratio, fitted ellipse for gland length and width analysis, and a newly defined gland irregularity parameter (28). Compared with the aforementioned approaches, our automated multiparametric algorithm identifies the ROI contours and segments meibomian glands based on image contrast enhancement and noise reduction. A subalgorithm has also been implemented to separate connected glands while not affecting their original appearance for further morphological analysis. In addition to traditional parameters, we proposed a novel method to objectively quantify gland diameter variations, gland tortuosity, and gland signal level, thus offering more comprehensive information which is likely to be advantageous for detecting local and subtle changes of meibomian glands.
The segmentation performance evaluation results indicate that our automatic algorithm produced a relatively high false-negative rate for meibomian gland segmentation compared with the mean manual segmentation. This is because manual segmentation tends to include the gaps between the gland acinus as the gland area, while automatic algorithms are more sensitive to image contrast and can detect and exclude these gap areas. Compared with former studies, the gland area ratio calculated by our algorithm is relatively smaller (12). This is because we excluded the intergland area from calculating the gland area ratio, as our method can precisely distinguish meibomian glands from the intergland area, while most other methods include it. The quality of the meibography images acquired for algorithm processing needs to be guaranteed. Images with low contrast, inhomogeneous illumination, and poor resolution could introduce extra error during ROI and meibomian gland segmentations, affecting the reliability of the final quantitative analysis results. Although our algorithm is currently adapted to the images acquired with the OCULUS Keratograph 5M (Oculus, Germany)—as most of the processing parameters are selected empirically—it may be adjusted to permit its use with other types of meibograpy instruments.
The algorithm offers more morphological and functional parameters for meibomian glands analysis, and our preliminary results show its potential in detecting subtle variations in meibomian glands. However, large scale clinical studies are needed to comprehensively characterize the relations between these quantitative parameters and the clinical manifestation of meibomian glands in different pathological stages of disease. Moreover, our current algorithm takes into account the analysis of the upper lid only. Since everting the lower eyelid is not as easy as the upper eyelid where a larger tarsal plate exists, meibography images of the lower lid are typically unevenly focused and only partially expose the meibomian glands; thus, automatic segmentation of the ROI and meibomian glands in the lower lid remains challenging. Future work will focus on lower lid meibography image analysis since combining the evaluation of both lids offers better clinical diagnostic performance.
Acknowledgments
Funding: This research is supported by the National Key Research and Development Program of China (2017YFC0112400) and the National Natural Science Foundation of China (81901788).
Footnote
Conflicts of Interest: All authors have completed the ICMJE uniform disclosure form (available at http://dx.doi.org/10.21037/qims-20-611). The authors have no conflicts of interest to declare.
Ethical Statement: The experimental procedures adhered to the tenets of the Declaration of Helsinki [1983] and were approved by the Institutional Review Board of Zhongshan Ophthalmic Centre, Sun Yat-sen University, China (protocol number: 2019KYPJ110). Informed consent was obtained from all subjects.
Open Access Statement: This is an Open Access article distributed in accordance with the Creative Commons Attribution-NonCommercial-NoDerivs 4.0 International License (CC BY-NC-ND 4.0), which permits the non-commercial replication and distribution of the article with the strict proviso that no changes or edits are made and the original work is properly cited (including links to both the formal publication through the relevant DOI and the license). See: https://creativecommons.org/licenses/by-nc-nd/4.0/.
References
- McCulley JP, Shine WE. Meibomian gland function and the tear lipid layer. Ocul Surf 2003;1:97-106. [Crossref] [PubMed]
- Ong BL, Larke JR. Meibomian gland dysfunction: some clinical, biochemical and physical observations. Ophthalmic Physiol Opt 1990;10:144-8. [Crossref] [PubMed]
- Schein OD, Muñoz B, Tielsch JM, Bandeen-Roche K, West S. Prevalence of dry eye among the elderly. Am J Ophthalmol 1997;124:723-8. [Crossref] [PubMed]
- Jie Y, Xu L, Wu YY, Jonas JB. Prevalence of dry eye among adult Chinese in the Beijing Eye Study. Eye (Lond) 2009;23:688-93. [Crossref] [PubMed]
- Schaumberg DA, Sullivan DA, Buring JE, Dana MR. Prevalence of dry eye syndrome among US women. Am J Ophthalmol 2003;136:318-26. [Crossref] [PubMed]
- Nelson JD, Shimazaki J, Benitez-del-Castillo JM, Craig JP, McCulley JP, Den S, Foulks GN. The international workshop on meibomian gland dysfunction: report of the definition and classification subcommittee. Invest Ophthalmol Vis Sci 2011;52:1930-7. [Crossref] [PubMed]
- Hom MM, Martinson JR, Knapp LL, Paugh JR. Prevalence of Meibomian gland dysfunction. Optom Vis Sci 1990;67:710-2. [Crossref] [PubMed]
- Amano S. Meibomian Gland Dysfunction: Recent Progress Worldwide and in Japan. Invest Ophthalmol Vis Sci 2018;59:DES87-DES93. [Crossref] [PubMed]
- Tomlinson A, Bron AJ, Korb DR, Amano S, Paugh JR, Pearce EI, Yee R, Yokoi N, Arita R, Dogru M. The international workshop on meibomian gland dysfunction: report of the diagnosis subcommittee. Invest Ophthalmol Vis Sci 2011;52:2006-49. [Crossref] [PubMed]
- Pult H, Riede-Pult BH. Non-contact meibography: keep it simple but effective. Cont Lens Anterior Eye 2012;35:77-80. [Crossref] [PubMed]
- Arita R, Itoh K, Inoue K, Amano S. Noncontact infrared meibography to document age-related changes of the meibomian glands in a normal population. Ophthalmology 2008;115:911-5. [Crossref] [PubMed]
- Pult H, Nichols JJ. A review of meibography. Optom Vis Sci 2012;89:E760-9. [Crossref] [PubMed]
- Srinivasan S, Menzies K, Sorbara L, Jones L. Infrared imaging of meibomian gland structure using a novel keratograph. Optom Vis Sci 2012;89:788-94. [Crossref] [PubMed]
- Hwang HS, Park CW, Joo CK. Novel noncontact meibography with anterior segment optical coherence tomography: Hosik meibography. Cornea 2013;32:40-3. [Crossref] [PubMed]
- Hwang HS, Shin JG, Lee BH, Eom TJ, Joo CK. In Vivo 3D Meibography of the Human Eyelid Using Real Time Imaging Fourier-Domain OCT. PLoS One 2013;8:e67143. [Crossref] [PubMed]
- Matsumoto Y, Sato EA, Ibrahim OM, Dogru M, Tsubota K. The application of in vivo laser confocal microscopy to the diagnosis and evaluation of meibomian gland dysfunction. Mol Vis 2008;14:1263-71. [PubMed]
- Villani E, Ceresara G, Beretta S, Magnani F, Viola F, Ratiglia R. In vivo confocal microscopy of meibomian glands in contact lens wearers. Invest Ophthalmol Vis Sci 2011;52:5215-9. [Crossref] [PubMed]
- Knop E, Knop N, Brewitt H, Pleyer U, Rieck P, Seitz B, Schirra F. Meibomian glands: part III. Dysfunction - argument for a discrete disease entity and as an important cause of dry eye. Ophthalmologe 2009;106:966-79. [Crossref] [PubMed]
- Nichols JJ, Berntsen DA, Mitchell GL, Nichols KK. An assessment of grading scales for meibography images. Cornea 2005;24:382-8. [PubMed]
- Shimazaki J, Sakata M, Tsubota K. Ocular surface changes and discomfort in patients with meibomian gland dysfunction. Arch Ophthalmol 1995;113:1266-70. [PubMed]
- Shehzad D, Gorcuyeva S, Dag T, Bozkurt B. Novel Application Software for the Semi-Automated Analysis of Infrared Meibography Images. Cornea 2019;38:1456-64. [PubMed]
- Arita R, Suehiro J, Haraguchi T, Shirakawa R, Tokoro H, Amano S. Objective image analysis of the meibomian gland area. Br J Ophthalmol 2014;98:746-55. [Crossref] [PubMed]
- Celik T, Lee HK, Petznick A, Tong L. Bioimage informatics approach to automated meibomian gland analysis in infrared images of meibography. J Optom 2018;6:194-204. [Crossref]
- Pult H, Riede-Pult B. Comparison of subjective grading and objective assessment in meibography. Cont Lens Anterior Eye 2013;36:22-7. [Crossref] [PubMed]
- Arita R, Itoh K, Maeda S, Maeda K, Furuta A, Fukuoka S, Tomidokoro A, Amano S. Proposed diagnostic criteria for obstructive meibomian gland dysfunction. Ophthalmology 2009;116:2058-63.e1. [Crossref] [PubMed]
- Koh YW, Celik T, Lee HK, Petznick A, Tong L. Detection of meibomian glands and classification of meibography images. J Biomed Opt 2012;17:086008. [Crossref] [PubMed]
- Koprowski R, Wilczyński S, Olczyk P, Nowińska A, Węglarz B, Wylęgała E. A quantitative method for assessing the quality of meibomian glands. Comput Biol Med 2016;75:130-8. [Crossref] [PubMed]
- Llorens-Quintana C, Rico-Del-Viejo L, Syga P, Madrid-Costa D, Iskander DR. A Novel Automated Approach for Infrared-Based Assessment of Meibomian Gland Morphology. Transl Vis Sci Technol 2019;8:17. [Crossref] [PubMed]
- Knop E, Knop N, Millar T, Obata H, Sullivan DA. The international workshop on meibomian gland dysfunction: report of the subcommittee on anatomy, physiology, and pathophysiology of the meibomian gland. Invest Ophthalmol Vis Sci 2011;52:1938-78. [Crossref] [PubMed]
- Obata H, Horiuchi H, Miyata K, Tsuru T, Machinami R. Histopathological study of the meibomian glands in 72 autopsy cases. Nippon Ganka Gakkai Zasshi 1994;98:765-71. [PubMed]
- Dougherty JM, McCulley JP. Bacterial lipases and chronic blepharitis. Invest Ophthalmol Vis Sci 1986;27:486-91. [PubMed]
- Lee JM, Yoon U, Nam SH, Kim JH, Kim IY, Kim SI. Evaluation of automated and semi-automated skull-stripping algorithms using similarity index and segmentation error. Comput Biol Med 2003;33:495-507. [Crossref] [PubMed]
- Zijdenbos AP, Dawant BM, Margolin RA, Palmer AC. Morphometric analysis of white matter lesions in MR images: method and validation. IEEE Trans Med Imaging 1994;13:716-24. [Crossref] [PubMed]
- Rohlfing T, Brandt R, Menzel R, Maurer CR Jr. Evaluation of atlas selection strategies for atlas-based image segmentation with application to confocal microscopy images of bee brains. Neuroimage 2004;21:1428-42. [Crossref] [PubMed]
- Lee CY, Chang TF, Chou YH, Yang KC. Fully automated lesion segmentation and visualization in automated whole breast ultrasound (ABUS) images. Quant Imaging Med Surg 2020;10:568-84. [Crossref] [PubMed]