An analysis of Ki-67 expression in stage 1 invasive ductal breast carcinoma using apparent diffusion coefficient histograms
Introduction
Breast carcinoma is one of the most frequent malignant tumors in females and a leading cause of mortality among women globally (1). Studies of breast carcinoma have shown that the size of the tumor depends not only on when it is detected, but also on its biological characteristics. Small tumors with poor biological characteristics may result in a poorer prognosis than large tumors with good biological characteristics (2). Tumor staging is mainly determined by the tumor size, and can provide some references for the surgical management of breast cancer and the selection of candidates for neoadjuvant chemotherapy (3,4). At present, due to increased public awareness and increased screening for breast cancer, the diagnosis rates of stage 1 or T1 breast cancer are increasing.
The Ki-67 proliferation index is associated with the biological characteristics of tumors. It reflects the extent of the proliferative activity of tumor cells and is a reliable identifier of more aggressive breast cancers (5-7). In patients with early-stage breast cancer, a high Ki-67 index is associated with a >50% increase in risk of death or higher and a 64% increased greater risk of recurrence (5). Thus, a non-invasive imaging assessment that can accurately predict the expression of Ki-67 prior to surgery would be beneficial in clinical practice.
Clinically, diffusion-weighted imaging (DWI) is widely used to describe and characterize breast tumors (8). Compared with normal tissues, restricted and hindered diffusion of water molecules in malignant tumors may lead to an increased signal on DWI images and a decreased signal on apparent diffusion coefficient (ADC) images (8). Numerous studies have reported that DWI can effectively reflect several histopathological features of malignant and benign lesions, and predict early response to treatment and clinical outcomes in different malignancies (9-13). Furthermore, as a quantitative value of diffusion restriction, the ADC can be obtained from DWI and has been correlated with the expression of Ki-67 in breast carcinoma (11,14). Some researchers have suggested that the ADC value is negatively correlated with Ki-67 expression (15,16), while others have failed to find any correlation between the two (17-19). Mori et al. (15) pointed out that one contributing factor to the discrepancies might be heterogeneity between subjects in different studies. Another factor to consider is necrosis within the lesion induced by prolonged hypoxia, which occurs when the tumor rapidly outgrows its vascular supply. Necrosis in a highly proliferative tumor may cause a decrease in cellularity and an increase in heterogeneity (20). As an emergent imaging analysis technique, histogram analysis can compile every voxel of a region of interest (ROI) into a histogram, from which information regarding tumor homogeneity/heterogeneity can be obtained (21). Histogram analysis includes several parameters including percentile values, kurtosis, and skewness (21). Previous studies examining different malignancies have shown that histogram analysis can provide more detailed information about tissue microstructure than conventional approaches performed using ROI-based analysis alone (22-26).
To our knowledge, there has been a paucity of studies demonstrating the relationship between ADC histograms and Ki-67 expression in stage 1 invasive ductal breast carcinomas (IDCs). The purpose of the present study was to explore the value of a histogram analysis method for ADC maps in distinguishing high Ki-67 groups from low Ki-67 groups in stage 1 IDC.
Methods
Patients
This study was approved by the ethics committee of the Hubei Cancer Hospital (No.: LLHBCH2020LW-016), and the requirement for written informed consent was waived due to its retrospective nature. All breast carcinoma patients at the Hubei Cancer Hospital (Wuhan, People’s Republic of China) between July 2013 and March 2018 were consecutively included in this study. A total of 309 patients with 382 breast carcinomas confirmed by postoperative pathology underwent preoperative breast magnetic resonance imaging (MRI) examinations. All patients were female and satisfied the following criteria: (I) no biopsy or medical treatment performed on the breast lesions before the MRI scan; (II) the MRI scan was performed in the second week of the menstrual cycle; and (III) the lesions were confirmed by histopathological and immunohistochemical examination of the specimens obtained from excisional biopsy. The exclusion criteria were as follows: (I) patients with multiple breast carcinomas (n=44), including bilateral breast carcinoma and ipsilateral breast carcinoma with multifocal lesions; (II) lesions less than 5 mm, or other histologic types or other stages of breast carcinoma (n=148); and (III) incomplete imaging acquisition or poor quality data (n=6). Finally, 111 patients with 111 IDC lesions and a mean age of 49.14 years (range, 23–74 years) were included in this study. Figure 1 provides a flowchart of the patient selection process.
Magnetic resonance imaging protocol
The images were acquired with a 3.0 Tesla (T) MR imaging system (Verio, Siemens Healthcare, Erlangen, Germany). A dedicated eight-channel phased array breast coil was used. A transverse T2-weighted turbo inversion recovery magnitude (TIRM) pulse sequence was performed with 5,600/56/170 ms [repetition time/echo time/inversion time (TR/TE/TI)], a 4-mm section thickness, a 0.8-mm intersection gap, a field of view (FOV) of 340 mm×340 mm, and a matrix of 314×320. A transverse T1-weighted fast low angle shot (FLASH) pulse sequence was performed with 6.1/2.6 ms (TR/TE), a 1.2-mm section thickness, a 0.24-mm intersection gap, a FOV of 340×340 mm, and a matrix of 384×365. DWI was acquired in the axial planes by using an echo-planar imaging sequence, parallel imaging with sensitivity encoding, volume shimming, b values of 50 and 800 s/mm2, TR/TE=7,300/83 ms, a 4-mm section thickness, a 2-mm intersection gap, a FOV of 320 mm×320 mm, and a matrix of 192×192. The ADC maps were created automatically by the system from the trace-weighted images and calculated according to the following formula: ADC = −(1/b) ln (S2/S1).
Dynamic contrast-enhanced MRI (DCE-MRI) images were acquired with eight phases, including one obtained before contrast and seven postcontrast phases. Axial dynamic imaging was performed (TR 4.67 ms, TE 1.66 ms, flip angle 10º, slice thickness 1.2 mm, matrix size 384×296, and FOV 360 mm×360 mm). The scanning time was 55 seconds per frame. Gadolinium (Magnevist; Beilu Pharmaceutical, BeiJin, China) was injected at a dose of 0.1 mmol/kg. Surgery was performed within 1 week following the MRI examination.
Region of interest selection
Image selection
Two radiologists (Maolin Xu and Yulin Liu, with 7 and 24 years of experience in MRI, respectively), who were blinded to the patients’ pathologic information, retrospectively reviewed the MRI images electronically on the picture archiving and communication system (PACS). In the case of disagreements, the two radiologists would reach a final decision by discussion and consensus. The final MRI images selected were exported from the PACS workstation in BMP format (bitmap image files) for histogram analysis. During export, the window width and window level were adjusted; thus, all images had consistent window width and window level, and they were saved on the hard disk.
Histogram analysis
The ADC histogram characteristics of the tumors were analyzed based on the magnetic resonance ADC image data. The MRI imaging data for the ADC maps were processed using the MaZda software (Version 4.6; www.eletel.p.lodz.pl/programy/mazda/). The largest lay of the selected lesions was analyzed. The software package ran stably in Windows 10. Based on the DWI, T1-weighted (T1WI), T2-weighted (T2WI), and T1WI enhanced images, two breast radiologists (Maolin Xu and Qi Tang, with 7 and 5 years of experience in breast MRI, respectively) delineated the ROIs manually along the edge of all 111 lesions on the ADC maps to calculate the histogram parameters. These parameters included mean value, variance, skewness, kurtosis, and the 1st, 10th, 50th, 90th, and 99th percentile values. All of the histogram parameters were defined according to Liang et al. (27) as follows. Mean was defined as the average pixel value. Variance was defined as variation from the mean gray-level value. Skewness was defined as asymmetry of the histogram. Kurtosis was defined as flatness of the histogram. The nth percentile was defined as the point at which n% of the voxel values that formed the histogram were found to the left. Gray-level normalization of each ROI was performed, using the limitation of dynamics to µ±3σ (µ, gray-level mean; and σ, gray-level standard deviation). The corresponding images before and after marking are shown in Figures 2 and 3. The x-axis of the histogram is the gray-level value, and the y-axis is the frequency of appearance corresponding to the gray-level value on the x-axis. The inter-observer reproducibility for the histogram parameter measurements was assessed by examining the data measured by each of the two radiologists.
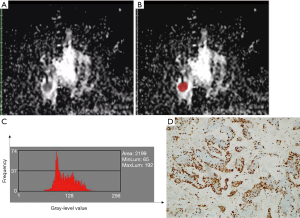
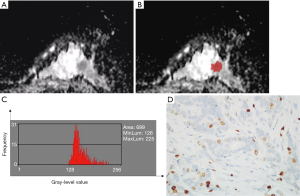
Histopathological analysis
In all 111 cases, specimens of breast cancer and axillary lymph nodes were surgically resected. Histological tumor grading was determined using the Nottingham combined histological grading system. Immunohistochemical (IHC) analyses were performed to measure the expression levels of the estrogen receptor (ER), progesterone receptor (PR), human epidermal growth factor receptor 2 (HER2), and Ki-67 as previously described (28). Positive expression of the ER and PR was defined as >1% of tumor nuclei showing positive staining (29). HER2 positivity was defined as having an IHC score of 3+. When gene amplification by fluorescence in situ hybridization (FISH) (28), tumors with an IHC score of 2+ were considered as HER2 positive (20). Ki-67 was scored by counting the number of cells with positively stained nuclei (30). Blinded to the MRI findings, two pathologists evaluated the Ki-67 immunoreactivity by assessing the percentage of immunoreactive cells from 1,000 tumor cells.
Regarding the use of Ki-67 expression as a diagnostic indicator, some international experts in the field have proposed a Ki-67 cut-off value of 20–29% as being indicative of luminal B carcinomas (31). Therefore, in this study, 25% was adopted as the Ki-67 cut-off value, and the patients were divided into two groups according to Ki-67 proliferation status, with a labeling index (LI) <25% being considered as low proliferation, and an LI of ≥25% being considered as high proliferation.
Patients were classified into the following four breast cancer subtypes: luminal A (ER positive and/or PR positive, and HER2 negative), luminal B (ER positive and/or PR positive, and HER2 positive, or HER2 negative but high Ki-67 proliferation), HER2-positive (ER negative, PR negative, and HER2 positive), and triple-negative (ER negative, PR negative, and HER2 negative).
Statistical analysis
Categorical variables were analyzed using the chi-squared test or Fisher’s exact test. All quantitative data derived from the histogram were averaged and expressed as the mean ± standard deviation (SD). One-sample Kolmogorov–Smirnov normality tests and Levene tests were used to evaluate normality and homoscedasticity of the ADC histogram data, respectively. If the data conformed to a normal distribution, the two independent samples t-test was applied to each parameter; otherwise, the Mann–Whitney U test was used. Pearson’s correlation analysis was performed to analyze the relationship between the ADC histogram parameters and the expression of Ki-67.
Inter-observer reliability of the ADC histogram parameters was assessed using the intraclass correlation coefficient (ICC).
The effectiveness of the ADC histogram parameters in differentiating the low-proliferation group from the high-proliferation group was evaluated by performing receiver operating characteristic (ROC) analysis. The area under the ROC curve (AUC) was calculated. The optimal threshold value for differentiating the low Ki-67 group from the high Ki-67 group was chosen at the maximum Youden index. The AUC was expressed as a mean and 95% confidence interval (CI). The method developed by Delong et al. (32) was applied to compare the AUCs. The Bonferroni correction was used for multiple testing.
SPSS software package (version 22.0; Chicago, IL) and MedCalc (version 15.2; Mariakierke, Belgium) were used for all statistical analyses. An emerging package of the R language, named ggplot2 (version 3.2.1), was applied to assist with the drawings.
Results
Clinicopathological findings
A comparison of the clinicopathological characteristics in the low Ki-67 group and the high Ki-67 group, as defined by Ki-67 proliferation status, is summarized in Table 1. All 111 breast carcinomas were solitary tumors, diagnosed histologically from surgical specimens. Histology of the lesions revealed that all were invasive ductal carcinomas. The tumor size of the target lesions ranged from 6 mm to 20 mm, including 55 stage 1b and 56 stage 1c cancers. The histological grade was G1/2 in 82 patients (73.9%) and G3 in 29 patients (26.1%). Positive ER protein findings were detected in 65 patients (58.6%), while positive PR protein expression was observed in 64 patients (57.7%). A positive HER2 score of 3+ was observed in 19 patients (17.1%), and a score of 2+ was detected in 31 patients (27.9%), of which, 9 cases (8.1%) were amplified by FISH. Ki-67 analyses showed high proliferation in 54 cases (48.6%) and low proliferation in 57 cases (51.4%). The distribution of the molecular subtypes was as follows: luminal A (34/111), luminal B (44/111), HER2 positive (13/111), and triple-negative (20/111). Patients in the high Ki-67 group showed a significantly higher histological grade, a larger tumor size, and a significantly higher occurrence of axillary node metastasis than patients in the low Ki-67 group (all P<0.05). No significant differences were found in age, menopausal status, or ER, PR, or HER2 expression status between the two groups (all P>0.05).
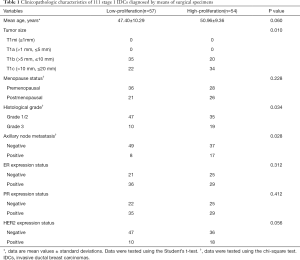
Full table
Interobserver agreement assessment
Inter-reader consistency data derived from the histogram are summarized in Table 2. Overall, the mean, variance, and all percentile values showed excellent inter-reader consistency (ICC range, 0.897–0.989), while inter-reader consistencies data regarding the skewness and kurtosis were relatively lower (ICC, 0.756 and 0.734, respectively).
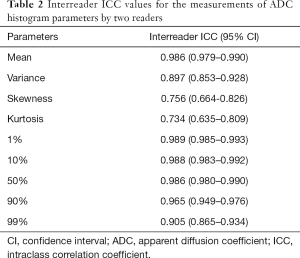
Full table
Correlation of ADC histogram parameters with Ki-67 expression
The correlation between the ADC histogram parameters and Ki-67 expression are shown in Table 3. The mean value, and the 1st, 10th, 50th, 90th, and 99th percentiles were negatively correlated with the expression of Ki-67 (all P<0.001), with a correlation coefficient of −0.624, −0.749, −0.717, −0.621, −0.500, and −0.410, respectively. However, neither variance, skewness, nor kurtosis showed a significant correlation with Ki-67 expression (P=0.197, 0.518, and 0.931, respectively).
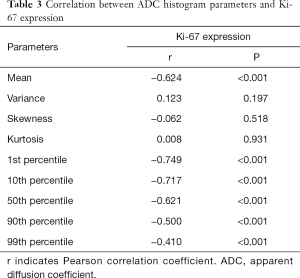
Full table
ADC histogram parameters between low- Ki-67 group and the high- Ki-67 group
The results of the analysis of the ADC histogram parameters for the low Ki-67 group and the high Ki-67 group are shown in Table 4. The mean value, and the 1st, 10th, 50th, 90th, and 99th percentiles in the high-proliferation and low-proliferation groups, were significantly different (all P<0.05); however, no significant differences were found in variance, skewness, or kurtosis (P=0.258, 0.411, and 0.998, respectively). Examples are shown in Figures 2 and 3. In Figure 4, box plots of the significant ADC histogram parameters between the two groups are presented.
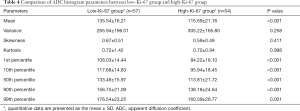
Full table
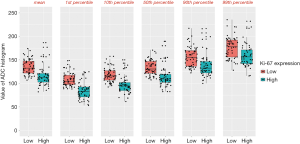
Comparison of ROC analysis of ADC histogram parameters in distinguishing low Ki-67 group from the high Ki-67 group
The results of the ROC analysis of the significant ADC histogram parameters are shown in Figure 5 and Table 5. The AUC values of the mean, and the 1st, 10th, 50th, 90th, and 99th percentiles for predicting Ki-67 proliferation status for patients with T1 stage IDC were 0.816, 0.856, 0.849, 0.815, 0.755, and 0.717, respectively. Using the method developed by Delong et al., pairwise AUC value comparisons among the mean, 1st percentile, 10th percentile, 50th percentile, 90th percentile, and 99th percentile were performed. As a result, the P-value of 0.05 was adjusted to 0.0033. The 1st and 10th percentiles had relatively higher AUC values, and the two values were not significantly different (P=0.6450). The 1st and 10th percentiles had higher AUC values than the mean or 50th percentile, but the difference was not significant (all P>0.0033). The mean and 50th percentile both demonstrated significantly higher AUC values than the 90th and 99th percentiles (all P<0.0033).
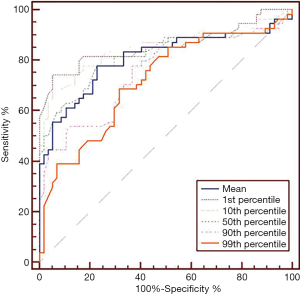
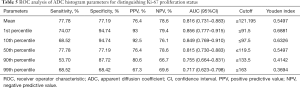
Full table
Discussion
Our study demonstrated that certain ADC histogram values showed a correlation with the expression of Ki-67 in stage 1 IDC. There were significant differences in the mean value, and the 1st, 10th, 50th, 90th, and 99th percentiles according to different Ki-67 expression classifications. Furthermore, with excellent inter-observer reproducibility (0.989 and 0.988), the 1st percentile and 10th percentile showed a relatively higher correlation (−0.749 and −0.717) with Ki-67 expression, and demonstrated higher AUC values (0.856 and 0.849) for predicting Ki-67 proliferation status. Thus, in this study, ADC map histogram analysis could effectively reflect tumor heterogeneity, and it may have tremendous potential to serve as a predictor of Ki-67 expression level in stage 1 IDC.
The Ki-67 LI has been widely used as a prognostic indicator for many malignant tumors, including breast cancer, soft-tissue tumors, and lung cancer (33). A higher Ki-67 index is associated with a poor outcome in IDC patients and can be regarded as a candidate biomarker for luminal B tumors (34). In contrast to previous research (5,15,35,36), in this study, the patients’ age, menopausal status, and ER, PR, and HER2 expression status were not significantly different between the Ki-67 high-proliferation and low-proliferation groups. This could be due to the low-stage IDCs and cutoff value of 25% for Ki-67 expression used in our study. Moreover, statistically significant differences were found between the low-proliferation group and the high-proliferation group with regard to tumor size, histological grade, and axillary node metastasis, which might be associated with the cell proliferation and cancer progression in the high-proliferation group (15,36). Ryu et al. (37) supposed that many clinicians might underestimate the risks in patients with smaller breast tumors, but the possibility of biologically aggressive characteristics might actually be increased for patients with small tumors with lymph node metastases. Considering this, more attention should be given to a T1 IDCs with a high Ki-67 index in clinical practice.
As the most influential factor of ADC value, the Ki-67 index is closely associated with the degree of cellularity and disease recurrence (38,39). Due to necrosis or degenerative components in the tumor, traditional ADC values cannot fully reflect the degree of cellularity (15,20). Voxel-based histogram analysis is broadly recognized as a reproducible technique for the analysis of ADC maps, which can provide comprehensive and objective information on intralesional heterogeneity and texture (40). ADC histograms can show the frequency of ADC values and provide more descriptive information to analyze the whole tumor, and are less dependent on the tumor microstructure (41). Previously, Hirata et al. (42) supposed that ADC histogram analysis could be an imaging biomarker for esophageal cancer patients treated with chemoradiotherapy. Numerous studies have also indicated that ADC histogram analysis can be applied in the differential diagnosis, lesion’s histological subtype, evaluation of therapeutic response, and prognostic prediction of tumor patients (23,25,26,43,44).
Most previous studies have use manual contouring of the entire tumor for histogram analyses, which might be more objective and better reflect the heterogeneity of the lesion. Nevertheless, histogram analysis of the entire tumor is cumbersome and may be impractical in daily clinical practice. Emulating some investigators in recent years, our histogram analysis was based on the largest representative cross section of tumors on ADC maps; therefore, some information about the rest of the volume was potentially excluded. However, the inclusion of several first and last slices would inevitably bring about a partial volume effect, because all of the tumors in this study were relatively small.
Histogram analysis of the largest cross section of the tumor has previously been used to differentiate between the various stages of bladder cancer and cervical cancer, as well as between medulloblastomas and pilocytic astrocytomas (41,45,46). In the current study, histogram analysis of pixel-based ADC maps of the largest cross section of the tumor was performed to acquire various parameters, including the mean value, variance, skewness, kurtosis, and the 1st, 10th, 50th, 90th, and 99th percentiles, which were used to determine the Ki-67 proliferation status in T1 stage IDC. The methodology of our histogram analysis was different from that reported by most previous studies, but was similar to that reported by Liang et al. (27) and Wang et al. (41). In this study, the ADC maps for the histogram analysis were not in DICOM (Digital Imaging and Communications in Medicine) format but in BMP format, due to the limitations of the MaZda software. As a result, the value of the horizontal axis of the histogram was just a gradation value of gray-scale maps between 1 and 256, and the mean and nth percentile values were not ADC values. Nevertheless, the gradation values of ADC maps could be assumed to correspond to a certain ADC value in this study because the window width and window level were fixed. Furthermore, most parameters, except skewness and kurtosis, showed almost perfect inter-observer reliability (ICC range, 0.897–0.989), suggesting the reproducibility of this ADC histogram method. Therefore, we hypothesized that the ADC histogram parameters from the part of the tumor with the largest diameter could also reliably reflect some characteristics of the entire lesion in stage 1 IDC.
Many studies have revealed a correlation between the ADC value and Ki-67 expression in breast carcinoma. Both Shen et al. (47) and Molinari et al. (48) proposed that lower ADC values are associated with Ki-67 proliferation index, with the cutoff value of 0.97×10−3 and 0.95×10−3 mm2/s able to differentiate the high-proliferation group and the low-proliferation group, respectively. In contrast, Kim et al. (20) reported there was no significant correlation between ADC value and Ki-67 expression because of necrosis in tumors that. However, the ADC values of all these previous studies were based on selected ROIs drawn on parts of the tumor for analysis, and therefore, characteristics of the whole tumor were not sufficiently reflected.
Using ADC histogram parameters from the part of the tumor with the largest diameter, our study indicated that the 1st and 10th percentiles showed a strong negative correlation with Ki-67 expression and had a better predictive value. When cancer cells grow rapidly, tumor necrosis is increased and the tumor becomes more heterogeneous (49). It is possible that the T1 stage IDCs could present a little necrosis, hemorrhage, and cysts, which might have contributed to higher ADC values (50). Therefore, lower percentiles could have better association with high cell compositions in the tumor. In partial disagreement with our research, Mori et al. (15) revealed that each of the minimum ADC, the mean ADC, and the 25th, 50th, and 75th percentile values all showed similar negative correlations with the Ki-67 LI in luminal-type breast cancers. They suggested that this might be attributable to the relatively homogeneous histologic components of luminal-type breast cancers. Also, You et al. (51) reported that a high proliferation of Ki-67 was significantly correlated with lower 50th and 95th percentile ADCs in HER2-positive breast cancers. It is possible that HER2-positive tumors have higher ADC values due to the increased tumor blood flow and a greater volume of extracellular fluid (17,38). The discrepancies in these studies may be associated with the sample size, method of histogram analysis, cutoff value of Ki-67, MRI system, and b values.
The asymmetry and peak of ADC distribution are visually reflected by skewness and kurtosis, respectively (40). Variance refers to the variation from mean gray-level value. Histogram skewness describes the distribution symmetry of variable values, indicating the degree of distribution asymmetry relative to the mean value. The histogram kurtosis can be used to reflect the relative sharpness or flatness of a distribution (41). Kurtosis can be regarded as a quantitative surrogate marker of tumor heterogeneity. Low kurtosis reflects a wide distribution linked to high variation in voxel value (44). Hu et al. (33) reported that neither standard deviation, skewness, nor kurtosis had any significant difference between low and high Ki-67 groups of hepatocellular carcinoma patients. Nevertheless, You et al. (51) demonstrated that kurtosis of the ADC histogram was positively correlated with the Ki-67 index in HER2-positive breast cancers. Our study found that the variance, skewness, and kurtosis showed no significant differences between the two groups, and there was no significant correlation with Ki-67 expression. These results were consistent with reports by Kim et al. (38). The reasons for the discrepancies among different investigators are difficult to explain. A potential explanation may be the smaller tumors featured in our study, which might have brought a certain partial volume effect and affected the measurement of tumor heterogeneity. The relatively inferior inter-observer reliability, especially for skewness and kurtosis, may also affect the results. Further studies are certainly warranted to clarify these results.
This study has some limitations. Firstly, this work was a retrospective study. Our study only included patients with stage 1b and 1c IDC, and did not include lesions measuring 5 mm or less due to possible partial volume artifacts and limited DWI resolution. Secondly, we adopted the LI of 25% as the cutoff value for Ki-67, and although similar research has suggested that a cutoff value of 25% for Ki-67 expression is a good classification tool for prognosis in colorectal cancer (52), the optimal LI cutoff value is still under debate. Thirdly, DWI was obtained at b values of 50 and 800 s/mm2 rather than multiple b value combinations, which can provide more accurate data about perfusion or diffusion. Fourthly, we manually drew ROIs within the part of the tumors with the largest diameter, which may not accurately reflect characteristics of the entire tumor. However, two-dimensional and three-dimensional measurements of single lesions have previously shown fairly similar results (53). Fifthly, this study used BMP-format ADC maps for histogram analysis. As a result, the mean and nth percentile values were not ADC values, which might reduce the usefulness of these values in the clinical setting. Future research should analyze the differences in predictive efficiency for Ki-67 expression between ADC histogram parameters (including mean and nth percentile values) and ADC values with different measurements. Finally, this investigation only examined Ki-67 expression and ADC histogram parameters, but other prognostic factors and molecular profiles of breast carcinomas were not explored.
In conclusion, ADC histograms are a particularly practical and useful non-invasive imaging method for assessing Ki-67 expression status in stage 1 IDC. The 1st and 10th percentiles derived from our ADC histogram showed a strong negative correlation with Ki-67 expression and provided a better predictive value.
Acknowledgments
Funding: None.
Footnote
Conflicts of Interest: All authors have completed the ICMJE uniform disclosure form ((available at http://dx.doi.org/10.21037/qims-20-615). The authors have no conflicts of interest to declare.
Ethical Statement: This study was approved by the Ethics Committee of our hospital. Informed consent was waived due to the retrospective nature of this study.
Open Access Statement: This is an Open Access article distributed in accordance with the Creative Commons Attribution-NonCommercial-NoDerivs 4.0 International License (CC BY-NC-ND 4.0), which permits the non-commercial replication and distribution of the article with the strict proviso that no changes or edits are made and the original work is properly cited (including links to both the formal publication through the relevant DOI and the license). See: https://creativecommons.org/licenses/by-nc-nd/4.0/.
References
- Torre LA, Islami F, Siegel RL, Ward EM, Jemal A. Global Cancer in Women: Burden and Trends. Cancer Epidemiol Biomarkers Prev 2017;26:444-57. [Crossref] [PubMed]
- Jiao DC, Qiao JH, Zhu JJ, Wang LN, Ma YZ, Lu ZD, Liu ZZ. Analysis of factors influencing the axillary lymph node metastasis and breast cancer-specific survival in patients with T1 breast cancer. Zhonghua Yi Xue Za Zhi 2018;98:3258-62. [PubMed]
- Burnside ES, Drukker K, Li H, Bonaccio E, Zuley M, Ganott M, Net JM, Sutton EJ, Brandt KR, Whitman GJ, Conzen SD, Lan L, Ji Y, Zhu Y, Jaffe CC, Huang EP, Freymann JB, Kirby JS, Morris EA, Giger ML. Using computer-extracted image phenotypes from tumors on breast magnetic resonance imaging to predict breast cancer pathologic stage. Cancer 2016;122:748-57. [Crossref] [PubMed]
- Allgood PC, Duffy SW, Kearins O, O'Sullivan E, Tappenden N, Wallis MG, Lawrence G. Explaining the difference in prognosis between screen-detected and symptomatic breast cancers. Br J Cancer 2011;104:1680-5. [Crossref] [PubMed]
- Petrelli F, Viale G, Cabiddu M, Barni S. Prognostic value of different cut-off levels of Ki-67 in breast cancer: a systematic review and meta-analysis of 64,196 patients. Breast Cancer Res Treat 2015;153:477-491. [Crossref] [PubMed]
- Kontzoglou K, Palla V, Karaolanis G, Karaiskos I, Alexiou I, Pateras I, Konstantoudakis K, Stamatakos M. Correlation between Ki67 and breast cancer prognosis. Oncology 2013;84:219-225. [Crossref] [PubMed]
- Wintzer HO, Zipfel I, Schulte-Monting J, Hellerich U, von Kleist S. Ki-67 immunostaining in human breast tumors and its relationship to prognosis. Cancer 1991;67:421-8. [Crossref] [PubMed]
- Surov A, Meyer HJ, Wienke A. Can apparent diffusion coefficient (ADC) distinguish breast cancer from benign breast findings? A meta-analysis based on 13 847 lesions. BMC Cancer 2019;19:955. [Crossref] [PubMed]
- Surov A, Meyer HJ, Wienke A. Correlation between apparent diffusion coefficient (ADC) and cellularity is different in several tumors: a meta-analysis. Oncotarget 2017;8:59492-9. [Crossref] [PubMed]
- Surov A, Meyer HJ, Wienke A. Correlation Between Minimum Apparent Diffusion Coefficient (ADCmin) and Tumor Cellularity: A Meta-analysis. Anticancer Res 2017;37:3807-10. [PubMed]
- Surov A, Meyer HJ, Wienke A. Associations between apparent diffusion coefficient (ADC) and KI 67 in different tumors: a meta-analysis. Part 1: ADCmean. Oncotarget 2017;8:75434-44. [Crossref] [PubMed]
- Bollineni VR, Kramer G, Liu Y, Melidis C, DeSouza NM. A literature review of the association between diffusion-weighted MRI derived apparent diffusion coefficient and tumour aggressiveness in pelvic cancer. Cancer Treat Rev 2015;41:496-502. [Crossref] [PubMed]
- Padhani AR, Liu G, Koh DM, Chenevert TL, Thoeny HC, Takahara T, Dzik-Jurasz A, Ross BD, Van Cauteren M, Collins D, Hammoud DA, Rustin GJ, Taouli B, Choyke PL. Diffusion-weighted magnetic resonance imaging as a cancer biomarker: consensus and recommendations. Neoplasia 2009;11:102-25. [Crossref] [PubMed]
- Suo S, Cheng F, Cao M, Kang J, Wang M, Hua J, Hua X, Li L, Lu Q, Liu J, Xu J. Multiparametric diffusion-weighted imaging in breast lesions: Association with pathologic diagnosis and prognostic factors. J Magn Reson Imaging 2017;46:740-50. [Crossref] [PubMed]
- Mori N, Ota H, Mugikura S, Takasawa C, Ishida T, Watanabe G, Tada H, Watanabe M, Takase K, Takahashi S. Luminal-type breast cancer: correlation of apparent diffusion coefficients with the Ki-67 labeling index. Radiology 2015;274:66-73. [Crossref] [PubMed]
- Choi SY, Chang YW, Park HJ, Kim HJ, Hong SS, Seo DY. Correlation of the apparent diffusion coefficiency values on diffusion-weighted imaging with prognostic factors for breast cancer. Br J Radiol 2012;85:e474-9. [Crossref] [PubMed]
- Martincich L, Deantoni V, Bertotto I, Redana S, Kubatzki F, Sarotto I, Rossi V, Liotti M, Ponzone R, Aglietta M, Regge D, Montemurro F. Correlations between diffusion-weighted imaging and breast cancer biomarkers. Eur Radiol 2012;22:1519-28. [Crossref] [PubMed]
- Jeh SK, Kim SH, Kim HS, Kang BJ, Jeong SH, Yim HW, Song BJ. Correlation of the apparent diffusion coefficient value and dynamic magnetic resonance imaging findings with prognostic factors in invasive ductal carcinoma. J Magn Reson Imaging 2011;33:102-9. [Crossref] [PubMed]
- Kim SH, Cha ES, Kim HS, Kang BJ, Choi JJ, Jung JH, Park YG, Suh YJ. Diffusion-weighted imaging of breast cancer: correlation of the apparent diffusion coefficient value with prognostic factors. J Magn Reson Imaging 2009;30:615-20. [Crossref] [PubMed]
- Kim SY, Kim EK, Moon HJ, Yoon JH, Koo JS, Kim SG, Kim MJ. Association among T2 signal intensity, necrosis, ADC and Ki-67 in estrogen receptor-positive and HER2-negative invasive ductal carcinoma. Magn Reson Imaging 2018;54:176-82. [Crossref] [PubMed]
- Just N. Improving tumour heterogeneity MRI assessment with histograms. Br J Cancer 2014;111:2205-13. [Crossref] [PubMed]
- Peng Y, Tang H, Meng X, Shen Y, Hu D, Kamel I, Li Z. Histological grades of rectal cancer: whole-volume histogram analysis of apparent diffusion coefficient based on reduced field-of-view diffusion-weighted imaging. Quant Imaging Med Surg 2020;10:243-56. [Crossref] [PubMed]
- Meyer HJ, Gundermann P, Hohn AK, Hamerla G, Surov A. Associations between whole tumor histogram analysis parameters derived from ADC maps and expression of EGFR, VEGF, Hif 1-alpha, Her-2 and Histone 3 in uterine cervical cancer. Magn Reson Imaging 2019;57:68-74. [Crossref] [PubMed]
- Wang K, Cheng J, Wang Y, Wu G. Renal cell carcinoma: preoperative evaluate the grade of histological malignancy using volumetric histogram analysis derived from magnetic resonance diffusion kurtosis imaging. Quant Imaging Med Surg 2019;9:671-80. [Crossref] [PubMed]
- Meyer HJ, Hohn A, Surov A. Histogram analysis of ADC in rectal cancer: associations with different histopathological findings including expression of EGFR, Hif1-alpha, VEGF, p53, PD1, and KI 67. A preliminary study. Oncotarget 2018;9:18510-7. [Crossref] [PubMed]
- Surov A, Hamerla G, Meyer HJ, Winter K, Schob S, Fiedler E. Whole lesion histogram analysis of meningiomas derived from ADC values. Correlation with several cellularity parameters, proliferation index KI 67, nucleic content, and membrane permeability. Magn Reson Imaging 2018;51:158-62. [Crossref] [PubMed]
- Liang HY, Huang YQ, Yang ZX. Ying-Ding, Zeng MS, Rao SX. Potential of MR histogram analyses for prediction of response to chemotherapy in patients with colorectal hepatic metastases. Eur Radiol 2016;26:2009-18. [Crossref] [PubMed]
- Zhang F, Shi M, Liao Q, Zhou CM. Clinicopathologic features of secretory breast carcinoma. Zhonghua Zhong Liu Za Zhi 2019;41:628-632. [PubMed]
- Hammond ME, Hayes DF, Dowsett M, Allred DC, Hagerty KL, Badve S, Fitzgibbons PL, Francis G, Goldstein NS, Hayes M, Hicks DG, Lester S, Love R, Mangu PB, McShane L, Miller K, Osborne CK, Paik S, Perlmutter J, Rhodes A, Sasano H, Schwartz JN, Sweep FC, Taube S, Torlakovic EE, Valenstein P, Viale G, Visscher D, Wheeler T, Williams RB, Wittliff JL, Wolff AC. American Society of Clinical Oncology/College Of American Pathologists guideline recommendations for immunohistochemical testing of estrogen and progesterone receptors in breast cancer. J Clin Oncol 2010;28:2784-95. [Crossref] [PubMed]
- Pathmanathan N, Balleine RL. Ki67 and proliferation in breast cancer. J Clin Pathol 2013;66:512-6. [Crossref] [PubMed]
- Jackisch C, Harbeck N, Huober J, von Minckwitz G, Gerber B, Kreipe HH, Liedtke C, Marschner N, Mobus V, Scheithauer H, Schneeweiss A, Thomssen C, Loibl S, Beckmann MW, Blohmer JU, Costa SD, Decker T, Diel I, Fasching PA, Fehm T, Janni W, Luck HJ, Maass N, Scharl A, Untch M. 14th St. Gallen International Breast Cancer Conference 2015: Evidence, Controversies, Consensus - Primary Therapy of Early Breast Cancer: Opinions Expressed by German Experts. Breast Care (Basel) 2015;10:211-9. [Crossref] [PubMed]
- DeLong ER, DeLong DM, Clarke-Pearson DL. Comparing the areas under two or more correlated receiver operating characteristic curves: a nonparametric approach. Biometrics 1988;44:837-45. [Crossref] [PubMed]
- Hu XX, Yang ZX, Liang HY, Ding Y, Grimm R, Fu CX, Liu H, Yan X, Ji Y, Zeng MS, Rao SX. Whole-tumor MRI histogram analyses of hepatocellular carcinoma: Correlations with Ki-67 labeling index. J Magn Reson Imaging 2017;46:383-92. [Crossref] [PubMed]
- Cheang MC, Chia SK, Voduc D, Gao D, Leung S, Snider J, Watson M, Davies S, Bernard PS, Parker JS, Perou CM, Ellis MJ, Nielsen TO. Ki67 index, HER2 status, and prognosis of patients with luminal B breast cancer. J Natl Cancer Inst 2009;101:736-50. [Crossref] [PubMed]
- Bediaga NG, Beristain E, Calvo B, Viguri MA, Gutierrez-Corres B, Rezola R, Ruiz-Diaz I, Guerra I, de Pancorbo MM. Luminal B breast cancer subtype displays a dicotomic epigenetic pattern. Springerplus 2016;5:623. [Crossref] [PubMed]
- Wiesner FG, Magener A, Fasching PA, Wesse J, Bani MR, Rauh C, Jud S, Schrauder M, Loehberg CR, Beckmann MW, Hartmann A, Lux MP. Ki-67 as a prognostic molecular marker in routine clinical use in breast cancer patients. Breast 2009;18:135-41. [Crossref] [PubMed]
- Ryu JM, Lee HJ, Yoon TI, Lee ES, Lee SJ, Jung JH, Chae BJ, Nam SJ, Lee JE, Lee SK, Bae SY, Yu J, Kim SW. Breast cancer-specific mortality in small-sized tumor with node-positive breast cancer: a nation-wide study in Korean breast cancer society. Breast Cancer Res Treat 2016;159:489-98. [Crossref] [PubMed]
- Kim EJ, Kim SH, Park GE, Kang BJ, Song BJ, Kim YJ, Lee D, Ahn H, Kim I, Son YH, Grimm R. Histogram analysis of apparent diffusion coefficient at 3.0t: Correlation with prognostic factors and subtypes of invasive ductal carcinoma. J Magn Reson Imaging 2015;42:1666-78. [Crossref] [PubMed]
- Ahn SJ, Choi SH, Kim YJ, Kim KG, Sohn CH, Han MH, Chang KH, Min HS. Histogram analysis of apparent diffusion coefficient map of standard and high B-value diffusion MR imaging in head and neck squamous cell carcinoma: a correlation study with histological grade. Acad Radiol 2012;19:1233-40. [Crossref] [PubMed]
- Tang Q, Li Q, Xie D, Chu K, Liu L, Liao C, Qin Y, Wang Z, Su D. An Apparent Diffusion Coefficient Histogram Method Versus a Traditional 2-Dimensional Measurement Method for Identifying Non-Puerperal Mastitis From Breast Cancer at 3.0 T. J Comput Assist Tomogr 2018;42:776-83. [Crossref] [PubMed]
- Wang W, Cheng J, Zhang Y, Wang C. Use of Apparent Diffusion Coefficient Histogram in Differentiating Between Medulloblastoma and Pilocytic Astrocytoma in Children. Med Sci Monit 2018;24:6107-12. [Crossref] [PubMed]
- Hirata A, Hayano K, Ohira G, Imanishi S, Hanaoka T, Murakami K, Aoyagi T, Shuto K, Matsubara H. Volumetric histogram analysis of apparent diffusion coefficient for predicting pathological complete response and survival in esophageal cancer patients treated with chemoradiotherapy. Am J Surg 2020;219:1024-9. [Crossref] [PubMed]
- Li HM, Zhang R, Gu WY, Zhao SH, Lu N, Zhang GF, Peng WJ, Qiang JW. Whole solid tumour volume histogram analysis of the apparent diffusion coefficient for differentiating high-grade from low-grade serous ovarian carcinoma: correlation with Ki-67 proliferation status. Clin Radiol 2019;74:918-925. [Crossref] [PubMed]
- Ren JL, Yuan Y, Li XX, Shi YQ, Tao XF. Histogram analysis of apparent diffusion coefficient maps in the prognosis of patients with locally advanced head and neck squamous cell carcinoma: Comparison of different region of interest selection methods. Eur J Radiol 2018;106:7-13. [Crossref] [PubMed]
- Suo ST, Chen XX, Fan Y, Wu LM, Yao QY, Cao MQ, Liu Q, Xu JR. Histogram analysis of apparent diffusion coefficient at 3.0 T in urinary bladder lesions: correlation with pathologic findings. Acad Radiol 2014;21:1027-34. [Crossref] [PubMed]
- Xue H, Ren C, Yang J, Sun Z, Li S, Jin Z, Shen K, Zhou W. Histogram analysis of apparent diffusion coefficient for the assessment of local aggressiveness of cervical cancer. Arch Gynecol Obstet 2014;290:341-8. [Crossref] [PubMed]
- Shen L, Zhou G, Tong T, Tang F, Lin Y, Zhou J, Wang Y, Zong G, Zhang L. ADC at 3.0T as a noninvasive biomarker for preoperative prediction of Ki67 expression in invasive ductal carcinoma of breast. Clin Imaging 2018;52:16-22. [Crossref] [PubMed]
- Molinari C, Clauser P, Girometti R, Linda A, Cimino E, Puglisi F, Zuiani C, Bazzocchi M. MR mammography using diffusion-weighted imaging in evaluating breast cancer: a correlation with proliferation index. Radiol Med 2015;120:911-8. [Crossref] [PubMed]
- Zhang H, Sui X, Zhou S, Hu L, Huang X. Correlation of Conventional Ultrasound Characteristics of Breast Tumors With Axillary Lymph Node Metastasis and Ki-67 Expression in Patients With Breast Cancer. J Ultrasound Med 2019;38:1833-40. [Crossref] [PubMed]
- Li X, Yuan Y, Ren J, Shi Y, Tao X. Incremental Prognostic Value of Apparent Diffusion Coefficient Histogram Analysis in Head and Neck Squamous Cell Carcinoma. Acad Radiol 2018;25:1433-8. [Crossref] [PubMed]
- You C, Li J, Zhi W, Chen Y, Yang W, Gu Y, Peng W. The volumetric-tumour histogram-based analysis of intravoxel incoherent motion and non-Gaussian diffusion MRI: association with prognostic factors in HER2-positive breast cancer. J Transl Med 2019;17:182. [Crossref] [PubMed]
- Tong G, Zhang G, Liu J, Zheng Z, Chen Y, Niu P, Xu X. Cutoff of 25% for Ki67 expression is a good classification tool for prognosis in colorectal cancer in the AJCC8 stratification. Oncol Rep 2020;43:1187-98. [PubMed]
- Ng F, Kozarski R, Ganeshan B, Goh V. Assessment of tumor heterogeneity by CT texture analysis: can the largest cross-sectional area be used as an alternative to whole tumor analysis? Eur J Radiol 2013;82:342-8. [Crossref] [PubMed]