The potential role of novel diffusion imaging techniques in the understanding and treatment of epilepsy
Introduction
Epilepsy is a common neurological disorder for which magnetic resonance imaging plays a key role in the investigation and management. Around 20-30% of patients with focal epilepsy have normal conventional magnetic resonance imaging (1) implying that current techniques are insensitive to underlying pathological changes. The availability of increasing magnetic field strengths, improved coil technology and novel acquisition and analysis techniques should benefit the diagnostic yield but in general novel contrasts have been disappointing.
A variety of techniques are now available that offer the opportunity to better characterise both structural (i.e. tissue microstructure) and functional (i.e., tissue metabolism) alterations. Arterial spin labelling (ASL) non-invasively evaluates cerebral blood flow (2) and may more easily provide information that is currently obtained by nuclear medicine techniques such as SPECT. Susceptibility-weighted imaging (SWI) is sensitive to brain iron content and may improve the identification of lesions such as small cavernomas (3). Chemical exchange saturation transfer (CEST) is sensitive to the exchange of protons between different pools of molecules. Glucose uptake can be measured in vivo using unlabelled glucose and the exchange of protons between hydroxyl groups and water (GlucoCEST) (4). This has the potential to improve the spatial resolution of FDG-PET.
Diffusion imaging
One area of magnetic resonance imaging that has seen a vast increase in its clinical and research applications is diffusion imaging. The contrast in diffusion imaging is derived from the molecular diffusion of water leading to an attenuation of the signal. Diffusion-weighted imaging was first used to identify the ischaemic area in acute stroke (5) but it was soon discovered that diffusion within white matter was anisotropic (i.e., occurring at different rates in different directions) (6).
Diffusion tensor imaging (DTI) is an extension in which diffusion is quantified in several different directions and a model represented by a tensor is then applied to the diffusion within each voxel (7). Several parameters can be derived from the tensor, including fractional anisotropy (FA) and mean diffusivity (MD), which are felt to represent alterations in the underlying tissue microstructure. Whilst these measures are relatively sensitive, they are non-specific.
Diffusion imaging has limitations derived from both the acquisition (e.g., spatial resolution and distortions from magnetic susceptibility) and in the model applied. DTI is based on a fundamental assumption of a single diffusion process within each voxel that follows a Gaussian distribution. In reality the relatively large size of a voxel (typically 2 mm isotropic) means that each voxel may contain a mixture of grey matter, white matter and cerebrospinal fluid (CSF) of varying orientations. Moreover, diffusion may deviate from a Gaussian process due to barriers such as the cell membrane.
A variety of more advanced models of tissue diffusion are now available that yield estimates of alternative parameters which may be helpful in both identifying a previously unseen focal lesion in MRI-negative epilepsy and in improving the understanding of the underlying microstructural changes. In this review, I discuss the principle and potential utility of three such techniques: Diffusional Kurtosis Imaging (DKI), Composite Hindered and Restricted Model of Diffusion (CHARMED) and Neurite Orientation Dispersion and Density Imaging (NODDI).
Diffusional kurtosis imaging (DKI)
Principle of method
As stated above, DTI assumes that diffusion follows a Gaussian profile and is thus unrestricted. With increasing diffusion time, barriers such as the cell membrane restrict the diffusion of water such that the diffusion profile no longer remains Gaussian. DKI characterises the degree to which diffusion deviates from Gaussian behaviour in order to better understand the tissue microstructure (8). Although related to q-space imaging, it can be implemented in a clinically feasible scan lasting under 10 minutes as whilst q-space imaging seeks to estimate the full diffusion displacement probability distribution, DKI just measures the kurtosis and hence is less demanding on magnetic field gradients and acquisition time.
The kurtosis can be represented by a rank 4 tensor with 15 independent components and thus requires diffusion-weighted images with at least three b values (e.g., 0, 1,000, 2,000 s/mm2) and 15 independent directions. In contrast, the diffusion tensor is a rank 2 tensor with six component components that requires only three b values (e.g., 0, 1,000 s/mm2) and six independent directions.
In the same way that MD, axial diffusivity (AD) and radial diffusivity (RD) can be derived from the diffusion tensor, three additional measures can be derived from the kurtosis tensor—mean kurtosis (MK), the average diffusion kurtosis in all directions; axial kurtosis (AK), the kurtosis along the axial direction of the diffusion ellipsoid and radial kurtosis (RK), the kurtosis along the radial direction (9).
The MK from DKI shows a greater contrast between grey and white matter than the corresponding MD from DTI. Further, in relatively more isotropic tissue such as grey matter, MK may be more sensitive than FA since the latter only reflects tissue structure when it is spatially orientated. DKI metrics also appear less sensitive to contamination from CSF. Despite these advances, the exact biological underpinning of these novel parameters remains unclear.
Application to epilepsy
Although the original description of DKI suggested it would be useful for diseases with white matter abnormalities, such as epilepsy (8), the technique has been little studied in this population. It has found more widespread use in other conditions such as stroke and tumours (10).
In patients with temporal lobe epilepsy (TLE), widespread alterations in DTI parameters (typically reduced FA and increased MD) are found predominantly in the ipsilateral temporal lobe but also in some extratemporal regions (reviewed elsewhere in this issue). DKI has been applied in such populations alongside DTI and found to be more sensitive.
The first published study was a small preliminary study in children with TLE and normal conventional MRI involving 8 patients and 8 healthy controls (11). DTI and DKI were compared using a region-of-interest encompassing the whole temporal lobe with grey and white matter analysed separately. In white matter, MD, AD and AK were reduced but this was a region-of-interest based analysis rather than voxel-based and the study was underpowered since it also failed to detect the widespread changes in FA seen in prior studies. In grey matter, both MD and MK were increased suggesting the technique should be studied further. A subsequent voxel-based analysis of a heterogenous group of children with bilateral epileptic discharges showed widespread complementary changes in DTI and DKI parameters in relation to healthy controls, including reduced MK (12).
A much larger voxel-based study comparing 32 adults with left TLE to 36 healthy controls demonstrated more widespread changes in DKI parameters than DTI suggesting that DKI provides more sensitive and complementary measures (13). In white matter, the previous reported findings of reduced FA and increased MD were replicated whilst more widespread reduction in MK and RK was observed particularly in the left temporal, bilateral orbitofrontal and left frontoparietal regions (Figure 1). In grey matter, increases in MD and RD were observed alongside reductions in MK, AK and RK in varying regions.
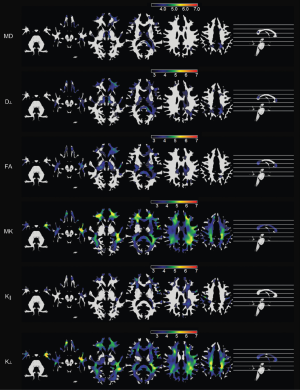
The same group also looked at specific tracts in 25 patients with TLE in comparison to 36 controls in order to compare the effects on early (posterior limb of internal capsule, cerebral peduncle) versus late myelinating tracts (superior longitudinal fasciculus, inferior longitudinal fasciculus) (14). Reductions in FA, MK and RK were observed particularly for later myelinating tracts. They reported that MK and RK were more sensitive than FA with changes in RK being more marked in later myelinating tracts.
DKI has also been applied to idiopathic generalised epilepsy (IGE), where conventional imaging is normal but the underlying deficit is felt to involve thalamo-cortical dysfunction. Voxel-based analysis with Tract-Based Spatial Statistics (TBSS) revealed increased MD and RD in the body and splenium of the corpus callosum, with reduced FA in the internal capsule and splenium suggesting involving of thalamo-cortical projections (15). Reductions in MK and RK were much more widespread encompassing the anterior and superior corona radiata, anterior and posterior limb of the internal capsule, corpus callosum, superior longitudinal fasciculus and posterior thalamic radiation and thus both thalamo-cortical and cortico-cortical projections (Figure 2).
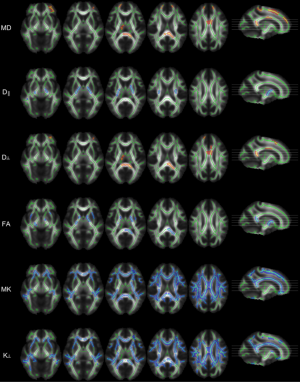
Potential and future developments
Studies to date suggest that parameters from DKI are more sensitive to underlying changes in both focal and generalised epilepsies, but it remains unclear what these parameters represent and how they correlate with underlying histology. It has been suggested that DKI parameters are related to tissue properties of intra- and extra-axonal fractions, such as axonal water fraction, axonal diffusivity and extra-axonal tortuosity (16) but this needs validation.
The predominant finding so far is of reduced kurtosis in disease suggesting a loss of diffusional heterogeneity and by inference some damage to the underlying tissue microstructure that needs further elucidation. This is the converse of increasing kurtosis with age and maturation as observed in both white and grey matter in rodent models (17). DKI may yield a better understanding of the underlying pathological changes in epilepsy, and such changes have the potential to be used as a biomarker or to predict outcome from surgery.
Improving the diffusion model
A second approach to improving the modelling of diffusion is to recognise that, given a voxel dimension several orders of magnitude larger than the underlying tissue structure, the observed signal will be a composite from several different tissue compartments (e.g., intra-axonal, extra-axonal).
There are many different approaches to compartment models that vary in their complexity (18). In general, the more complex a model the better fit to the observed data that is achieved. However this is at the expense of the necessity for more data to be collected in order to fit the model which translates into a longer scan duration. For clinical utility, a model is required that has fitted parameters with a plausible biological meeting and which can be obtained with a scan of clinically feasible duration (for example, less than 20 minutes).
The general approach is to consider each voxel as a mixture of different compartments (typically two or three) and to apply a different mathematical model to each compartment depending on its properties. The idea is to produce a descriptive model of the tissue in which specific parameters such as cell size and density can be related to signal. Two such models, CHARMED and NODDI, are discussed here.
Composite Hindered and Restricted Model of Diffusion (CHARMED)
Principle of method
In this model, the signal from each voxel is considered as arising from two compartments, the extra-axonal and (one or more) intra-axonal fractions (19). The extra-axonal fraction is modelled as hindered (Gaussian) diffusion with a diffusion tensor, whilst the intra-axonal fractions is modelled as impermeable cylinders with restricted (non-Gaussian) diffusion based on work by Neuman (20).
The model requires an acquisition with multiple b values (multiple shells) which is demanding. However recent work on optimisation of the sampling scheme has reduced the time requirement to 12 minutes for whole brain coverage (21).
Potential and future developments
The model introduces several new parameters that may be of interest. In particular, the population fractions of the restricted and hindered components may be sensitive to white matter disorders. The restricted fraction is considered a marker of axonal density, whilst intra-axonal longitudinal diffusivity may better characterise axonal health. Such parameters have the potential to be more specific and provide greater understanding of the underlying disorder.
Indices derived from CHARMED have shown greater sensitivity for changes during plasticity than those from DTI (22). However to date, no studies using CHARMED in patients with epilepsy have been performed but promising results from another technique described below, NODDI, suggest that it should be explored further.
The original CHARMED model assumes the intra-axonal fraction has cylinders with radii following a (fixed) gamma distribution. AxCaliber is an extension to CHARMED incorporating a diameter distribution of the axons that is estimated (23). It does however rely on a fixed gradient direction perpendicular to the axon so can only be applied in structures such as the corpus callosum. These techniques need to be developed further before they could be used in humans, but a window in the axon diameter distribution might provide further microstructural information of interest.
Neurite Orientation Dispersion and Density Imaging (NODDI)
Principle
One of the common criticisms of the metrics derived from DTI is that whilst sensitive they are non-specific to underlying pathological changes. A key part of the anatomy and morphology of the brain is the dendrites and axons, collectively termed neurites. Information regarding their morphology in both white and grey matter would be advantageous.
Both the DTI and CHARMED models fail to account for the dispersion of axonal orientation, as both assume a single orientation within each voxel. NODDI aims to introduce a model sufficient to capture the morphology of neurites in vivo whilst ensuring that it remains simple enough to be acquired in a clinically feasible duration (24).
Each voxel is modelled as a combination of three compartments. The intracellular component (restricted diffusion) is modelled as sticks (cylinders of zero radius) in contrast to CHARMED where there is an orientation distribution for the radii. The orientation of the sticks is modelled by a Watson distribution to account for dispersion ranging from highly parallel structures such as the corpus callosum to wide dispersion such as within grey matter. The extracellular component (hindered diffusion) is modelled by Gaussian anisotropic diffusion. There is an additional third component, the CSF, modelled by isotropic diffusion.
The model has been shown to work well with a two shell protocol and relatively low b values and thus can be easily acquired on a clinical scanner in under 20 minutes (24). The additional CSF compartment minimises the contamination from this compartment and the inclusion of two full shells is beneficial as the acquired data may also be used for DTI and other models useful for tractography such as constrained spherical deconvolution (CSD) (25). The incorporation of the orientation dispersion of neurites means the model is equally applicable to white and grey matter, but necessary simplification means that no account is made of the axon diameter distribution.
The two key additional parameters that are obtained are intracellular volume fraction (ICVF) expressed as a fraction of the non-CSF compartment and the orientation dispersion index (ODI), which is calculated from the concentration parameter of the Watson distribution in such a way as to give a figure ranging from 0 (no dispersion of neurites) to 1 (fully dispersed neurites). Both of these parameters can affect FA and the method enables their differing effects to be disentangled.
Application to epilepsy
An exploratory study has suggested that NODDI may be beneficial in identifying the seizure focus in patients with focal cortical dysplasia (FCD). In some types of FCD, ICVF is reduced (26) which could potentially be detected in imaging. In five patients with suspected dysplasia, a consistent finding of reduced ICVF was noted which was more readily identifiable that on other clinical or diffusion imaging sequences (Figure 3), as evidenced by an increased contrast-to-noise ratio (27).

Potential and future developments
This study needs to be replicated in a larger cohort and applied to different types of cortical malformation and patients who are MR negative. Like the other techniques presented in this paper, the derived parameters needs validation with post-operative histology.
The NODDI model can be further enhanced, for example by incorporating more advanced distributions for the neurite orientation and also to cope with multiple axonal populations, in the same way that can be achieved with CHARMED.
Conclusions
The diffusion tensor model has served the imaging community well for a couple of decades, but the parameters are non-specific and the model is limited by the assumption of a single Gaussian diffusion process within a relatively large voxel. Advantages in imaging techniques now enable us to go beyond this model and apply more advanced models.
DKI characterises the deviation from Gaussian diffusion and the measured kurtosis may give an indication of the degree of tissue complexity. It is the most studied technique in epilepsy to date showing promise to be sensitive to detect changes in both focal and generalised epilepsies.
A second approach is multi-compartment models. CHARMED separates intra- and extra-axonal fractions and can give measures such as axonal density that appear useful in other conditions but are yet to be applied to epilepsy. NODDI can measure and disentangle two key factors contributing to the FA, namely ICVF and orientation dispersion. Preliminary data suggest it may be more sensitive to detect focal abnormalities than DTI.
All these novel techniques are still being developed and may be adapted and improved over time. Whilst the parameters obtained are of potential interest, all techniques need histological validation to clarify what the fitted parameters represent on a microstructural level.
Disclosure: The author declares no conflict of interest.
References
- Duncan JS. Imaging in the surgical treatment of epilepsy. Nat Rev Neurol 2010;6:537-50. [PubMed]
- Alsop DC, Detre JA. Multisection cerebral blood flow MR imaging with continuous arterial spin labeling. Radiology 1998;208:410-6. [PubMed]
- Haacke EM, Mittal S, Wu Z, Neelavalli J, Cheng YC. Susceptibility-weighted imaging: technical aspects and clinical applications, part 1. AJNR Am J Neuroradiol 2009;30:19-30. [PubMed]
- Walker-Samuel S, Ramasawmy R, Torrealdea F, Rega M, Rajkumar V, Johnson SP, Richardson S, Gonçalves M, Parkes HG, Arstad E, Thomas DL, Pedley RB, Lythgoe MF, Golay X. In vivo imaging of glucose uptake and metabolism in tumors. Nat Med 2013;19:1067-72. [PubMed]
- Moseley ME, Cohen Y, Mintorovitch J, Chileuitt L, Shimizu H, Kucharczyk J, Wendland MF, Weinstein PR. Early detection of regional cerebral ischemia in cats: comparison of diffusion- and T2-weighted MRI and spectroscopy. Magn Reson Med 1990;14:330-46. [PubMed]
- Moseley ME, Cohen Y, Kucharczyk J, Mintorovitch J, Asgari HS, Wendland MF, Tsuruda J, Norman D. Diffusion-weighted MR imaging of anisotropic water diffusion in cat central nervous system. Radiology 1990;176:439-45. [PubMed]
- Winston GP. The physical and biological basis of quantitative parameters derived from diffusion MRI. Quant Imaging Med Surg 2012;2:254-65. [PubMed]
- Jensen JH, Helpern JA, Ramani A, Lu H, Kaczynski K. Diffusional kurtosis imaging: the quantification of non-gaussian water diffusion by means of magnetic resonance imaging. Magn Reson Med 2005;53:1432-40. [PubMed]
- Jensen JH, Helpern JA. MRI quantification of non-Gaussian water diffusion by kurtosis analysis. NMR Biomed 2010;23:698-710. [PubMed]
- Steven AJ, Zhuo J, Melhem ER. Diffusion kurtosis imaging: an emerging technique for evaluating the microstructural environment of the brain. AJR Am J Roentgenol 2014;202:W26-33. [PubMed]
- Gao Y, Zhang Y, Wong CS, Wu PM, Zhang Z, Gao J, Qiu D, Huang B. Diffusion abnormalities in temporal lobes of children with temporal lobe epilepsy: a preliminary diffusional kurtosis imaging study and comparison with diffusion tensor imaging. NMR Biomed 2012;25:1369-77. [PubMed]
- Zhang Y, Yan X, Gao Y, Xu D, Wu J, Li Y. A preliminary study of epilepsy in children using diffusional kurtosis imaging. Clin Neuroradiol 2013;23:293-300. [PubMed]
- Bonilha L, Lee CY, Jensen JH, Tabesh A, Spampinato MV, Edwards JC, Breedlove J, Helpern JA. Altered Microstructure in Temporal Lobe Epilepsy: A Diffusional Kurtosis Imaging Study. AJNR Am J Neuroradiol 2014. [Epub ahead of print]. [PubMed]
- Lee CY, Tabesh A, Benitez A, Helpern JA, Jensen JH, Bonilha L. Microstructural integrity of early- versus late-myelinating white matter tracts in medial temporal lobe epilepsy. Epilepsia 2013;54:1801-9. [PubMed]
- Lee CY, Tabesh A, Spampinato MV, Helpern JA, Jensen JH, Bonilha L. Diffusional kurtosis imaging reveals a distinctive pattern of microstructural alternations in idiopathic generalized epilepsy. Acta Neurol Scand 2014;130:148-55. [PubMed]
- Fieremans E, Jensen JH, Helpern JA. White matter characterization with diffusional kurtosis imaging. Neuroimage 2011;58:177-88. [PubMed]
- Cheung MM, Hui ES, Chan KC, Helpern JA, Qi L, Wu EX. Does diffusion kurtosis imaging lead to better neural tissue characterization? A rodent brain maturation study. Neuroimage 2009;45:386-92. [PubMed]
- Panagiotaki E, Schneider T, Siow B, Hall MG, Lythgoe MF, Alexander DC. Compartment models of the diffusion MR signal in brain white matter: a taxonomy and comparison. Neuroimage 2012;59:2241-54. [PubMed]
- Assaf Y, Basser PJ. Composite hindered and restricted model of diffusion (CHARMED) MR imaging of the human brain. Neuroimage 2005;27:48-58. [PubMed]
- Neuman CH. Spin echo of spins diffusing in a bounded medium. J Chem Phys 1974;60:4508-11.
- De Santis S, Assaf Y, Evans CJ, Jones DK. Improved precision in CHARMED assessment of white matter through sampling scheme optimization and model parsimony testing. Magn Reson Med 2013. [Epub ahead of print]. [PubMed]
- Tavor I, Hofstetter S, Assaf Y. Micro-structural assessment of short term plasticity dynamics. Neuroimage 2013;81:1-7. [PubMed]
- Assaf Y, Blumenfeld-Katzir T, Yovel Y, Basser PJ. AxCaliber: a method for measuring axon diameter distribution from diffusion MRI. Magn Reson Med 2008;59:1347-54. [PubMed]
- Zhang H, Schneider T, Wheeler-Kingshott CA, Alexander DC. NODDI: practical in vivo neurite orientation dispersion and density imaging of the human brain. Neuroimage 2012;61:1000-16. [PubMed]
- Tournier JD, Calamante F, Connelly A. Robust determination of the fibre orientation distribution in diffusion MRI: non-negativity constrained super-resolved spherical deconvolution. Neuroimage 2007;35:1459-72. [PubMed]
- Vargova L, Homola A, Cicanic M, Kuncova K, Krsek P, Marusic P, Sykova E, Zamecnik J. The diffusion parameters of the extracellular space are altered in focal cortical dysplasias. Neurosci Lett 2011;499:19-23. [PubMed]
- Winston GP, Micallef C, Symms MR, Alexander DC, Duncan JS, Zhang H. Advanced diffusion imaging sequences could aid assessing patients with focal cortical dysplasia and epilepsy. Epilepsy Res 2014;108:336-9. [PubMed]