Artificial intelligence-based vessel suppression for detection of sub-solid nodules in lung cancer screening computed tomography
Introduction
Lung cancer screening (LCS) with low-dose computed tomography (LDCT) is based on the premise that detecting early lung cancer in eligible at-risk subjects reduces mortality (1-5). The U.S. Preventive Services Task Force recommended LCS for a high-risk population in 2013. The American College of Radiology (ACR) adopted Lung-RADS as a tool to standardize reporting and recommendations for nodules detected on LCS (6). Early lung cancers seen as small pulmonary nodules may be either solid or sub-solid in attenuation. Approximately 10–31% small lung nodules are not detected on standard CT, and there is increased detection with multiplanar reformats (MPR) and maximum intensity projection (MIP) images (7).
Artificial intelligence (AI)-based approaches help improve the detection of lung nodules and cancer on chest radiographs and CT (8-10). Previous CT studies have reported variable sensitivities (70–83%) and false-positive rates (3–9.6/scan) for evaluation of pulmonary nodules with computer-aided detection (CADe) and computer-aided diagnosis (CADx) algorithms (7,11). CADe uses the recognition of image patterns to detect the presence of specific imaging findings, Whereas CADx analyzes radiographic findings to classify findings based on their imaging features (10) Recently introduced AI techniques use image segmentation, enhancement, feature extraction, and artificial neural networks to detect lung cancer from chest CT (12,13). Artificial neural network-based deep learning solutions have higher sensitivities (87–93%) and low false-positive rates for the detection of pulmonary nodules on chest CT (14,15).
Although most research has focused on the detection of solid pulmonary nodules, subsolid nodules (SSNs) are often more difficult to detect (16). SSNs, which include both ground-glass (GGNs) or part-solid (PSNs) nodules, have a higher likelihood of malignancy than solid nodules regardless of size (17). Lower attenuation makes the detection of these nodules difficult compared to solid nodules. Also, proximity to pulmonary vessels can also limit the detection of GGN or the identification of a solid component within PSN. We hypothesized that an AI-based vessel suppression (AI-VS) and detection algorithm (ClearRead CT Vessel Suppression and Detect, Riverain TechnologiesTM) can improve detection of GGN and PSN on chest CT images. We assessed the effect of AI-VS and AI-based automatic detection (AI-AD) on the detection of GGN and PSN in LDCT.
Methods
Patients
This retrospective study included 123 adult patients (69 women and 54 men; mean age =63±6 years) who underwent LDCT of the chest in the National Lung Cancer Screening Trial (NLST) and had reported lung nodules. From the NLST database of eligible patients, a study co-investigator (R Singh, with 5 years of experience) selected the first 125 patients with mixed attenuation or GGNs and 25 patients with no nodules. After excluding the chest CT with artifacts or which could not be processed by the software (n=27), the final dataset included 123 patients, 100 patients with SSNs (average diameter ≥6 mm (true positive) and less than 30mm), and 23 patients with no true SSNs on their chest CT (true negative). For each dominant nodule, the Lung-RADS category was assigned per Lung-RADS 1.1 (https://www.acr.org/-/media/ACR/Files/RADS/Lung-RADS/LungRADSAssessmentCategoriesv1-1.pdf) on standard CT images (Lung-RADS 2: n=43; Lung-RADS 3: n=7; Lung-RADS 4A: n=50), and reassigned on AI-VS images. Neither the standard of reference (SOR) radiologists nor the test radiologists took part in the selection of these patients. The selected LDCT exams were not used in training or validation of the assessed AI software (ClearRead CT, RiverainTM Inc.). Figure 1 shows the flowchart for data collection.
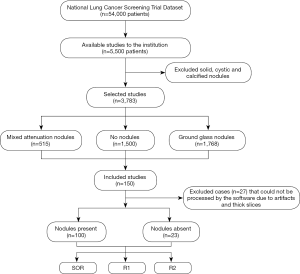
Patient demographics and radiology findings were recorded from the datasheets of NLST with encoded information on the attenuation, location, size (average dimension in millimeter), and helical slice location of the dominant lung nodule. Thin-section (1.2–2 mm) CT data were exported to an encrypted external portable hard drive for processing.
AI algorithm
Details of the AI algorithm applied in our study can be obtained from the United States Patent application number US20150279034A1 (https://patentimages.storage.googleapis.com/cd/f7/66/5b0fce93a2cadf/US20150279034A1.pdf. Accessed on January 6, 2020). We processed thin-section CT datasets for all the included 123 patients with a commercially available AI software (ClearRead CT software). The AI-based software uses proprietary machine learning techniques to suppress the lung vessels (AI-VS without automatic nodule detection feature) without introducing artifacts to aid in nodule detection and without the need for maximum or minimum intensity projection images. In addition, the software also detects and annotates (AI-AD) the dominant pulmonary nodules, regardless of their attenuation (solid and SSN). The AI-AD displays the detected nodules with their boundary contours and information about their CT numbers (Hounsfield units), minimum and maximum dimensions, and volume. The software is approved for commercial use by the United States Food and Drug Administration (FDA). Processing of LDCT exams with the AI software resulted in three image series for each patient—baseline standard CT (without vessel suppression or auto-detect), AI-VS (with vessel suppression without automatic nodule detection feature), and AI-AD (with vessel suppression and autodetection of pulmonary nodules) image series.
Image interpretation
All image series were reviewed on a commercial DICOM viewer (ClearCanvas Inc., Toronto, Canada) in lung window settings (window width 1,500, window level −600). The radiologists could adjust the window level and width according to their individual preferences. Two experienced thoracic subspecialty radiologists (with 11 and 27 years of experience in thoracic imaging) reviewed all the image series for the selected 123 patients to create the ground truth or the SOR for presence, location (lobe and image number), average size of the dominant nodule [(maximum dimension of the nodule in mm + orthogonal dimension in mm)/2], and attenuation of GGN and PSN ≥6 mm in average diameter. The dominant nodule was defined as the single largest GGN or PSN in each CT scan. Both radiologists were requested to ignore the totally solid and calcified pulmonary nodules. The SOR readouts were conducted in consensus; both radiologists had access to all image types for interpretation, and the discrepancy in 7% (9/123) cases was resolved with adjudication by a separate radiologist with 17 years of experience in cancer imaging. Separately, two test radiologists (C Nitiwarangkul with 5 years, and S McDermott with 10 years of thoracic CT experience) independently assessed each examination for the same attributes. Both test radiologists were blinded to the SOR interpretation. Each test radiologist assessed the images for SSNs separately and was instructed not to share their experience or findings. First, the test radiologists evaluated the standard CT images for presence, location (lobe and image number), average size, and attenuation of GGN and PSN ≥6 mm in size. Then, they evaluated the AI-VS images for the same attributes. For comparison with the AI-AD images, five dominant nodules per exam were considered. The size of the dominant nodule and the solid component, if present, were also recorded. The methodology of our study was similar to previous reported works (8,17). Another study by Jacobs et al. on-nodule detection using CAD had 4 test radiologists and one adjudicator for the results (18). The measurements were as per the criteria prescribed by Lung-RADS: the mean of two axes for the entire SSN, rounded to the nearest mm, and the long axis for the solid component (19). In a study, the mean radiologist’s interpretation time for chest CT without and with administration of intravenous iodinated contrast has been found 803 (±376 seconds) and 767 (±309 seconds) (20). We did not specifically assess the radiologist interpretation time for standard, AI-VS and AI-AD images. However, previous studies using the same algorithm have reported lesser interpretation time using AI-AD approach (15). Computer aided detection has shown to improve the radiologist’s efficiency and accuracy in lung nodule detection with minimal impact on interpretation time (21-23).
Statistical analyses
Data were recorded in Microsoft EXCEL worksheets (Microsoft Inc. 2016, Redmond, Washington, USA). Statistical analysis was performed using SPSS 24.0 (IBM SPSS Statistics Inc., Armonk, NY, USA). The area under the curve (AUC) of nodule detection by each test radiologist was calculated separately for GGNs and PSNs. The AUC was estimated from receiver operator characteristics (ROC) analyses to determine the AUC of the two test radiologists for the detection of total and dominant GGNs and PSNs. Since the AI-AD program detects up to five dominant nodules per CT examination, a stratified analysis was performed for which the detected nodules by SOR and test radiologists were capped at five dominant GGN and PSN per CT examination. Interobserver agreement between the two test radiologists was determined with Cohen’s Kappa analysis for the dominant nodule. Two tail Student’s t-test was used to determine statistical significance for detection of additional GGN and PSN. A P value of <0.05 was deemed statistically significant. Since the ROC is a non-parametric test, we did not compare ROC analysis with two tail Student’s t-test.
Results
Number of nodules
The two SOR radiologists detected 310 nodules (156 GGN and 154 PSN) in 100 chest CT examinations with the following distribution: no nodule (n=23 CT), one nodule (n=35 CT), 2 nodules (n=24), 3 nodules (n=6), 4 nodules (n=13), 5 nodules (n=5), 6 nodules (n=6), 7 nodules (n=3), 10 nodules (n=1); 12 nodules (n=2), 16 nodules (n=1), and 25 nodules (n=1). The two test radiologists detected 232 nodules (R1: 114 GGN, 118 PSN) and 255 nodules (R2: 122 GGN, 133 PSN) on the baseline, standard CT images (P values ≥0.08). Table 1 summarizes the respective accuracies for detection of GGN and PSN on standard CT images.

Full table
On AI-VS images, the two test radiologists detected 249 nodules (R1: 119 GGN, 130 PSN) and 277 nodules (R2: 128 GGN, 149 PSN) (P≥0.12). R1 detected 17 additional nodules, including 5 GGN and 12 PSN, and R2 detected 22 additional nodules, including 6 GGN and 16 PSN. Both test radiologists detected solid components in five PSN, which they had deemed as GGN on the standard CT images (Figure 2). The Lung-RADS category changed for five nodules from Lung-RADS 2 to Lung-RADS 4A. The average size of nodules correctly identified by AI-VS was 12±5 mm for GGN and 15±7 mm for PSN. The average size of missed nodules by AI-VS was 9±2 mm for GGN and 8±2 mm for PSN. The respective accuracies for the detection of nodules on AI-VS images are shown in Table 1. All nodules detected on the standard CT images were also present on the AI-VS images.
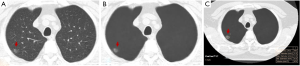
With the five dominant nodules per CT, SOR detected 237 nodules (111 GGN and 126 PSN). AI-AD annotated 113 (35 GGN, 78 PSN) nodules. In nine patients, AI-AD detected no pulmonary nodules. There were 10 false-positive nodules on AI-AD images (mediastinal fat 2, pleural thickening 1, airspace opacities 4, and focal atelectasis 3). The AUC, sensitivity, and specificity of AI-AD are summarized in Table 1.
Dominant nodules
The average sizes (± standard deviation) of dominant GGN and PSN were 12.7±5.0, and 15.7±7.0 mm, respectively. The average size of the solid component in PSN was 9±5 mm. There was no change in the dominant nodules between the standard CT and the AI-VS image series (Figure 3). ROC generated AUC for detection of dominant nodules on standard CT images are depicted in Figure 4. There was a substantial interobserver agreement between the test radiologists (kappa =0.63).
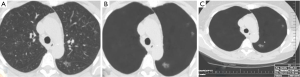
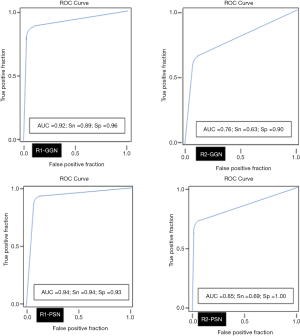
AI-AD led to a significant change in the dominant nodule in 26 patients for both test radiologists (P<0.0001). For the same dominant nodule, the size estimated by the AI-AD was 12±3 mm and by SOR was 14±5 mm (P=0.02). The AUC, sensitivity, and specificity of the detection of dominant PSN and GGN are summarized in Figure 5.
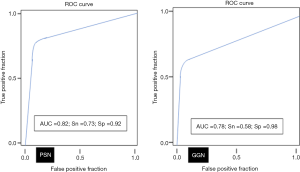
Discussion
Our study demonstrates the superiority of AI-VS over standard CT images for the detection of GGN and PSN in LDCT for LCS. Both test radiologists detected more GGN and PSN on the AI-VS images. The test radiologists detected 7–9% additional nodules on the AI-VS images than on the standard CT images. Both test radiologists also achieved better detection of PSN among the SSNs (0.81 AUC for R1 and 0.80 AUC for R2) compared to standard CT images (0.76 AUC for R1 and 0.70 AUC for R2).
The improved detection of PSN with AI-VS likely resulted from improved identification of solid components within PSN from the removal of pulmonary vessels, which obscure pulmonary nodules on standard chest CT images. Though both the test radiologists detected additional GGN on the AI-VS images compared to standard CT images, the improvement did not reach statistical significance (P>0.05) since there were fewer additional GGN compared to PSN. The GGNs are often difficult to identify as these lesions are faint, and subtle density GGN can be missed on standard CT. While AI-VS subtracts the vessels and helps make the faint GGN, more conspicuous as also our readers did detect additional nodules, the subtle GGN in close proximity to the vessel can be affected with change in relative pixel attenuation during vessel suppression. However, in our study the size of missed nodule was smaller as compared to detected GGN. A previous study with this algorithm did not separately assess the AI-VS and the AI-AD images for nodule detection (15).
All the Lung-RADS category determining lung nodules were identified on AI-VS images. However, AI-AD did not detect dominant nodules in 21% of patients. The performance of AI-AD was lower than the standard CT and AI-VS images (GGN AUC =0.60) and PSN AUC =0.72). These results are similar to the accuracy of 72.6% reported by Yuan et al. (17) as well as other studies with an accuracy of 54% of GGN and 72% of PSN (24). With the same AI-AD algorithm, Lo et al. reported an improved AUC =0.69 for the detection of all pulmonary nodules compared to the standard CT images (AUC =0.54) (15). Earlier studies have reported much lower sensitivities of 49% and 50% for GGN and PSN with other algorithms (25,26).
In our study, the false positive rate for AI-AD was 9% and related to mislabeling of mediastinal fat, pleural thickening, airspace opacities, and focal atelectasis as pulmonary nodules. Two prior studies have reported a 4–10% false-positive rate with CAD algorithms (27,28). In contrast to another study using the same algorithm (15) that did not have any false negatives, AI-AD in our study missed 7% of SSNs. A possible explanation for this discrepancy is the inclusion of subtle GGN and PSN.
The primary implication of our study is that the use of AI-enabled subtraction of pulmonary vessels increased the detection of GGN and PSN. While most prior studies and AI algorithms focus on automatic detection of solid lung nodules (17,24,25), our study expands the role of AI to image processing (with vessel suppression) to improve lung nodule detection by interpreting radiologists, before the application of automatic detection feature. Another implication pertains to improved detection of solid components within PSN with AI-VS, which were otherwise labeled as GGN. This characterization of nodules can change the Lung-RADS category and impact the management of patients. In the five patients, the category changed from Lung-RADS 2 to Lung-RADS 4A after the identification of solid components that were previously labeled as GGN. Improved detection and classification of nodules into PSN and GGN with AI-VS lead to accurate Lung-RADS categorization of nodules.
Although no dominant GGN or PSN was missed on the AI-VS images, 7% of non-dominant GGN and PSN were missed in our study. All missed nodules were smaller than the dominant nodules and did not impact the management of the patient or the Lung-RADS category. The miss rate for GGN and PSN in our study was similar to other studies (25,26). Further algorithm development and research are necessary to improve the automated detection of GGN and PSN on the LDCT of the chest.
Our study has limitations. All interpreting radiologists in our study were subspecialty fellowship-trained radiologists with over 5 years of subspecialty experience. Our results, therefore, may underestimate the advantage of AI-VS, when compared to a non-subspecialty-trained or general radiology practice. We did not compare the benefit of the AI-VS or AI-AD over the traditional non-AI based CADe algorithm or MIP images. Another limitation is the sequential review of images in our study from standard lung images to AI-VS and the AI-AD images, which may have affected the study results. Since there was no washout period between interpretation of baseline, AI-VS and AI-AD images, there is a possibility of recall bias in our study. However, as noted from our results section, sequential interpretation of these images without washout did not lead to any bias since some nodules were missed on both AI-VS and AI-AD images. Also, consecutive interpretation is in line with the practice in most institutions where standard lung images are reviewed before the review of processed images. All radiologists in our study specifically tested the LDCT examinations for GGN and PSN without the distraction of other lung or thoracic findings. This may have led to relatively modest improvement in the detection of GGN and PSN with AI-VS as compared to the real-life, clinical interpretation of LDCT, where radiologists have a different interpretation pattern. Although not ideal, the targeted approach used in our study ensures that there is no overestimation of the benefits of a detection algorithm, such as the AI-VS used in our study. We did not calculate the impact on image interpretation when AI-VS images are added to standard lung images. Reduction in interpretation time has been reported in prior studies using the same algorithm (13). The reason that we did not evaluate the radiologist’s image interpretation time was that we focused on the reader accuracy for detection of GGN and PSN using AI-VS and AI-AD images, and did not perform our study in a picture archiving and communication system (PACS) enabled monitor in an actual clinical workflow setting. Since the AI-AD feature detects only up to a maximum of five nodules per CT examination, it was not possible to estimate sensitivity for the detection of all lung nodules, if greater than five. Another limitation of our study is smaller sample size. We believe that adding more and diverse data from different datasets can impart better evaluation of the AI-VS and AI-AD. However, since this was a retrospective study done from NLST datasets, we were limited in our sample size. Since this was a retrospective research study done from NLST datasets and thus was limited in sample size with the images interpreted specifically by expert thoracic radiologists, we realized that the recall bias may not still be fully excluded, even with approaches which include intervening washout period and random combination and interpretation of standard, AI-VS and AI-AD images. Finally, 27/150 (18%) chest CT exams could not be processed with the AI algorithm since they had thicker sections and/or missing images in the downloaded datasets. We do not believe that these cases would have skewed or altered our study results.
To conclude, the AI-assisted vessel suppression algorithm improved the detection of both GGN and PSN for both readers compared to standard CT images. The algorithm enabled more accurate categorization of SSNs and assignment of Lung-RADS category through better delineation of solid component.
Acknowledgments
ClearRead CT Vessel Suppression and Detect, Riverain TechnologiesTM provided technical help for image processing but had no input in the selection or exclusion of cases, study design, or interpretation of results.
Funding: None.
Footnote
Conflicts of Interest: All authors have completed the ICMJE uniform disclosure form (available at http://dx.doi.org/10.21037/qims-20-630). MKK received unrelated research grants from Siemens Healthineers, and Riverain technologies LLC. BPL receives royalties as an academic textbook author and associate editor from Reed Elsevier, Inc. SRD provides independent image analysis for hospital contracted clinical research trials programs for Merck, Pfizer, Bristol Mayer Squibb, Novartis, Roche, Polaris, Cascadian, Abbvie, Gradalis, Clinical Bay, Zai laboratories. Received honorarium from Siemens Healthineers for unrelated work and received research funding from Lunit Technology, S. Korea for unrelated work. JAOS reports other from Royalties from Elsevier, outside the submitted work. The other authors have no conflicts of interest to declare.
Ethical Statement: This retrospective study was performed on LDCT images from the National Lung Cancer Screening Trial (NLST). The National Cancer Institute (NCI) approved the NLST data transfer for this research study. Since we used publicly available non-institutional data, the study was exempt from the institutional review board (IRB) approval.
Open Access Statement: This is an Open Access article distributed in accordance with the Creative Commons Attribution-NonCommercial-NoDerivs 4.0 International License (CC BY-NC-ND 4.0), which permits the non-commercial replication and distribution of the article with the strict proviso that no changes or edits are made and the original work is properly cited (including links to both the formal publication through the relevant DOI and the license). See: https://creativecommons.org/licenses/by-nc-nd/4.0/.
References
- National Lung Screening Trial Research Team. Aberle DR, Adams AM, Berg CD, Black WC, Clapp JD, Fagerstrom RM, Gareen IF, Gatsonis C, Marcus PM, Sicks JD. Reduced lung-cancer mortality with low-dose computed tomographic screening. N Engl J Med 2011;365:395-409. [Crossref]
- Black WC, Gareen IF, Soneji SS, Sicks JD, Keeler EB, Aberle DR, Naeim A, Church TR, Silvestri GA, Gorelick J, Gatsonis C. Cost-Effectiveness of CT Screening in the National Lung Screening Trial. N Engl J Med 2014;371:1793-802. [Crossref] [PubMed]
- Jemal A, Fedewa SA. Lung Cancer Screening With Low-Dose Computed Tomography in the United States-2010 to 2015. JAMA Oncol 2017;3:1278-81. [Crossref] [PubMed]
- Becker N, Motsch E, Trotter A, Heussel CP, Dienemann H, Schnabel PA, Kauczor HU, Maldonado SG, Miller AB, Kaaks R, Delorme S. Lung cancer mortality reduction by LDCT screening-Results from the randomized German LUSI trial. Int J Cancer 2020;146:1503-13. [Crossref] [PubMed]
- Digumarthy SR, De Man R, Canellas R, Otrakji A, Wang G, Kalra MK. Multifactorial Analysis of Mortality in Screening Detected Lung Cancer. J Oncol 2018;2018:1296246. [Crossref] [PubMed]
- Fintelmann FJ, Bernheim A, Digumarthy SR, Lennes IT, Kalra MK, Gilman MD, Sharma A, Flores EJ, Muse VV, Shepard JA. The 10 Pillars of Lung Cancer Screening: Rationale and Logistics of a Lung Cancer Screening Program. Radiographics 2015;35:1893-908. [Crossref] [PubMed]
- Messay T, Hardie RC, Rogers SK. A new computationally efficient CAD system for pulmonary nodule detection in CT imagery. Med Image Anal 2010;14:390-406. [Crossref] [PubMed]
- Singh R, Kalra MK, Nitiwarangkul C, Patti JA, Homayounieh F, Padole A, Rao P, Putha P, Muse VV, Sharma A, Digumarthy SR. Deep learning in chest radiography: Detection of findings and presence of change. PLoS One 2018;13:e0204155. [Crossref] [PubMed]
- Rajpurkar P, Irvin J, Ball RL, Zhu K, Yang B, Mehta H, Duan T, Ding D, Bagul A, Langlotz CP, Patel BN, Yeom KW, Shpanskaya K, Blankenberg FG, Seekins J, Amrhein TJ, Mong DA, Halabi SS, Zucker EJ, Ng AY, Lungren MP. Deep learning for chest radiograph diagnosis: A retrospective comparison of the CheXNeXt algorithm to practicing radiologists. PLoS Med 2018;15:e1002686. [Crossref] [PubMed]
- Singh R, Homayounieh F, Vining R, Digumarthy SR, Kalra MK. The Value in Artificial Intelligence. Medical Radiology. Springer Berlin Heidelberg; 2019.
- Digumarthy SR, Gullo RL, Levesque MH, Sayegh K, Rao S, Raymond SB, Otrakji A, Kalra MK. Cause determination of missed lung nodules and impact of reader training and education: Simulation study with nodule insertion software. J Cancer Res Ther 2020;16:780-7. [Crossref] [PubMed]
- Wang X, Mao K, Wang L, Yang P, Lu D, He P. An Appraisal of Lung Nodules Automatic Classification Algorithms for CT Images. Sensors (Basel) 2019;19:194. [Crossref] [PubMed]
- Digumarthy SR, Padole AM, Gullo RL, Sequist LV, Kalra MK. Can CT radiomic analysis in NSCLC predict histology and EGFR mutation status? Medicine (Baltimore) 2019;98:e13963. [Crossref] [PubMed]
- Godoy MC, Naidich DP. Overview and strategic management of subsolid pulmonary nodules. J Thorac Imaging 2012;27:240-8. [Crossref] [PubMed]
- Lo SB, Freedman MT, Gillis LB, White CS, Mun SK. JOURNAL CLUB: Computer-Aided Detection of Lung Nodules on CT with a Computerized Pulmonary Vessel Suppressed Function. AJR Am J Roentgenol 2018;210:480-8. [Crossref] [PubMed]
- Digumarthy SR, Padole AM, Rastogi S, Price M, Mooradian MJ, Sequist LV, Kalra MK. Predicting malignant potential of subsolid nodules: can radiomics preempt longitudinal follow up CT? Cancer Imaging 2019;19:36. [Crossref] [PubMed]
- Yuan R, Vos PM, Cooperberg PL. Computer-aided detection in screening CT for pulmonary nodules. AJR Am J Roentgenol 2006;186:1280-7. [Crossref] [PubMed]
- Jacobs C, van Rikxoort EM, Murphy K, Prokop M, Schaefer-Prokop CM, van Ginneken B. Computer-aided detection of pulmonary nodules: a comparative study using the public LIDC/IDRI database. Eur Radiol 2016;26:2139-47. [Crossref] [PubMed]
- Bankier AA, MacMahon H, Goo JM, Rubin GD, Schaefer-Prokop CM, Naidich DP. Recommendations for Measuring Pulmonary Nodules at CT: A Statement from the Fleischner Society. Radiology 2017;285:584-600. [Crossref] [PubMed]
- Forsberg D, Rosipko B, Sunshine JL. Radiologists' Variation of Time to Read Across Different Procedure Types. J Digit Imaging 2017;30:86-94. [Crossref] [PubMed]
- Bogoni L, Ko JP, Alpert J, Anand V, Fantauzzi J, Florin CH, Koo CW, Mason D, Rom W, Shiau M, Salganicoff M, Naidich DP. Impact of a computer-aided detection (CAD) system integrated into a picture archiving and communication system (PACS) on reader sensitivity and efficiency for the detection of lung nodules in thoracic CT exams. J Digit Imaging 2012;25:771-81. [Crossref] [PubMed]
- Valente IR, Cortez PC, Neto EC, Soares JM, de Albuquerque VH, Tavares JM. Automatic 3D pulmonary nodule detection in CT images: A survey. Comput Methods Programs Biomed 2016;124:91-107. [Crossref] [PubMed]
- Brown MS, Lo P, Goldin JG, Barnoy E, Kim GHJ, McNitt-Gray MF, Aberle DR. Toward clinically usable CAD for lung cancer screening with computed tomography. Eur Radiol 2014;24:2719-28. [Crossref] [PubMed]
- Benzakoun J, Bommart S, Coste J, Chassagnon G, Lederlin M, Boussouar S, Revel MP. Computer-aided diagnosis (CAD) of subsolid nodules: Evaluation of a commercial CAD system. Eur J Radiol 2016;85:1728-34. [Crossref] [PubMed]
- Yanagawa M, Honda O, Yoshida S, Ono Y, Inoue A, Daimon T, Sumikawa H, Mihara N, Johkoh T, Tomiyama N, Nakamura H. Commercially Available Computer-Aided Detection System for Pulmonary Nodules on Thin-Section Images Using 64 Detectors-Row CT. Acad Radiol 2009;16:924-33. [Crossref] [PubMed]
- Aissa J, Schaarschmidt BM, Below J, Bethge OT, Böven J, Sawicki LM, Hoff N-P, Kröpil P, Antoch G, Boos J. Performance and clinical impact of machine learning based lung nodule detection using vessel suppression in melanoma patients. Clin Imaging 2018;52:328-33. [Crossref] [PubMed]
- Al Mohammad B, Brennan PC, Mello-Thoms C. A review of lung cancer screening and the role of computer-aided detection. Clin Radiol 2017;72:433-42. [Crossref] [PubMed]
- Li Q, Li F, Doi K. Computerized Detection of Lung Nodules in Thin-Section CT Images by Use of Selective Enhancement Filters and an Automated Rule-Based Classifier. Acad Radiol 2008;15:165-75. [Crossref] [PubMed]