The potential role of leukoaraiosis in remodeling the brain network to buffer cognitive decline: a Leukoaraiosis And Disability study from Alzheimer’s Disease Neuroimaging Initiative
Introduction
White matter, or substantia alba, is composed primarily of myelinated axons and support cells named oligodendroglia (1). Causes of changes in white matter include vascular or other risk factors, as well as genetic variation. The mechanisms underlying the development of white matter changes (WMCs) are extremely complex. Histopathologic correlates have been reported in the enlarged perivascular (Virchow-Robin) spaces, and myelin degeneration and axons with increased intracellular and extracellular water content, gliosis, and even infarction, have also been observed (2-11). In aging individuals with Alzheimer’s disease (AD), stroke, and cerebral small vessel disease, white matter hyperintensities (WMHs) are often found on proton density (PD) and T2-weighted magnetic resonance imaging (MRI) scans, including fluid-attenuated inversion recovery (FLAIR) MRI scans. WMHs on MRI are also referred to as leukoaraiosis (LA) (12-14); this term was first used by Hachinski, who combined two Greek roots, “leuko” and “raiosis”, to reflect the white matter and radiological phenomena found, respectively (3). Usually, MRI is preferred for the detection of age-related WMCs (ARWMCs) due to it having higher sensitivity than computed tomography (CT). Wahlund et al. studied ARWMCs in a large cohort of patients who underwent examination with both MRI and CT; a rating scale was developed and evaluated, with MRI found to have a slightly better interrater reliability in the detection of small lesions (15). A large number of current studies are focused on WMHs (16); however, the role of LA in human cognitive function is not fully understood, and the clinical significance of LA has not been fully elucidated. As a phenomenon observed in the brains of AD patients or aging individuals, the structure, function, and behavioural characteristics of LA need to be explored. The in vivo human brain is a complex network that is neither uniformly random nor ordered and shares a number of common features with the networks of other biological and physical systems. For this reason, to study the human brain in vivo, a complex network method is needed.
Recent advances in quantitative MRI (qMRI) and modern in vivo brain mapping techniques, such as diffusion MRI, voxel-based morphometry (VBM), and the network-based statistic (NBS), have facilitated a complex network analysis, including graph theory, to describe the important properties of complex systems. These advances have also enabled the parameters of neuroanatomical connectivity to be defined through quantifying the topologies of their respective network representations, which allows us to characterize large brain networks with a small number of neurobiologically meaningful and easily computable measures (17-19). The network analyses can be represented in multiple ways, and the measures of individual network elements (such as nodes or links) typically quantify connectivity profiles associated with these elements (17). Therefore, the terms “nodes”, “edges”, and “rich club” have been used to represent brain cortical and subcortical structures, white matter connections, and key hubs with a high density of interconnections, respectively. Comparisons of structural or functional network topologies between subject populations have revealed presumed connectivity abnormalities in disorders related to human cognitive function (17). These developments led us to quantify and compare white matter connectivity properties and gray matter (GM) volume of brain regions of interest (ROIs) between AD patients and elderly individuals with normal cognitive (NC) function by combining structural and diffusion MRI to model the brain as a network and evaluate its topological properties and VBM. These measurements can help us to evaluate the compensatory mechanism hypotheses of LA in cognitive function and verify if LA, the phenomena “of loose texture” (13), in NC individuals or AD patients could modulate global and local structural brain networks with GM against serious hippocampal and amygdala atrophy.
In this study, we aimed to investigate changes in the patterns of rich-club organization in NC individuals and AD patients with the same or different LA ratings, and to characterize the relationship between the GM volume and LA rating by using advanced diffusion MRI analysis techniques and complex network analysis combined with VBM. These changes and correlations in rich-club organization might serve as a biomarker for early cognitive dysfunction, as well as a guide for clinical trials and interventions.
Methods
Study design and participants
This was a retrospective case-control observational MRI study. All AD patients and age- and sex-matched NC individuals were selected from the Alzheimer’s Disease Neuroimaging Initiative (ADNI) database, where participants were recruited from over 50 institutions across US and Canada (20). All participants had provided informed written consent before recruitment and filled out questionnaires approved by the respective Institutional Review Board (IRB).
Image acquisition and LA classification
The ADNI image acquisition protocol was adopted for all MRI scans used in this study. The 3D T1-weighted images were acquired with the following parameters: Acquisition plane = sagittal; Acquisition type =3D; Coil = PA; Field strength =3.0 tesla; Flip angle =9.0 degree; Manufacturer = Siemens; Matrix X =240.0 pixels; Matrix Y =256.0 pixels; Matrix Z =176.0 pixels; Mfg model = Prisma-Fit; Pixel spacing X =1.1 mm; Pixel spacing Y =1.1 mm; Pulse sequence = GR/IR; Slice thickness =1.2 mm; TE =3.0 ms; TI =900.0 ms; TR =2,300.0 ms; Weighting = T1.
Sagittal 3D FLAIR images were acquired with the following parameters: Acquisition plane = sagittal; Acquisition type =3D; Coil = PA; Field strength =3.0 tesla; Flip angle =120.0 degree; Manufacturer = Siemens; Matrix X =256.0 pixels; Matrix Y =256.0 pixels; Matrix Z =160.0 pixels; Mfg Model = Prisma-Fit; Pixel spacing X =1.0 mm; Pixel spacing Y =1.0 mm; Pulse sequence = SE/IR; Slice thickness =1.2 mm; TE =441.0 ms; TI =1,650.0 ms; TR =4,800.0 ms; Weighting = T2.
Diffusion-weighted images from axial diffusion tensor imaging (DTI) scans were acquired with the following parameters: 59 slices of 2.7-mm thickness, with no gap between slices; repetition time/echo time =9 s/60 ms; 256×256 matrix with a field of view of 35 cm; flip angle 90. T1-weighted (T1W) images were obtained from sagittal inversion recovery-prepared spoiled gradient-echo scan. Forty-one diffusion-weighted images (b =1,000 s/mm2) with noncollinear directions and one with a volume without diffusion weighting (b =0 s/mm2) were collected.
The clinical and demographic characteristics of the participants were collected. The MRI images were evaluated by one radiology fellow who had 3 years of experience in neuroimaging diagnosis, and 2 chief radiologists who had >20 years of experience in neuroimaging diagnosis. To reduce interobserver variability, the two chief radiologists redid the evaluations of MRI images based on raw data downloaded by the radiology fellow. The participants with poor MR image quality were excluded. The LA classification were respectively performed according to Wahlund’s WMC rating scale (15) by the three evaluators. Subsequently, the AD and NC cohorts were each divided into three groups: LA1, LA2, and LA3, Namely, there were AD LA1, AD LA2 and AD LA3 in AD group; NC LA1, NC LA2, and NC LA3 in NC group.
Image preprocessing, tractography, and VBM
All diffusion-weighted imaging data involved in this study were analyzed with the software library of FMRIB (FSL) toolbox. The ‘Eddy’ script was used to correct motion and eddy current distortions. The FSL brain extraction tool (BET) was utilized to create a brain mask of the non-diffusion-weighted images. The ‘ditfit’ script was used to calculate diffusion tensors and created fractional anisotropy (FA) images, which were later co-registered linearly in native space to their corresponding T1-weighted images. Structural images were non-linearly registered to the standard Montreal Neurological Institute (MNI) space (the ICBM152 template). By inversely transforming the above two steps, the automated anatomical labeling (AAL) atlas, with its 90 GM ROIs in the standard space, inversely warped images back to individual native space.
Deterministic tractography was performed on whole brain MR images in subject-specific native space. Fiber assignment by continuous tracking (FACT) algorithm in Diffusion Toolkit (http://www.trackvis.org/dtk/) was used to reconstruct a streamline that started from each seed (8 seeds per voxel) and terminated when streamline reached the following conditions: reached voxel with an FA value lower than 0.2, exceeded the brain mask, or the trajectory of the streamline made a turn sharper than 45°.
Statistical Parametric Mapping package [SPM12, Wellcome Department of Imaging Neuroscience, London, UK (www.fil.ion.ucl.ac.uk/spm)], and the Computational Anatomy Toolbox 12 [CAT12 (http://dbm.neuro.uni-jena.de/cat.html)] were used in voxel-based morphometric analysis. Bias-field inhomogeneity correction, white and GM segmentation, and normalization to diffeomorphic anatomical registration through exponentiated lie algebra (DARTEL) template in MNI space (voxel size: 1.5 mm × 1.5 mm × 1.5 mm) were performed on the T1-weighted MR images in the computational anatomy toolbox (CAT12) on default setting. Next, the preprocessed GM data were smoothed with an 8-mm full-width-half-maximum isotropic Gaussian kernel.
Image construction, connectivity matrix, and metrics
A weighted and undirected 90×90 connectivity matrix was created for each subject by mapping tractography streamlines through cortical and subcortical structures. Changes in the structural volumes of ROIs were not corrected. Weighted and undirected edges were analyzed by obtaining streamline counts defined as the total number of streamline connections between two nodes, and FA edge weight defined as the FA mean between two nodes (21). FA edge weights were adopted in graph theory and sub-network analysis to account for FA reductions (22). Effects of high connection volume nodes were examined through utilizing streamline count edge weights in rich-club connection analysis.
Graph theory measures were applied to weighted undirected edge strengths for all analyses through the Brain Connectivity Toolbox (17). Regional and global measures were used to explore integration and segregation properties (17,22-24). The analysis metrics used in this study were common weighted graph properties including weighted degree, clustering coefficient, characteristic path length, and global and local efficiency (22). By referencing a previous DTI analysis with similar cohort (25,26), 9 bilateral nodes were selected (listed in Table 1) as endpoints of callosal splenium, cingulum, and prefrontal white matter connections (25). Due to the variability of structural connectivity among participants, our study focused on the backbone network mask, which was consistent among the participants.
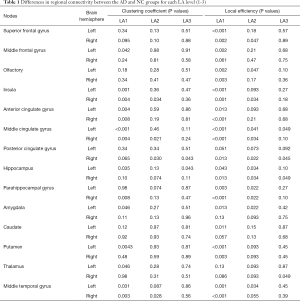
Full table
NBS
The NBS toolbox allowed for mass univariate testing in identifying the cluster lever experimental effect. We performed 5,000 permutation tests at a significance threshold of P<0.05. Investigation across three suprathreshold values (27,28) was adopted. The suprathreshold of the connected components was compared between groups (27).
Rich-club organization and membership
Nodes clubbing with dense connections, also known as a rich club (29,30), among cortical–subcortical regions were investigated for structural membership and between-group variations. Rich-club regions were defined as the top 10 (11%) brain regions with the highest degree averaged across all groups (31-33). The top 10 highest degree nodes were chosen to validate the threshold selection (30,34), which confirmed that rich-club members were not dependent on one hub definition alone. Rich-club membership was investigated following examination of rich-club connectivity effects. This rich-club selection ensured equal numbers of nodes were used to construct the rich clubs, regardless of the average degree across subjects.
The rich-club coefficient Ф(k) (29,35), defined as the density of connections (average connection weight) between rich-club nodes (36), reflects the level of between-node interconnectivity. A normalized rich-club coefficient Фnorm(k) can be used to compare random networks with preserved degree distribution in order to eliminate effects attributable only to chance (30). We selected rich-club structures by the group-averaged cortico-subcortical network for the statistically significant Фnorm(k) and identified nodes connected by this pathway. Rich-club members defined at the statistically significant network between AD and NC (k=56) displayed approximately 10–12 highly connected nodes. We applied a threshold of 60% to identify the findings that were consistent. In a previous study, pathways present in >50% of participants were analyzed (30). Rich-club members with Фwnorm >1 across range k at a group threshold of 60% and 70% of participants would result in the most consistent rich-club structures across individual networks. Based on the categorization of the nodes of the network into rich-club and peripheral regions, the edges of the network were classified as follows: rich-club connections, linking two rich-club nodes; feeder connections, linking one rich-club node to one peripheral node; and local connections, linking two peripheral nodes (32,37). The ‘connectivity strength’, a summary measure of connectivity, was calculated as the sum of the edge weights for each connection type.
Statistical analysis
The GM volume of brain ROIs in the AD and NC groups with 3 degrees of LA were examined by t-tests. These ROIs included the superior frontal, middle frontal, olfactory, insula, anterior cingulate, middle cingulate, posterior cingulate, hippocampus, parahippocampal, amygdala, caudate, putamen, thalamus, and middle temporal gyri. To compare the aberrant connections in the AD and NC groups, we explored the significant non-zero connections within each group by one-tailed Wilcoxon test (P<0.05) with the false discovery rate (FDR) corrected to the P values to rectify for multiple comparisons across all edges (FDR <0.05). Then, the non-zero connections within LA1, LA2, and LA3 groups within the AD and NC cohorts were selected and combined into a connection mask, respectively. The t-test was used to detect significant differences between each AD and NC group with P value <0.05 and FDR <0.05. For each AD group, a chi-squared (χ2) test was performed to assess the differences between the expected proportions of the edges (i.e., the known number of average rich-club, feeder, and local connections) and the observed proportions compared to its respective NC group (33).
Statistical analysis of global and regional metrics (degree, clustering coefficient, characteristic path length, global efficiency, and local efficiency) applied multivariate analysis of covariance (MANCOVA) tests, co-varied for age and gender, using IBM SPSS statistics software version 22 (SPSS Inc., IBM Company, Chicago, IL, USA). The calculated global values from nodal measures were averaged across all 90 nodes to generate a global average for each measure. We corrected for global connectivity to assess whether findings were indicative of reduced connectivity globally, or potentially affected by topological organization. Global analyses and regional comparisons underwent FDR correction for multiple comparisons (38).
Permutation testing was used to assess between-group effects in rich-club connectivity. We performed 9,999 Monte Carlo resamples using R software. Multiple comparisons corrected for 28 possible values of k density were implemented using the FDR method (38).
Results
Demographic and clinical information for all participants are reported in Table 2. There were 76 AD patients and 82 NC individuals enrolled in this study. The study participants had an average age of 76.3±10.0 years (range, 55–90 years for the AD group, and 63–89 years for the NC group). Females accounted for 40.5% of the study participants. We combined the assessments of the participants’ 3T MR axial DTI, T2, fluid attenuated inversion recovery (FLAIR), and T1-weighted images; It was found that the same classification of LA was visible in the brains of both the AD patients and NC individuals (Figure 1).
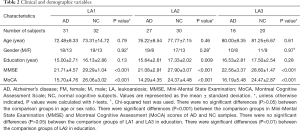
Full table
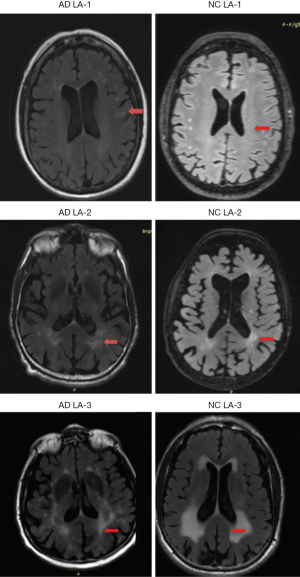
According to Wahlund’s ARWMC rating scale (15), the AD patients were divided into the LA1 (n=31), LA2 (n=27), and LA3 (n=18) groups, and the NC individuals were divided into the LA1 (n=32), LA2 (n=30), and LA3 (n=20) groups (Table 2). We found that Wahlund’s ARWMC rating score of AD patients was weakly correlated with individual age (correlation coefficient r≈0.349), and was not correlated to Mini-Mental State Examination (MMSE) and Montreal Cognitive Assessment Scale (MoCA) cognitive scores (correlation coefficients were r≈0.066 and r≈0.014, respectively).
Subsequent analyses focused on DTI and T1-weighted MR images because they had the advantage of analyzing white matter connectivity and GM atrophy in the whole brain. To date, macroscopically, anatomical connections (structural connectivity) were often estimated by fiber tractography from DTI (30,39). The structural connectivity measured by MRI usually reflects large-range fiber bundles inferred from DTI (40); one could derive a structural brain network in terms of fiber bundles according to the regions they interconnect (41,42).
Brain structural connectivity characteristics in different LA conditions
We explored the brain structural connections among rich-club and peripheral regions in the condition of LA; if AD patients with the same LA rating as NC individuals displayed structural connections different from that of the NC individuals; and if there were different structural connections among the different LA stages in both NC individuals and AD patients.
Analysis of global properties revealed statistically significant group differences whereby the AD LA1 and 2 groups displayed increased characteristic path length, and reduced global efficiency and clustering coefficient compared with the matched NC group when the connections were weighted by FA (Figure 2A,B,C). Some of the regions connected by frontolimbic pathways revealed significantly reduced connectivity that survived multiple comparison correction in the LA1 and 2 groups. Particularly in the AD LA1 group, reduced clustering and local efficiency predominantly incorporated the bilateral insula, anterior and middle cingulate nodes, as well as the middle temporal nodes. Meanwhile, significantly reduced local efficiency was observed in the superior and middle frontal, olfactory, hippocampus, parahippocampal, caudate, posterior cingulate, putamen, and left amygdala and right thalamus nodes in the AD LA1 group. In the AD LA2 group, significantly reduced local efficiency was observed in the right superior frontal, left olfactory, right insula, bilateral middle cingulate, right posterior cingulate, bilateral hippocampal and parahippocampal, as well as the left amygdala and middle temporal nodes (Table 1).
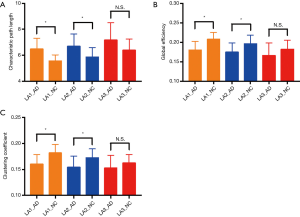
The two different local graph measures in FA connections are shown. Multivariate analyses of covariance (MANCOVA) were carried out between groups. Bilateral nodes (n=14) were selected a priori from a previous data-driven DTI analysis (26,27) for analyses of local graph theory measures.
All P values were obtained from the t-tests between the AD and NC groups after FDR correction.
Our data showed that all AD and NC brain structural networks constructed from DTI had rich-club hubs in the precuneus, superior frontal and superior parietal cortex, subcortical hippocampus, putamen, and thalamus (Figure 3). In the AD group, rich-club membership at the group threshold of 60% indicated that the right angular gyrus was not included in the rich-club in most patients (Figure 3A,C,D); however, most AD rich-club structures incorporated the right superior and middle temporal gyri (Figure 3A,B,C). In the AD LA1 group, rich-club membership at the group threshold of 60% indicated that the superior orbital and dorsolateral superior frontal gyri in the left hemisphere were not included in the rich-club in most patients (Figure 3B). In the AD LA2 group, rich-club structures incorporated the right superior and middle temporal, as well as the right superior orbital gyri (Figure 3C). In the AD LA3 group, rich-club structures mainly incorporated the left dorsolateral superior frontal, and right precentral and precuneus gyri (Figure 3D).
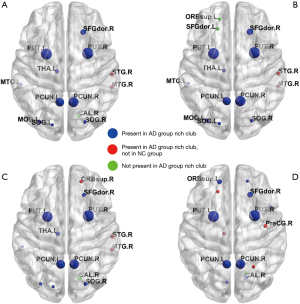
When connections were weighted by FA, the AD LA1 and LA2 groups displayed significant increases in characteristic path length, and reduction in global efficiency and clustering coefficient compared to the NC LA1 and LA2 groups, respectively (P<0.05). Meanwhile, there was a significant difference (P<0.05) in characteristic path length, global efficiency, and clustering coefficient between the AD LA1 vs. NC LA2, and AD LA2 vs. NC LA1 groups, respectively. In the AD LA3 group increased characteristic path length, reduced global efficiency and clustering coefficient showed a significant difference (P<0.05) compared to the NC LA1 and NC LA2 groups, respectively. Moreover, increased characteristic path length, and reduced global efficiency and clustering coefficient in the NC LA3 individuals showed significant difference (P<0.05) compared to the NC LA1 individuals. However, these increased characteristic path lengths, and reduced global efficiencies and clustering coefficients did not show the significant difference (P>0.05) between the groups of AD LA3 vs. NC LA3, AD LA3 vs. AD LA1, and AD LA3 vs. AD LA2, respectively (Figure 2A,B,C). Furthermore, there was no significant difference (P>0.05) in characteristic path length, reduced global efficiency, and clustering coefficient between the NC LA3 and AD LA1 groups, or between the NC LA3 and AD LA2 groups, meaning that that LA3 might be a critical point, at which the white matter connectivity in NC LA3 individuals is almost similar to that in all AD LA patients (P>0.05 in NC LA3 vs. AD LA3, NC LA3 vs. AD LA2, NC LA3 vs. AD LA1). Essentially, the NC LA3 group may be considered “abnormal”, and the connectivity differences compared to the AD LA groups may become obscured. The white matter connectivity abnormalities in the NC LA3 group may resemble pathological changes in the AD LA groups, although these are representative of a normal cognitive state. The individuals in NC LA3 showed normal cognitive function probably due to compensatory mechanisms, cognitive reserve, as well as absence of the pathophysiological factors specific for AD.
Further, connectivity strength in rich-club, local, and feeder connections was examined in AD LA patients and NC LA individuals (Figure 4). Connectivity strength was examined by distinguishing different types of connections including: local connections between peripheral nodes; feeder connections between peripheral and rich-club nodes; and rich club connections between rich-club nodes only. The results in connectivity strength showed that rich-club, feeder, and local connectivity strength was significantly decreased in the AD LA1 and AD LA2 groups compared to that in the NC LA1 and NC LA2 groups (P<0.05, respectively). In the LA3 stage, the decreased connectivity in AD patients was not significantly different (P>0.05) compared to that in the NC individuals (Figure 4A,B,C). Basically, AD patients from the LA1–LA3 groups and NC LA3 individuals suffered disturbances in the connections between rich-club regions, local connections between peripheral nodes, as well as feeder connections between peripheral and rich-club regions. In NC individuals, the rich-club, feeder, and local connectivity strength in the LA3 group was significantly decreased compared to that in the LA1 group (P<0.05, respectively), similar to the ones in all AD LA patients (P>0.05 in NC LA3 vs. AD LA3, NC LA3 vs. AD LA2, NC LA3 vs. AD LA1), meaning that the WMH may reach its limit to buffer cognitive decline. Meanwhile, these results suggest that these disturbances appear to be more likely to occur in the peripheral brain regions where connectivity strength was <0.05 (Figure 4C).
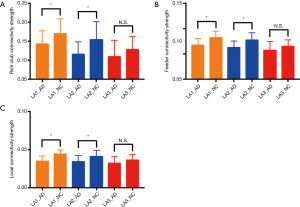
LA modulation in cognitive structural connections
We tested how LA modulated the structural connections among rich-club and peripheral regions against hippocampal and amygdala atrophy in AD patients and NC individuals (Figure 5). We analyzed the rich-club coefficient (Ø) (Figure 5A), and normalized rich-club coefficient (Ønorm) (Figure 5B) as a function of node degree (k), respectively. Our findings did not change when corrected for global connectivity, defined by the global density metric. Preserved measures of degree and density indicated that significant differences in topology might be the primary feature of AD. Rich-club organization was evident in all groups, with Ønorm increasing as a function of node degree (k) >1. A k-value of 16 was the highest value for which all subjects had data, and the k-value functions exhibited no sharp discontinuities, as the edges that interconnected brain regions dropped off more readily in patients (especially AD patients) than in individuals with NC. We only reported the k-levels of patient groups from which the rich-club effects were detected across most participants (90%) (31). Especially at low-degree k-levels such as k=3–13 in AD LA1 patients, k=4–11 in AD LA2 patients, and k=9–11 in AD LA3 patients (Bonferroni-corrected for groups and all degree levels, Figure 5A), the rich-club coefficient (Ø) was significantly lower (P<0.01) in all AD LA patients compared to their matched NC individuals (AD LA1 vs. NC LA1, AD LA2 vs. NC LA2, and AD LA3 vs. NC LA3). At low-degree k-levels such as: k=5–15 in AD LA1 patients, k=4–8 in AD LA2 patients (Bonferroni-corrected for groups and all degree levels, Figure 5B), the Ønorm was significantly higher (P<0.01) in the LA1 and LA2 AD groups relative to their matched NC counterparts, respectively. Ønorm in the LA3 NC individuals almost matched the high of the AD patients at k=1–16. Similar to other AD study findings (43), these data might suggest that the low k-value regions were more affected than the high-degree regions in patients, as indicated by the tendency for higher Ønorm values with mounting disease progression. Consistent with Daianu’s research, the Ønorm increased in the AD LA1–LA3 groups and in the LA3 NC group, mostly in the low-degree k-value regime (k<15) (31).
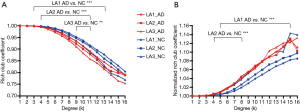
Rich-club distribution in different LA and cognitive conditions
An additional analysis was performed to assess the distribution among rich-club, feeder, or local edges between each LA level in the AD and NC cohorts (Figure 6). The connectivity was affected in the AD LA1 group, with 66 connections (1/41 rich-clubs, 21/553 feeders, and 44/1,399 local edges. Figure 6A), in the AD LA2 group, with 45 connections (1/41 rich-clubs, 14/563 feeders, and 30/1527 local edges. Figure 6B), and in the AD LA3 group, with 20 connections (1/36 rich-clubs, 7/453 feeders, and 12/1,142 local edges. Figure 6C), relative to the NC group, which showed a lower connection trend in LA3. No significant differences were found in the proportions of significantly altered rich-club, local, and feeder connections between any of the AD and NC groups (χ2 LA1 P=0.746, LA2 P=0.744, LA3 P=0.511. Figure 6D), indicating that the differences in the abnormal edges in the NC and AD groups were proportionally distributed between rich-club and feeder, rich-club and local edges, and between feeder and local edges. These findings also indicated that the connectivity effects were affected throughout the brain networks in the all AD LA groups and NC LA3 groups. This finding of the connectivity effects in NC LA3 groups similar to all AD LA groups offers a new evidence to support the involvement of LA in the neural basis of the progressive risk for cognitive decline.
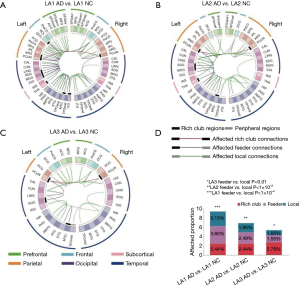
Nodes with abnormal connectivity in different LA stages
Some nodes exhibited abnormal connectivity in AD and NC groups. To test if the disturbance in these nodes was an important source of abnormal communication, we explored the effects of different LA stages on whole-brain connectivity within this subset of nodes. We identified the nodes with higher numbers of abnormal connections (range >3 aberrant connections). The nodes with the most aberrant connections that showed significant group differences (P<0.05) in nodal efficiency between the AD and NC groups were further analyzed and plotted (Figure 7).
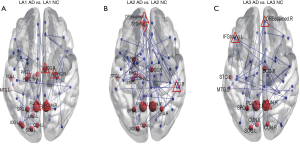
The nodes with the highest numbers of aberrant connections in AD participants relative to NC were DCG.R, PHG.R, PCUN.R, and PCUN.L in LA1 (Figure 7A); ORBsupmed.L, SFGmed.L, and ITG.R in LA2 (Figure 7B); and IFGtrang.L, ORBsupmed.R, and PCUN.R in LA3 (Figure 7C), respectively.
From LA1 to LA2, the results indicated that rich-club regions tended to remain persistent in AD patients and were disrupted gradually as the disease progressed compared to those in the NC individuals. Especially in the LA2 groups, AD patients were more likely to suffer disruptions in the peripheral regions relative to their NC counterparts. The results from the LA3 groups showed that aberrant nodes and connections were reduced in AD patients relative to NC individuals, with the nodes with the highest number of aberrant connections being IFGtrang.L, ORBsupmed.R, and PCUN.R, which are related to many high-level cognitive functions. These results suggested that the compensatory changes of LA reached their limits as a result of the brain’s plasticity, to buffer cognitive decline.
Collective network dysconnectivity in different LA stages
We identified collective network dysconnectivity differences with suprathreshold connections (t=3, P<0.05) consisting of frontolimbic connections in AD. Higher suprathreshold connections (t=4, P<0.05; and t=5, P<0.05) revealed structural dysconnectivity among frontolimbic connections in AD patients compared to the NC individuals. The AD group displayed significantly weaker subnetwork connectivity, with a single disconnected sub-network identified for each threshold (3-5). The NBS provides two outputs, (I) the suprathreshold set of connections involved in the graph component found to show a significant effect; and (II) a corresponding P value for each such network (27).
In the AD LA1 group, the inferior frontal triangular, parahippocampal, amygdala, lenticular pallidum nucleus, thalamus, middle temporal, and superior frontal medial gyri in the left hemisphere, as well as the anterior cingulate, paracingulate and posterior cingulate gyri in the right hemisphere, displayed significantly weaker suprathreshold connectivity compared to those of the NC individuals; the same results were observed in the bilateral median cingulate and paracingulate gyri, and precuneus (Figure 8A). In the AD LA2 group, the parahippocampal and superior frontal medial gyri, amygdala in the left hemisphere, and the anterior cingulate and paracingulate, posterior cingulate gyri in the right hemisphere, the bilateral median cingulate and paracingulate gyri, and precuneus, displayed significantly weaker suprathreshold connectivity compared to those of the NC individuals (Figure 8B). In the AD LA3 group, the parahippocampal and superior frontal medial gyri, amygdala, in the left hemisphere, as well as the anterior cingulate, paracingulate, and posterior cingulate gyri in right hemisphere, displayed significantly weaker suprathreshold connectivity compared to those of the NC individuals, as did the bilateral median cingulate and paracingulate gyri and precuneus (Figure 8C).
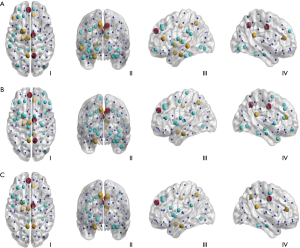
Relationship between network metrics and cognitive function in LA
We tested the relationship between network metrics and cognitive scores (Figure 9) as well as their relationship in each LA stage (Figure S1) for the AD and NC groups. Our data showed that: the rich-club connectivity strength was highly correlated to the clustering coefficient, feeder connectivity strength, global efficiency, and local connectivity strength, respectively; the clustering coefficient was highly correlated to the feeder connectivity strength, global efficiency, and local connectivity strength, respectively; the feeder connectivity strength was highly correlated to global efficiency, and local connectivity strength, respectively; and global efficiency was highly correlated to local connectivity strength. However, the characteristic path length was negatively low correlated with the MMSE (r=−0.36) and MoCA (r=−0.29) scores, respectively, after Bonferroni corrections, the rich-club connectivity strength, global efficiency, clustering coefficient, and connectivity strength (feeder and local) in group together (Figure 9) were low positively correlated (r between 0.3 to 0.5) with MMSE and MoCA scores, respectivley, and negligible correlated (r between 0.0 to 0.3) with MMSE and MoCA scores in each LA staged (Figure S1), respectively. Similar to Seo’s findings (44), WMH independently affected cognitive impairment. But, the correlation between network metrics and cognitive scores of the AD patients and NC individuals in each LA stage was not strong.
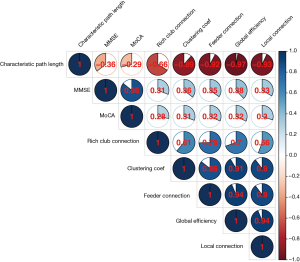
GM changes in different LA stages
We analyzed GM changes on selected brain ROIs of each AD patient and their matched NC counterparts (Table 3). Extensive and significant voxel-wise GM volume differences in AD patients from LA1–LA3 compared to the matched NC individuals (P<0.05) (Table 3) were illustrated by VBM. The frontal limbic pathway including the frontal, parahippocampal, and temporal gyri, as well as cingulate cortex, amygdala is a core neural pathway representing the pathophysiological feature of cognition. In particular, both the hippocampus and amygdala are critically involved in memory processes. In this study, AD patients with LA showed decreased GM density in the frontal and temporal gyri, cingulate cortex. GM atrophy was correlated with the higher white matter lesions (WML) in AD patients, which was consistent with research into GM changes in WMLs and AD (45-47). In the LA3 stage, only the left parahippocampal gyrus and amygdala volume showed significant differences (P<0.05) in AD patients compared to their matched NC counterparts, and this relationship was further analyzed. The maximum t-score (Tmax) at the left parahippocampal gyrus and amygdala was found to be highly nonlinearly negatively correlated with LA rating (correlation coefficients r≈−1.000, −0.999, respectively), and the left parahippocampal gyrus and amygdala volume (size in voxels) and LA rating were also found to be highly nonlinearly negatively correlated (the correlation coefficients are r≈−0.936, −0.870, respectively). Previous studies have reported that GM tissue loss could subsequently cause lower cognitive reserve (48,49), and that both hippocampal and amygdala atrophy were biomarkers of Alzheimer’s pathology (50-53). Our current study showed results consistent with these reports.
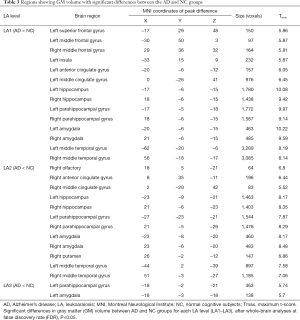
Full table
Discussion
In this study, LA in the brains of AD patients and individuals with normal cognition matched for age, gender, and education was found to have the same rating. Meanwhile, the increase of LA rating with age in AD patients was not directly related to the cognitive changes in MMSE and MoCA in AD patients. These results offer new evidence about WMH and general cognitive function in disabled elderly individuals, such as AD patients. Prior to our study, the Leukoaraiosis And Disability (LADIS) study in the elderly had reported that cognitive impairment was related to the WMH Fazekas score in nondisabled elderly individuals (54).
We reported that LA remodeled the structural connections among rich-club and peripheral regions against hippocampus and amygdala atrophy in AD patients and NC individuals. Based on the characteristics of MRI and cognitive function in AD patients, quantitative MR analysis techniques such as quantitative analysis of white matter fibers and complex graph theory network analysis were used to determine the white matter connections, rich-club and feeder distributions, and node connectivity, as well as GM changes in different LA conditions by quantifying topologies of their respective network representations and VBM. Our data were consistent with previous studies in human brain structural organization. Rich clubs in humans include the precuneus, superior frontal and superior parietal cortex, hippocampus, putamen, and thalamus (30). A greater vulnerability and topological reorganization forms after damage to central hubs or rich clubs (30,55). The results showing that the peripheral brain regions were more likely to be disturbed and the low-degree k-value regions were more affected than the high-degree regions in AD were similar to those of previous studies (43). Our data were also consistent with a former study about anatomical integration and rich-club connectivity, which found that the normalized rich-club coefficient showed trend connectivity effects and rich-club membership differences, as well as global efficiency associated with rich-club connectivity, thus supporting the role of rich-club connections in global integration (22). These phenomena of rich-club organization might help to elucidate a buffer or reserve capacity of the human brain to counteract a certain degree of cognitive decline brought about by aging or disease (56).
Moreover, our data offered new evidences of LA being in the neural basis of the progressive risk for cognitive decline. We successfully leveraged cognitive information and a complex network analysis with its graph theory to describe the collective network dysconnectivity differences in different stages of LA. In NC individuals, although there were significantly increased characteristic path lengths and significantly reduced global efficiency, clustering coefficient, and strength of network connectivity in the NC LA3 group (P<0.05, respectively) compared to in the NC LA1 group, there were no significant differences in their MMSE and MoCA scores. The pattern changes of rich-club, feeder, and local connectivity strength in NC LA3 were similar to those in all AD LA patients. This meant that rich-club regions became involved, and the brain network was damaged more severely as more LA formed due to aging or disease progression.
White matter played an important role in the stable rich-club connections, helping to maintain the core organization of the brain when some rich-club regions suffered from disruptions (30). However, LA might represent a degenerative change of white matter fibers, buffering, modulating, or counteracting a certain degree of neurodegeneration or the progression of diseases such as small vessel diseases or ischaemic disorders, as well as inflammation. Prior to the atrophy of hippocampus and amygdala that are closely related to cognitive function, LA could be an intermediate compensatory change produced by strong brain plasticity and reserve capacity. Although the probabilistic tractography is generally considered a superior method for reconstructing and dissecting individual white matter fascicles, particularly there is a need to quantify the confidence (probability) of each reconstructed pathway and to imagine this kind of compensatory response. Therefore, longitudinal investigations involving bigger cohorts and in vivo quantitative MRI that directly address white matter pathophysiology changes would be helpful to validating our connectivity results and theories.
The analyses in the current study showed only that volume changes of the hippocampus and amygdala played an important role in cognitive function as biomarkers of cognitive decline when LA diffuses throughout the entire brain through aging or disease progression. There were no data available to confirm whether these NC individuals would develop cognitive impairment in the future. A separate longitudinal in-depth study will be required to substantiate this theory.
The suffix “-osis” means “the action or process of”; therefore, leukoaraiosis may help brain GM to organize local interactions to cope with diverse environmental demands, ensuring adaptability, robustness, resilience to damage, efficient message relay, and diverse functionality (communications or neuronal coupling) from a fixed structure. Our current topological network analyses may shed some light on white matter functionality mechanism research. However, our understanding of brain white matter at the network level is still in its infancy; we have studied few aspects of brain networks, and with rather crude measurements. Although the organization of structural networks supports local and global integration (57), the small world of weak ties for global integration from structural connectivity based on DTI cannot resolve intracortical or intrinsic connections (58,59). The structural connectivity based on DTI is potentially blind to weak long-range axonal connections.
Conclusions
Taken together, our in vivo data indicated that LA could serve as an imaging biomarker of the potential or underlying hippocampal and amygdala atrophy. The data provided in this study are valuable for AD research. Our findings offer a deeper understanding of the relationships between LA, GM atrophy, and cognitive function in AD patients and older individuals with normal cognition. However, the current applications of network theory and theoretical neuroscience to brain networks are inceptive in demystifying LA, and give some suggestions and direction for future research in brain white matter, and LADIS as well as brain plasticity. More studies about the relationship between LA and disability, LA and brain plasticity, LA and cognitive rehabilitation and their biology mechanisms will be needed.
Acknowledgments
Data collection and sharing for this project was funded by the Alzheimer’s Disease Neuroimaging Initiative (ADNI) (National Institutes of Health, U01 AG024904) and DOD ADNI (Department of Defense, W81XWH-12-2-0012). Funders for ADNI include the National Institute on Aging, and National Institute of Biomedical Imaging and Bioengineering, and generous contributions have been made by the following: AbbVie; Alzheimer’s Association; Alzheimer’s Drug Discovery Foundation; Araclon Biotech; BioClinica, Inc.; Biogen; Bristol-Myers Squibb; CereSpir, Inc.; Eisai Inc.; Elan Pharmaceuticals, Inc.; Eli Lilly and Company; EuroImmun; F. Hoffmann-La Roche Ltd and its affiliated company Genentech, Inc.; Fujirebio; GE Healthcare; IXICO Ltd.; Janssen Alzheimer Immunotherapy Research & Development, LLC.; Johnson & Johnson Pharmaceutical Research & Development LLC.; Lumosity; Lundbeck; Merck & Co., Inc.; Meso Scale Diagnostics, LLC.; NeuroRx Research; Neurotrack Technologies; Novartis Pharmaceuticals Corporation; Pfizer Inc.; Piramal Imaging; Servier; Takeda Pharmaceutical Company; and Transition Therapeutics. The Canadian Institutes of Health Research is providing funds to support ADNI clinical sites in Canada. Private sector contributions are facilitated by the Foundation for the National Institutes of Health (www.fnih.org). The grantee organization is the Northern California Institute for Research and Education, and the study is coordinated by the Alzheimer’s Disease Cooperative Study at the University of California, San Diego. The ADNI data are disseminated by the Laboratory for Neuro Imaging at the University of Southern California.
Funding: This work was supported by National Natural Science Foundation of China (81830059, 81771889, and 81571655); Science and Technology Commission of the Shanghai Municipality (19411951400); Shanghai Health and Family Planning Committee (2018ZHYL0105); Shanghai Shenkang Hospital Development Center (SHDC22015021); and Fundamental Research Funds for the Central Universities (22120190219); the Shenzhen Municipal Basic Science and Technology Development Project (JCYJ20170818164405101), JCYJ (20190806164409040), Natural Science Foundation of Guangdong Province (2020A1515010918).
Footnote
Conflicts of Interest: All authors have completed the ICMJE uniform disclosure form (available at http://dx.doi.org/10.21037/qims-20-580). The authors have no conflicts of interest to declare.
Ethical Statement: The study was conducted in accordance with the Declaration of Helsinki (as revised in 2013). All Alzheimer’s disease (AD) patients and age- and sex-matched normal cognitive (NC) individuals were selected from the Research Ethics Board approved study database of the Alzheimer’s Disease Neuroimaging Initiative (ADNI) clinical sites. Ethical approval was obtained, and informed consent was provided from all participants.
Open Access Statement: This is an Open Access article distributed in accordance with the Creative Commons Attribution-NonCommercial-NoDerivs 4.0 International License (CC BY-NC-ND 4.0), which permits the non-commercial replication and distribution of the article with the strict proviso that no changes or edits are made and the original work is properly cited (including links to both the formal publication through the relevant DOI and the license). See: https://creativecommons.org/licenses/by-nc-nd/4.0/.
References
- Mills SE. Histology for Pathologists. Philadelphia: Lippincott Williams & Wilkins, 2012:301-2.
- Scarpelli M, Salvolini U, Diamanti L, Montironi R, Chiaromoni L, Maricotti M. MRI and pathological examination of post-mortem brains: the problem of white matter high signal areas. Neuroradiology 1994;36:393-8. [Crossref] [PubMed]
- Brun A, Englund E. A white matter disorder in dementia of the Alzheimer type: a pathoanatomical study. Ann Neurol 1986;19:253-62. [Crossref] [PubMed]
- Scheltens P, Barkhof F, Leys D, Wolters EC, Ravid R, Kamphorst W. Histopathologic correlates of white matter changes on MRI in Alzheimer's disease and normal aging. Neurology 1995;45:883-8. [Crossref] [PubMed]
- van Swieten JC, van den Hout JH, van Ketel BA, Hijdra A, Wokke JH, van Gijn J. Periventricular lesions in the white matter on magnetic resonance imaging in the elderly. A morphometric correlation with arteriolosclerosis and dilated perivascular spaces. Brain 1991;114:761-74. [Crossref] [PubMed]
- Janota I, Mirsen TR, Hachinski VC, Lee DH, Merskey H. Neuropathologic correlates of leuko-araiosis. Arch Neurol 1989;46:1124-8. [Crossref] [PubMed]
- Awad IA, Johnson PC, Spetzler RF, Hodak JA. Incidental subcortical lesions identified on magnetic resonance imaging in the elderly. II. Postmortem pathological correlations. Stroke 1986;17:1090-7. [Crossref] [PubMed]
- Maclullich AM, Wardlaw JM, Ferguson KJ, Starr JM, Seckl JR, Deary IJ. Enlarged perivascular spaces are associated with cognitive function in healthy elderly men. J Neurol Neurosurg Psychiatry 2004;75:1519-23. [Crossref] [PubMed]
- Wardlaw JM, Smith EE, Biessels GJ, Cordonnier C, Fazekas F, Frayne R, Lindley RI, O'Brien JT, Barkhof F, Benavente OR, Black SE, Brayne C, Breteler M, Chabriat H, Decarli C, de Leeuw FE, Doubal F, Duering M, Fox NC, Greenberg S, Hachinski V, Kilimann I, Mok V, Oostenbrugge R, Pantoni L, Speck O, Stephan BC, Teipel S, Viswanathan A, Werring D, Chen C, Smith C, van Buchem M, Norrving B, Gorelick PB, Dichgans M. nEuroimaging STfRVco. Neuroimaging standards for research into small vessel disease and its contribution to ageing and neurodegeneration. Lancet Neurol 2013;12:822-38. [Crossref] [PubMed]
- Ding J, Sigurethsson S, Jonsson PV, Eiriksdottir G, Charidimou A, Lopez OL, van Buchem MA, Guethnason V, Launer LJ. Large Perivascular Spaces Visible on Magnetic Resonance Imaging, Cerebral Small Vessel Disease Progression, and Risk of Dementia: The Age, Gene/Environment Susceptibility-Reykjavik Study. JAMA Neurol 2017;74:1105-12. [Crossref] [PubMed]
- Arba F, Quinn TJ, Hankey GJ, Lees KR, Wardlaw JM, Ali M, Inzitari D, Collaboration V. Enlarged perivascular spaces and cognitive impairment after stroke and transient ischemic attack. Int J Stroke 2018;13:47-56. [Crossref] [PubMed]
- Malone MJ, Szoke MC. Neurochemical changes in white matter. Aged human brain and Alzheimer's disease. Arch Neurol 1985;42:1063-6. [Crossref] [PubMed]
- Hachinski VC, Potter P, Merskey H. Leuko-araiosis. Arch Neurol 1987;44:21-3. [Crossref] [PubMed]
- Ter Telgte A, van Leijsen EMC, Wiegertjes K, Klijn CJM, Tuladhar AM, de Leeuw FE. Cerebral small vessel disease: from a focal to a global perspective. Nat Rev Neurol 2018;14:387-98. [Crossref] [PubMed]
- Wahlund LO, Barkhof F, Fazekas F, Bronge L, Augustin M, Sjogren M, Wallin A, Ader H, Leys D, Pantoni L, Pasquier F, Erkinjuntti T, Scheltens P. European Task Force on Age-Related White Matter C. A new rating scale for age-related white matter changes applicable to MRI and CT. Stroke 2001;32:1318-22. [Crossref] [PubMed]
- Frey BM, Petersen M, Mayer C, Schulz M, Cheng B, Thomalla G. Characterization of White Matter Hyperintensities in Large-Scale MRI-Studies. Front Neurol 2019;10:238. [Crossref] [PubMed]
- Rubinov M, Sporns O. Complex network measures of brain connectivity: uses and interpretations. Neuroimage 2010;52:1059-69. [Crossref] [PubMed]
- Mezer A, Yeatman JD, Stikov N, Kay KN, Cho NJ, Dougherty RF, Perry ML, Parvizi J. Hua le H, Butts-Pauly K, Wandell BA. Quantifying the local tissue volume and composition in individual brains with magnetic resonance imaging. Nat Med 2013;19:1667-72. [Crossref] [PubMed]
- Hahm S, Lotze M, Domin M, Schmidt S. The association of health-related quality of life and cerebral gray matter volume in the context of aging: A voxel-based morphometry study with a general population sample. Neuroimage 2019;191:470-80. [Crossref] [PubMed]
- Petersen RC, Aisen PS, Beckett LA, Donohue MC, Gamst AC, Harvey DJ, Jack CR Jr, Jagust WJ, Shaw LM, Toga AW, Trojanowski JQ, Weiner MW. Alzheimer's Disease Neuroimaging Initiative (ADNI): clinical characterization. Neurology 2010;74:201-9. [Crossref] [PubMed]
- Levitt JJ, Alvarado JL, Nestor PG, Rosow L, Pelavin PE, McCarley RW, Kubicki M, Shenton ME. Fractional anisotropy and radial diffusivity: diffusion measures of white matter abnormalities in the anterior limb of the internal capsule in schizophrenia. Schizophr Res 2012;136:55-62. [Crossref] [PubMed]
- O'Donoghue S, Kilmartin L, O'Hora D, Emsell L, Langan C, McInerney S, Forde NJ, Leemans A, Jeurissen B, Barker GJ, McCarthy P, Cannon DM, McDonald C. Anatomical integration and rich-club connectivity in euthymic bipolar disorder. Psychol Med 2017;47:1609-23. [Crossref] [PubMed]
- Bullmore E, Sporns O. Complex brain networks: graph theoretical analysis of structural and functional systems. Nat Rev Neurosci 2009;10:186-98. [Crossref] [PubMed]
- Bullmore E, Sporns O. The economy of brain network organization. Nat Rev Neurosci 2012;13:336-49. [Crossref] [PubMed]
- Emsell L, Langan C, Van Hecke W, Barker GJ, Leemans A, Sunaert S, McCarthy P, Nolan R, Cannon DM, McDonald C. White matter differences in euthymic bipolar I disorder: a combined magnetic resonance imaging and diffusion tensor imaging voxel-based study. Bipolar Disord 2013;15:365-76. [Crossref] [PubMed]
- Emsell L, Leemans A, Langan C, Van Hecke W, Barker GJ, McCarthy P, Jeurissen B, Sijbers J, Sunaert S, Cannon DM, McDonald C. Limbic and callosal white matter changes in euthymic bipolar I disorder: an advanced diffusion magnetic resonance imaging tractography study. Biol Psychiatry 2013;73:194-201. [Crossref] [PubMed]
- Zalesky A, Fornito A, Bullmore ET. Network-based statistic: identifying differences in brain networks. Neuroimage 2010;53:1197-207. [Crossref] [PubMed]
- Zalesky A, Cocchi L, Fornito A, Murray MM, Bullmore E. Connectivity differences in brain networks. Neuroimage 2012;60:1055-62. [Crossref] [PubMed]
- Mcauley JJ, Costa LDF, Caetano TSJ. Rich-club phenomena across complex network hierachies. Applied Physics Letters 2007;91:84103. [Crossref]
- van den Heuvel MP, Sporns O. Rich-club organization of the human connectome. J Neurosci 2011;31:15775-86. [Crossref] [PubMed]
- Daianu M, Jahanshad N, Nir TM, Jack CR Jr, Weiner MW, Bernstein MA, Thompson PM. Alzheimer's Disease Neuroimaging I. Rich club analysis in the Alzheimer's disease connectome reveals a relatively undisturbed structural core network. Hum Brain Mapp 2015;36:3087-103. [Crossref] [PubMed]
- van den Heuvel MP, Sporns O, Collin G, Scheewe T, Mandl RC, Cahn W, Goni J, Hulshoff Pol HE, Kahn RS. Abnormal rich club organization and functional brain dynamics in schizophrenia. JAMA Psychiatry 2013;70:783-92. [Crossref] [PubMed]
- Daianu M, Mezher A, Mendez MF, Jahanshad N, Jimenez EE, Thompson PM. Disrupted rich club network in behavioral variant frontotemporal dementia and early-onset Alzheimer's disease. Hum Brain Mapp 2016;37:868-83. [Crossref] [PubMed]
- Collin G, Kahn RS, de Reus MA, Cahn W, van den Heuvel MP. Impaired rich club connectivity in unaffected siblings of schizophrenia patients. Schizophr Bull 2014;40:438-48. [Crossref] [PubMed]
- Collin G, Sporns O, Mandl RC, van den Heuvel MP. Structural and functional aspects relating to cost and benefit of rich club organization in the human cerebral cortex. Cereb Cortex 2014;24:2258-67. [Crossref] [PubMed]
- Colizza V, Flammini A, Serrano MA, Vespignani AJNP. Detecting rich-club ordering in complex networks. Nat. Phys 2(2), 110-115. 2006;2:110-5.
- van den Heuvel MP, Kahn RS, Goni J, Sporns O. High-cost, high-capacity backbone for global brain communication. Proc Natl Acad Sci U S A 2012;109:11372-7. [Crossref] [PubMed]
- Benjamini Y, Hochberg Y. Controlling the false discovery rate: a practical and powerful approach to multiple testing. J R Stat Soc Series B Stat Methodol 1995;57:289-300.
- Bassett DS, Sporns O. Network neuroscience. Nat Neurosci 2017;20:353-64. [Crossref] [PubMed]
- Basser PJ, Mattiello J, LeBihan D. MR diffusion tensor spectroscopy and imaging. Biophys J 1994;66:259-67. [Crossref] [PubMed]
- Park HJ, Kubicki M, Westin CF, Talos IF, Brun A, Peiper S, Kikinis R, Jolesz FA, McCarley RW, Shenton ME. Method for combining information from white matter fiber tracking and gray matter parcellation. AJNR Am J Neuroradiol 2004;25:1318-24. [PubMed]
- Hagmann P, Kurant M, Gigandet X, Thiran P, Wedeen VJ, Meuli R, Thiran JP. Mapping human whole-brain structural networks with diffusion MRI. PLoS One 2007;2:e597. [Crossref] [PubMed]
- Yan T, Wang W, Yang L, Chen K, Chen R, Han Y. Rich club disturbances of the human connectome from subjective cognitive decline to Alzheimer's disease. Theranostics 2018;8:3237-55. [Crossref] [PubMed]
- Seo SW, Lee JM, Im K, Park JS, Kim SH, Kim ST, Ahn HJ, Chin J, Cheong HK, Weiner MW, Na DL. Cortical thinning related to periventricular and deep white matter hyperintensities. Neurobiol Aging 2012;33:1156-67. [Crossref] [PubMed]
- Wang J, Liang Y, Chen H, Wang W, Wang Y, Liang Y, Zhang Y. Structural changes in white matter lesion patients and their correlation with cognitive impairment. Neuropsychiatr Dis Treat 2019;15:1355-63. [Crossref] [PubMed]
- Raji CA, Lopez OL, Kuller LH, Carmichael OT, Longstreth WT Jr, Gach HM, Boardman J, Bernick CB, Thompson PM, Becker JT. White matter lesions and brain gray matter volume in cognitively normal elders. Neurobiol Aging 2012;33:834.e7-16. [PubMed]
- Kim HJ, Im K, Kwon H, Lee JM, Kim C, Kim YJ, Jung NY, Cho H, Ye BS, Noh Y, Kim GH, Ko ED, Kim JS, Choe YS, Lee KH, Kim ST, Lee JH, Ewers M, Weiner MW, Na DL, Seo SW. Clinical effect of white matter network disruption related to amyloid and small vessel disease. Neurology 2015;85:63-70. [Crossref] [PubMed]
- Stern Y, Alexander GE, Prohovnik I, Mayeux R. Inverse relationship between education and parietotemporal perfusion deficit in Alzheimer's disease. Ann Neurol 1992;32:371-5. [Crossref] [PubMed]
- Sandry J, DeLuca J, Chiaravalloti N. Working memory capacity links cognitive reserve with long-term memory in moderate to severe TBI: a translational approach. J Neurol 2015;262:59-64. [Crossref] [PubMed]
- Murray AD, Staff RT, McNeil CJ, Salarirad S, Ahearn TS, Mustafa N, Whalley LJ. The balance between cognitive reserve and brain imaging biomarkers of cerebrovascular and Alzheimer's diseases. Brain 2011;134:3687-96. [Crossref] [PubMed]
- Prieto Del Val L, Cantero JL, Atienza M. Atrophy of amygdala and abnormal memory-related alpha oscillations over posterior cingulate predict conversion to Alzheimer's disease. Sci Rep 2016;6:31859. [Crossref] [PubMed]
- Horínek D, Varjassyova A, Hort J. Magnetic resonance analysis of amygdalar volume in Alzheimer's disease. Curr Opin Psychiatry 2007;20:273-7. [Crossref] [PubMed]
- Klein-Koerkamp Y, Heckemann RA, Ramdeen KT, Moreaud O, Keignart S, Krainik A, Hammers A, Baciu M, Hot P, Alzheimer'sdisease Neuroimaging I. Amygdalar atrophy in early Alzheimer's disease. Curr Alzheimer Res 2014;11:239-52. [Crossref] [PubMed]
- van der Flier WM, van Straaten EC, Barkhof F, Verdelho A, Madureira S, Pantoni L, Inzitari D, Erkinjuntti T, Crisby M, Waldemar G, Schmidt R, Fazekas F, Scheltens P. Small vessel disease and general cognitive function in nondisabled elderly: the LADIS study. Stroke 2005;36:2116-20. [Crossref] [PubMed]
- Alstott J, Breakspear M, Hagmann P, Cammoun L, Sporns O. Modeling the impact of lesions in the human brain. PLoS Comput Biol 2009;5:e1000408. [Crossref] [PubMed]
- Staff RT. Reserve, brain changes, and decline. Neuroimaging Clin N Am 2012;22:99-105. viii-iv. [Crossref] [PubMed]
- Park HJ, Friston K. Structural and functional brain networks: from connections to cognition. Science 2013;342:1238411. [Crossref] [PubMed]
- Markov NT, Misery P, Falchier A, Lamy C, Vezoli J, Quilodran R, Gariel MA, Giroud P, Ercsey-Ravasz M, Pilaz LJ, Huissoud C, Barone P, Dehay C, Toroczkai Z, Van Essen DC, Kennedy H, Knoblauch K. Weight consistency specifies regularities of macaque cortical networks. Cereb Cortex 2011;21:1254-72. [Crossref] [PubMed]
- Gallos LK, Makse HA, Sigman M. A small world of weak ties provides optimal global integration of self-similar modules in functional brain networks. Proc Natl Acad Sci U S A 2012;109:2825-30. [Crossref] [PubMed]