Heterogeneous parameters based on 18F-FET PET imaging can non-invasively predict tumor grade and isocitrate dehydrogenase gene 1 mutation in untreated gliomas
Introduction
Prognostication and treatment monitoring are related to glioma grade. Patients with different glioma grades have different natural histories and treatments. Isocitrate dehydrogenase (IDH) gene mutation is frequently observed in grades II and III glioma and secondary glioblastoma patients. In contrast, only limited IDH mutations are observed in primary glioblastoma patients (1,2). IDH-mutated gliomas have different biologic behavior and better treatment response and prognosis than IDH wild-type gliomas (3). Both glioma grade and IDH mutation status need to be confirmed by pathological results based on surgical resection or biopsy tissues. Non-invasive glioma grade and IDH mutation status prediction are crucial for treatment decision-making and prognostic evaluation in untreated glioma patients (4).
Amino acid tracer positron emission tomography (PET) imaging focuses on the metabolic status of glioma cells and could be used as an effective complementary diagnostic modality besides magnetic resonance imaging (5). O-(2-[18F]fluoroethyl)-l-tyrosine (18F-FET) is an amino acid tracer that generally has significant uptake in glioma tissues and lower uptake in background brain tissues. The characteristic of 18F-FET PET imaging has led to wide clinical application, including grading (6,7), differential diagnosis (8,9), delineation of tumors (10,11), treatment plan decision-making (12-14), and prognostication (15,16), and has been recommended for use during all phases of glioma diagnosis and treatment (17,18).
Diagnostic semi-quantitative parameters, including maximal tumor-to-background ratio (TBRmax) and mean tumor-to-background ratio (TBRmean), can be obtained from static 18F-FET imaging. Volumetric parameters, such as metabolic tumor volume (MTV) and total lesion tracer standardized uptake (TLU), which is similar to total lesion glycolysis in 18F-fluorodeoxyglucose (FDG) imaging, are widely used in tumor imaging analysis (19,20). Dynamic 18F-FET imaging could provide extra tracer uptake information other than static imaging (21). Further imaging analyses, such as texture analyses and radiomics, can be used for imaging data mining to extract a vast amount of imaging features for analysis; however, these techniques are complicated and not suitable for routine clinical imaging analysis and diagnosis.
Heterogeneity is a feature of malignant tumors. Intratumoral heterogeneous parameters based on 18F-FDG PET imaging, such as the heterogeneity index (HI) and coefficient of variation (COV) of the tumor lesion, have been used in predicting treatment response and prognosis, and in the differentiation between benign and malignant lymph nodes in different tumors (22-25). Based on their nature, these intratumoral, heterogeneous, semi-quantitative parameters have the potential to predict glioma grade and IDH mutation status.
The purpose of the present study was to investigate the non-invasive predictive efficacy of heterogeneous parameters, other than the regular static parameters of 18F-FET PET imaging, in glioma grade and IDH1 mutation.
Methods
The institutional ethics board approved the present study of Huashan Hospital, Fudan University (No. 2017-332). Due to the retrospective nature of the study and anonymous clinical data, written informed consent from enrolled patients was waived. The design and methods involved in this research complied with the Declaration of Helsinki and its amendments.
Patients
In this single-center research study, we retrospectively reviewed 280 consecutive adult 18F-FET PET/computed tomography (CT) patients referred to the PET Center of Huashan Hospital, Fudan University, from November 2017 to December 2018. The inclusion criteria were as follows: (I) adult patients with an untreated brain lesion; (II) positive 18F-FET PET imaging findings without artifacts; and (III) a confirmed histological diagnosis of glioma and IDH mutation status after 18F-FET PET imaging. The exclusion criteria were as follows: (I) any diagnostic and/or treatment procedures before 18F-FET PET investigation; (II) patients without a pathological diagnosis after 18F-FET PET imaging; and (III) negative or background-like 18F-FET PET uptake of a tumor lesion. The interval between 18F-FET PET imaging and following surgical resection or biopsy was no more than 90 days for grade II or III glioma patients, and no more than 30 days for grade IV glioma patients. Based on these criteria, 58 adult patients with untreated gliomas were enrolled in the present study.
Patient information was obtained and reviewed, including demographic and clinical data, treatment plan, and pathological diagnosis.
18F-FET PET/CT imaging and analysis
18F-FET PET scan was performed using a Biograph 64 PET/CT system (Siemens, Erlangen, Germany). Patients fasted for at least 4 h before 18F-FET PET imaging, and 370±20 MBq 18F-FET was intravenously injected before the PET/CT scan. A static scan was performed 20 min after the tracer injection and lasted for 20 min. In total, 8 of 58 patients underwent dynamic 18F-FET PET scanning. The dynamic scan was performed for >40 min after the tracer injection; 20–40-min images were reconstructed for diagnosis and analysis. Attenuation correction was performed using a low-dose CT (120 KV, 150 mA, Acq. 64 mm × 0.6 mm, 3-mm slice thickness, and 0.55 pitch) before the emission scan. PET images were reconstructed using the iterative 3D method with a Gaussian filter (6 iterations, 14 subsets, full width at half maximum: 2 mm, zoom: 2), which resulted in axial, coronal, and sagittal brain imaging of PET, CT, and PET/CT fusion for diagnosis and analysis.
The Syngo.via workstation (Siemens, Erlangen, Germany) was used to analyze the 18F-FET PET/CT imaging. Background mean standardized uptake value was measured in a crescent-shape area, including gray and white matter on the contralateral hemisphere (26). Tumor maximal standardized uptake value (SUVmax), peak standardized uptake value (SUVpeak), mean standardized uptake value (SUVmean), the standard deviation of lesion standardized uptake value (SUVsd), and MTV of the tumor lesion were semi-automatically obtained after glioma volume of interest (VOI) delineation, with a threshold of 1.6 times that of background SUVmean (27). Background brain tissue SUVmean and glioma lesion VOI delineation were determined by 2 experienced nuclear medicine physicians (TH: 10 years’ experience, WZ: 5 years’ experience) using 2 separate measurements. Each physician applied the same lesion delineation procedures, and the other physician ensured that the procedures were carried out appropriately. After the first physician completed the procedures, the physicians switched roles and repeated the lesion delineation procedure. We also analyzed the interobserver agreement indices in order to reach measurement consensus. TBRmax, peak tumor-to-background ratio (TBRpeak), and TBRmean were calculated by the division of tumor SUVmax, SUVpeak, and SUVmean with background SUVmean. The SUVsd of lesion VOI divided by lesion SUVmean was used to obtain the COV of the lesion. Lesion SUVmax divided by lesion SUVmean was applied to obtain the lesion HI. TLU was calculated as MTV×lesion SUVmean.
Pathological diagnosis
Glioma tissues obtained from surgical resection or stereotactic biopsy were used for the pathological diagnosis. Hematoxylin-eosin staining results and IDH1 R132H immunohistochemical staining results were combined for the pathological diagnosis. The 2016 World Health Organization glioma classification was incorporated in the diagnosis if the necessary molecular biomarker data were complete. All pathological diagnoses were completed in the Pathology Department of Huashan Hospital, Fudan University (Shanghai, China).
Statistical analysis
Means and standard deviations or medians and ranges were calculated in continuous variables. Percentages were used for dichotomous and categorical variables. Logistic regression was used to investigate the predictive efficacy of the parameters; the variance inflation factor was observed to control multicollinearity. Receiver-operating characteristic curve analysis was applied to evaluate different predictive models. A reasonable cutoff was decided when the combination of sensitivity and specificity reached its maximum. The area under the curve (AUC) was calculated to compare predictive efficacy. The Hosmer-Lemeshow goodness-of-fit test was used for the calibration of models. Student’s t-test was used to compare continuous variables between 2 groups if the variables were normally distributed and adjustment was considered based on equal or unequal variances; Wilcoxon rank-sum test was performed if the normal distribution of variables was not met. One-way analysis of variance was used to compare continuous variables between different glioma grades; non-parametric Kruskal-Wallis tests were used if the normal distribution or equal variance criteria were not met. P<0.05 was considered statistically significant. Interobserver agreements of 18F-FET PET parameter measurements were evaluated with interclass correlation coefficients (ICC), defined as poor (<0.2), fair (0.21–0.4), moderate (0.41–0.6), good (0.61–0.8), and very good (0.81–1.0). Statistical analyses were completed with STATA version 14.1 (College Station, TX, USA) and MedCalc version 13.1.0 (https://www.mdcalc.com/).
Results
Patient characteristics
Patients’ demographic and clinical data are summarized in Table 1. There were 33, 13, and 12 patients with grades II, III, and IV gliomas, respectively. Interested reader can find the detailed data in a supplementary appendix online (Table S1).
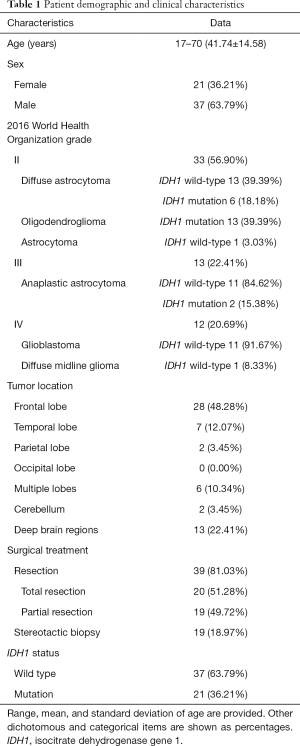
Full table
Interobserver agreement results
The ICC showed satisfactory agreement for the 18F-FET PET semi-quantitative parameter measurement results between the 2 physicians (ICC >0.95, P<0.01). Based on those findings, the first physician’s measurement results (TH) were used for the following analysis. The means and standard deviations of the semi-quantitative parameters among the different glioma grades and the IDH1 status are shown in Table 2.
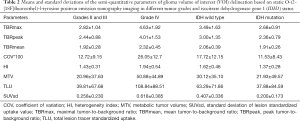
Full table
Predictive efficacy of gliomas between grade II or III and grade IV
All 8 semi-quantitative parameters (TBRmax, TBRpeak, TBRmean, HI, COV, SUVsd, MTV, and TLU) could significantly differentiate between grade II or III and grade IV gliomas (P<0.0001). TLU (AUC: 0.841, P<0.0001), followed by TBRpeak (AUC: 0.832, P<0.0001) and HI (AUC: 0.826, P<0.0001), had the top 3 single-parameter predictive performance. Combinations of TBRmax, SUVsd, and TBRmean (AUC: 0.850, P<0.0001); HI, SUVsd, and MTV (AUC: 0.848, P<0.0001); and HI, SUVsd, and TLU (AUC: 0.848, P<0.0001) had the top 3 multiple-parameter predictive performance (Figure 1 and Table 3).
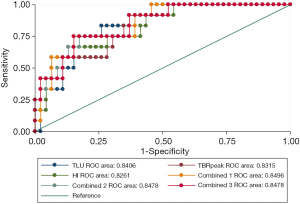
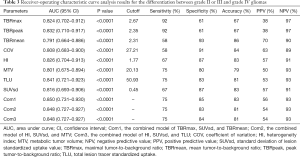
Full table
The P value of the difference between the top 3 combined models and the top 3 single-parameter predictive models was insignificant (P=0.752). The Hosmer-Lemeshow goodness-of-fit test results indicated that, for these models, P>0.05, indicating satisfactory calibration (Tables S2,S3).
Predictive efficacy of IDH1 mutation based on immunohistochemical analysis
Five of the 8 parameters (SUVsd, TLU, HI, MTV, and TBRmax) could significantly predict the IDH1 mutation, whereas the predictive efficacy of COV, TBRpeak, and TBRmean was around borderline. SUVsd (AUC: 0.710, P=0.0028), TLU (AUC: 0.698, P=0.0074), and HI (AUC: 0.676, P=0.0159) had the top 3 single-parameter predictive performance. Combinations of TBRmax, SUVsd, and TBRmean (AUC: 0.821, P<0.0001), SUVsd and TBRmean (AUC: 0.804, P<0.0001), and SUVsd, HI, and TBRmean (AUC: 0.799, P<0.0001) had the top 3 multiple-parameter predictive performance (Figure 2 and Table 4).
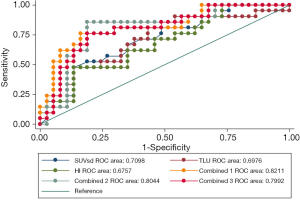
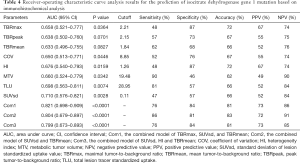
Full table
The P value of the difference between the top 3 combined models and the top 3 single-parameter predictive models was insignificant (P=0.155). The Hosmer-Lemeshow goodness-of-fit test results indicated that, for these models, P>0.05, indicating satisfactory calibration (Tables S4,S5).
Grading efficacy of the semi-quantitative parameters
A descriptive statistical analysis was performed to investigate the efficacy of these parameters. All 8 semi-quantitative parameters showed statistical significance between grade II or III and grade IV gliomas (Table 5). For differentiation between grades II and III gliomas, none of these 8 parameters could significantly differentiate between these 2 groups. For differentiation between grades II and IV gliomas, all 8 parameters could significantly differentiate between these 2 groups. For differentiation between grades III and IV gliomas, TBRpeak, TBRmax, and HI showed a significant difference between these 2 groups, whereas the COV P value was around borderline (Table 5).
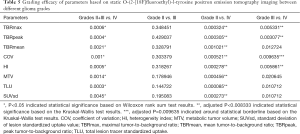
Full table
Discussion
In the present study, we investigated the non-invasive predictive efficacy of some easily obtained and highly repetitive intratumoral heterogeneous parameters, other than the commonly used semi-quantitative parameters, after lesion delineation based on static 18F-FET PET/CT imaging in glioma patients. To the best of our knowledge, this is the first report demonstrating how these extra intratumoral heterogeneous parameters could predict glioma grade and IDH1 mutation status based on static 18F-FET PET imaging analysis.
The main findings of the present study are as follows. First, all the semi-quantitative parameters based on static 18F-FET PET imaging could significantly differentiate between glioma grade II or III and grade IV. TLU, TBRpeak, and HI had the top 3 single-parameter predictive performance, and combinations of TBRmax, SUVsd, and TBRmean had the best multiple-parameter predictive performance. Second, SUVsd, TLU, HI, MTV, and TBRmax could significantly predict IDH1 mutation status based on the immunohistochemical analysis results, whereas the combination of TBRmax, SUVsd, and TBRmean had the best multiple-parameter predictive performance. Third, the 8 semi-quantitative parameters demonstrating grading efficacy in grades II, III, and IV gliomas to some degree.
SUVpeak can be defined as the highest mean SUV from a fixed 1-cm3 spherical volume centered over the highest metabolic part of the tumor VOI. SUVsd is the parameter describing the standard deviation of lesion delineation standardized uptake value. TBRpeak, HI, COV, and SUVsd, further describe the intratumoral imaging heterogeneity features, other than those commonly used, such as TBRmax and TBRmean. During the static 18F-FET imaging analysis of glioma patients, well-accepted procedures of lesion VOI delineation can be semi-automatically completed with the aid of 1.6 times the background SUVmean (27). As a result, all 8 semi-quantitative parameters could be conveniently obtained and reproduced after lesion delineation, which will help routine clinical imaging diagnoses and further imaging analyses.
The multiple-parameter models showed some efficacy enhancement in both glioma grade and IDH1 status prediction, but the probability value indicated that there was no significant difference between the models. A large number of patients and complete molecular biomarker status information are warranted to investigate further the efficacy of these intratumoral heterogeneous parameters based on 18F-FET PET imaging.
Different cell groups in malignant tumors could have different behaviors and treatment responses, and the heterogeneity of malignant tumors is related to oxygen consumption and glucose metabolism (28). Previous studies have demonstrated that heterogeneous parameters, such as HI and COV in 18F-FDG PET imaging, could benefit treatment response evaluation and patient outcomes in non-small cell lung cancer, rectal carcinoma, and nasopharyngeal carcinoma (29-31). The findings of the present study confirm the potential of these intratumoral heterogeneous semi-quantitative parameters after lesion delineation procedures based on static 18F-FET PET/CT imaging, which could also contribute to the further understanding of glioma heterogeneity mechanisms in amino acid tracer imaging.
The IDH mutation status of glioma patients is an important prognostic factor. In some glioma classifications, the IDH mutation could indicate better treatment response and prognosis (32). Our findings demonstrated that SUVsd, TLU, HI, MTV, and TBRmax have significant predictive efficacy in IDH1 mutation, which indicates that these semi-quantitative parameters could also contribute to the non-invasive prediction of the IDH1 mutation status of glioma patients, other than the most commonly used TBRmax. SUVsd and HI demonstrated reasonable predictive efficacy in IDH1 mutation status in our research, indicating that the intratumoral heterogeneity of glioma 18F-FET PET/CT imaging could be related to glioma IDH1 mutation status. TLU and MTV are metabolic volumetric semi-quantitative parameters often associated with tumor treatment response and prognosis (19,20). An interesting finding of our research was that these volumetric semi-quantitative parameters had shown potential in IDH1 mutation status prediction. IDH1 mutation status in glioma patients could affect tumor growth and 18F-FET metabolic patterns, which, as a result, could lead to different treatment results and prognoses.
Although these static semi-quantitative parameters have many benefits, there are still challenges in 18F-FET PET imaging, such as the need for better non-invasive predictive efficacy in the IDH1 genotype and glioma grade, as well as the need for better grading efficacy between grades II and III gliomas. Dynamic 18F-FET PET imaging could provide additional valuable parameters, such as lesion time activity curve pattern, time to peak, and slope (21). Moreover, dynamic imaging parameters of amino acid tracer PET could also enhance the performance of static imaging parameters for IDH mutation detection (33). More complicated texture and radiomics analysis could also be applied in glioma imaging research (34-36). We believe that these easily applied and relatively stable parameters could be used in combination with dynamic 18F-FET PET imaging parameters and other more complicated methods for glioma imaging prediction analyses.
The present study has some limitations. The retrospective nature of the study, the relatively low number of patients, and incomplete molecular pathological diagnostic data limit the strength of the results. To avoid a false-negative IDH mutation status, IDH sequencing should be used for negative IDH immunohistochemical analysis results. A larger number of patients and more comprehensive pathological biomarker status information are needed in further studies of the non-invasive predictive efficacy of static 18F-FET PET imaging parameters in adults with untreated gliomas.
These convenient heterogeneous semi-quantitative parameters based on static 18F-FET PET imaging could benefit the non-invasive prediction of glioma grade and IDH1 mutation status in adults with untreated gliomas, which could influence treatment decision-making and the prognostic evaluation.
Acknowledgments
Funding: This research has received grant support from the National Natural Science Foundation of China (grant No. 81701755) and Science and Technology Commission of Shanghai Municipality (grant No.18411952100, 2018SHZDZX01), and ZJ Lab.
Footnote
Conflicts of Interest: All authors have completed the ICMJE uniform disclosure form (available at http://dx.doi.org/10.21037/qims-20-723). The authors have no conflicts of interest to declare.
Ethical Statement: The ethical committee approved the present study of Huashan Hospital, Fudan University (No. 2017-332). Due to the retrospective nature of the study and anonymous clinical data, written informed consent from enrolled patients was waived. The design and methods involved in this research complied with the Declaration of Helsinki and its amendments.
Open Access Statement: This is an Open Access article distributed in accordance with the Creative Commons Attribution-NonCommercial-NoDerivs 4.0 International License (CC BY-NC-ND 4.0), which permits the non-commercial replication and distribution of the article with the strict proviso that no changes or edits are made and the original work is properly cited (including links to both the formal publication through the relevant DOI and the license). See: https://creativecommons.org/licenses/by-nc-nd/4.0/.
References
- Lohmann P, Lerche C, Bauer EK, Steger J, Stoffels G, Blau T, Dunkl V, Kocher M, Viswanathan S, Filss CP, Stegmayr C, Ruge MI, Neumaier B, Shah NJ, Fink GR, Langen KJ, Galldiks N. Predicting IDH genotype in gliomas using FET PET radiomics. Sci Rep 2018;8:13328. [Crossref] [PubMed]
- Eckel-Passow JE, Lachance DH, Molinaro AM, Walsh KM, Decker PA, Sicotte H, Pekmezci M, Rice T, Kosel ML, Smirnov IV, Sarkar G, Caron AA, Kollmeyer TM, Praska CE, Chada AR, Halder C, Hansen HM, McCoy LS, Bracci PM, Marshall R, Zheng S, Reis GF, Pico AR, O'Neill BP, Buckner JC, Giannini C, Huse JT, Perry A, Tihan T, Berger MS, Chang SM, Prados MD, Wiemels J, Wiencke JK, Wrensch MR, Jenkins RB. Glioma Groups Based on 1p/19q, IDH, and TERT Promoter Mutations in Tumors. N Engl J Med 2015;372:2499-508. [Crossref] [PubMed]
- Bready D, Placantonakis DG. Molecular Pathogenesis of Low-Grade Glioma. Neurosurg Clin N Am 2019;30:17-25. [Crossref] [PubMed]
- Verger A, Stoffels G, Bauer EK, Lohmann P, Blau T, Fink GR, Neumaier B, Shah NJ, Langen KJ, Galldiks N. Static and dynamic (18)F-FET PET for the characterization of gliomas defined by IDH and 1p/19q status. Eur J Nucl Med Mol Imaging 2018;45:443-51. [Crossref] [PubMed]
- Shooli H, Dadgar H, Wang YJ, Vafaee MS, Kashuk SR, Nemati R, Jafari E, Nabipour I, Gholamrezanezhad A, Assadi M, Larvie M. An update on PET-based molecular imaging in neuro-oncology: challenges and implementation for a precision medicine approach in cancer care. Quant Imaging Med Surg 2019;9:1597-610. [Crossref] [PubMed]
- Calcagni ML, Galli G, Giordano A, Taralli S, Anile C, Niesen A, Baum RP. Dynamic O-(2-[18F]fluoroethyl)-L-tyrosine (F-18 FET) PET for glioma grading: assessment of individual probability of malignancy. Clin Nucl Med 2011;36:841-7. [Crossref] [PubMed]
- Jansen NL, Suchorska B, Wenter V, Eigenbrod S, Schmid-Tannwald C, Zwergal A, Niyazi M, Drexler M, Bartenstein P, Schnell O, Tonn JC, Thon N, Kreth FW, la Fougère C. Dynamic 18F-FET PET in newly diagnosed astrocytic low-grade glioma identifies high-risk patients. J Nucl Med 2014;55:198-203. [Crossref] [PubMed]
- Rapp M, Heinzel A, Galldiks N, Stoffels G, Felsberg J, Ewelt C, Sabel M, Steiger HJ, Reifenberger G, Beez T, Coenen HH, Floeth FW, Langen KJ. Diagnostic performance of 18F-FET PET in newly diagnosed cerebral lesions suggestive of glioma. J Nucl Med 2013;54:229-35. [Crossref] [PubMed]
- Galldiks N, Dunkl V, Stoffels G, Hutterer M, Rapp M, Sabel M, Reifenberger G, Kebir S, Dorn F, Blau T, Herrlinger U, Hau P, Ruge MI, Kocher M, Goldbrunner R, Fink GR, Drzezga A, Schmidt M, Langen KJ. Diagnosis of pseudoprogression in patients with glioblastoma using O-(2-[18F]fluoroethyl)-L-tyrosine PET. Eur J Nucl Med Mol Imaging 2015;42:685-95. [Crossref] [PubMed]
- Vees H, Senthamizhchelvan S, Miralbell R, Weber DC, Ratib O, Zaidi H. Assessment of various strategies for 18F-FET PET-guided delineation of target volumes in high-grade glioma patients. Eur J Nucl Med Mol Imaging 2009;36:182-93. [PubMed]
- Kunz M, Thon N, Eigenbrod S, Hartmann C, Egensperger R, Herms J, Geisler J, la Fougere C, Lutz J, Linn J, Kreth S, von Deimling A, Tonn JC, Kretzschmar HA, Pöpperl G, Kreth FW. Hot spots in dynamic (18)FET-PET delineate malignant tumor parts within suspected WHO grade II gliomas. Neuro Oncol 2011;13:307-16. [Crossref] [PubMed]
- Ewelt C, Floeth FW, Felsberg J, Steiger HJ, Sabel M, Langen KJ, Stoffels G, Stummer W. Finding the anaplastic focus in diffuse gliomas: the value of Gd-DTPA enhanced MRI, FET-PET, and intraoperative, ALA-derived tissue fluorescence. Clin Neurol Neurosurg 2011;113:541-7. [Crossref] [PubMed]
- Niyazi M, Geisler J, Siefert A, Schwarz SB, Ganswindt U, Garny S, Schnell O, Suchorska B, Kreth FW, Tonn JC, Bartenstein P, la Fougère C, Belka C. FET-PET for malignant glioma treatment planning. Radiother Oncol 2011;99:44-8. [Crossref] [PubMed]
- Galldiks N, Law I, Pope WB, Arbizu J, Langen KJ. The use of amino acid PET and conventional MRI for monitoring of brain tumor therapy. Neuroimage Clin 2016;13:386-94. [Crossref] [PubMed]
- Piroth MD, Holy R, Pinkawa M, Stoffels G, Kaiser HJ, Galldiks N, Herzog H, Coenen HH, Eble MJ, Langen KJ. Prognostic impact of postoperative, pre-irradiation (18)F-fluoroethyl-l-tyrosine uptake in glioblastoma patients treated with radiochemotherapy. Radiother Oncol 2011;99:218-24. [Crossref] [PubMed]
- Piroth MD, Pinkawa M, Holy R, Klotz J, Nussen S, Stoffels G, Coenen HH, Kaiser HJ, Langen KJ, Eble MJ. Prognostic value of early [18F]fluoroethyltyrosine positron emission tomography after radiochemotherapy in glioblastoma multiforme. Int J Radiat Oncol Biol Phys 2011;80:176-84. [Crossref] [PubMed]
- Law I, Albert NL, Arbizu J, Boellaard R, Drzezga A, Galldiks N, la Fougère C, Langen KJ, Lopci E, Lowe V, McConathy J, Quick HH, Sattler B, Schuster DM, Tonn JC, Weller M. Joint EANM/EANO/RANO practice guidelines/SNMMI procedure standards for imaging of gliomas using PET with radiolabelled amino acids and [(18)F]FDG: version 1.0. Eur J Nucl Med Mol Imaging 2019;46:540-57. [Crossref] [PubMed]
- Albert NL, Weller M, Suchorska B, Galldiks N, Soffietti R, Kim MM, laFougère C, Pope W, Law I, Arbizu J, Chamberlain MC, Vogelbaum M, Ellingson BM, Tonn JC. Response Assessment in Neuro-Oncology working group and European Association for Neuro-Oncology recommendations for the clinical use of PET imaging in gliomas. Neuro Oncol 2016;18:1199-208. [Crossref] [PubMed]
- Lopez CJ, Nagornaya N, Parra NA, Kwon D, Ishkanian F, Markoe AM, Maudsley A, Stoyanova R. Association of Radiomics and Metabolic Tumor Volumes in Radiation Treatment of Glioblastoma Multiforme. Int J Radiat Oncol Biol Phys 2017;97:586-95. [Crossref] [PubMed]
- Nakajo M, Kajiya Y, Tani A, Jinguji M, Nakajo M, Kitazono M, Yoshiura T. A pilot study for texture analysis of 18F-FDG and 18F-FLT-PET/CT to predict tumor recurrence of patients with colorectal cancer who received surgery. Eur J Nucl Med Mol Imaging 2017;44:2158-68. [Crossref] [PubMed]
- Röhrich M, Huang K, Schrimpf D, Albert NL, Hielscher T, von Deimling A, Schüller U, Dimitrakopoulou-Strauss A, Haberkorn U. Integrated analysis of dynamic FET PET/CT parameters, histology, and methylation profiling of 44 gliomas. Eur J Nucl Med Mol Imaging 2018;45:1573-84. [Crossref] [PubMed]
- Pyka T, Gempt J, Hiob D, Ringel F, Schlegel J, Bette S, Wester HJ, Meyer B, Förster S. Textural analysis of pre-therapeutic [18F]-FET-PET and its correlation with tumor grade and patient survival in high-grade gliomas. Eur J Nucl Med Mol Imaging 2016;43:133-41. [Crossref] [PubMed]
- Ha S, Park S, Bang JI, Kim EK, Lee HY. Metabolic radiomics for pretreatment 18F‐FDG PET/CT to characterize locally advanced breast cancer: Histopathologic characteristics, response to neoadjuvant chemotherapy, and prognosis. Sci Rep 2017;7:1556. [Crossref] [PubMed]
- Lee JW, Lee SM. Radiomics in Oncological PET/CT: Clinical Applications. Nucl Med Mol Imaging 2018;52:170-89. [Crossref] [PubMed]
- Gong C, Ma G, Hu X, Zhang Y, Wang Z, Zhang J, Zhao Y, Li Y, Xie Y, Yang Z, Wang B. Pretreatment 18F-FDG Uptake Heterogeneity Predicts Treatment Outcome of First-Line Chemotherapy in Patients with Metastatic Triple-Negative Breast Cancer. Oncologist 2018;23:1144-52. [Crossref] [PubMed]
- Unterrainer M, Vettermann F, Brendel M, Holzgreve A, Lifschitz M, Zähringer M, Suchorska B, Wenter V, Illigens BM, Bartenstein P, Albert NL. Towards standardization of (18)F-FET PET imaging: do we need a consistent method of background activity assessment? EJNMMI Res 2017;7:48. [Crossref] [PubMed]
- Pauleit D, Floeth F, Hamacher K, Riemenschneider MJ, Reifenberger G, Müller HW, Zilles K, Coenen HH, Langen KJ. O. -(2-[18F]fluoroethyl)-L-tyrosine PET combined with MRI improves the diagnostic assessment of cerebral gliomas. Brain 2005;128:678-87. [Crossref] [PubMed]
- Tixier F, LeRest CC, Hatt M, Albarghach N, Pradier O, Metges JP, Corcos L, Visvikis D. Intratumor heterogeneity characterized by textural features on baseline 18F‐FDG PET images predicts response to concomitant radiochemotherapy in esophageal cancer. J Nucl Med 2011;52:369-78. [Crossref] [PubMed]
- Lee JW, Choi JS, Lyu J, Lee SM. Prognostic significance of 18F-fluorodeoxyglucose uptake of bone marrow measured on positron emission tomography in patients with small cell lung cancer. Lung Cancer 2018;118:41-7. [Crossref] [PubMed]
- Moon SH, Cho YS, Son YI, Ahn YC, Ahn MJ, Choi JY, Kim BT, Lee KH. Value of 18F-FDG heterogeneity for discerning metastatic from benign lymph nodes in nasopharyngeal carcinoma patients with suspected recurrence. Br J Radiol 2016;89:20160109. [Crossref] [PubMed]
- Shipitsin M, Campbell LL, Argani P, Weremowicz S, Bloushtain-Qimron N, Yao J, Nikolskaya T, Serebryiskaya T, Beroukhim R, Hu M, Halushka MK, Sukumar S, Parker LM, Anderson KS, Harris LN, Garber JE, Richardson AL, Schnitt SJ, Nikolsky Y, Gelman RS, Polyak K. Molecular definition of breast tumor heterogeneity. Cancer Cell 2007;11:259-73. [Crossref] [PubMed]
- Louis DN, Perry A, Reifenberger G, von Deimling A, Figarella-Branger D, Cavenee WK, Ohgaki H, Wiestler OD, Kleihues P, Ellison DW. The 2016 World Health Organization Classification of Tumors of the Central Nervous System: a summary. Acta Neuropathol 2016;131:803-20. [Crossref] [PubMed]
- Ginet M, Zaragori T, Marie PY, Roch V, Gauchotte G, Rech F, Blonski M, Lamiral Z, Taillandier L, Imbert L, Verger A. Integration of dynamic parameters in the analysis of 18 F-FDopa PET imaging improves the prediction of molecular features of gliomas. Eur J Nucl Med Mol Imaging 2020;47:1381-90. [Crossref] [PubMed]
- Molina D, Pérez-Beteta J, Luque B, Arregui E, Calvo M, Borrás JM, López C, Martino J, Velasquez C, Asenjo B, Benavides M, Herruzo I, Martínez-González A, Pérez-Romasanta L, Arana E, Pérez-García VM. Tumour heterogeneity in glioblastoma assessed by MRI texture analysis: a potential marker of survival. Br J Radiol 2016;89:20160242. [Crossref] [PubMed]
- Kickingereder P, Burth S, Wick A, Götz M, Eidel O, Schlemmer HP, Maier-Hein KH, Wick W, Bendszus M, Radbruch A, Bonekamp D. Radiomic profiling of glioblastoma: identifying an imaging predictor of patient survival with improved performance over established clinical and radiologic risk models. Radiology 2016;280:880-9. [Crossref] [PubMed]
- Chaddad A, Daniel P, Desrosiers C, Toews M, Abdulkarim B. Novel radiomic features based on joint intensity matrices for predicting glioblastoma patient survival time. IEEE J Biomed Health Inform 2019;23:795-804. [Crossref] [PubMed]