Live feedback and 3D photoacoustic remote sensing
Introduction
Photoacoustic (PA) imaging techniques represent a family of non-invasive modalities that hold potential for clinical applications such as ophthalmology (1), slide-free histology (2), and dermatology (3), among others (4,5). This is in part due to its ability to provide excellent contrast and specificity without the use of exogenous dyes. PA imaging takes advantage of the large endogenous optical absorption of biomolecules to provide label-free contrast. This optical absorption contrast is also highly specific and provides a means to distinguish between chromophores (6). Fine microscopy PA methods, such as optical-resolution photoacoustic microscopy (OR-PAM), employ a tightly focused laser to target chromophores, such as DNA or hemoglobin (7). The targeted chromophores absorb the pulsed light and undergo rapid expansion due to the thermoelastic effect. This rapid expansion generates broadband ultrasonic pressures local to the absorber. As these pressures propagate through the sample, they can be detected at the surface using an ultrasonic transducer, resulting in time-encoded depth-resolved PA signals (8). Since these depth-resolved signals are localized to the excitation volume, PA microscopy must raster-scan the excitation laser over the sample, effectively forming a map of the optical absorption present within the sample in 2D and 3D. OR-PAM has been efficacious in visualizing microvasculature and tissue morphology with high-resolution and contrast. Effectual image reconstruction, however, requires a large amount of finely space point acquisitions which can be potentially slow and impede real-time imaging. Nevertheless, as PA imaging begins to progress towards clinical adoption, providing live operator feedback becomes essential.
To keep up with the instantaneous optical feedback from conventional light microscopes, PA methods would need to provide high-resolution video-rate live feed to the clinicians. Conventional PA microscopy methods rely on a strong confocal geometry between the excitation beam and the resulting acoustic waves from the target (9). This imposes several constraints in terms of component placement in the light delivery system and generally requires a trade-off between resolution, signal to noise ratio (SNR), field of view (FOV), rate of imaging and the imaging depth. In preceding years, a variety of methods have been developed to increase the rate of acquisition. Xie et al. employed scanning mirrors to raster-scan the laser over the target while keeping the ultrasonic transducer stationary. Despite significant increases in imaging speeds over mechanical scanning, this method suffers from low SNR due to an unfocused transducer which results in a weak confocal arrangement between the optical and acoustic beams (10). Hybrid scanning methods, where the fast axis is scanned optically and the slow axis is scanned using a mechanical stage, have demonstrated fast imaging rates with a linearly focused transducer but result in non-portable systems due to bulky mechanical stages (11,12). Micro-lens arrays have been employed to increase the rate of imaging significantly, but require lasers with a significantly higher pulse energy. These systems may also result in an increased rate of heat deposition over the target, which may not be feasible for clinical settings (13,14). Water immersible two-axis micro-electromechanical system (MEMS) mirrors have been employed to maintain the confocal arrangement between the acoustic and the optical beam and enable rapid imaging for OR-PAM systems with a high SNR (15). MEMS mirrors, nevertheless, suffer from a reduced FOV unless they are operated at their resonant frequencies. In recent years, water immersible hexagonal mirrors have also been employed to increase the rate and area of scanning while maintaining the strong confocal arrangement afforded by MEMS mirrors. However, this method reported a resolution of ~10 µm due to the use of a low NA objective lens (16). In these works, to maintain a confocal opto-acoustic geometry, the scanning optics need to be placed between the objective lens and the target. However, this precludes the use of high NA objective lenses due to their small working distances and leads to low resolution imaging systems. While hexagonal and MEMS mirrors have resulted in systems with improved sensitivity and scanning rates, the imaging speed for conventional PA microscopy is ultimately limited by the rate of acoustic propagation. This is because excitation pulses that have been fired in quick succession can lead to acoustic interference within the sample as the ultrasonic pressures propagate. To avoid this interference, there is typically a minimum interval between consecutive excitation pulses depending on the imaging depth, leading to a trade-off between imaging depth and speed. For example, when imaging a sample with a thickness of 1 mm, there must be a minimum interval of 0.65 µs between consecutive excitation pulses to avoid acoustic interference within the sample. This leads to a maximum laser repetition rate of 1.5 MHz (17). Previously, all-optical 3D PA imaging has been demonstrated with a kilohertz repetition rate laser ultrasound. This technique exhibits excellent contrast and resolution. However, the system design is interferometric and thus susceptible to unwanted vibrations (18). Shintate et al. demonstrate a 660 nm resolution with a transmission-mode OR-PAM system (19). This sub-micron resolution is achieved using a piezo mechanical stage with step sizes as fine as 200 nm. However, piezo mechanical stages are slow and are not suitable for real-time imaging systems.
Photoacoustic remote sensing (PARS™) is an emerging biomedical imaging modality which has experimentally demonstrated sub-micron resolution in a reflection-mode architecture (20). PARS replaces the acoustic transducer with a continuous-wave detection laser (21). This detection laser provides a means to optically probe the initial acoustic pressures local to the absorber. This leads to an all-optical design which relies on an optical confocal geometry between the excitation and the detection laser. This all-optical confocal arrangement is considerably simpler and is free from constraints imposed by an opto-acoustic confocal geometry, leading to a more versatile design that can offer high resolution and SNR simultaneously in a reflection-mode architecture. Unlike conventional PA microscopy, PARS relies on initial pressure measurements for contrast and is not limited by the rate of ultrasonic propagation. Instead, PARS is mainly limited by stress confinement conditions and usually requires short point acquisitions, typically in the range of hundreds of nanoseconds, which do not encode depth information (21,22). This can potentially lead to acquisition rates in the range of tens of megahertz. This rapid rate of imaging can potentially enable video-rate live feedback. Furthermore, this rate of imaging can be combined with adaptive optics to quickly acquire optical sections for the purposes of rapid 3D visualizations (23). These advantages position PARS to be a promising modality to achieve high-resolution reflection-mode video-rate live feedback as well as rapid 3D imaging for clinical applications.
Most of the literature on real-time imaging is focused on improvements in purely the acquisition speed (10-16). The image reconstruction is typically post-reconstruction and thus not live. To this end, taking advantage of the all-optical design, we present a system capable of live real-time imaging and 3D visualizations. Employing an integrated two-axis optical scanning head with a simple interpolation scheme based on nearest neighbors, we demonstrated sustained C-scan rate of up to 2.5 Hz with a 600 kHz pulse repetition rate laser and a FOV of 167 µm × 167 µm. A lateral resolution of 1.2 µm was measured from the live feed which we believe is the highest reported for a live feedback reflection-mode architecture. The system’s performance is demonstrated with carbon fiber phantoms as well as in-vivo imaging. The first results of 3D imaging with PARS are also shown, with an 8.5 µm axial resolution. We benchmark the performance of 3D reconstruction with phantoms and demonstrate its efficacy by visualizing microvasculature structures in 3D in-vivo.
Methods
Optical system
Figure 1 illustrates the experimental apparatus. The optical setup employs a 600 kHz repetition rate 532 nm laser (GLPM-10, IPG Photonics) for excitation. The excitation beam is first expanded and coupled into an optical fiber for spatial filtering. The filtered light from the fiber is collimated and expanded to fill the entrance aperture of the focusing objective (approximately 8 mm). A 1,310 nm continuous-wave superluminescent diode (S5FC1018P, ThorLabs Inc.) is employed as the detection beam. The detection beam is collimated, expanded to approximately 8 mm and passed through a polarized beam splitter (PBS). The PBS transmits the detection beam towards a quarter wave plate (WPQ10M-1310, Thorlabs Inc.) which converts the linearly polarized light into circularly polarized light. A dichroic mirror (DMLP900R, ThorLabs Inc.) is used to combine the detection and excitation beams. This dichroic directs the combined light towards a pair of scanning mirrors (GVS412, Thorlabs Inc.). This combined beam is then focused using an 0.40 NA objective lens (MY20X-824, Mitutoyo Corp.).
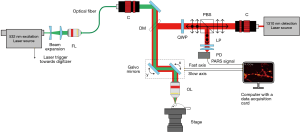
The back-reflected light from the sample is collected using the same objective lens. The dichroic mirror transmits the majority of the back-reflected light towards the quarter-wave plate which converts the circularly polarized light to linearly polarized light. Subsequently, the PBS reflects this linearly polarized light towards the photodiode (PDB425C-AC, Thorlabs Inc.). A long-pass filter (FELH1000, ThorLabs Inc.) is employed to block any 532 nm reflection. The remaining 1,310 nm light is focused using an aspherical lens onto the photodiode.
Image acquisition
Microscopes that rely on point acquisitions require the desired FOV to be well sampled for effectual image reconstruction. To this end, the scanning mirrors are arranged orthogonally and allow the co-focused excitation and detection beam to be steered at any location in the FOV. The mirrors are driven using a ramp waveform from a function generator. The amplitude and frequency of the waveforms determines the FOV and the rate of the mirror swing. The ramp waveforms provide a constant acceleration and allow a consist step size between point acquisitions. The driving frequency of the mirror depends upon the laser repetition rate and the desired number of point acquisitions per frame. For example, when the excitation laser repeats at 600 kHz, the fast axis is driven at 900 Hz and the slow axis at 3.6 Hz.
The laser trigger, photodiode and the mirror feedback signals are connected to a 14-bit four-channel high-speed digitizer (CSE1442, GageApplied) as shown in Figure 2A,B,C,D. PARS signal bandwidth has previously been measured to be around 65 MHz (21). Thus, to ensure signal capture with high fidelity a 75 MHz photodiode was chosen. The digitizer is configured to sample at a rate of 200 million samples per second to accommodate the photodiode bandwidth. To synchronize the collection events, the excitation laser is used as a trigger signal for the digitizer. The scanning mirrors are not triggered or synchronized to any waveform and are instead continuously driven using the function generator. The digitizer is configured to record a brief segment, typically a few hundred nanoseconds, after each trigger event from all three input channels. A few hundred nanosecond segment is collected as longer recording lengths typically provide higher frequency resolution, thus improving the effectiveness of lowpass filtering in the Fourier domain (24). This recording segment is represented by the dashed rectangles on Figure 2B,C,D. These signals are digitized and streamed to the computer memory where they are managed using an in-house developed software. With a sampling rate of 200 million samples per second, this results in an array length of 128 segments for each time domain signal. The digitizer records a set number of time domain signals (A-scans) per frame. This acquisition method is used for real-time imaging as well as for capturing a set of 2D slices at different depths for 3D volumetric reconstruction. Between each 2D slice, the target is moved at a prescribed amount vertically using a mechanical stage.
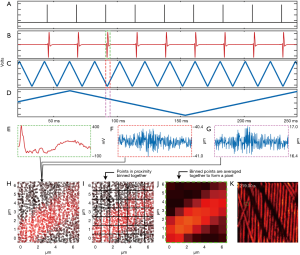
Image formation
Providing a live display requires transferring, processing and interpolating the streamed data in real-time. The digitizer streams the PA signal and the two mirror position signals in the form of a 16-bit interlaced array. This array is deinterlaced to separate the three individual signals into independent arrays. For each point acquisition, the mirror signals (Figure 2F,G) are averaged to extract x,y coordinates. Averaging the temporal waveforms in this way reduces signal noise by the square root of the number of elements averaged. For instance, assuming that the noise is uncorrelated, if the waveform had a length of 128 elements the noise reduction would be eleven times. Note that the mirror averaging operation does not result in a significant loss of accuracy. The mirror signals are sampled at 200 MHz, which is over 200,000 times faster than even the fast axis’ movement. The mirror time-domains effectively represent one value measured several times. The resulting averaged arrays are stored as 64-bit floats to match the native architecture of the imaging computer. The PARS time domain signals are converted to the frequency domain via the Fast Fourier Transform and passed through a 10 MHz 2nd order low pass filter. This filter attenuates the noise from the digitizer, photodiode or the detection source present in the signal. The resulting signal is then transformed back to the time domain. The filtering is implemented in frequency domain to lower the computational cost. The amplitude of each filtered time domain signal is then computed by adding the signal’s maximum and minimum values. This processing results in each point acquisition having an intensity and x,y spatial coordinate specifying where in the FOV it was captured. These points lack a regular grid-like structure and must be interpolated to form an image. Figure 2A shows the scatter data over a small FOV.
The digitizer provides 14-bit analog to digital conversion for each analogue input. With a 5 V input waveform, the least significant bit equates to approximately 300 µV, which is likely far lower than the noise floor of the system. This implies that the mirror positional signals likely do not represent 14-bits precision. Recognizing this, the averaged mirror feedback is converted to a discretized waveform. This process begins by scaling the mirror feedback between 0 and 1 and subsequently multiplying the normalized waveform with the desired width of the image in pixels. Supposing the desired image width is 320 pixels, this would result in a waveform that extends from 0 to 319 as array addressing begins from 0. Each element of this waveform is then rounded off to the nearest integer. In this manner, the pixel size (or bin size) determines the rounding off of the mirror feedback and also sets the image size. This conversion has the effect of binning points that are close together, as illustrated in Figure 2B. For example, coordinates such as (34.3, 10.5) and (34.7, 10.2) are rounded to [10, 34]. This method, therefore, does not require a computationally expensive search to determine a given point’s nearest neighbors, saving considerable execution time. The interpolation begins by declaring a zeroed out 2D array for the final interpolated image. Subsequently, for each coordinate, the corresponding signal strength is looked up from the internal amplitude array and added to the appropriate pixel in the final image. The coordinate values from the discretized positional arrays are used as the indices for the interpolated image. Since the discretization process results in multiple points being binned together, multiple amplitudes are added together in the final image and averaged together to enhance the SNR (Figure 2C,D). This approach makes two basic assumptions about the data acquisition. First, it assumes that the FOV is sampled evenly and there are no regions with significant variation in sample density. Second, it assumes that the pixel size is large enough to contain at least one point acquisition. If a pixel does not contain any measurements, it is never assigned a value and remains at zero. These locations appear as black pixels in the interpolated image. As a final step, the pixels in the image are iterated over and locations which have a value of zero are assigned the average of the surrounding neighbors towards the left, right, top and bottom of the pixel in question.
The same algorithm is utilized for reconstructing each slice in a 3D volume. Once all the levels in a 3D volume have been reconstructed, we render the final 3D volume using ImageJ’s ClearVolume plugin (25).
System characterization
Carbon fibers with a width of approximately 7 µm were imaged to characterize the lateral resolution of the imaging system (Figure 3A). The edge profile was measured across the green a–a’ line and an edge spread function was fitted. The first derivate of this edge spread function is computed resulting in a line spread function. The full width at half maximum (FWHM) of this line spread function is considered as the lateral resolution. The FWHM, along with the edge profile and line spread function are shown in Figure 3B. This revealed a lateral resolution of 1.2 µm, more than sufficient to resolve capillaries and even cell nuclei. Concurrently, the axial resolution was determined to be ~8.5 µm. Figure 3C shows an image of microvasculature structure captured in a mouse ear used to characterize the SNR. The noise is defined as the standard deviation measured in the yellow box in Figure 3C. The peak SNR is computed by dividing the maximum PARS signal by the noise whereas the mean SNR is computed by dividing the PARS signals above a certain threshold by the noise. This yielded a peak SNR of 62 dB and a mean SNR of 44 dB in-vivo. The theoretical dynamic range offered by the digitizer can be calculated as 20·log10(214) which equates to 84 dB. However, the photodiode’s noise equivalent power of 5.2 pW/√Hz limits the overall dynamic range of the system to approximately 59 dB. Compared to the peak SNR of 62 dB, it becomes evident that the filtering and averaging improve the effective SNR of the system significantly.
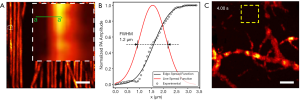
Excitation beam laser safety
The mouse ear has numerous blood vessels 70–100 µm below the skin (26). Assuming a focal spot diameter of 1 µm and the optical focus depth to be 70 µm, the radius of the laser spot at the surface of the mouse ear can be calculated to be ~24 µm. The actual imaging is done at the focal spot of the laser, approximately 70–100 µm below the surface of the ear. During imaging, the pulse energy of the excitation laser was measured to be 45 nJ. For a single pulse, this yields a fluence at the skin of ~2.6 mJ/cm2 which is considerably below the maximum permissible skin exposure limit of 20 mJ/cm2 set by the American National Standards Institute (ANSI) (27). When the target is moved vertically, the pulse energy of the laser is lowered to ensure no damaged is caused to the animal.
Detection beam laser safety
The detection laser was set to a power of 5 mW and areas as large as 500 µm × 500 µm are scanned in this work. This results in an irradiance of 2.4 W/cm2. For a continuous wave laser with a wavelength of 1,310 nm and an exposure time between 100 ns and 10 s, the maximum exposure limit (MPE) on the skin is limited to an energy density of MPE=1.1 CAt0.25, where CA is defined as the corrected factor and is defined as 5 for a wavelength of 1,310 nm (27). The demonstrated in-vivo imaging had an exposure time of 1 s. The average power limit set by ANSI is calculated as MPEavg=MPE⁄t and equates to 5.5 W/cm2. The detection laser is, therefore, operating at half the ANSI limit.
Animal preparation for in-vivo imaging
All animal experiments were approved by the University of Waterloo Anima Care Facility and were carried out in accordance with the University of Waterloo ethics committee. A nude SKH1-Elite Charles River mouse was anesthetized with 5% isoflurane. The anesthesia was maintained with 1% isoflurane during the in-vivo imaging. A heating pad was placed under the mouse to maintain body temperature.
Results
Carbon fibers were used as phantom targets to benchmark the proposed method’s performance. Multiple layers of carbon fibers were placed on top of a glass slide and placed underneath the microscope’s objective. Figure 4A shows select frames from a live video where the fibers are panned and optically sectioned. Carbon fiber shadows from superficial layers can be observed to partially block the image. Such shadows are present as the incidental excitation light is absorbed by carbon fibers placed above the plane of imaging. These shadows can be mitigated by employing a low NA lens for a higher depth of field at the cost of lateral and axial resolution. A total of 200,000 A-scans were captured in a FOV of 167 µm × 167 µm resulting in an average step size of 400 nm. To ensure the A-scans are spread over the FOV, the scanning mirrors are driven at 3.6 and 900 Hz. With a laser repetition rate of 600 kHz, the digitizer collects 200,000 points at a rate of 3 Hz. The slow-axis rate of 3.6 Hz ensures the full coverage of the FOV within each frame including some overlap. The mechanical stages are used to translate the carbon fibers underneath the objective, as shown in the first two frames. The depth stage is then moved to focus onto a second layer of carbon-fibers underneath. With a A-scan count of 200,000 and a pulse repetition rate of 600 kHz, the acquisition time was approximately 300 ms per frame. The image reconstruction takes approximately 100 ms on a 2.7 GHz Intel Core i7 processor resulting in a real-time visualization frame rate of nearly 2.5 Hz in 2D. A sped-up video can be viewed at this link: https://amepc.wistia.com/medias/rf1z7gpayr (Video 1).
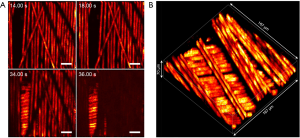
Figure 4B shows the 3D capabilities of the system. By leveraging the optical sectioning properties of the PARS modality, 3D visualizations can be reconstructed from a series of 2D images. The axial resolution is then determined by the Rayleigh range of the excitation spot. Thus, the 3D visualizations provide an axial resolution of ~8.5 µm while maintaining 1.2 µm lateral resolution. To collect volumetric images, the depth stage was utilized to move the sample vertically between 2D acquisitions. A total of 14 2D frames were captured with a vertical step size of 5 µm between them. The 3D view shows the multiple layers of carbon fibers in high fidelity. The FOV was set to 167 µm × 167 µm with a total depth of 70 µm.
The performance of the image reconstruction was investigated by measuring the execution time of all major steps involved. A special benchmarking program was written to consistently measure the image reconstruction process. For the sake of testing consistency, this benchmarking program read raw data from a file rather than processing an incoming data stream. This avoided any potential variables associated with the data acquisition card such as initialization and configuration steps. The file was read once into memory and the execution times of all major processes were measured about one hundred times. The time taken to read the file was not measured as it does not reflect real-world use. These results are summarized in Table 1. The numbers below are a result of running the benchmarking program one hundred times and averaging the result for a 100,000-sample count image. By far the most computationally expensive process is the fast Fourier transform, filtering and the inverse fast Fourier transform. These three steps take up to 74% of the total processing time. The Fourier transform is implemented via the Fastest Fourier Transform In The West library (28). The interpolation by itself takes only 0.7% of the total computation time.
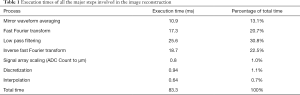
Full table
The proposed method’s efficacy is demonstrated with in-vivo imaging of mouse ears. Figure 5 shows select snapshots from a live video of microvasculature structure within a mouse ear. The figure illustrates the microvasculature coming into focus as different planes within the ear are visualized. The FOV is then panned using the 2D mechanical stages to visualize different regions in the mouse ear. The FOV was set to 183 µm × 183 µm and the average step size was 800 nm. To improve the margin of safety within the mouse ear we reduced the tissues fluence exposure to less than half of the ANSI limit. We decreased the laser repetition rate to 50 kHz and acquired 50,000 A-scans per frame to achieve a real-time visualization frame rate of approximately 1 Hz. With these scan parameters delivered fluence is 2.6 mJ/cm2 while the maximum permissible exposure MPE according to ANSI standards was ~5.7 mJ/cm2. We emphasize that these results are from a live video and not from a post-acquisition reconstruction. A sped up live video, located at the following address https://amepc.wistia.com/medias/mwhctzqlz7, shows in-vivo microvascular structure from a mouse ear (Video 2).
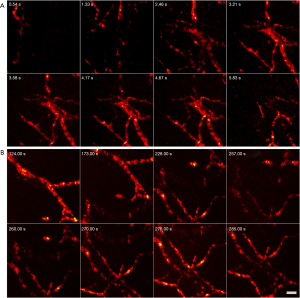
Figure 6 further demonstrates PARS’s ability to capture 3D volumes. Two different locations on a mouse ear were captured in-vivo. These results represent the first 3D images captured with a non-contact label-free reflection-mode method. Similar to the 3D phantom image, the depth stage was utilized to move the mouse in a perpendicular direction. A total of 17 frames were acquired with an axial step size of 3.5 µm. This captured a total depth of 60 µm, providing 1.2 µm lateral resolution and ~8.5 µm axial resolution. The total FOV was set to 500 µm × 500 µm for both 3D volumes. A rotating volume is provided at the following web address https://amepc.wistia.com/medias/v98sgjwey0. This video illustrates the 3D capabilities of the system (Video 3).
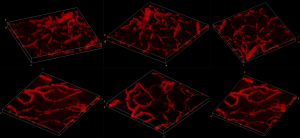
Discussion
The proposed method demonstrates several benefits over previous reports of real-time PA imaging systems (1). Analogous to OR-PAM’s requirement of a confocal geometry between the excitation and the resulting acoustic waves. PARS is reliant on a strong confocal arrangement between the excitation and the detection beam. In general, achieving con-focal arrangement between two lasers is significantly simpler than aligning an acoustic beam with a laser. Managing acoustic beams requires specialized components such as water-immersible scanning optics or opto-acoustic splitters between the target and the objective lens. In contrast, optical con-focal arrangement is simpler since multiple laser beams can be combined into a single light delivery path using a dichroic beam combiner. The combined light can then be optically scanned and subsequently focused using an objective lens. This advantage allows PARS to employ high NA objective lenses to achieve a lateral resolution of 1.2 µm, the highest reported by a real-time reflection-mode PA imaging system (2). A standard x-y galvanometer scanner steers the optical beams simultaneously and maintains a strong confocal geometry between the two leading to a system with appropriate sensitivity for recovering blood vessels in vivo. This allows real-time imaging with a mean and peak SNR of 44 and 62 dB, respectively, with a laser fluence of 10 mJ/cm2. The SNR measurements exceed previous reports of PARS systems with comparable values for laser fluence (3). Employing a computationally inexpensive reconstruction technique that bins points in proximity and averages the amplitudes, we demonstrate a live video feed that enhances the high SNR afforded by the optical system and sustains frame rates up to 2.5 Hz in 2D.
As with the vast majority of real-time point by point scanning microscopes, there exists a trade-off between sampling density, FOV and the resolution. For example, captures a FOV of 50×50 µm2 with a 400 nm step size (19). Assuming we sample around 25,000 A-scans, the proposed method in this study can acquire a 50×50 µm2 in approximately 40 ms or at a C-scan rate of 25 Hz. Similarly, demonstrates a 1.5×1.5 mm2 FOV (29). Assuming a step size of 2 µm, the number of A-scans required would be 560,000. With a repetition rate of 600 kHz, the proposed method would take approximately 900 ms to acquire. Achieving true live feedback imaging at such FOVs is limited by the laser repetition rate and computational cost of processing half a million A-scans. These limitations can be bypassed if a higher repetition rate laser is used and the A-scan processing is partially transferred to a GPU.
We also demonstrate PARS’s ability to capture 3D volumes in-vivo. These results represent the first reports of 3D imaging with PARS. Presently, these volumes are captured by acquiring multiple 2D layers at various depths by moving the surface of the sample perpendicular to the optical axis using a mechanical stage. This process can be sped up considerably by using a laser with a faster repetition rate and employing adaptive optics to move the focal spot instead of moving the sample (23). For example, it would take approximately 25 ms to acquire 50,000 A-scans per layer with a 2 MHz repetition rate laser. Assuming a total of 14 slices are required, the total acquisition time would be 350 ms. This can enable high-speed 3D visualizations, potentially in real-time. Alternatively, architectures like coherence-gated PARS can be explored to provide depth-resolved PA signals for potentially even faster 3D imaging with higher axial resolution (30).
To render live video, the proposed method relies on a computationally lightweight interpolation scheme. In most nearest neighbor based interpolation schemes, the process of searching for a given point’s neighbors dominates the interpolation time. To this end, several data structures have been explored to speed up a nearest neighbor search. The proposed method is able to find a given point’s nearest neighbors by rounding off the positional arrays. This is effectual because the positional information of each A-scan likely does not have 14-bits of precision due to a small amount of measurement noise associated with each digitization. High-SNR interpolation is realized by averaging the binned scatter points. The key advantage of this approach is that allows the positional arrays to be treated as indexing arrays for the final image. This enables direct access of the pixel locations in constant time [time complexity of O(1)] (31) rather than a computationally expensive nearest neighbor search. This results in rapid image reconstruction from the scatter data with high SNR and contrast suitable for a high-quality live video stream. The most computationally expensive task in the image reconstruction is the low pass filtering which, typically, accounts for 40% of the total image reconstruction time. However, in the future, this step, along with other parallelizable code, can be executed on a graphics processing unit which can potentially reduce the execution time even further.
The overall rendering time for the live video is a sum of the acquisition time and the image reconstruction time. Since the digitizer is configured to stream the acquisitions directly to system memory, the time taken to transfer the data is effectively zero. This makes the acquisition time entirely dependent on the laser repetition rate and the number of A-scans desired per frame. The image reconstruction takes approximately 100 ms per 100,000 A-scans to fully render a scene, and is usually not the limiting factor in terms of frame rate. Instead, it was found that the primary limiting factor for the frame rate and the FOV were the scanning mirrors. The mirrors have a maximum swing rate of 1 kHz for angles smaller than 0.2°. This angle translates to approximately 120 µm × 120 µm in terms of FOV with the current objective lens. As mentioned, the phantom studies captured 200,000 A-scans per frame with a repetition rate of 600 kHz. The mirrors were driven at 3.6 and 900 Hz, which is close to their maximum specification. Although the shorter acquisition and reconstruction times can be realized by reducing the point count per frame, the mirror swing rate would need to be increased as well. For example, from our in-vivo results, it can be observed that even 50,000 A-scans per frame provide remarkable contrast and resolution, resolving capillaries as small as 6 µm. However, capturing 50,000 A-scans per frame at a repetition rate of 600 kHz would require fast axis to swing at a rate 3.6 kHz, which is far higher than their specification. Future work can aim to increase the imaging frame rate by using faster scanning optics, optimizing scanning patterns and employing higher pulse repetition rate lasers.
Conclusions
In summary, we presented a PA method capable of high-resolution reflection-mode imaging in real-time. Employing this method, we demonstrated real-time imaging in-vivo and the first reports of 3D imaging with PARS. A lateral resolution of 1.2 µm and axial resolution of 8.5 µm was extracted from the live video feed, which is the highest lateral resolution reported for a real-time PA imaging system. The all-optical confocal geometry avoids the trade-offs from opto-acoustic geometries and enables a high-resolution system while simultaneously maintaining C-scan rates as high as 2.5 Hz with up to 62 dB peak SNR. Moreover, the non-contact label-free reflection-mode architecture lends itself to clinical applications, such as ophthalmologic imaging and surgical procedures. The authors believe this work represents a vital step towards a video-rate real-time imaging system that can provide high-resolution absorption contrast in a reflection-mode architecture, increasing the clinical accessibility of PA techniques.
Acknowledgments
The authors gratefully acknowledge funding from Natural Sciences and Engineering Research Council of Canada, Canada Foundation for Innovation, Mitacs Accelerate, University of Waterloo Startup funds, Centre for Bioengineering and Biotechnology, illumiSonics Inc and New frontiers in research fund – exploration.
Footnote
Provenance and Peer Review: With the arrangement by the Guest Editors and the editorial office, this article has been reviewed by external peers.
Conflicts of Interest: All authors have completed the ICMJE uniform disclosure form (available at http://dx.doi.org/10.21037/qims-20-758). The special issue “Advanced Optical Imaging in Biomedicine” was commissioned by the editorial office without any funding or sponsorship. SA reports grants from illumiSonics Inc., grants from NSERC, grants from Mitacs, grants from New frontiers in research, grants from CFI, during the conduct of the study. KB reports grants, personal fees, non-financial support and other from illumiSonics Inc., grants from NSERC, grants from Mitacs, grants from New frontiers in research, grants from CFI, during the conduct of the study; other from illumiSonics Inc., outside the submitted work. In addition, KB has a patent US10117583B2 issued, a patent US20190320908A1 issued, a patent US20180275046A1 issued, a patent WO2019145764A1 pending, and a patent I am not willing to disclose my other pending and planned IPs until they are publicly available pending. BE reports grants from illumiSonics Inc., grants from NSERC, grants from Mitacs, grants from New frontiers in research, grants from CFI, during the conduct of the study. PHR reports grants, personal fees, non-financial support and other from illumiSonics Inc., grants from NSERC, grants from Mitacs, grants from New frontiers in research, grants from CFI, during the conduct of the study; in addition, PHR has a patent US10117583B2 issued, a patent US20190320908A1 issued, a patent US20180275046A1 issued, a patent WO2019145764A1 pending, and a patent I am not willing to disclose my other pending and planned IPs until they are publicly available pending. The authors have no other conflicts of interest to declare.
Open Access Statement: This is an Open Access article distributed in accordance with the Creative Commons Attribution-NonCommercial-NoDerivs 4.0 International License (CC BY-NC-ND 4.0), which permits the non-commercial replication and distribution of the article with the strict proviso that no changes or edits are made and the original work is properly cited (including links to both the formal publication through the relevant DOI and the license). See: https://creativecommons.org/licenses/by-nc-nd/4.0/.
References
- Jiao S, Jiang M, Hu J, Fawzi A, Zhou Q, Shung KK, Puliafito CA, Zhang HF. Photoacoustic ophthalmoscopy for in vivo retinal imaging. Opt Express 2010;18:3967-72. [Crossref] [PubMed]
- Abbasi S, Le M, Sonier B, Dinakaran D, Bigras G, Bell K, Mackey JR, Reza PH. All-optical Reflection-mode Microscopic Histology of Unstained Human Tissues. Sci Rep 2019;9:13392. [Crossref] [PubMed]
- Zhou Y, Xing W, Maslov KI, Cornelius LA, Wang LV. Handheld photoacoustic microscopy to detect melanoma depth in vivo. Opt Lett 2014;39:4731-4. [Crossref] [PubMed]
- Zhang C, Cheng YJ, Chen J, Wickline S, Wang LV. Label-free photoacoustic microscopy of myocardial sheet architecture. J Biomed Opt 2012;17:060506. [Crossref] [PubMed]
- Hu S, Wang LV. Neurovascular photoacoustic tomography. Front Neuroenergetics 2010;2:10. [PubMed]
- Beard P. Biomedical photoacoustic imaging. Interface Focus 2011;1:602-31. [Crossref] [PubMed]
- Yao DK, Chen R, Maslov K, Zhou Q, Wang LV. Optimal ultraviolet wavelength for in vivo photoacoustic imaging of cell nuclei. J Biomed Opt 2012;17:056004. [Crossref] [PubMed]
- Wang LV, Hu S. Photoacoustic Tomography: In Vivo Imaging from Organelles to Organs. Science 2012;335:1458-62. [Crossref] [PubMed]
- Maslov K, Zhang HF, Hu S, Wang LV. Optical-resolution photoacoustic microscopy for in vivo imaging of single capillaries. Opt Lett 2008;33:929-31. [Crossref] [PubMed]
- Xie Z, Jiao S, Zhang HF, Puliafito CA. Laser-scanning optical-resolution photoacoustic microscopy. Opt Lett 2009;34:1771-3. [Crossref] [PubMed]
- Rao B, Li L, Maslov K, Wang L. Hybrid-scanning optical-resolution photoacoustic microscopy for in vivo vasculature imaging. Opt Lett 2010;35:1521-3. [Crossref] [PubMed]
- Yao J, Wang L, Yang JM, Gao LS, Maslov KI, Wang LV, Huang CH, Zou J. Wide-field fast-scanning photoacoustic microscopy based on a water-immersible MEMS scanning mirror. J Biomed Opt 2012;17:080505. [Crossref] [PubMed]
- Song L, Maslov K, Wang LV. Section-illumination photoacoustic microscopy for dynamic 3D imaging of microcirculation in vivo. Opt Lett 2010;35:1482-4. [Crossref] [PubMed]
- Song L, Maslov K, Wang LV. Multi-focal optical-resolution photoacoustic microscopy in vivo. Opt Lett 2011;36:1236-8. [Crossref] [PubMed]
- Kim JY, Lee C, Park K, Lim G, Kim C. Fast optical-resolution photoacoustic microscopy using a 2-axis water-proofing MEMS scanner. Sci Rep 2015;5:7932. [Crossref] [PubMed]
- Lan B, Liu W, Wang Y, Shi J, Li Y, Xu S, Sheng H, Zhou Q, Zou J, Hoffmann U, Yang W, Yao J. High-speed widefield photoacoustic microscopy of small-animal hemodynamics. Biomed Opt Express 2018;9:4689-701. [Crossref] [PubMed]
- Yao J, Wang LV. Photoacoustic microscopy. Laser Photon Rev 2013;7:758-78. [Crossref] [PubMed]
- Pelivanov I, Ambroziński Ł, Khomenko A, Koricho EG, Cloud GL, Haq M, O’Donnell M. High resolution imaging of impacted CFRP composites with a fiber-optic laser-ultrasound scanner. Photoacoustics 2016;4:55-64. [Crossref] [PubMed]
- Shintate R, Morino T, Kawaguchi K, Nagaoka R, Kobayashi K, Kanzaki M, Saijo Y. Development of optical resolution photoacoustic microscopy with sub-micron lateral resolution for visualization of cells and their structures. Jpn J Appl Phys 2020;59:SKKE11.
- Reza PH, Bell K, Shi W, Shapiro J, Zemp RJ. Deep non-contact photoacoustic initial pressure imaging. Optica 2018;5:814-20. [Crossref]
- Hajireza P, Shi W, Bell K, Paproski RJ, Zemp RJ. Non-interferometric photoacoustic remote sensing microscopy. Light Sci Appl 2017;6:e16278. [Crossref] [PubMed]
- Bell KL, Hajireza P, Shi W, Zemp RJ. Temporal evolution of low-coherence reflectrometry signals in photoacoustic remote sensing microscopy. Appl Opt 2017;56:5172-81. [Crossref] [PubMed]
- Kner P, Sedat JW, Agard DA, Kam Z. High-resolution wide-field microscopy with adaptive optics for spherical aberration correction and motionless focusing. J Microsc 2010;237:136-47. [Crossref] [PubMed]
- Devasahayam SR. Signals and Systems in Biomedical Engineering: Physiological Systems Modeling and Signal Processing. Berlin: Springer Science & Business Media, 2000:366.
- Royer LA, Weigert M, Günther U, Maghelli N, Jug F, Sbalzarini IF, Myers EW. ClearVolume: open-source live 3D visualization for light-sheet microscopy. Nat Methods 2015;12:480-1. [Crossref] [PubMed]
- Pavone FS, So PTC, French PMW. Microscopy Applied to Biophotonics. Amsterdam: IOS Press, 2014:219.
- Laser Institute of America. ANSI Z136.1-2007 American National Standard for Safe Use of Lasers. ANSI. 2007.
- Frigo M, Johnson SG. The Design and Implementation of FFTW3. Proc IEEE 2005;93:216-31. [Crossref]
- Pleitez MA, Khan AA, Soldà A, Chmyrov A, Reber J, Gasparin F, Seeger MR, Schätz B, Herzig S, Scheideler M, Ntziachristos V. Label-free metabolic imaging by mid-infrared optoacoustic microscopy in living cells. Nat Biotechnol 2020;38:293-6. [Crossref] [PubMed]
- Bell KL, Hajireza P, Zemp RJ. Coherence-gated photoacoustic remote sensing microscopy. Opt Express 2018;26:23689-704. [Crossref] [PubMed]
- Sipser M. Introduction to the Theory of Computation. Thomson Course Technology. Available online: https://notendur.hi.is/mae46/Haskolinn/5.%20misseri%20-%20Haust%202018/Formleg%20ma%CC%81l%20og%20reiknanleiki/Introduction%20to%20the%20theory%20of%20computation_third%20edition%20-%20Michael%20Sipser.pdf