3D Monte Carlo simulation of light distribution in mouse brain in quantitative photoacoustic computed tomography
Introduction
Small animal neuroimaging has gained increasing interest in the past decades. This appeal is motivated by the progress in the transgenic manipulation of small animals, especially mice, as models of human brain diseases and pathological conditions. As mouse models share many genes, physiological processes, and disease loci with humans, investigation of these models helps improve the understanding, prevention, diagnosis, and treatment of human diseases. A variety of imaging modalities have been applied for small animal brain imaging, including magnetic resonance microscopy (MRM), positron emission tomography (PET), single-photon emission computed tomography (SPECT), ultrasound (US) imaging, and multiphoton microscopy. Using these imaging modalities, great advancements have been made in understanding brain function and neurological pathologies, yet major technical challenges remain unsolved.
MRM, or high-resolution magnetic imaging (MRI), can visualize neuroanatomical structures at resolutions <100 µm in at least one dimension. Yet tradeoffs exist between imaging time and spatial resolution, and long scanning times are required for better signal-to-noise ratio (SNR) (1). PET relies on radioactive tracer molecules and provides quantitative measure in living organisms, including regional cerebral glucose utilization, oxygen metabolism, and cerebral blood flow. However, the application of small animal PET is limited by its low availability, high ionizing exposure, relatively high cost, and low spatial resolution (2). Like PET, SPECT is a nuclear imaging technique, and can reach a resolution of 0.35 mm, perform longitudinal studies, and distinguish among multiple radioisotopes (3). However, SPECT also suffers from the potential high ionizing exposure. US imaging is nonionizing and derives contrast from the mechanical properties of the tissue. US imaging can provide tissue morphology and map blood flow with resolution as high as 48 µm in rodent brain with the aid of microbubbles. However, US imaging cannot provide information about the brain’s neuroactivities so far. Multiphoton microscopy [two-photon (2PM) or three-photon microscopy], which provides micron-scale resolution and readily reveals brain cortical organization and function, is the most widely used optical technology for in vivo mouse brain imaging, at depths up to 1.5 mm using contrast agents that are bright/biocompatible and absorbing at longer wavelengths (4). Optical coherence tomography (OCT) has also been explored for mouse brain imaging, although it lacks the cellular and molecular specificity (5). However, both multiphoton microscopy and OCT lack the penetration depth to study the mouse brain beyond the cortical layer.
In the past several decades, photoacoustic (PA) imaging has gradually attracted attention for rodent brain imaging. PA imaging is a nonionizing hybrid imaging modality that physically combines light and sound. In typical PA imaging, short laser pulse illuminates the target, and the incident photons are subsequently absorbed by the chromophores in the target. The absorption leads to a transient increase in temperature and induces a local pressure rise, resulting in the emission of broadband ultrasonic waves (6). Hemoglobin is one of the most commonly used chromophores in PA imaging as its two forms, oxygenated and deoxygenated, have strong optical absorption in the visible and near-infrared (NIR) spectral range. With multispectral PA imaging, functional information such as blood oxygenation can also be estimated. Depending upon the configuration of optical illumination and US detection, PA imaging can be further classified into two major types: PA microscopy (PAM) and PA computed tomography (PACT). While PAM relies on tightly or weakly focused light for excitation and uses a single element focused ultrasonic transducer for direct image formation. It provides high spatial resolution in ballistic and quasidiffusive regimes to image rodent cortex (7-11). Cao et al. built a head-restrained PAM that allows awake-animal imaging and provides metabolic parameters including oxygen extraction fraction and the cerebral metabolic rate of oxygen (12). Recently developed wearable optical-resolution PAM features high spatiotemporal resolutions with a large FOV, allowing investigation of brain activities of free-moving rodents (13). PACT relies on homogenized widefield illumination and the photons can reach the diffusive regime. The PA signals are acquired with an ultrasonic transducer array, and the images are formed with an inverse algorithm (8,14). PACT is highly compatible with commercially available US imaging systems (15-21), and has been widely used in rodent whole brain imaging as it allows monitoring of the brain hemodynamics with balanced spatiotemporal resolution and field of view. Based on the estimated hemoglobin concentration and change in blood oxygenation, neuronal activity can be studied through the neurovascular coupling. Single-wavelength PACT has been used to characterize the resting-state functional connectivity in the mouse brain (22). Hemodynamic change in somatosensory cortex during electrical paw stimulation was observed with multispectral PACT (23). In addition, previous studies have shown PACT’s feasibility in studying neurological diseases. Zhang et al. used a linear array PACT system and observed epileptic wave spreading in both hemispheres (24). Gottschalk et al. correlated the volumetric blood oxygenation with the concurrent electroencephalogram recording in real-time, using a PACT system with a spherical matrix array (25). Ni et al. studied the cortical oxygen perfusion and metabolic rate of oxygen in an arcAβ mouse model of Alzheimer’s disease (26). Furthermore, PACT has been used to detect, track, and characterize tumor cells in the mouse brain (27,28). With the development of calcium indicators and voltage sensors, direct PA visualization of neuronal activities become feasible. For example, hydrophobic anions dipicrylamine has been used for PA imaging of resting potential change (29). Fast brain activation in response to stimulus have been monitored by PAT ex vivo and in vivo on GCaMP mice (30,31).
Most of the functional information provided by PACT, such as blood oxygenation, heavily relies on the estimation of the chromophore concentration. Based on the imaging formation mechanism, the amplitude of the initial PA wave pressure mainly depends on three parameters: the absorption coefficient of the chromophore, the local optical fluence, and the Grüneisen parameter (6,32). In most in vivo cases, the PA signal amplitude is assumed to be directly proportional to the chromophore concentration and does not consider the optical fluence heterogeneity across the region of interest. However, as the brain is a strongly scattering organ and functional regions possess different optical properties, the PA signal strength could reflect different combinations of local optical fluence and chromophore concentrations. Different methods have been reported in order to overcome this limitation and improve the quantitative fidelity of the reconstructed PA images, including adaptive filtered back-projection and model-based reconstruction with fluence distribution estimation (33,34). However, these methods have difficulty for in vivo use, especially in mouse brain tissues that have dense structures.
Therefore, to better understand the impact of optical fluence heterogeneity in mouse brain on the PA imaging and potentially correct it, we propose to apply Monte Carlo (MC) method to simulate the light propagation and resultant optical fluence distribution inside the mouse brain. The MC method constructs a stochastic model to determine the expected light propagation and is the gold standard for studying fluence distribution in biological tissue. It is relatively easy to implement and widely accepted as an accurate method by which to simulate light propagation in tissues (35-37). The MC method has been utilized in PA imaging for simulating imaging depth at different wavelengths, optimizing experimental setup for maximum and homogenous light delivery, and studying the optical fluence distribution inside human infant brain (38-41). So far, the light propagation and fluence distribution inside mouse brain remains to be studied for quantitative PACT. In this paper, we have investigated the optical fluence distribution inside the mouse brain while taking blood vessels into account, and its subsequent impact on the reconstructed PA images.
Methods
Digital mouse brain model
As blood vessels are highly absorbing and scattering compared to other brain tissues, even though they make up only ~5% of the total brain volume, their impact on optical fluence distribution cannot be omitted in in vivo experiments. A hybrid model was thus constructed with blood vessels identified separately from other brain tissues. Due to the lack of a model that identifies both anatomical structure and blood vessel distribution, a hybrid mouse brain model with vasculature identified was created using two published data sets: the Digimouse atlas developed by Dogdas et al. and light sheet fluorescent microscopy (LSFM) images of mouse-brain vasculature acquired by Di Giovanna et al. (Figure 1A) (42,43). Both data sets are volumetric. The Digimouse atlas identifies mouse brain functional regions, such as olfactory bulbs and external cerebrum, as well as anatomical structures, such as skin and skull, all of which are included in the tetrahedral mesh for simulation (Figure 1B). LSFM mouse-brain vasculature images provide the blood vessel population density in different parts of the mouse brain. The hybrid brain model with vasculature identified (Digimouse-vasculature atlas, in short) was created by manually registering the LSFM mouse-brain vasculature images with Digimouse atlas through resizing, shifting, and thresholding (Figure 1C,D,E,F).
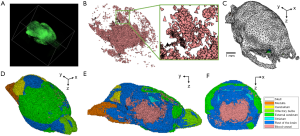
3D MC modeling software
MMCLAB, the native MEX version of mesh-based Monte Carlo (MMC) photon simulation software for MATLAB, was used for our simulation (44,45). Different from existing MC software designed for layered or voxel-based media, MMC can represent a complex domain using a volumetric mesh. It can utilize a tetrahedral mesh to model a complex anatomical structure and has been shown to be more accurate and computationally efficient than the conventional MC methods (46,47). In MMC, anatomical structures in the model are identified and assigned with optical properties, including the scattering coefficient (µs), absorption coefficient (µa), anisotropy (g), and refractive index (n). The model is then converted to a tetrahedral mesh with the iso2mesh MATLAB toolbox. The maximum voxel volume is 0.001 mm3 (48). Other configurations, including light source type, location, illumination direction, and number of photons, are predefined before the simulation. The MMC software then simulates photon propagation from the predefined light source through the volume consisted of tetrahedral voxels, and outputs optical fluence distribution normalized to the initial optical energy launched into the simulation volume.
3D MC model construction
In this work, to study the potential effect of blood vasculature on optical fluence distribution in mouse brain, we performed 3D MC simulations on mouse brain models with and without integrated blood vasculature. Both the Digimouse and Digimouse-vasculature atlas were converted into tetrahedral mesh using the iso2mesh toolbox (48). To validate the hybrid Digimouse-vasculature mesh, we estimated the cerebral blood volume (CBV) percentage by calculating the element number ratio between blood vessels and other brain tissues (49). The estimated CBV ratio was 5.9%, with relatively high blood vessel population density in cortex, hypothalamus, and thalamus. Though the two data sets are from two different sources and cannot be perfectly registered, the overall blood vessel population density within the hybrid model is consistent with the reported literature values (49).
3D MC modeling parameters
In PACT of mouse brains, a linear array transducer is commonly used due to its wide availability and high compatibility with commercial US scanners. Figure 2A shows the PACT setup used in our simulations, which images the sagittal planes of the mouse brain. Due to the relatively small skull curvature, imaging the sagittal plane allows better skull aberration correction. The linear array transducer such as L22-14v (Verasonics Inc., Kirkland, WA), is placed on top of the mouse head with coupling gel to image the sagittal plane (Figure 2B). A linear illumination pattern is chosen because it can provide relatively homogenous optical fluence at the imaging plane. Such illumination is achieved either by mounting optical fiber bundles on both sides of the transducer array or by guiding a free-space linear light beam directly onto the sample surface. In our simulation, the linear light sources were placed outside the model, and each source provided a uniform fluence at the scalp surface with an area of 2×6 mm2. The distance between the light incident position and the center of the transducer imaging plane was 3 mm (Figure 2A). As suggested by Sowers et al., the illumination angle was set to 45-deg to achieve highest fluence at the imaging plane (39). The transducer and light sources were immersed in water as the background medium. We assume the background medium was non-scattering and non-absorbing and had no refractive index mismatch with the mouse model.

NIR light is preferred for deep brain imaging due to the relatively low optical scattering from tissues and low optical absorption from water. Using hemoglobin as the endogenous contrast, NIR light can provide relatively deep penetration (6,7). Here, we chose 700 and 1,064 nm as the excitation wavelengths to maximize the absorption difference between oxygenated and deoxygenated hemoglobin. Both wavelengths are commonly available in PAT. In our simulations, two different meshes were simulated at 700 and 1,064 nm using the optical parameters listed in Table 1.
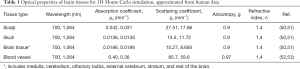
Full table
Although further studies are required, Hoshi et al. have suggested that the optical properties of rodent and human tissues are comparable (54). Because the optical properties of mouse brain tissue at 700 and 1,064 nm are not available, we used the optical properties of human tissues based on the work by Jacques et al. (50). The main tissue types in the hybrid mouse model include scalp, skull, eye, brain (with several functional regions), muscles, glands, and blood vessels (50). The optical properties of glands were approximated to those of muscles. Since the lipid makes up 40% of the brain’s dry weight, the absorption coefficient of the brain tissue was approximated to that of lipid (55). As it is computationally challenging to assign each individual blood vessel element with different optical properties, we assumed an average hematocrit of 45% and an average blood oxygenation (sO2) of 85% (52,56,57). Here, we need to note that the reported optical properties of the brain tissues in the literatures might include the contributions from the blood vessels, depending on the preparation of the specimen for the optical measurement. Nevertheless, we consider the contribution of blood vessels, especially on the µs, to be negligible as the average CBV ratio in the brain is only 5% (49).
k-Wave modeling parameters
Quantitative PA imaging relies on accurate estimation of optical fluence inside the tissue, but most of the PA imaging reconstruction algorithms assume constant fluence distribution; therefore, we used the k-Wave toolbox to investigate the effect of inhomogeneous illumination on the reconstructed images and chromophore concentration estimation (58). With the same experimental setup as shown in Figure 2A, a 350×640 k-Wave grid was created to cover a region of 7×12.8 mm2. A group of blood vessels were used to simulate the deep brain vasculature, and the initial PA pressure was simulated based on the fluence distribution obtained from the 3D MC simulation results. As absorption coefficient of the hemoglobin is at least one magnitude higher than those of other tissues at both wavelengths, PA signals generated from other tissues are negligible. Therefore, in our k-Wave simulation, initial pressure was only assigned to blood vessels. The US detector used in k-Wave was L22-14v (Verasonics Inc., Kirkland, WA), with 128 channels and a 0.1 mm pitch. The simulated PA signals were first band-pass filtered (12.3–24.7 MHz) to assimilate the experimental US transducer with a limited detection bandwidth, and then used for image reconstruction with a time-reversal based method (58,59). The other brain tissues were assumed to be acoustically homogenous, with constant tissue density and zero acoustic attenuation.
Results
Overall 3D optical fluence distribution in mouse brain
The simulated optical fluence maps of several coronal and sagittal planes at selected locations are shown in Figures 3-6, respectively, from models with and without blood vessels. As expected, due to the strong optical scattering of brain tissues, the light is largely diffused after propagating just a few millimeters for either wavelength. In the imaging plane (x=6 mm), the optical fluence with 1,064 nm illumination remains relatively homogenous within the cortex region (1–2 mm deep) and decreases by a factor of 5 at 5 mm depth. Similar fluence pattern is observed at 700 nm, except faster decay and lower fluence at deep region (3–4 mm from scalp surface) due to stronger light attenuation. Outside the imaging plane, the optical fluence is much stronger near the incident location and decreases by a factor of 10 within 2–3 mm propagation for either wavelength. The high optical fluence outside the imaging plane can generate strong out-of-plane signals and result in image artifacts, yet currently there are very few alternative illumination schemes available for linear array transducers.
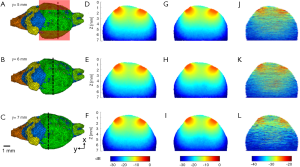
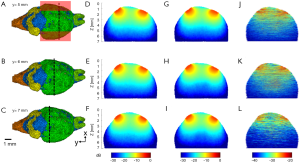
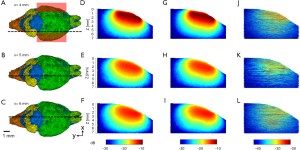
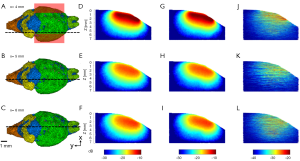
The influence of blood vessels on optical fluence distribution
The simulation results show that blood vessels have clear influence on the optical fluence distribution. Macroscopically, the optical fluence maps are similar in MC models with and without incorporating blood vessels as shown in Figures 3-6 (D,E,F,G,H,I), but the existence of blood vessels clearly changes the local optical fluence at most of the brain regions [Figures 3-6 (J,K,L)]. We analyzed the optical fluence as a function of depth at six locations, y=4, 5, 6, 7, 8, 9 mm, along the sagittal suture (x=6 mm) (Figures 7,8). At each depth of the selected location, the total optical fluence within a 0.2×0.2 mm2 area was plotted. The optical fluence remained relatively flat for the first 1–2 mm beneath the scalp surface, which is mainly the cortex layer, regardless of the existence of blood vessels. However, the optical fluence in the cortex is significantly lower with the blood vessels. Such difference gradually diminishes in the deeper brain beyond 4 mm. As most of the blood vessel elements are concentrated in the cortex, hypothalamus, and thalamus, the strong optical attenuation of blood vessels reduces the local optical fluence. Since the cortex is closer to the illumination location and thus has a higher optical fluence, the fluence reduction is more pronounced than other blood-rich regions. In addition, even in deep brain, where the blood vessel population becomes denser in the model, less light is available and thus the effect of the blood vessels on the fluence is less obvious.
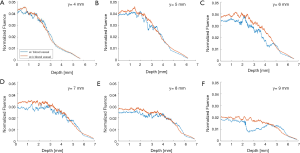
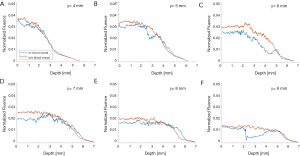
Although the optical fluence distributions on the global level are similar with and without blood vessels, the blood vessels introduce clear local fluctuations in the fluence distribution. The local fluctuations are expected to be more pronounced for actual in vivo applications, as the structural and functional conditions are much more complex. Both the global decay and local fluctuation in optical fluence contribute to the heterogeneity of the fluence distribution in PA imaging. Consequently, such heterogeneous fluence distribution causes errors in the quantification of the chromophore concentration. Most of the conventional PA reconstruction algorithms, such as the back-projection based method, do not take the fluence heterogeneity into account, resulting in low quantitative accuracy (60).
The influence of heterogeneous optical fluence on quantitative PACT
The fluence distribution inside the tissue is wavelength dependent, a common phenomenon in multispectral PA imaging, also known as spectral coloring. Targets in the deeper and peripheral regions receive lower optical fluence and thus generate weaker PA signals, and thus the homogenous fluence assumption for image reconstruction is no longer valid. The impact of heterogeneous optical fluence on quantitative PA image reconstruction is shown in Figure 9. Using k-Wave, we simulated PA signal generation with heterogeneous fluence at 700 and 1,064 nm. A 4×4 mm2 region (y-axis ranges from 2.8 to 6.8 mm, z-axis ranges from 2 to 6 mm, as shown in Figure 9A) was extracted at the sagittal plane x=6 mm. The initial PA pressure was normalized by the fluence map, assuming a homogenous Grüneisen parameter (Figure 9B). The acquired PA signals were bandpass filtered and reconstructed by using a time-reversal based method (Figure 9E,F). Without fluence correction, the recovered µa of the deep targets was underestimated in the reconstructed image. For fluence correction, the reconstructed images were normalized to the fluence map. The corrected images can better recover the actual µa of the deep targets (Figure 9G,H), despite the missing vertical structures due to the limited-view detection and increased noise floor at deep region (61,62). The oxygenation of hemoglobin (sO2) was also calculated based on the reconstructed images with and without fluence correction (Figure 9I,J). As expected, without fluence correction, the estimated blood oxygenation became less accurate at larger depth. With fluence correction, the blood oxygenation estimation has much improved accuracy.
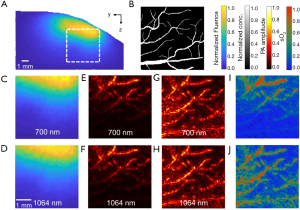
Conclusions
With its balanced resolution and penetration depth and the inherent functional sensitivity, PACT is of high interest to the large neuroscience community. We have previous studied the skull’s impact on the acoustic wave propagation in PACT of mouse brain (63). In this paper, we investigate the impact of optical fluence distribution in mouse brain on quantitative PACT. Though the simulation setup is based on a linear-array PACT system, the results can be generalized and translated to other PACT configurations. Our results show that, mouse brain is a highly scattering organ, and the global decay of the optical fluence with depth from the brain surface is non-negligible, regardless of the existence of blood vessels. The optical fluence decreases by a factor of 4–5 over the entire field of view with illumination from one side. The existence of blood vessels introduces additional local fluctuations in optical fluence, due to optical property variation within the tissue structure. Both the global decay and the local fluctuations can lead to inaccurate estimation of chromophore concentrations in quantitative PACT. Correcting the optical fluence distribution can improve the quantitative accuracy of PACT.
Our 3D MC simulation accuracy depends on the digital mouse brain model, which was generated by combining the anatomical information and vascular information from two different data sets. It is technically challenging to register two data sets accurately. In this study, the two data sets were registered manually by mapping their functional regions to the maximum extent while ensuring that the final CBV ratio was consistent with the literature (~5%). To improve the model’s accuracy, regional CBV ratios should also be considered. For example, as shown by Chugh et al., cerebral cortex, hypothalamus, and hippocampus have a total CBV ratio of 7.9%, 4.5%, and 3.7%, respectively (49). The regional differences in both CBV ratio and vasculature pattern are non-negligible. An adaptive registration should be developed to better match different regions of the brain and further improve the accuracy of the combined mouse brain model.
Though our mouse brain model included different functional regions, we used the optical properties extracted from human brain data, due to the lack of published values for moue brains at 1,064 nm. Several studies have shown that the optical properties (especially µs) vary for different functional regions of the mouse brain, mainly due to different composition of cell types (64-66). Even though the differences might be insignificant, more accurate optical properties can further improve our mouse brain model’s accuracy.
We used k-Wave simulation to demonstrate the impact of the global decay and local fluctuations in optical fluence on the reconstructed PA images. However, such simulation results are oversimplified. We used only 2d k-Wave simulation and did not consider out-of-plane signals or acoustic attenuation/reverberation. We did not consider the skull’s impact either. Liang et al. have shown that the skull causes strong aberrations in high-frequency transcranial PA signals, leading to inaccurate target location and deteriorated PACT image quality (63). Therefore, for in vivo applications, we expect that the optical fluence heterogeneity is much more complex, and its impact on quantitative PACT is more significant (63).
Acknowledgments
The authors thank Caroline Connor for editing the manuscript.
Funding: This work was supported in part by National Institute of Health (R01 EB028143, R01 NS111039, R01 NS115581, R01 GM134036, R21 EB027304, R21 EB027981, R43 CA243822, R43 CA239830, R44 HL138185); Duke MEDx Basic Science Grant; Duke Center for Genomic and Computational Biology Faculty Research Grant; Duke Institute of Brain Science Incubator Award; and American Heart Association Collaborative Sciences Award (18CSA34080277).
Footnote
Provenance and Peer Review: With the arrangement by the Guest Editors and the editorial office, this article has been reviewed by external peers.
Conflicts of Interest: Both authors have completed the ICMJE uniform disclosure form (available at http://dx.doi.org/10.21037/qims-20-815). JY served as the unpaid Guest Editor of the special issue and serves as an unpaid editorial board member of Quantitative Imaging in Medicine and Surgery. The authors have no other conflicts of interest to declare.
Ethical Statement: This article does not contain any studies involving animals or human performed by any of the authors.
Open Access Statement: This is an Open Access article distributed in accordance with the Creative Commons Attribution-NonCommercial-NoDerivs 4.0 International License (CC BY-NC-ND 4.0), which permits the non-commercial replication and distribution of the article with the strict proviso that no changes or edits are made and the original work is properly cited (including links to both the formal publication through the relevant DOI and the license). See: https://creativecommons.org/licenses/by-nc-nd/4.0/.
References
- Benveniste H, Blackband S. MR microscopy and high resolution small animal MRI: applications in neuroscience research. Prog Neurobiol 2002;67:393-420. [Crossref] [PubMed]
- Martín A, Ramos-Cabrer P, Planas AM. Noninvasive Brain Imaging in Small Animal Stroke Models: MRI, PET, and SPECT. In: Dirnagl U. editor. Rodent Models of Stroke. New York, NY: Springer, 2016:147-86.
- Golestani R, Wu C, Tio RA, Zeebregts CJ, Petrov AD, Beekman FJ, Dierckx RAJO, Boersma HH, Slart RHJA. Small-animal SPECT and SPECT/CT: application in cardiovascular research. Eur J Nucl Med Mol Imaging 2010;37:1766-77. [Crossref] [PubMed]
- Miller DR, Jarrett JW, Hassan AM, Dunn AK. Deep tissue imaging with multiphoton fluorescence microscopy. Current Opinion in Biomedical Engineering 2017;4:32-9. [Crossref] [PubMed]
- Chong SP, Merkle CW, Cooke DF, Zhang T, Radhakrishnan H, Krubitzer L, Srinivasan VJ. Noninvasive, in vivo imaging of subcortical mouse brain regions with 1.7 µm optical coherence tomography. Opt Lett 2015;40:4911-4. [Crossref] [PubMed]
- Beard P. Biomedical photoacoustic imaging. Interface Focus 2011;1:602-31. [Crossref] [PubMed]
- Xia J, Yao J, Wang LV. Photoacoustic tomography: principles and advances. Electromagn Waves (Camb) 2014;147:1-22. [Crossref] [PubMed]
- Wang LV. Multiscale photoacoustic microscopy and computed tomography. Nat Photonics 2009;3:503-9. [Crossref] [PubMed]
- Hu S, Yan P, Maslov K, Lee JM, Wang LV. Intravital imaging of amyloid plaques in a transgenic mouse model using optical-resolution photoacoustic microscopy. Opt Lett 2009;34:3899-901. [Crossref] [PubMed]
- Yao J, Wang L, Yang JM, Maslov KI, Wong TTW, Li L, Huang CH, Zou J, Wang LV. High-speed label-free functional photoacoustic microscopy of mouse brain in action. Nature Methods 2015;12:407-10. [Crossref] [PubMed]
- Hu S, Maslov KI, Tsytsarev V, Wang LV. Functional transcranial brain imaging by optical-resolution photoacoustic microscopy. J Biomed Opt 2009;14:040503. [Crossref] [PubMed]
- Cao R, Li J, Ning B, Sun N, Wang T, Zuo Z, Hu S. Functional and Oxygen-metabolic Photoacoustic Microscopy of the Awake Mouse Brain. Neuroimage 2017;150:77-87. [Crossref] [PubMed]
- Chen Q, Xie H, Xi L. Wearable optical resolution photoacoustic microscopy. Journal of Biophotonics 2019;12:e201900066. [Crossref] [PubMed]
- Biomedical Optics: Principles and Imaging | Optics & Photonics | Physics & Astronomy | Subjects | Wiley n.d.
- Kolkman RGM, Brands PJ, Steenbergen W, Leeuwen TGC, van . Real-time in vivo photoacoustic and ultrasound imaging. J Biomed Opt 2008;13:050510. [Crossref] [PubMed]
- Homan K, Shah J, Gomez S, Gensler H, Karpiouk A, Brannon-Peppas L, Emelianov S. Combined ultrasound and photoacoustic imaging of pancreatic cancer using nanocage contrast agents. Photons Plus Ultrasound: Imaging and Sensing 2009, vol. 7177, International Society for Optics and Photonics; 2009, p. 71771M.
- Daoudi K, Berg PJ, van den , Rabot O, Kohl A, Tisserand S, Brands P, Steenbergen W. Handheld probe integrating laser diode and ultrasound transducer array for ultrasound/photoacoustic dual modality imaging. Opt Express 2014;22:26365-74. [Crossref] [PubMed]
- Kang J, Chang JH, Kim SM, Lee HJ, Kim H, Wilson BC, Song TK. Real-time sentinel lymph node biopsy guidance using combined ultrasound, photoacoustic, fluorescence imaging: in vivo proof-of-principle and validation with nodal obstruction. Sci Rep 2017;7:45008. [Crossref] [PubMed]
- Erpelding TN, Kim C, Pramanik M, Jankovic L, Maslov K, Guo Z, Margenthaler JA, Pashley MD, Wang LV. Sentinel Lymph Nodes in the Rat: Noninvasive Photoacoustic and US Imaging with a Clinical US System. Radiology 2010;256:102-10. [Crossref] [PubMed]
- Karpiouk AB, Aglyamov SR, Mallidi S, Shah J, Scott WG, Rubin JM, Emelianov SY. Combined ultrasound and photoacoustic imaging to detect and stage deep vein thrombosis: phantom and ex vivo studies. J Biomed Opt 2008;13:054061. [Crossref] [PubMed]
- Mehrmohammadi M, Yoon SJ, Yeager D, Emelianov SY. Photoacoustic Imaging for Cancer Detection and Staging. Curr Mol Imaging 2013;2:89-105. [Crossref] [PubMed]
- Nasiriavanaki M, Xia J, Wan H, Bauer AQ, Culver JP, Wang LV. High-resolution photoacoustic tomography of resting-state functional connectivity in the mouse brain. PNAS 2014;111:21-6. [Crossref] [PubMed]
- Mc Larney B, Hutter MA, Degtyaruk O, Deán-Ben XL, Razansky D. Monitoring of Stimulus Evoked Murine Somatosensory Cortex Hemodynamic Activity With Volumetric Multi-Spectral Optoacoustic Tomography. Front Neurosci 2020;14:536. [Crossref] [PubMed]
- Zhang P, Li L, Lin L, Hu P, Shi J, He Y, Zhu L, Zhou Y, Wang LV. High-resolution deep functional imaging of the whole mouse brain by photoacoustic computed tomography in vivo. Journal of Biophotonics 2018;11:e201700024. [Crossref] [PubMed]
- Gottschalk S, Fehm TF, Deán-Ben XL, Tsytsarev V, Razansky D. Correlation between volumetric oxygenation responses and electrophysiology identifies deep thalamocortical activity during epileptic seizures. Neurophotonics 2017;4:011007. [Crossref] [PubMed]
- Ni R, Rudin M, Klohs J. Cortical hypoperfusion and reduced cerebral metabolic rate of oxygen in the arcAβ mouse model of Alzheimer’s disease. Photoacoustics 2018;10:38-47. [Crossref] [PubMed]
- Burton NC, Patel M, Morscher S, Driessen WHP, Claussen J, Beziere N, Jetzfellner T, Taruttis A, Razansky D, Bednar B, Ntziachristos V. Multispectral Opto-acoustic Tomography (MSOT) of the Brain and Glioblastoma Characterization. NeuroImage 2013;65:522-8. [Crossref] [PubMed]
- Li L, Zhu L, Ma C, Lin L, Yao J, Wang L, Maslov K, Zhang R, Chen W, Shi J, Wang LV. Single-impulse panoramic photoacoustic computed tomography of small-animal whole-body dynamics at high spatiotemporal resolution. Nat Biomed Eng 2017;1:1-11. [Crossref] [PubMed]
- Rao B, Zhang R, Li L, Shao JY, Wang LV. Photoacoustic imaging of voltage responses beyond the optical diffusion limit. Sci Rep 2017;7:2560. [Crossref] [PubMed]
- Gottschalk S, Degtyaruk O, Larney BM, Rebling J, Hutter MA, Deán-Ben XL, Shoham S, Razansky D. Rapid volumetric optoacoustic imaging of neural dynamics across the mouse brain. Nat Biomed Eng 2019;3:392-401. [Crossref] [PubMed]
- Deán-Ben XL, Sela G, Lauri A, Kneipp M, Ntziachristos V, Westmeyer GG, Shoham S, Razansky D. Functional optoacoustic neuro-tomography for scalable whole-brain monitoring of calcium indicators. Light Sci Appl 2016;5:e16201. [Crossref] [PubMed]
- Xia J, Wang LV. Small-Animal Whole-Body Photoacoustic Tomography: A Review. IEEE Trans Biomed Eng 2014;61:1380-9. [Crossref] [PubMed]
- Huang H, Bustamante G, Peterson R, Ye JY. An adaptive filtered back-projection for photoacoustic image reconstruction. Med Phys 2015;42:2169-78. [Crossref] [PubMed]
- Rosenthal A, Razansky D, Ntziachristos V. Quantitative Optoacoustic Signal Extraction Using Sparse Signal Representation. IEEE Trans Med Imaging 2009;28:1997-2006. [Crossref] [PubMed]
- Ren N, Liang J, Qu X, Li J, Lu B, Tian J. GPU-based Monte Carlo simulation for light propagation in complex heterogeneous tissues. Opt Express 2010;18:6811-23. [Crossref] [PubMed]
- Hasegawa Y, Yamada Y, Tamura M, Nomura Y. Monte Carlo simulation of light transmission through living tissues. Appl Opt 1991;30:4515-20. [Crossref] [PubMed]
- Periyasamy V, Pramanik M. Advances in Monte Carlo Simulation for Light Propagation in Tissue. IEEE Rev Biomed Eng 2017;10:122-35. [Crossref] [PubMed]
- Sharma A, Srishti S, Periyasamy V, Pramanik M. Monte Carlo simulations and photoacoustic experiments to compare imaging depth at 532 nm, 800 nm, and 1064 nm. Photons Plus Ultrasound: Imaging and Sensing 2020, vol. 11240, International Society for Optics and Photonics; 2020, p. 1124043.
- Sowers T, Yoon H, Emelianov S. Investigation of light delivery geometries for photoacoustic applications using Monte Carlo simulations with multiple wavelengths, tissue types, and species characteristics. J Biomed Opt 2020;25:1-16. [Crossref] [PubMed]
- Periyasamy V, Pramanik M. Monte Carlo simulation of light transport in tissue for optimizing light delivery in photoacoustic imaging of the sentinel lymph node. J Biomed Opt 2013;18:106008. [Crossref] [PubMed]
- Mahmoodkalayeh S, Lu X, Ansari MA, Li H, Nasiriavanaki M. Optimization of light illumination for photoacoustic computed tomography of human infant brain. Photons Plus Ultrasound: Imaging and Sensing 2018, vol. 10494, International Society for Optics and Photonics; 2018, p. 104946U.
- Dogdas B, Stout D, Chatziioannou AF, Leahy RM. Digimouse: a 3D whole body mouse atlas from CT and cryosection data. Phys Med Biol 2007;52:577-87. [Crossref] [PubMed]
- Di Giovanna AP. Mouse-brain vasculature, dorsal side. Downscaled 2018.
- Fang Q, Boas DA. Monte Carlo Simulation of Photon Migration in 3D Turbid Media Accelerated by Graphics Processing Units. Opt Express 2009;17:20178-90. [Crossref] [PubMed]
- Yu L, Nina-Paravecino F, Kaeli DR, Fang Q. Scalable and massively parallel Monte Carlo photon transport simulations for heterogeneous computing platforms. J Biomed Opt 2018;23:1-4. [Crossref] [PubMed]
- Fang Q. Mesh-based Monte Carlo method using fast ray-tracing in Plücker coordinates. Biomed Opt Express 2010;1:165. [Crossref] [PubMed]
- Fang Q, Kaeli DR. Accelerating mesh-based Monte Carlo method on modern CPU architectures. Biomed Opt Express 2012;3:3223-30. [Crossref] [PubMed]
- Fang Q, Boas DA. Tetrahedral mesh generation from volumetric binary and gray-scale images. Proceedings of the Sixth IEEE international conference on Symposium on Biomedical Imaging: From Nano to Macro, Boston, Massachusetts, USA: IEEE Press; 2009:1142-5.
- Chugh BP, Lerch JP, Yu LX, Pienkowski M, Harrison RV, Henkelman RM, Sled JG. Measurement of cerebral blood volume in mouse brain regions using micro-computed tomography. Neuro Image 2009;47:1312-8. [Crossref] [PubMed]
- Jacques SL. Optical properties of biological tissues: a review. Phys Med Biol 2013;58:R37. [Crossref] [PubMed]
- Cheong WF, Prahl SA, Welch AJ. A review of the optical properties of biological tissues. IEEE Journal of Quantum Electronics 1990;26:2166-85. [Crossref]
- Bosschaart N, Edelman GJ, Aalders MCG, Leeuwen TG, van , Faber DJ. A literature review and novel theoretical approach on the optical properties of whole blood. Lasers Med Sci 2014;29:453. [Crossref] [PubMed]
- Friebel M, Roggan A, Müller GJ, Meinke MC. Determination of optical properties of human blood in the spectral range 250 to 1100 nm using Monte Carlo simulations with hematocrit-dependent effective scattering phase functions. J Biomed Opt 2006;11:34021. [Crossref] [PubMed]
- Hoshi Y, Tanikawa Y, Okada E, Kawaguchi H, Nemoto M, Shimizu K, Kodama T, Watanabe M. In situ estimation of optical properties of rat and monkey brains using femtosecond time-resolved measurements. Sci Rep 2019;9:9165. [Crossref] [PubMed]
- Dawson G. Measuring Brain Lipids. Biochim Biophys Acta 2015;1851:1026-39. [Crossref] [PubMed]
- Harrop GA. The oxygen and carbon dioxide content of arterial and of venous blood in normal individuals and in patients with anemia and heart disease. J Exp Med 1919;30:241-57. [Crossref] [PubMed]
- Classification & Structure of Blood Vessels | SEER Training n.d.
- Treeby BE, Cox BT. k-wave: MATLAB toolbox for the simulation and reconstruction of photoacoustic wave fields. J Biomed Opt 2010;15:021314. [Crossref] [PubMed]
- Xu M, Wang LV. Universal back-projection algorithm for photoacoustic computed tomography. Phys Rev E Stat Nonlin Soft Matter Phys 2005;71:016706. [Crossref] [PubMed]
- Cox B, Laufer JG, Arridge SR, Beard PC. Quantitative spectroscopic photoacoustic imaging: a review. J Biomed Opt 2012;17:061202. [Crossref] [PubMed]
- Vu T, Li M, Humayun H, Zhou Y, Yao J. A generative adversarial network for artifact removal in photoacoustic computed tomography with a linear-array transducer. Exp Biol Med (Maywood) 2020;245:597-605. [Crossref] [PubMed]
- Deán-Ben XL, Razansky D. On the link between the speckle free nature of optoacoustics and visibility of structures in limited-view tomography. Photoacoustics 2016;4:133-40. [Crossref] [PubMed]
- Liang B, Liu W, Zhan Q, Li M, Zhuang M, Liu QH, Yao J. Impacts of the murine skull on high-frequency transcranial photoacoustic brain imaging. Journal of Biophotonics 2019;12:e201800466. [Crossref] [PubMed]
- Al-Juboori SI, Dondzillo A, Stubblefield EA, Felsen G, Lei TC, Klug A. Light Scattering Properties Vary across Different Regions of the Adult Mouse Brain. PLoS One 2013;8:e67626. [Crossref] [PubMed]
- Azimipour M, Baumgartner R, Liu Y, Jacques SL, Eliceiri KW, Pashaie R. Extraction of optical properties and prediction of light distribution in rat brain tissue. J Biomed Opt 2014;19:75001. [Crossref] [PubMed]
- Keller D, Erö C, Markram H. Cell Densities in the Mouse Brain: A Systematic Review. Front Neuroanat 2018;12:83. [Crossref] [PubMed]