Virtually structured detection enables super-resolution ophthalmoscopy of rod and cone photoreceptors in human retina
Introduction
In vivo visualization of retinal abnormalities is important for eye disease study and diagnosis. However, optical resolution of in vivo retinal imaging has been limited due to the relatively low numerical aperture, ocular aberration, and involuntary movements of the human eye. Structured illumination microscopy (SIM) has been established to break the diffraction-limit by shifting high-frequency signal of the sample into the passing band of the imaging system (1-3). However, a direct implementation of SIM for in vivo retinal imaging is difficult. Traditional SIM requires reliable illumination pattern with accurate phase control, which is challenging for imaging moving samples, such as the retina due to inevitable eye movements.
By providing an alternative way to SIM, virtually structured detection (VSD) was firstly demonstrated for resolution improvement in in vitro microscopy of the retina (4-6). Without the system complexity of SIM required for precise pattern and phase controls, the VSD based approach provides an easy, low-cost, and phase-artifact-free strategy to achieve super-resolution imaging. Moreover, digital masks are applied to modulate the two-dimensional (2D) light profiles in VSD processing. Therefore, the direct-current (DC) component, which affects noise level and then effective dynamic range in traditional SIM, of the image spectrum can be suppressed due to the sinusoidal intensity modulation without DC offset in VSD processing (4,7). This merit further supports VSD as a good candidate for in vivo retinal imaging, considering the inherent weak light reflectance from the retina.
VSD has been recently demonstrated for resolution improvement in in vivo imaging of anesthetized animals (8,9). However, practical implementation of VSD for in vivo retinal imaging of awake human is still challenging, due to involuntary eye movements and uncertain cut-off frequency, which is essential for VSD processing, of ocular optics of each subject (10,11). In principle, digital image registration can be applied to raw images to compensate for the effect of eye movements, before the VSD processing for resolution improvement. Therefore, VSD promises a potential to improve the resolution of in vivo retinal imaging. Accurate assessment of the cut-off frequency, which defines the frequency shift for VSD processing, of ocular optics is required. It is known that an inadequate amount of frequency shift will result in a poor resolution improvement, due to less high-frequency components being shifted into the passing band of the imaging system; while excessive frequency shift will introduce artifacts to degrade the image quality. For our preliminary study with anesthetized frogs, which are known to have relatively low aberration, empirical frequency shift was adopted for VSD processing (8,9). However, optical property of human subjects can be significantly variable among different subjects, making the selection of a proper frequency shift difficult. In this study, we developed a method to retrieve the modulation transfer function (MTF) of the imaging system to enable an objective identification of the cut-off frequency of ocular optics and validated it for in vivo retinal imaging of awake human subjects.
Methods
Figure 1 illustrates the experimental setup, i.e., a line-scan scanning laser ophthalmoscopy (SLO) system. As shown in Figure 1, the compact line-scan SLO can fit onto a stage with dimension of ~46 cm × 46 cm (Figure 1A). The line-scan strategy was selected to provide high imaging speed to minimize the effect of eye movements on retinal imaging. A near-infrared superluminescent diode (SLD-35-HP, Superlum), with 830 nm central wavelength and 60 nm bandwidth was used as the light source. The line illumination was achieved by using a cylindrical lens (CL, Figure 1B) to condense the illumination light in one dimension. The galvo scanning mirror (GVS011, Thorlabs) was placed at the conjugate plane of the pupil to minimize the vignetting effect. The reflected light from the retina with line-illumination was de-scanned by the scanning mirror and recorded by a high-speed CMOS camera (FastCam Mini Ax50, Photron) as a 2D line-profile pattern (Figure 1B). The line profile of the illumination was along the X-direction and the scanning was performed in the Y-direction. The 2D line-profile patterns were acquired at a speed of 25,000 Hz to minimize the intra-frame blur correlated with eye movements. The system possesses a theoretical pixel resolution of 0.85 µm at the retina and can achieve a super-resolution imaging speed of 40 fps with a frame resolution of 500×512 pixels, considering a 0.8 duty ratio.
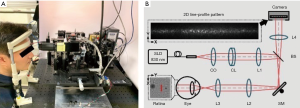
Three human subjects, without known ocular diseases, were recruited for this study with informed consents. The imaging experiment was conducted in a room with minimized ambient light. The pupil size was measured as 4–5 mm, without pharmacological pupil dilation. A dim red fixation target was used to minimize voluntary eye movements. For retinal imaging, the illumination power at cornea was ~2.5 mW, which is within exposure safety limit defined by the American National Standards Institute (ANSI) (12). The illumination light beam has a width of ~2.75 mm at the surface of the cornea to optimize the image focusing of the retina (13).
Before VSD processing, digital registration of sequential line-profile patterns was implemented to compensate for inter-frame shift correlated with eye movements. Basic principle of VSD processing has been reported in previous publications (4,7). To achieve robust resolution improvement in VSD processing, we developed an objective method to estimate the cut-off frequency. The reflected optical signal from each illuminated line area of the retina is recorded by a 2D camera as a line-profile pattern (512×64 pixels, Figure 1B). Each recording signal can be expressed as:
[1]
where Ilp(x,y) is the line-profile pattern recorded by the camera; (x,y) indicates the spatial domain; hil(x,y) and hde(x,y) are the point spread functions (PSF) of the illumination and detection paths, respectively; s(x,y) represents the reflectivity of the sample, i.e., the retina; ⊗ represents convolution.
For VSD processing, a recorded line-profile pattern will first be digitally modulated by a sinusoidal mask (4,6):
[2]
where Imlp(x,y) is the line-profile pattern after intensity modulation by the mask m(x,y). m(x,y) has an expression:
[3]
where fm is the modulation frequency. For the line-scan modality, the modulation is performed along the scanning direction, i.e., Y-direction. Thus θ = 90°, and
[4]
Each Imlp(x,y) is then spatially integrated in the Y-direction and assigned to a corresponding position yi. The output picture p(xi,yi) can be then expressed as (4,6):
[5]
The Fourier transform of Eq. [5] is:
[6]
where (fx,fy) indicates the Fourier domain and
[7]
Therefore,
[8]
To get
[9]
and
[10]
[11]
Taking the case of
[12]
As fm is the modulation frequency of the digital mask, it can be assigned with different values, such as f1..fi. Figure 2A1 illustrates three representative spectra of
[13]
If we further assume the optical transfer functions (OTF) of illumination and detection path are same:
[14]
where
By identifying the value
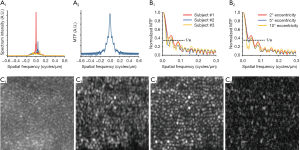
Results
As shown in Figure 2B, the MTF profiles exhibit significant differences between subjects and retinal locations. To balance the resolution improvement and noise artifacts, the frequency where MTF attenuates to 1/e of its maximum was selected as the cut-off frequency for VSD processing. To validate this method, comparative EWF and VSD based super-resolution images were reconstructed from the same datasets acquired at ~2° eccentricity (Figure 2C). The super-resolution images were processed with three different frequency shift values. The corresponding MTF profile is shown as the red curve in Figure 2A,B, and the frequency corresponds to 1/e attenuation is 0.06 cycles/µm. Figure 2, C2,C3,C4 show super-resolution images reconstructed with frequency shift values of 0.02, 0.06, 0.14 cycles/µm, to demonstrate the effect of insufficient, proper, and excessive frequency shift, respectively. By using the frequency shift specified by the proposed method, the reconstructed super-resolution image (Figure 2, C3) presents significantly better image quality compared to the images reconstructed with the other two frequencies (Figure 2, C2,C4). Individual photoreceptors can be clearly observed in Figure 2, C3.
Figure 3A,B show the representative equivalent wide-field (EWF) image and the super-resolution image acquired at ~3° eccentricity from fovea with a frame rate of 40 fps. Both EWF and super-resolution image were reconstructed from the same dataset. The EWF image was obtained by sequentially superimposing the registered line-profile patterns. The super-resolution image was reconstructed with the frequency shift specified by the corresponding MTF profile. As shown in Figure 3A,B, the super-resolution image provides enhanced resolution, compared to the EWF image, to reveal individual photoreceptors. Moreover, the image contrast is also significantly improved in the super-resolution image (0.88), compared to that in the EWF image (0.32). Given the improved resolution and contrast in the super-resolution image, the projection of micro-capillary structures can be observed (yellow arrowheads in Figure 3, B1.
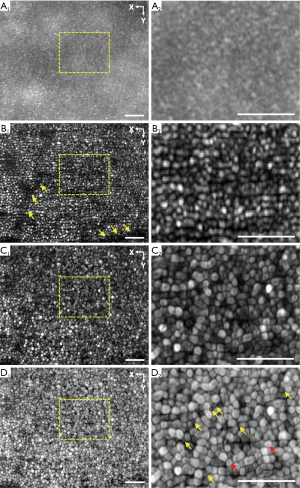
Motility processing was further applied to the super-resolution images of retinal photoreceptors. Dynamic change of photoreceptor reflectance has been observed previously (14,15). This motility property provides additional contrast of photoreceptors and is used to further enhance the image quality in this study. A 2-second sequence of super-resolution images (80 frames) were obtained from the same retinal location as shown in Figure 3A,B. A combination of strip registration (16,17) and affine registration (ImageJ, NIH) was performed to register the time-lapse super-resolution images (Video 1). A 2D standard deviation map was then generated for the registered super-resolution image sequence and was used to reflect the dynamic reflectance change of photoreceptors (Figure 3C). As shown in Figure 3C, the photoreceptors exhibit clearer boundaries and more integrated shape, compared to that in the super-resolution image (Figure 3B). The image contrast in Figure 3C is further improved to 0.92. By using the logarithmic scale, the motility images of photoreceptors in Figure 3D show that rod photoreceptors can be observed at ~3° eccentricity from fovea.
Figure 4 shows montaged EWF (Figure 4A), super-resolution (Figure 4B), and motility (Figure 4C) images to cover an enlarged field of view. Each subpanel was acquired at a frame rate of 40 fps. The location of the fovea is indicated by the white asterisks. Consistent with the results shown in Figure 3, photoreceptor mosaics cannot be well resolved in the EWF images. However, super-resolution images revealed individual cone photoreceptors up to ~1° eccentricity from fovea. The super-resolution image also exhibits improvement in contrast, compared to the EWF image. Specifically, the average contrast of the EWF image is 0.31. In comparison, the super-resolution image has an average contrast of 0.86. Due to the quality improvement in super-resolution image, the projection of micro-capillary structures, which cannot be observed in the EWF image, are further confirmed in the super-resolution image (yellow arrowheads in Figure 4B. As shown in Figure 4C, clearer pattern of photoreceptor mosaics is presented by the motility processing and the motility image possesses an improved contrast of 0.90, compared to the super-resolution image.
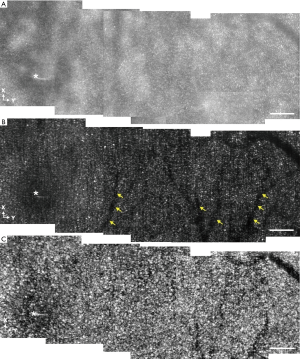
Discussion
In summary, VSD has been demonstrated for resolution improvement of in vivo imaging of retinal photoreceptors in awake human. In order to minimize the effect of the involuntary eye movements on inter-frame blur, a line-scan modality was adopted to provide ultra-high speed, i.e., 25,000 Hz, for rapid acquisition of 2D line-profile sequence required for VSD based super-resolution image reconstruction. Before the VSD processing, digital image registration was implemented for further compensation of the effect of eye movements on intra-frame shifts among sequential line-profile patterns. For practical implementation of VSD in human retinal imaging, the method developed to retrieve system MTF facilitated an objective estimation of the optimal frequency shift for the VSD processing.
The VSD based super-resolution images exhibited significantly improved image quality, in the aspects of resolution and contrast, compared to that of the EWF images. Individual cone photoreceptors from ~1° eccentricity from fovea were clearly resolved (Figure 4). It is known that rod photoreceptors have smaller diameter and weaker reflectance, compared with cone photoreceptors in human retina (18-20). It is usually difficult to image rod photoreceptors in vivo. In conjunction with the image quality improvements brought by the VSD and motility processing, logarithmic scale display enabled unambiguous observation of rod photoreceptors in the super-resolution image (Figure 3D2). Moreover, the shape of cone photoreceptors revealed in the motility image are closer to the actual pentagonal/hexagonal pattern of the cone photoreceptors (21-23). Motility processing also revealed sub-cellular dynamics in individual cone photoreceptors (specified by the red arrow heads in Figure 3D2. The dark areas exhibit in some photoreceptors but are absent in some other photoreceptors, indicating they may not be artifacts caused by registration. We speculate that the sub-cellular dynamics may reflect motility of cell membrane, disc, or waveguide property of retinal photoreceptors. Further investigation is required to understand the mechanism of the subcellular motility properties, which may provide new biomarkers of functional assessment of retinal photoreceptors. It is also noticed that the motility processing provided a better performance at the parafoveal area compared with the foveal region. The reason is that the motility processing relies on the performance of registration. As photoreceptors can be well resolved at the parafovea area, it allows a precise registration between time-lapse images and produces high-quality motility image. However, as the photoreceptors at the foveal area cannot be well resolved in the super-resolution images, the corresponding motility processing may suffer from mismatch or translocation between registered images. Therefore, better registration algorithm and improved image resolution will benefit the performance of motility processing at the foveal region. Additionally, as line-illumination is used in the current system, the resolution improvement is anisotropic, i.e., along the scanning direction. Using multi-orientation scan modality can achieve an isotropic improvement in the resolution and improve the performance of VSD and motility processing (5).
The visible edges in Figure 4A are caused by the non-uniform illumination between different recordings. The ocular position changes relative to the illumination light position for sequential view field recordings, and thus the illumination intensity may vary for a specific retinal location in different images. Moreover, at different view fields, actual magnification of the ocular optics may also have slight changes due to possible eye movement and accommodation. In principle, further data processing can be involved to balance the image. The visibility of the edges is reduced in Figure 4B,C, suggesting that the VSD processing effectively suppressed the direct-current noises. The mismatches at the edge area are caused by the slight difference in magnification, which is also related with the ocular position changes among recordings.
As a proof-of-concept study, the system was constructed with all commercially available components. We anticipate that further optimization of the system, such as customized optical design, can further improve the imaging performance and promise a cost-effective method to foster clinical deployments of high-resolution ophthalmoscopy. Moreover, adaptive optics (AO) has been well established to compensate for the aberration of ocular optics to achieve diffraction-limit resolution (24,25). We are currently pursuing the feasibility of combining the VSD and AO for super-resolution ophthalmoscopy, i.e., breaking the diffraction-limit, which can further improve the imaging resolution to advance retinal study and eye disease management.
Conclusions
In conjunction with rapid line-scan imaging and digital registration to minimize the effect of eye movements, VSD enabled resolution improvement for unambiguous observation of individual retinal photoreceptors without the involvement of AO. An objective method has been developed to derive MTF from digital image profiles to enable quantitative estimation of the cut-off frequency required for robust VSD processing. Dynamic motility processing further enhanced the image contrast of individual photoreceptors, allowing reliable identification of rod and cone photoreceptors. We anticipate that further development of the VSD based super-resolution SLO can provide an easy, low-cost solution to foster clinical deployment of quantitative imaging of retinal photoreceptors, which are essential for advanced study and diagnosis of eye disease, such as age-related macular degeneration, retinitis pigmentosa, etc., which are known to damage photoreceptors, particularly rod cells at the early stages (26-28).
Acknowledgments
The authors thank Dr. Benquan Wang, Dr. Changgeng Liu, Dr. Yanan Zhi, and Dr. Rongwen Lu for their valuable suggestions and discussions.
Funding: The study was supported by National Institutes of Health (NIH) (R01 EY024628, R01 EY023522, R01 EY030101, R01 EY029673, R01 EY030842, P30 EY001792); Unrestricted grant from Research to Prevent Blindness; Richard and Loan Hill endowment.
Footnote
Provenance and Peer Review: With the arrangement by the Guest Editors and the editorial office, this article has been reviewed by external peers.
Conflicts of Interest: All authors have completed the ICMJE uniform disclosure form (available at http://dx.doi.org/10.21037/qims-20-542). The special issue “Advanced Optical Imaging in Biomedicine” was commissioned by the editorial office without any funding or sponsorship. The authors have no other conflicts of interest to declare.
Open Access Statement: This is an Open Access article distributed in accordance with the Creative Commons Attribution-NonCommercial-NoDerivs 4.0 International License (CC BY-NC-ND 4.0), which permits the non-commercial replication and distribution of the article with the strict proviso that no changes or edits are made and the original work is properly cited (including links to both the formal publication through the relevant DOI and the license). See: https://creativecommons.org/licenses/by-nc-nd/4.0/.
References
- Gustafsson MG. Surpassing the lateral resolution limit by a factor of two using structured illumination microscopy. J Microsc 2000;198:82-7. [Crossref] [PubMed]
- Gustafsson MG, Shao L, Carlton PM, Wang CR, Golubovskaya IN, Cande WZ, Agard DA, Sedat JW. Three-dimensional resolution doubling in wide-field fluorescence microscopy by structured illumination. Biophys J 2008;94:4957-70. [Crossref] [PubMed]
- Yamanaka M, Smith NI, Fujita K. Introduction to super-resolution microscopy. Microscopy 2014;63:177-92. [Crossref] [PubMed]
- Lu RW, Wang BQ, Zhang QX, Yao XC. Super-resolution scanning laser microscopy through virtually structured detection. Biomed Opt Express 2013;4:1673-82. [Crossref] [PubMed]
- Zhi Y, Lu R, Wang B, Zhang Q, Yao X. Rapid super-resolution line-scanning microscopy through virtually structured detection. Opt Lett 2015;40:1683-6. [Crossref] [PubMed]
- Zhi Y, Wang B, Yao X. Super-resolution scanning laser microscopy based on virtually structured detection. Crit Rev Biomed Eng 2015;43:297-322. [Crossref] [PubMed]
- Lu J, Min W, Conchello JA, Xie XS, Lichtman JW. Super-resolution laser scanning microscopy through spatiotemporal modulation. Nano Lett 2009;9:3883-9. [Crossref] [PubMed]
- Liu C, Zhi Y, Wang B, Thapa D, Chen Y, Alam M, Lu Y, Yao X. In vivo super-resolution retinal imaging through virtually structured detection. J Biomed Opt 2016;21:120502. [Crossref] [PubMed]
- Lu Y, Liu C, Yao X. In vivo super-resolution imaging of transient retinal phototropism evoked by oblique light stimulation. J Biomed Opt 2018;23:1-4. [Crossref] [PubMed]
- Ditchburn RW, Ginsborg B. Involuntary eye movements during fixation. J Physiol 1953;119:1-17. [Crossref] [PubMed]
- Robinson D. The mechanics of human saccadic eye movement. J Physiol 1964;174:245-64. [Crossref] [PubMed]
- Delori FC, Webb RH, Sliney DH. Maximum permissible exposures for ocular safety (ANSI 2000), with emphasis on ophthalmic devices. J Opt Soc Am A Opt Image Sci Vis 2007;24:1250-65. [Crossref] [PubMed]
- Donnelly WJ, Roorda A. Optimal pupil size in the human eye for axial resolution. J Opt Soc Am A Opt Image Sci Vis 2003;20:2010-5. [Crossref] [PubMed]
- Cooper RF, Tuten WS, Dubra A, Brainard DH, Morgan JIW. Non-invasive assessment of human cone photoreceptor function. Biomed Opt Express 2017;8:5098-112. [Crossref] [PubMed]
- Jonnal RS, Rha J, Zhang Y, Cense B, Gao W, Miller DT. In vivo functional imaging of human cone photoreceptors. Opt Express 2007;15:16141-60. [Crossref]
- Bedggood P, Metha A. De-warping of images and improved eye tracking for the scanning laser ophthalmoscope. PLoS One 2017;12:e0174617. [Crossref] [PubMed]
- Vogel CR, Arathorn DW, Roorda A, Parker A. Retinal motion estimation in adaptive optics scanning laser ophthalmoscopy. Opt Express 2006;14:487-97. [Crossref] [PubMed]
- Dubra A, Sulai Y, Norris JL, Cooper RF, Dubis AM, Williams DR, Carroll J. Noninvasive imaging of the human rod photoreceptor mosaic using a confocal adaptive optics scanning ophthalmoscope. Biomed Opt Express 2011;2:1864-76. [Crossref] [PubMed]
- Lu J, Gu B, Wang X, Zhang Y. High-speed adaptive optics line scan confocal retinal imaging for human eye. PLoS One 2017;12:e0169358. [Crossref] [PubMed]
- Merino D, Duncan JL, Tiruveedhula P, Roorda A. Observation of cone and rod photoreceptors in normal subjects and patients using a new generation adaptive optics scanning laser ophthalmoscope. Biomed Opt Express 2011;2:2189-201. [Crossref] [PubMed]
- Curcio CA, Millican CL, Allen KA, Kalina RE. Aging of the human photoreceptor mosaic: evidence for selective vulnerability of rods in central retina. Invest Ophthalmol Vis Sci 1993;34:3278-96. [PubMed]
- Curcio CA, Sloan KR, Kalina RE, Hendrickson AE. Human photoreceptor topography. J Comp Neurol 1990;292:497-523. [Crossref] [PubMed]
- Ahnelt PK, Kolb H, Pflug R. Identification of a subtype of cone photoreceptor, likely to be blue sensitive, in the human retina. J Comp Neurol 1987;255:18-34. [Crossref] [PubMed]
- Liu Z, Kurokawa K, Zhang F, Lee JJ, Miller DT. Imaging and quantifying ganglion cells and other transparent neurons in the living human retina. Proc Natl Acad Sci U S A 2017;114:12803-8. [Crossref] [PubMed]
- Roorda A, Romero-Borja F, Donnelly WJ III, Queener H, Hebert TJ, Campbell MC. Adaptive optics scanning laser ophthalmoscopy. Opt Express 2002;10:405-12. [Crossref] [PubMed]
- Curcio CA, Medeiros NE, Millican CL. Photoreceptor loss in age-related macular degeneration. Invest Ophthalmol Vis Sci 1996;37:1236-49. [PubMed]
- Jackson GR, Owsley C, Curcio CA. Photoreceptor degeneration and dysfunction in aging and age-related maculopathy. Ageing Res Rev 2002;1:381-96. [Crossref] [PubMed]
- Portera-Cailliau C, Sung C, Nathans J, Adler R. Apoptotic photoreceptor cell death in mouse models of retinitis pigmentosa. Proc Natl Acad Sci U S A 1994;91:974-8. [Crossref] [PubMed]