18F-FDG PET-based radiomics model for predicting occult lymph node metastasis in clinical N0 solid lung adenocarcinoma
Introduction
Lung cancer remains the most commonly diagnosed cancer and the leading cause of cancer-related death worldwide, with an estimated 2.1 million newly diagnosed lung cancer cases and 1.8 million deaths in 2018 (1). Among these, adenocarcinoma is the most prevalent subtype. The routine treatment for early-stage adenocarcinoma patients is anatomical resection and systemic nodal dissection. Recently, several studies have suggested that limited surgery, such as wedge resection or sublobar resection, is a reasonable treatment option for lung cancer patients without lymph node metastasis (LNM) to preserve lung function (2-4). Adjuvant radiotherapy and chemotherapy were recommended for patients with any sign of LNM (5). Also, the International Association for the Study of Lung Cancer (IASLC) suggested that clinical and pathological lymph node status were closely related to the 5-year survival rate (6). Therefore, identification of lymph node status is a crucial step in early-stage lung cancer and is considered a significant prognostic factor that can be used to determine the surgical plan.
Fluorine-18-fludeoxyglucose (18F-FDG) positron emission tomography/computed tomography (PET/CT) can noninvasively detect the metabolic activity of the disease. It has become an indispensable methodology for the evaluation of the Tumor Node Metastasis (TNM) staging of lung cancers, especially in higher-risk patients with planned surgical treatment (7). However, studies have reported that the incidence of occult lymph node metastasis (OLM) on PET/CT was up to 12.6–26.7% (8-11). Thus, a novel method is needed to improve the diagnostic accuracy of OLM in clinical N0 (cN0) patients. It has become widely acknowledged that the information obtained from the conventional images with unaided eyes is merely a minor representation, while a large amount of valuable data remains concealed in the images (12-14).
Radiomics provides a vital complementary tool to extract the high-dimensional and valuable data from the images (15,16). With the rapid development of radiomics, many predictive models combining radiomics signatures with clinical and radiologic data have been developed to predict the preoperative stage and prognosis of various malignant tumors (14,17-19). However, to our knowledge, there is currently no PET/CT-based radiomics model for predicting OLM of lung adenocarcinoma. Thus, the purpose of the present study was to develop a preoperative model that incorporates radiomics features, clinical characteristics, and PET parameters to predict the OLM of cN0 solid lung adenocarcinoma.
Methods
Patients
Our Institutional Review Board approved this study, and the requirement for informed consent was waived. The inclusion criteria were as follows: (I) patients underwent surgical resection and lymphadenectomy; (II) patients were confirmed to have lung adenocarcinoma postoperatively via histopathological examination; (III) PET-CT was performed before surgery, with a time interval of less than 1 month; and (IV) the lesion was diagnosed as cN0; all short axis of LN were less than 10 mm and the FDG uptake did not exceed that of normal tissue (20). The exclusion criteria were as follows: (I) the lesion appeared as ground-glass opacity (GGO), including pure GGO and GGO-predominant lesion on chest CT scans; (II) FDG uptake of the lesions was lower than or close to the surrounding normal pulmonary parenchyma; (III) patients received chemotherapy or radiotherapy before the PET/CT examination; and (IV) the lesion had distant metastasis.
The histologic classification of the tumor was defined according to the 2015 World Health Organization classification (21). The clinical and pathological staging of the tumor was based on the TNM Classification of Malignant Tumors, the eighth edition (22). Ultimately, a total of 370 patients were enrolled in our study between January 2012 and May 2019. The patients were divided into two groups according to the date they underwent PET/CT imaging. Specifically, patients examined before January 2018 were assigned to the training set, and patients examined after January 2018 was assigned to the validation set (18,23). Their clinical data, including age, gender, surgical procedure, and serum carcinoembryonic antigen (CEA) level, were recorded.
PET/CT image acquisition and analysis
All PET/CT examinations were performed using a hybrid PET/CT system (Gemini 64 TF, Philips Medical Systems, Best, The Netherlands). An intravenous injection of approximately 3.7 MBq/kg of 18F-FDG was administered after fasting for at least 6 hours (blood glucose levels below 110 mL/dL). After resting for 60 minutes, the PET/CT scan was performed with patients in the supine position. For precise anatomical localization and attenuation correction, a low dose unenhanced CT was performed from the skull base to the mid-thigh with the following parameters: tube voltage of 120 kV, tube current of 249 mA, detector collimation of 64 mm × 0.625 mm, the pitch of 0.829, a tube rotation speed of 0.5 seconds, and reconstruction thickness and interval of 5.0 mm. Subsequently, PET images were obtained by using the three-dimensional model, and the parameters were set as follows: field of view of 576 mm, a matrix of 144×144, slice thickness and interval of 5 mm, and an emission scan time of each bed position of 1.5 minutes. PET images with CT attenuation correction were reconstructed using the time of the fight algorithm.
Data measurement and collection were performed on the Philips EBW 3.0 by two radiologists. The tumor size was measured at the longest diameter of the lesion on transverse lung window settings of the CT images (window width, 1,500 HU; window level, −750 HU). Volume viewer software was used to calculate PET parameters, and the tumor volumes of interest (VOIs) were automatically generated using the threshold standardized uptake value (SUV). The maximum standardized uptake value (SUVmax) was defined as the highest SUV voxel in the volume of interest (VOI). Metabolic tumor volume (MTV) was defined as the sum of voxels with SUV ≥40% of the SUVmax (24). Total lesion glycolysis (TLG) was calculated by using the result of MTV multiplied by the mean SUV of the tumor. SURmax represents the ratio of SUVmax to the mean SUV of descending aorta (blood SUVmean). TLGsur represents the ratio of TLG to blood SUVmean.
Tumor segmentation and feature extraction
Commercial software (Region Studio, Regiontec Ltd., Shanghai, China) was used to extract radiomics features. FDG-PET images were imported into the software using the Digital Imaging and Communications in Medicine (DICOM) protocol. Regions of interest (ROIs) were manually delineated around the tumor outline slice-by-slice on the PET images by one radiologist (reader 1) who was blinded to the clinical information and pathological results, and segmentation of the tumor was then performed semi-automatically by the software. The image analysis pipeline was built to extract radiomics features from PET imaging. After 1 week, 60 cases were randomly selected for repeat delineation of the ROIs by reader 1 and another radiologist (reader 2). Both readers had 5 years of clinical experience in CT diagnosis. The inter- and intra-class correlation coefficients (ICCs) were used to assess inter- and intra-observer agreement of the radiomics feature. The intra-observer ICCs were calculated based on the two-time feature extraction of reader 1, and the inter-observer ICCs were calculated based on the first-extracted features drawn by both readers, with ICCs greater than 0.75 denoting a favorable agreement. The radiomics features included first-order features, shape features, gray level co-occurrence matrix (GLCM) features, gray level size zone matrix (GLSZM) features, gray level run length matrix (GLRLM) features, neighbouring gray tone difference matrix (NGTDM) features, and gray level dependence matrix (GLDM) features. The specific radiomics features are provided in Table S1.
Radiomics feature selection and development of a radiomics signature
The 10-fold cross-validated least absolute shrinkage and selection operator (LASSO) method, which is suitable for the regression analysis of high-dimensional data, was used to select valuable radiomics features from the training set (18). Multivariate logistic regression analysis was subsequently applied to the selected radiomics features. A radiomics signature was calculated via a linear combination of finally selected features weighted by their respective coefficient.
Construction of the model and clinical utility
Clinical features, PET parameters, and radiomics signatures with statistical significance (P<0.05) in the univariate analysis of the training set were selected into a multivariable binary logistic regression analysis using backward stepwise selection. Next, a nomogram was constructed based on the multivariable binary logistic regression analysis with P<0.05. The model was applied to the validation dataset to assess accuracy. The performance of the nomogram was assessed in the training and validation sets by concordance-index (C-index), and calibration curves. Analysis decision curve analysis (DCA) was performed by calculating the net benefits of joint training and validation sets under different threshold probabilities to evaluate the clinical effectiveness of the nomogram.
Statistical analysis
The analyses were performed using IBM SPSS (version 23.0) and R software (version 3.6.1). Continuous variables were described as mean ± standard deviations and analyzed by the Mann-Whitney U test. Categorical variables, including sex and tumor location, were expressed as frequency and analyzed using the Chi-square test. The “glmnet” package administered LASSO binary logistic regression analysis. Multivariate binary logistic regression analysis, nomograms, and calibration plots were performed with the “rms” package. The C-index calculation was conducted using the “Hmisc” package. DCA was performed using the “rmda” package. A two-sided P value <0.05 was considered statistically significant.
Results
Baseline characteristics
The characteristics of 370 patients (165 men, 205 women; mean age, 62.95±9.40 years) are shown in Table 1. A total of 236 patients were assigned to the training set, and 134 patients were assigned to the validation set. There were no statistically significant differences in age, gender, and tumor location between patients with and without OLM in both the training and validation sets, while CEA, tumor size, SUVmax, MTV, TLG, SURmax, and TLGsur were significantly different between the two sets.
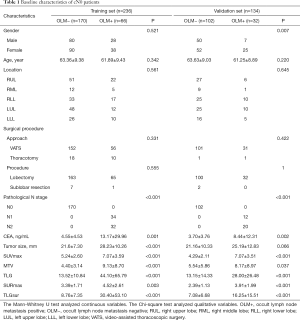
Full table
Radiomics feature selection and development of the radiomics signature
One hundred seven robust radiomics features were extracted in the present study. After removing the features with an ICC ≤0.75, a total of 62 stable radiomics features were retained for subsequent analysis. After LASSO logistic regression analysis (Figure 1A,B), only skewness, total energy, small dependence low gray-level emphasis (SDLGLE) and gray level non-uniformity normalized (GLNN) of the gray level run length matrix (GLRLM) features were incorporated into the radiomics signature: Radiomics signature =−0.995–0.398 skewness + 1.416 total energy + 1.119 SDLGLE − 1.519 GLNN.
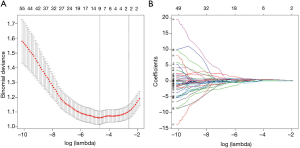
The radiomics signature in patients with OLM was significantly higher than those without OLM in the training set (−1.43±0.87 vs. 0.14±2.75, P<0.0001). These findings were confirmed in the validation set (−1.42±1.06 vs. −0.35±2.74, P<0.0001).
Construction of the model and clinical utility
The results of the univariate and multivariate binary logistic regression analysis in the training set are shown in Table 2. The univariate analysis showed significant differences between patients with and without OLM in eight parameters, including CEA, tumor size, SUVmax, MTV, TLG, SURmax, TLGsur, and the radiomics signature. The multivariate logistic regression analysis showed that CEA, MTV, and the radiomics signature were significant independent risk factors in the prediction of OLM. Subsequently, CEA, MTV, and the radiomics signature were incorporated to construct the nomogram (Figure 2). The prediction nomogram showed a C-index of 0.769 in the training set and 0.768 in the validation set. The calibration curve demonstrated good agreement between the nomogram-predicted probability of OLM and the actual OLM rate in the training and validation sets (Figure 3A,B). The decision curve showed that if the threshold probability of a patient was >10%, using this nomogram to predict OLM would provide greater benefit than the treat-all-patients strategy or the treat-none strategy (Figure 4).
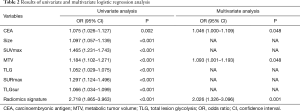
Full table
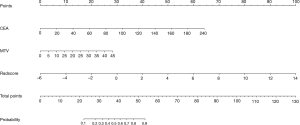
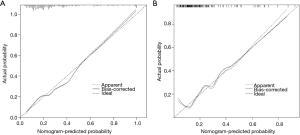
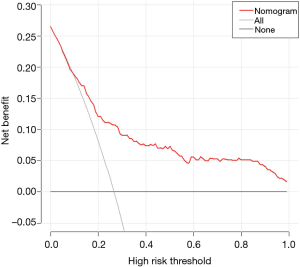
Discussion
In recent years, radiomics have been widely studied as a noninvasive and reproducible method to extract high-dimensional features from radiological images. Radiomics features can objectively describe tumor characteristics in terms of border, shape, texture, and spatial structure, which could reflect intra-tumor heterogeneity and gene-expression patterns (25-27). They can also help to discriminate different types of cancer, differentiate LNM from benign LN, and determine tumor treatment plans (28,29). Furthermore, it was reported that the predictive accuracy of the radiomics signature based on CT scans was favorable for predicting OLM in patients with lung adenocarcinoma (17). However, the radiomics model based on PET/CT for predicting OLM of lung adenocarcinoma is rarely reported. Thus, this study aimed to develop a PET-based radiomics model to predict OLM.
Based on the results of the LASSO binary logistic regression analysis, skewness, total energy, SDLGLE, and GLNN were considered the four most valuable features in our study. Among these, skewness and total energy belonged to the first-order features. The skewness measured the asymmetry of the distribution of values about the mean value. The total energy was defined as the value of energy feature (a measure of the magnitude of voxel values in the image) scaled by the volume of the voxel (in cubic mm). This ensured that voxels with the lowest gray values contributed least to energy, instead of voxels with gray level intensity closest to zero. SDLGLE belonged to the gray level dependence matrix (GLDM) features and measured the joint distribution of small dependence with lower gray-level values. GLNN of GLRLM features measured the similarity of gray-level intensity values in the image, where a lower GLNN value correlated with a greater similarity in intensity values. The features reflected the heterogeneity of the various aspects of the ROIs in the images. The radiomics signature constructed by these radiomics features was superior to a single variable and was similar to the multi-dimensional panels method (17,18). As an important radiomics predictive index, the radiomics signature in this study resulted in a more accurate diagnosis and helped to predict OLM before surgery, with a greater value indicating a higher likelihood of OLM.
Many studies have shown that radiomics signatures combined with clinical and radiological data have a better predictive value (30-34). Thus, we also studied PET/CT parameters and clinical data. We identified that the CEA, tumor size, and 18F-FDG PET/CT parameters, including SUVmax, MTV, TLG, SURmax, and TLGsur, were risk factors for OLM, which is consistent with reports from previous studies (9,10,20,35). Also, multivariate logistic regression analysis found that CEA, MTV, and the radiomics signature were independent risk predictors for OLM. Numerous studies had reported that serum CEA level was associated with the pathological stage, rate of LNM, and prognosis of cancer (8,31,36,37). Also, Miao et al. found that CEA was an independent risk factor for LNM in adenocarcinoma (8). Our study also demonstrated that CEA was an independent risk predictor for OLM, which was consistent with the findings of Miao et al. MTV was a composite parameter reflecting both tumor metabolic activity and tumor volume, and was significantly associated with OLM in patients. Previous studies reported that MTV had a better predictive performance than other PET parameters and might be a possible indicator for sublobar resection in clinically node-negative lung cancer (9,24). However, it is always difficult to identify OLM from benign LN by conventional methods, and using a sole parameter to differentiate malignant from benign LN remains challenging. Thus, the present study developed a PET/CT-based nomogram model incorporating CEA, MTV, and the radiomics signature to predict OLM in patients of cN0 solid lung adenocarcinoma, and demonstrated adequate discrimination in the training set (C-index, 0.769) and the validation set (C-index, 0.768).
A nomogram is a statistical model that can calculate the numerical probability of a patient by integrating multiple variables and provides an intuitive and convenient method for doctors to clinically diagnose OLM as well as fulfilling the drive towards personalized medicine (38). Moreover, it is more concise and effective than other methods in the prediction of the LN metastasis in lung adenocarcinomas (39,40). Another advantage of the nomogram is its noninvasiveness and repeatability, thereby allowing for routine application. In this study, a PET/CT-based nomogram model was developed and demonstrated high diagnostic accuracy. The C-index, calibration curve, and DCA indicated that the nomogram shows good clinical utility. Given that our nomogram has favorable discrimination and clinical utility, it might facilitate the individualized prediction of OLM and precise individualized medical treatment.
A further advantage of this study is that only solid lung adenocarcinoma patients were enrolled. This is because patients with different histological types would influence the size, metabolic parameters of the primary tumor, among many others (24). For example, SUVmax of squamous cell carcinoma is higher than adenocarcinoma in general (41). Therefore, a combined analysis of these histological types is not logical, especially in a PET study. The ground glass nodules of lung adenocarcinoma manifested most frequently as lepidic predominant growth patterns microscopically and generally considered as without LNM (8,42), which can affect the incidence of OLM. Therefore, only solid lung adenocarcinoma patients were enrolled in the present study, thus allowing us to avoid such problems.
However, although the results were encouraging, our study still had several limitations. Firstly, this was a single-center retrospective study, which means that it has potential data selection bias. Secondly, the number of patients with OLM was much lower than the number of patients with negative LNM, which may affect the P value in comparative analysis. Consequently, more prospective and large-samples studies are needed to determine these predictive factors and confirm the accuracy of the nomogram for predicting OLM. Finally, the pathological stage and CT features of the tumor were not included in the present study, which may have affected the results.
Conclusions
In our study, the diagnostic PET/CT-based radiomics nomogram, including CEA, MTV, and the radiomics signature, was developed to predict the OLM in cN0 solid lung adenocarcinoma. The nomogram demonstrated adequate predictive accuracy and clinical net benefit and was conveniently used to facilitate the individualized preoperative prediction of OLM in cN0 solid lung adenocarcinoma.
Acknowledgments
Xiangwu Zheng kindly provided statistical advice for this manuscript. The authors would like to thank Kun Tang for the study design and editing the draft of the manuscript.
Funding: This research was supported by the Zhejiang Province Public Welfare Technology Application Research Project (No. LGF19H180012).
Footnote
Conflicts of Interest: All authors have completed the ICMJE uniform disclosure form(available at http://dx.doi.org/10.21037/qims-20-337). The authors have no conflicts of interest to declare.
Ethical Statement: The local institutional review board approved this retrospective study and waived the requirement for informed consent.
Open Access Statement: This is an Open Access article distributed in accordance with the Creative Commons Attribution-NonCommercial-NoDerivs 4.0 International License (CC BY-NC-ND 4.0), which permits the non-commercial replication and distribution of the article with the strict proviso that no changes or edits are made and the original work is properly cited (including links to both the formal publication through the relevant DOI and the license). See: https://creativecommons.org/licenses/by-nc-nd/4.0/.
References
- Bray F, Ferlay J, Soerjomataram I, Siegel RL, Torre LA, Jemal A. Global cancer statistics 2018: GLOBOCAN estimates of incidence and mortality worldwide for 36 cancers in 185 countries. CA Cancer J Clin 2018;68:394-424. [Crossref] [PubMed]
- Yerokun BA, Yang CJ, Gulack BC, Li X, Mulvihill MS, Gu L, Wang X, Harpole DH, D'Amico TA, Berry MF, Hartwig MG. A national analysis of wedge resection versus stereotactic body radiation therapy for stage IA non-small cell lung cancer. J Thorac Cardiovasc Surg 2017;154:675-86.e4. [Crossref] [PubMed]
- Zhang Z, Feng H, Zhao H, Hu J, Liu L, Liu Y, Li X, Xu L, Li Y, Lu X, Fu X, Yan H, Liu D. Sublobar resection is associated with better perioperative outcomes in elderly patients with clinical stage I non-small cell lung cancer: a multicenter retrospective cohort study. J Thorac Dis 2019;11:1838. [Crossref] [PubMed]
- Grills IS, Mangona VS, Welsh R, Chmielewski G, McInerney E, Martin S, Wloch J, Ye H, Kestin LL. Outcomes After Stereotactic Lung Radiotherapy or Wedge Resection for Stage I Non-Small-Cell Lung Cancer. J Clin Oncol 2010;28:928-35. [Crossref] [PubMed]
- Ettinger DS, Akerley W, Borghaei H, Chang AC, Cheney RT, Chirieac LR, D'Amico TA, Demmy TL, Govindan R, Grannis FW Jr, Grant SC, Horn L, Jahan TM, Komaki R, Kong FM, Kris MG, Krug LM, Lackner RP, Lennes IT, Loo BW Jr, Martins R, Otterson GA, Patel JD, Pinder-Schenck MC, Pisters KM, Reckamp K, Riely GJ, Rohren E, Shapiro TA, Swanson SJ, Tauer K, Wood DE, Yang SC, Gregory K, Hughes M. National comprehensive cancer n. Non-small cell lung cancer, version 2.2013. J Natl Compr Canc Netw 2013;11:645-53. [Crossref] [PubMed]
- Asamura H, Chansky K, Crowley J, Goldstraw P, Rusch VW, Vansteenkiste JF, Watanabe H, Wu YL, Zielinski M, Ball D, Rami-Porta R, International Association for the Study of Lung Cancer S, Prognostic Factors Committee ABM, Participating I. The International Association for the Study of Lung Cancer Lung Cancer Staging Project: Proposals for the Revision of the N Descriptors in the Forthcoming 8th Edition of the TNM Classification for Lung Cancer. J Thorac Oncol 2015;10:1675-84.
- Shim SS, Lee KS, Kim BT, Chung MJ, Lee EJ, Han J, Choi JY, Kwon OJ, Shim YM, Kim S. Non-small cell lung cancer: Prospective comparison of integrated FDG PET/CT and CT alone for preoperative staging. Radiology 2005;236:1011-9. [Crossref] [PubMed]
- Miao H, Shaolei L, Nang L, Yumei L, Shanyuan Z, Fangliang L, Yue Y. Occult mediastinal lymph node metastasis in FDG-PET/CT node-negative lung adenocarcinoma patients: Risk factors and histopathological study. Thoracic Cancer 2019;10:1453-60. [Crossref] [PubMed]
- Park SY, Yoon JK, Park KJ, Lee SJ. Prediction of occult lymph node metastasis using volume-based PET parameters in small-sized peripheral non-small cell lung cancer. Cancer Imaging 2015;15:21. [Crossref] [PubMed]
- Li L, Ren S, Zhang Y, Guan Y, Zhao J, Liu J, Wang Q, Chen G, Chen H, Xiang J, Fu X. Risk factors for predicting the occult nodal metastasis in T1-2N0M0 NSCLC patients staged by PET/CT: potential value in the clinic. Lung Cancer 2013;81:213-7. [Crossref] [PubMed]
- Park HK, Jeon K, Koh WJ, Suh GY, Kim H, Kwon OJ, Chung MP, Lee KS, Shim YM, Han J, Um SW. Occult nodal metastasis in patients with non-small cell lung cancer at clinical stage IA by PET/CT. Respirology 2010;15:1179-84. [Crossref] [PubMed]
- Gillies RJ, Kinahan PE, Hricak H. Radiomics: Images Are More than Pictures, They Are Data. Radiology 2016;278:563-77. [Crossref] [PubMed]
- Lee G, Lee HY, Park H, Schiebler ML, van Beek EJR, Ohno Y, Seo JB, Leung A. Radiomics and its emerging role in lung cancer research, imaging biomarkers and clinical management: State of the art. Eur J Radiol 2017;86:297-307. [Crossref] [PubMed]
- Wang Y, Liu W, Yu Y, Liu JJ, Xue HD, Qi YF, Lei J, Yu JC, Jin ZY. CT radiomics nomogram for the preoperative prediction of lymph node metastasis in gastric cancer. Eur Radiol 2020;30:976-86. [Crossref] [PubMed]
- Lambin P, Leijenaar RTH, Deist TM, Peerlings J, de Jong EEC, van Timmeren J, Sanduleanu S, Larue RTHM, Even AJG, Jochems A, van Wijk Y, Woodruff H, van Soest J, Lustberg T, Roelofs E, van Elmpt W, Dekker A, Mottaghy FM, Wildberger JE, Walsh S. Radiomics: the bridge between medical imaging and personalized medicine. Nat Rev Clin Oncol 2017;14:749-62. [Crossref] [PubMed]
- Lambin P, Rios-Velazquez E, Leijenaar R, Carvalho S, van Stiphout RGPM, Granton P, Zegers CML, Gillies R, Boellard R, Dekker A, Aerts HJWL. Consortium Q-C. Radiomics: Extracting more information from medical images using advanced feature analysis. Eur J Cancer 2012;48:441-6. [Crossref] [PubMed]
- Zhong Y, Yuan M, Zhang T, Zhang YD, Li H, Yu TF. Radiomics Approach to Prediction of Occult Mediastinal Lymph Node Metastasis of Lung Adenocarcinoma. AJR Am J Roentgenol 2018;211:109-13. [Crossref] [PubMed]
- Zhao W, Xu YN, Yang ZM, Sun YL, Li C, Jin L, Gao P, He WJ, Wang PJ, Shi HL, Hua YQ, Li M. Development and validation of a radiomics nomogram for identifying invasiveness of pulmonary adenocarcinomas appearing as subcentimeter ground-glass opacity nodules. Eur J Radiol 2019;112:161-8. [Crossref] [PubMed]
- Liu H, Zhang C, Wang L, Luo R, Li J, Zheng H, Yin Q, Zhang Z, Duan S, Li X, Wang D. MRI radiomics analysis for predicting preoperative synchronous distant metastasis in patients with rectal cancer. Eur Radiol 2019;29:4418-26. [Crossref] [PubMed]
- Ouyang ML, Tang K, Xu MM, Lin J, Li TC, Zheng XW. Prediction of Occult Lymph Node Metastasis Using Tumor-to-Blood Standardized Uptake Ratio and Metabolic Parameters in Clinical N0 Lung Adenocarcinoma. Clin Nucl Med 2018;43:715-20. [Crossref] [PubMed]
- Travis WD, Brambilla E, Nicholson AG, Yatabe Y, Austin JHM, Beasley MB, Chirieac LR, Dacic S, Duhig E, Flieder DB, Geisinger K, Hirsch FR, Ishikawa Y, Kerr KM, Noguchi M, Pelosi G, Powell CA, Tsao MS, Wistuba I, Panel WHO. The 2015 World Health Organization Classification of Lung Tumors: Impact of Genetic, Clinical and Radiologic Advances Since the 2004 Classification. J Thorac Oncol 2015;10:1243-60. [Crossref] [PubMed]
- Rami-Porta R, Asamura H, Travis WD, Rusch VW. Lung cancer - major changes in the American Joint Committee on Cancer eighth edition cancer staging manual. CA Cancer J Clin 2017;67:138-55.
- Xue X, Yang Y, Huang Q, Cui F, Lian YQ, Zhang SY, Yao LP, Peng W, Li X, Pang PP, Yan JH, Chen F. Use of a Radiomics Model to Predict Tumor Invasiveness of Pulmonary Adenocarcinomas Appearing as Pulmonary Ground-Glass Nodules. Biomed Res Int 2018;2018:6803971. [Crossref] [PubMed]
- Ouyang ML, Xia HW, Xu MM, Lin J, Wang LL, Zheng XW, Tang K. Prediction of occult lymph node metastasis using SUV, volumetric parameters and intratumoral heterogeneity of the primary tumor in T1-2N0M0 lung cancer patients staged by PET/CT. Ann Nucl Med 2019;33:671-80. [Crossref] [PubMed]
- Gatenby RA, Grove O, Gillies RJ. Quantitative imaging in cancer evolution and ecology. Radiology 2013;269:8-15. [Crossref] [PubMed]
- Aerts HJWL, Velazquez ER, Leijenaar RTH, Parmar C, Grossmann P, Carvalho S, Bussink J, Monshouwer R, Haibe-Kains B, Rietveld D, Hoebers F, Rietbergen MM, Leemans CR, Dekker A, Quackenbush J, Gillies RJ, Lambin P. Decoding tumour phenotype by noninvasive imaging using a quantitative radiomics approach. Nat Commun 2014;5:4006. [Crossref] [PubMed]
- Carvalho S, Leijenaar RTH, Troost EGC, van Timmeren JE, Oberije C, van Elmpt W, de Geus-Oei LF, Bussink J, Lambin P. 18F-fluorodeoxyglucose positron-emission tomography (FDG-PET)-Radiomics of metastatic lymph nodes and primary tumor in non-small cell lung cancer (NSCLC) - A prospective externally validated study. PLoS One 2018;13:e0192859. [Crossref] [PubMed]
- Coroller TP, Agrawal V, Huynh E, Narayan V, Lee SW, Mak RH, Aerts H. Radiomic-Based Pathological Response Prediction from Primary Tumors and Lymph Nodes in NSCLC. J Thorac Oncol 2017;12:467-76. [Crossref] [PubMed]
- Song SH, Park H, Lee G, Lee HY, Sohn I, Kim HS, Lee SH, Jeong JY, Kim J, Lee KS, Shim YM. Imaging Phenotyping Using Radiomics to Predict Micropapillary Pattern within Lung Adenocarcinoma. J Thorac Oncol 2017;12:624-32. [Crossref] [PubMed]
- Chen A, Lu L, Pu X, Yu T, Yang H, Schwartz LH, Zhao B. CT-Based Radiomics Model for Predicting Brain Metastasis in Category T1 Lung Adenocarcinoma. AJR Am J Roentgenol 2019.1-6. [PubMed]
- Ji GW, Zhu FP, Zhang YD, Liu XS, Wu FY, Wang K, Xia YX, Zhang YD, Jiang WJ, Li XC, Wang XH. A radiomics approach to predict lymph node metastasis and clinical outcome of intrahepatic cholangiocarcinoma. Eur Radiol 2019;29:3725-35. [Crossref] [PubMed]
- Alahmari SS, Cherezov D, Goldgof D, Hall L, Gillies RJ, Schabath MB. Delta Radiomics Improves Pulmonary Nodule Malignancy Prediction in Lung Cancer Screening. IEEE Access 2018;6:77796-806.
- Tan Y, Zhang ST, Wei JW, Dong D, Wang XC, Yang GQ, Tian J, Zhang H. A radiomics nomogram may improve the prediction of IDH genotype for astrocytoma before surgery. Eur Radiol 2019;29:3325-37. [Crossref] [PubMed]
- Zhang R, Xu L, Wen X, Zhang J, Yang P, Zhang L, Xue X, Wang X, Huang Q, Guo C, Shi Y, Niu T, Chen F. A nomogram based on bi-regional radiomics features from multimodal magnetic resonance imaging for preoperative prediction of microvascular invasion in hepatocellular carcinoma. Quant Imaging Med Surg 2019;9:1503-15. [Crossref] [PubMed]
- Kaseda K, Asakura K, Kazama A, Ozawa Y. Risk Factors for Predicting Occult Lymph Node Metastasis in Patients with Clinical Stage I Non-small Cell Lung Cancer Staged by Integrated Fluorodeoxyglucose Positron Emission Tomography/Computed Tomography. World J Surg 2016;40:2976-83. [Crossref] [PubMed]
- Inoue M, Minami M, Shiono H, Sawabata N, Ideguchi K, Okumura M. Clinicopathologic study of resected, peripheral, small-sized, non-small cell lung cancer tumors of 2 cm or less in diameter: pleural invasion and increase of serum carcinoembryonic antigen level as predictors of nodal involvement. J Thorac Cardiovasc Surg 2006;131:988-93. [Crossref] [PubMed]
- Bao F, Yuan P, Yuan X, Lv X, Wang Z, Hu J. Predictive risk factors for lymph node metastasis in patients with small size non-small cell lung cancer. J Thorac Dis 2014;6:1697-703. [PubMed]
- Li M, Chen T, Zhao W, Wei C, Li X, Duan S, Ji L, Lu Z, Shen J. Radiomics prediction model for the improved diagnosis of clinically significant prostate cancer on biparametric MRI. Quant Imaging Med Surg 2020;10:368-79. [Crossref] [PubMed]
- Wang X, Zhao X, Li Q, Xia W, Peng Z, Zhang R, Li Q, Jian J, Wang W, Tang Y, Liu S, Gao X. Can peritumoral radiomics increase the efficiency of the prediction for lymph node metastasis in clinical stage T1 lung adenocarcinoma on CT? Eur Radiol 2019;29:6049-58. [Crossref] [PubMed]
- Yang X, Pan X, Liu H, Gao D, He J, Liang W, Guan Y. A new approach to predict lymph node metastasis in solid lung adenocarcinoma: a radiomics nomogram. J Thorac Dis 2018;10:S807-19. [Crossref] [PubMed]
- Kim DH, Jung JH, Son SH, Kim CY, Hong CM, Oh JR, Jeong SY, Lee SW, Lee J, Ahn BC. Prognostic Significance of Intratumoral Metabolic Heterogeneity on 18F-FDG PET/CT in Pathological N0 Non-Small Cell Lung Cancer. Clin Nucl Med 2015;40:708-14. [Crossref] [PubMed]
- Gardiner N, Jogai S, Wallis A. The revised lung adenocarcinoma classification-an imaging guide. J Thorac Dis 2014;6:S537-46. [PubMed]