Advances in stimulated Raman scattering imaging for tissues and animals
Introduction
Imaging in live cells, tissues, and organisms is important for studies in biology and medicine because it reveals the structural and functional status of biomolecules inside the living biological system. Optical spectroscopy offers a non-invasive means to investigate underlying molecular processes in biological and chemical systems. Of note are fluorescence-based processes, which reveal electronic states, and Raman-based processes, which reveal vibrational states. Fluorescence microscopy is a well-known imaging method used to study living systems with high spatial and temporal resolution. There are, however, limitations such as unwanted photo toxicity and fluorescent probes (including fluorescent dyes and proteins) that are often bulky and would thus disturb or alter the distribution and activity of small molecules in subcellular microdomains. Additionally, the excitation and emission spectra of fluorophores are broader than the Raman spectra of Raman dyes, and thus have a current maximum multiplexing of 6 simultaneous and distinct fluorescent channels without unmixing overlapping peaks or considering fluorescent lifetime and decay (1). The tradeoffs between resolution, signal-to-noise ratios (SNR), equipment costs, and unwanted photo effects are shared among imaging techniques, and fluorescence microscopy has seen swift development in recent years to address these concerns. While fluorescence microscopy is used in countless studies worldwide, Raman has certain advantages in both the label-free imaging, and probed imaging of small biomolecules. The number of vibrational modes observed by Raman is greater than the number of auto-fluorescent species in tissues, which are often consigned to NADH and FAD pools (2) with relatively broad band spectra. This means label-free Raman imaging of many mammalian cells and tissues has a larger theoretical multiplexing ability than label-free fluorescence microscopy.
Vibrational spectroscopy emerges as a complimentary chemical imaging method by probing molecular vibrations rather than fluorescent contrast from electronic states. Chemical bonds of molecules vibrate at different frequencies, producing distinct fingerprints of the molecules that can be recorded by infrared (IR) or Raman spectroscopy. Raman spectroscopy measures the inelastic scattering of light by vibrating molecules. It depends on a change in the polarizability of a molecule. While IR spectroscopy measures the absolute absorption of IR radiation by vibrating molecules, it depends on the change in the dipole moment. Although IR spectroscopy has many merits such as the robust signal fingerprint region, it has limitation in live-cell imaging due to strong water absorption in the IR spectrum, and the translation of IR spectroscopy in clinic is elusive, which is discussed in (3). This is avoided in Raman spectroscopy, which uses visible or near-IR light sources. Raman spectroscopy has been widely employed for biomedical imaging, as reviewed by (4-8). However, the signal intensity of spontaneous Raman scattering is very weak and its Raman cross-section (a measure of probability that a specific interaction will take place) is extremely small compared with fluorescence cross-section (~10−30vs. ~10−16 cm2 per molecule) (9), which greatly limits the imaging speed and is unsuitable for visualizing biomolecule metabolic dynamics inside living cells and tissues.
Stimulated Raman scattering (SRS) phenomenon was discovered in 1962 (10), but not until a decade ago has an SRS imaging platform developed. The first SRS loss/gain using two laser beams with frequencies separated by vibrational frequency was developed by Owyoung and Jones in 1977 (11). SRS microscopy imaging was demonstrated on polystyrene beads in 2007 by employing a femtosecond laser/amplifier system (12). Shortly thereafter, bio-imaging was developed in 2008 by Xie group (13) using a high-repetition rate picosecond laser implemented with a high-frequency phase-sensitive detection scheme, as well as by other researchers in 2009 (14,15). Ever since, SRS has been applied countless times to visualize and image biological tissue with mounting academic and clinical interest. A brief overview of SRS publications using Microsoft Academic in Figure 1 illustrates this exponential trend.
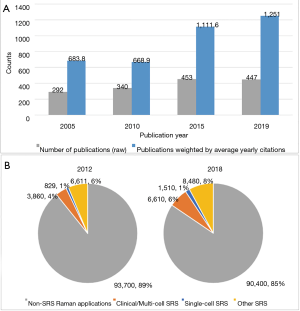
SRS is a nonlinear process that uses two synchronized pulse lasers, namely a pump beam (ωp) and a Stokes beam (ωs), to coherently excite the vibration of molecules. When the frequency difference between the pump and Stokes beams equals the vibration frequency of a chemical bond inside the molecule, the intensity of the Stokes beam increases (stimulated Raman gain, SRG) and the intensity of pump beam decreases (stimulated Raman loss, SRL). Both SRG and SRL belong to the SRS process, and either can be detected by SRS microscopy. Nevertheless, configuration to detect SRL is used more often. In this configuration, the Stokes beam is filtered out and the pump beam is directed to a silicon photodiode so that the SRL is detected by a lock-in amplifier. In SRG spectroscopy, the pump beam is filtered and the SRG at Stokes beam is detected. SRS microscopy has a high imaging speed (1,000 times faster) of up to video rate (17), high specificity, high spectral resolution, and 3D imaging capability in tissues and animals (18). Compared to another widely used coherent Raman process, coherent anti-Stokes Raman scattering (CARS), SRS is non-resonant background-free and the SRS signal of a molecular species is linearly proportional to its concentration, whereas the signal in CARS is proportional to the square of the concentration (17). These advantages provide an array of biological research niches. For example, in the study of retinoids within the liver, the high concentrations of retinoid overwhelm CARS with non-resonant background. Quantitative analysis is also complicated by the inability of CARS to resolve intensities easily and reliably. However, SRS demonstrated clear label-free quantification of retinoid stores in C. elegans, as well as their effects on macromolecular metabolism (19). In addition, the SRS signal occurs at the same wavelength as the excitation beam, and therefore has the same spectral profile as the spontaneous Raman spectrum, which enables quantitative image analysis. Because of these advantages, SRS microscopy has grown rapidly as a powerful and favorable technique for imaging in biology and medicine.
SRS imaging can be demonstrated in three modes: single-frequency, hyperspectral, and multiplex. Single-frequency SRS microscopy uses two narrowband picosecond lasers that provide high speed vibrational imaging of a single Raman band at video rate (20), but without the spectral information. It is commonly used to image known components in samples. Hyperspectral SRS (hsSRS) imaging can be achieved by changing the wavelength of one or both lasers, by continual tuning of a narrowband picosecond laser, chirping pump and Stokes lasers and tuning the time delay between them (called spectral focusing) (21,22), or femtosecond pulse-shaping technology (23-25). Pulse shaping technology may obtain a spectral resolution higher than 10 cm−1. However, hsSRS imaging speeds are too slow (seconds to minutes for reconstructing an entire stack of images) (26) to visualize fast dynamics in living organisms. In multiplex SRS imaging, the SRS spectrum at each pixel is captured simultaneously over a large spectral window by using broadband excitation, and usually, multi-channel detection and lock-in modulation are also used. Fu et al. obtained multicolor SRS imaging by modulating three wavelength components of a filtered broadband pulse at three different frequencies, which could be extracted after signal collection to provide the amplitude components at three different modulations (27). A fast array detector was also used and synchronized to the modulation frequency. Liao et al. (28) used a 16-channel tuned amplifier array, lock-in-free, and obtained an SRS spectrum of 180 cm−1 in 32 µs by spectrally separating a scattered broadband pulse with a diffraction grating. Further, Zhang et al. (29) developed a 32-channel tuned amplifier array that allowed for an acquisition speed at 5 µs per spectrum (~200 cm−1). More details of SRS imaging modalities, workflow, and applications can be found in (26,30-32).
SRS imaging was originally developed as a label-free modality. Together with other advantages including high speed, high chemical specificity, and high (subcellular) resolution, label-free SRS microscopy has been widely used for imaging biomolecules in cells such as lipids (33-35), protein (36,37), nucleic acids (38), cholesterol (39), as well as for direct imaging of a variety of tissues and organs such as neural (40,41), brain (37,42), and skin tissues (43) ex vivo and in animals (37,41). Recently, a new SRS imaging strategy has been developed to increase molecular specificity of SRS, by incorporating a variety of special vibrational probes, including stable-isotope (e.g., deuterium and 13C) (18,44-46), triple-bond tags (e.g., alkyne, nitrile, diyne) (47-52), small molecules (53,54), and nanomaterials (55-57). Chemical bonds associated with many of these probes have distinct vibrational frequencies in the cell-silent region (1,800–2,800 cm−1), making them easily discernable. Not only can the spatial information of targeted biomolecules be revealed, but the metabolic activities of these molecules can also be visualized in live cells, tissues, and organisms as well. This is currently not achievable using label-free imaging.
SRS microscopy has been evolving quickly and has been extensively applied for bioimaging in the last decade. Tremendous work has been conducted on cellular imaging, and the developments and applications of SRS in this area have been thoroughly discussed and reviewed (9,26,58,59). In this review we focus on recent advances and applications of SRS imaging in tissues, organs, and animals, both in the laboratory and in the clinic. Studying the whole organism will provide more structural and functional information on its in vivo processes under physiological or pathological conditions than is attainable solely on cells. Many technologies and methods for SRS cell imaging can be extended to imaging tissues and animals. As another powerful Raman imaging technology, CARS microscopy has been more often used for label-free imaging. The details of CARS, its technological developments and applications in life science have been nicely reviewed in (9,60-63). Due to the scope and space constraints, there may be studies that were regrettably omitted. We will review the advances and applications of label-free SRS microscopy imaging first, and then review labeled (stable isotope- and small molecule-labeled) SRS imaging.
Label-free SRS imaging in tissues and animals
Instrumentation advances
Label-free SRS imaging is frequently conducted by probing the signals of lipids, proteins and DNA in cells and tissues, which are found in the C-H stretching region at 2,800–3,100 cm−1. In general, transmissive mode imaging is often used for thin samples, and epi mode imaging for thick samples—typical for imaging live animals. Most recently, Hill et al. (64) employed a simultaneous epi and transmissive detection configuration and compared the SRS signal size and imaging depth in murine tissues (brain, lung, kidney, liver) ex vivo. The imaging depth of epi and transmissive was shown dependent on tissue type and tissue thickness. Transmissive imaging obtained higher SNR and imaging depths in brain tissue samples under 2-mm thick, and in kidney and liver tissues. Epi imaging achieved higher SNR and imaging depth in lung tissue. Using a convolutional neural network (CNN) based denoising algorithm the maximum imaging depth could be further increased (e.g., from 170 to 210 µm in brain tissue) (64).
Elimination or attenuation of background noise is sorely needed in tissue imaging as SRS is not completely background free. This is due to a series of nonlinear scattering, mixing, and absorptive processes (such as cross-phase modulation and thermal lensing etc.). Instead of the conventional intensity modulation, frequency modulation has been used to subtract the broadband backgrounds while keeping the narrowband signal. Frequency modulation uses two identical excitations with a phase difference of 180° to automatically cancel the broadband background. This is usually done by synchronizing picosecond pulsed lasers or amplifiers (65,66), and precisely shaping and controlling broadband femtosecond pulses (67,68). In a latest study Xiong et al. (69) reports a robust frequency modulation method based on self-phase modulation (SPM) of picosecond pulses. By harnessing the spectral splitting for SPM-induced self-balance of dual-frequency picosecond Stokes pulses, they successfully suppressed non-resonant cross-phase modulation backgrounds and the overwhelming electronic transition derived background.
Broadband coherent Raman imaging (CRI) techniques such as pulse-shaping faster lasers for better spectral focusing have been shown to improve spectral resolution and optimize Raman signal intensity (70). These techniques are used to adapt the laser source in order to selectively amplify the vibrational modes of interest in the analyte and can be achieved by carefully shaping the laser pulse’s waveform and tuning the scanning units (71). There are several analog and digital methods to accomplish this, including recent conjugation of acousto-optic programmable dispersive filters (AOPDF) (72), as well as asymmetric pulse shaping with bandpass filters (73). In a first-of-its-kind application of AOPDF modulated SRS on biological samples, Audier et al. reported imaging of spectral windows larger than 200 cm−1 with 12 µs pixel dwell time and a scanning rate of 40 kHz with shot noise limited sensitivity of an impressive 25 cm−1 resolution (72). Their SRS instrumentation was equipped with femtosecond pump and Stokes lasers chirped at 1 picosecond, enabling them to take a 100 µm × 100 µm image of a frozen colon cancer tissue, stitched over 80 scanning areas in just 15 minutes.
Another direction for improving sensitivity and resolution is to spatially or temporally modulate the electronic resonance condition to overcome the diffraction limit. By detuning the stimulated Raman pump to the electronic pre-resonance SRS (EPR-SRS) condition, Wei et al. (74) reported the detection sensitivity of 250 nM (30–50 molecules in focal volume) with an acquisition time of 1ms, a 1,000 times sensitivity increase compared to non-resonance SRS imaging. A significant improvement of spatial resolution was also reported by Bi et al. (75) as well. By doubling the frequencies of near-IR pump and Stokes lasers to visible lasers, they achieved a sub-diffraction spatial resolution of ~130 nm and 23-fold increase of sensitivity, whereas the typical lateral resolution of SRS is ~300 nm.
Efforts have also been made to increase imaging acquisition and hyperspectral scanning speeds. By replacing the lock-in amplifier with a gate array, lock-in free single-color line scan SRS imaging acquired 20 frames per second (76). Multiplex SRS imaging has been applied such that the spectrum at each pixel is captured simultaneously. Using a 16-channel tuned amplifier array could acquire a ~200 cm−1 spectrum in 32 µs (28). Using a 32-channel tuned amplifier array Zhang et al. (29) obtained an acquisition speed at 5 µs per spectrum (~200 cm−1). At 60 µs per spectrum for a 200 cm−1 spectral window, high speed imaging has been achieved at 15 cm−1 resolution (77). Later, Alshaykh et al. (78) used an acousto-optic filter and achieved ~12 µs for a ~200 cm−1 spectral window with a 25 cm−1 resolution.
In many cases, SRS microscopy may not be the only modality in the room. Some studies and operations require a multi-modal approach—incorporating various optical techniques such as CARS, SRS, second harmonic generation (SHG), and multi-photon fluorescence (MPF). These operations can all occur and be detected at the same time, but various disparities in signal intensity, laser power, and speed make these modes difficult to conjugate. By combining SRS with fluorescence microscopy, Xiong et al. (79,80) developed stimulated Raman excited fluorescence (SREF) microscopy and achieved superb sensitivity down to 10 nM (at single molecule level). This is performed by upconverting the EPR-SRS excited population to the electronic fluorescent state. A recent study demonstrated another multi-modal platform called “MANTIS”, capable of CRI as well as thermal imaging (81). They reported micron and millisecond spatiotemporal resolution using an SRS instrumentation sporting a galvo-scanner at 1 kHz, as opposed to the faster resonant scanner. Importantly, the MANTIS included both picosecond and femtosecond functionality, and could capture fast dynamic processes in vivo, with a resolution of 10 cm−1 using the picosecond laser. In general, there is a tradeoff between resolution and peak power when electing a picosecond or femtosecond laser, respectively.
Using a spectrally sliced broadband femtosecond pulsed laser, Francis et al. (82) conducted two-color SRS imaging of mouse tissues (brain, liver, kidney, and skin) ex vivo, to systematically investigate the effect of laser bandwidth on SNR and image contrast. They obtained a linear increase of SNR and a pulse bandwidth increase up to 40 cm−1 and maintained the same protein/lipid contrast as narrowband picosecond SRS.
There has been a recent push to apply these new technologies to previous experiments and delve deeper. The premise is primarily that of improved resolution for chemometric purposes such as analyte identification and diagnoses. Other driving motives include investigations in pharmacokinetics and targeted delivery of therapeutics, as well as the ever-present pursuit of understanding complex biological processes with minimal interference.
SRS imaging in tissues and animals for basic scientific research
Tumor biology
Hematoxylin and eosin (H&E) staining has been the “gold standard” for pathologies like cancer, yet it requires extensive time and labor, making it not ideal in the operation room where quick decisions must be made. Fast and accurate pathological diagnoses require real-time assessment of excised tissue. Two-color SRS microscopy has been used for virtual histology (called stimulated Raman histology, SRH) by imaging at 2,845 and 2,930 cm−1, respectively, for lipid and protein, and has achieved excellent results comparable to H&E staining (37,42). Ji et al. (37) conducted the first study to detect tumor infiltration in brain tissues ex vivo from human glioblastoma multiforme (GBM) xenograft mice and in fresh human brain tumor specimens. Lipid-rich white matter could be differentiated from the protein-rich cortex and tumor by the lipid and protein spectral intensities, and two-color SRS images could achieve comparable morphological contrast to H&E staining. Furthermore, they performed intraoperative SRS imaging of mice in vivo during surgery and discerned tumor margins that were undetectable with bright-field microscopy, which shows potential for improving accuracy in cancer surgery. In their following study (83), they performed quantitative SRS microscopy on brain tumor infiltration in fresh unprocessed surgical specimens from neurosurgery patients ex vivo, and demonstrated that SRS microscopy could detect tumor infiltration by revealing changes in tissue cellularity, axonal density, and protein/lipid ratios in tumor-filtered tissues (Figure 2). Spectral focusing techniques improved these label-free capabilities in a study that further distinguished the proneural and mesenchymal subtypes of GBM with specificity and sensitivity of 96.7% (29/30) and 77.8% (28/36) respectively (84). Lu et al. (42) compared large scale SRS imaging of brain tumor tissue resection from patients with H&E staining, and found that SRS microscopy could capture not only fundamental diagnostic hallmarks (such as cell nuclei and cell density) for tumor classification, but also features not detectable by H&E approaches including abundant lipid droplets within glioma cells, collagen deposition in gliosarcoma, and disruption of myelinated fibers.
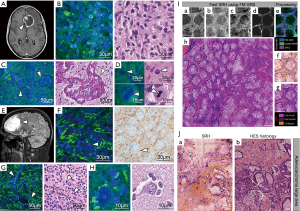
Conjugating SHG to visualize collagen contents is a popular means of achieving a third color. Sarri et al. (65) developed an imaging framework that combined SRS and SHG to produce SRH images of normal, colorectal, and pancreatic cancer tissues from human surgical specimens ex vivo (after surgery) (Figure 2). The generated SRH image was in excellent agreement with conventional hematoxylin, eosin, and saffron (HES) staining. Two novel SRS modalities were used in the study, λ-switch SRS and frequency modulated SRS (FM-SRS). Both achieved SRH images with 1 mm × 1 mm field of view at a dwell time 40 µs per pixel. The FM-SRS modality also allowed for instantaneous semantic segmentation, at the pixel level, of cell nuclei to afford HES-quality SRH images (Figure 2). These images were acquired and virtually stained in 25 minutes. Even though the need for staining chemicals is circumvented, label-free imaging speeds will still need to improve before SRH truly replaces conventional histology in many applications.
To complement the intraoperative acquisition speeds, intraoperative diagnostic speeds must also continue developing. While label-free SRH has proven comparable to traditional staining and analysis by pathologists, it often requires insightful knowledge of the subtle vibrational modes of various biochemical species. Deep learning algorithms such as CNN have been combined with SRH for rapid diagnosis (85,86). Deep learning removes the a priori requirement of manual feature selection and structured datasets to improve the classification potential of SRH, as well as increasing imaging depth. Zhang et al. (85) recently integrated deep learning CNN with SRH for rapid diagnosis of laryngeal squamous cell carcinoma (SCC). Comparing SRH images with H&E staining slides of frozen tissues, they reported a high concordance (Cohen’s score of κ >0.90). Further, using a residual CNN-based diagnosis of SRH images of fresh tissues, they obtained a 100% classification accuracy. Hollon et al. (86) also combined SRH with CNN-based deep learning for intraoperative brain tumor diagnosis, and achieved rapid prediction in near real-time.
While CNNs have great denoising and resolution restorative effects, they are traditionally employed for their advantageous 2D dataset applications, for which, volumetric 3D datasets qualify. However, processing hsSRS image datasets with smaller Raman cross sections require unique 3D CNN filters where instrumentation advances fall short. Most recently, Lin et al. (87) created an SRS imaging platform that achieved distortion-free SRS imaging in the fingerprint region with a resolution of 10 cm−1 and microsecond temporal resolution. The group applied their system to image whole mouse brain slices for cholesterol metabolism analysis—an important discussion in tumor biology. Hypercholesterolemia has been implicated in tumorigenesis in numerous ways, as reviewed by Ding et al. (88). For one, there is a positive correlation between increased serum cholesterol and a higher incidence of developing cancers. More importantly, compared to normal cells, cancer cells demonstrate higher cholesterol synthesis and uptake and lower cholesterol outflow. Therefore, SRS imaging holds great potential in tumor biology through its utilization in mapping cholesterol metabolism and distributions in the brain and other tissues throughout the body.
Neurodegenerative diseases
In addition to tumors, SRS imaging has been applied to detect a variety of brain diseases such as Alzheimer’s disease (AD) and amyotrophic lateral sclerosis (ALS). Two pathological hallmarks of AD are the extracellular plaque deposition of amyloid-β (Aβ) peptide and neurofibrillary tangles. Ji et al. (36) applied multicolor narrowband SRS microscopy to detect Aβ plaques in the brain tissue of APP:PS1 AD mouse model ex vivo (Figure 3). They differentiated misfolded Aβ plaques from normal proteins and lipids in both frozen and fresh brain tissues based on the spectral shift (~10 cm−1) of the amide I band of SRS spectra. This spectral shift is due to conformational change in the secondary structure of proteins, which leads to enriched contents of β sheets that resulting in changes in vibrational spectra. A broadband hsSRS microscopy with parabolic pulse amplification was recently developed and employed to image amyloid plaques in human brain tissue with AD disease (89). Compared to most existing hsSRS modalities (bandwidth 300 cm−1 and resolution 25 cm−1), this approach achieved a bandwidth over 600 cm−1 and resolution of 10 cm−1.
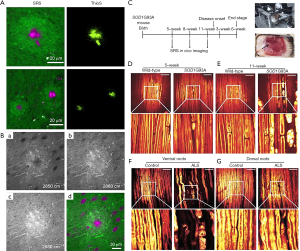
Tian et al. (41) used SRS microscopy to visualize peripheral degeneration in ALS mouse models and human postmortem tissue ex vivo. They found that the earliest detectable pathology in ALS mouse by SRS was peripheral nerve degeneration. By performing long-term serial in vivo SRS imaging, they also monitored disease progression and drug effects for ALS in living mice. The antibiotic minocycline was shown to significantly slow the peripheral nerve degeneration in ALS mice (Figure 3).
Basic neuroscience
Acetylcholine (ACh) is a neurotransmitter released by motor neurons to activate muscles and plays crucial roles in the central nervous system. It is highly concentrated in neuromuscular junctions. Fu et al. (67) reported the first label-free imaging of ACh in frog muscle ex vivo, by employing frequency-modulated spectral-focusing SRS microscopy, and using the vibrational signature of ACh at 720 cm−1 (intrinsic C-N bonds stretching). With this modality, the local concentration of ACh at the neuromuscular junction of frog cutaneous pectoris muscle was also quantified.
Cheng group reported real-time in vivo SRS imaging of the dynamic behaviors in the nervous system of living tadpoles, including myelination in a single Schwann cell, maturation of a node of Ranvier, and myelin degradation after nerve transaction (90). Using hsSRS imaging at the CH3 Fermi resonance peak, they visualized real-time neuronal depolarization of patched primary neurons in live mouse brain slices and detected single action potentials in neurons (40).
SRS imaging for clinics
For in vivo and in situ imaging of live animals and in the clinic, miniaturization of the SRS microscopy system is desired. The advancement of fiber-laser technology (91) allows for in vivo application of SRS microscopy in clinic. A fiber-delivered handheld SRS imaging system was reported (92) recently, and background-free in situ and in vivo imaging was achieved on normal and tumorigenic dog brain tissues, and on live human skin. SRS imaging methods offer substantial diagnostic performance increases compared to spontaneous fiber-delivered systems, which have already demonstrated successful performance on nasopharyngeal carcinoma (NPC) even without deep learning algorithms (93). However, it is still a challenge to achieve real-time SRS imaging for intraoperative assessment.
He et al. reported a parallel two-color SRS imaging design by using a dual-phase lock-in amplifier (94). This allows for real-time virtual histology and was validated by imaging mouse coronal tissue ex vivo, as well as in live zebrafish embryos and mouse ears in vivo. These were demonstrated in both transmissive and epi modes. Later that year, Orringer et al. (95) reported the first application of SRS microscopy in the operation room. They demonstrated the first intraoperative SRS imaging of fresh unprocessed brain tumor tissues from neurosurgical patients and achieved SRH images with high diagnostic accuracy compared with conventional H&E pathology. Based on quantified SRH images, a machine learning approach was also developed to differentiate brain tumors. The same group next applied SRH for intraoperative diagnosis of pediatric type brain tumors (96) and demonstrated how SRH could facilitate accurate brain tumor diagnosis while preserving the cytologic and histoarchitectural features of tumor specimens.
SRH has shown comparable accuracy with H&E staining for pathologic diagnosis of subjects with high cytoplasmic lipid content since the lipid signal is a primary source of contrast. However, it was unclear whether SRH is applicable to entities with less lipid contents such as in some tumor subtypes. Shin et al. (97) applied SRH for skull base tumors, which are more complex due to low lipid and high protein contents in the cytoplasm. By using two 90° phase shifted Stokes pulses for orthogonal lock-in detection, simultaneous two-channel SRS imaging was performed on fresh resected skull base tumor tissues from patients undergoing operation and SRH images were produced. In most cases, SRH images matched well with H&E staining results; however, not all tumor types were accurately diagnosed, poor diagnosis rates were shown for chondrosarcoma and schwannoma (0% and 44%, respectively), and the inter-pathologist reliability was consistently lower with SRH across the board. This may not only be attributed to the Raman active background from the proteinaceous microenvironment but may also be attributed to the nascency of the SRH method and recoloring scheme. For cases with limited lipid signals to provide enough contrast, pathologic diagnosis can be improved with pseudo-H&E recoloring using chemical information from SRS. Still, a benefit to SRH is that sectioning and conventional staining may still be performed later. As such, other non-destructive hyperspectral image (HSI) techniques may complement SRH and improve diagnostic agreement and accuracy.
Most recently, by combining hsSRS microscopy and SHG, Shin et al. (98) studied the association of breast calcifications with breast changes from benign to neoplastic, and revealed a close relationship of intra-patient calcification carbonate content with breast local malignancy (i.e., the carbonate content decreased as malignancy potential increased). SRS imaging was also applied to the field of plastic surgery for detecting silicone debris in tissues from breast implant capsules (99). SRS images of H&E stained slides were acquired at 2,905 cm−1 (silicone) and 2,933 cm−1 (surrounding tissue) for optimal contrast. Co-registered with bright-field images, SRS imaging could detect and quantify silicone debris in the tissue. Compared to current techniques (such as X-ray, atomic absorption spectroscopy, and IR spectroscopy) based on morphological features, SRS imaging provides a fast and convenient method with high molecular specificity and spatial resolution.
SRH products have recently emerged in the commercial space as well, like the NIO fiber laser SRS Imaging System by Invenio. This label-free system streamlines and standardizes fast biopsy squash-sample prep for SRH intraoperatively. A 16-megapixel image of a 3 mm × 3 mm biopsy can be acquired in 2–3 minutes, stored, recolored from the SRS images at protein and lipid Raman shifts in the C-H stretching region, and displayed on a high-resolution touchscreen. A continued effort in miniaturization and portability of SRS systems will pave the way for further commercialization of the promising technology for clinical use. Handheld spontaneous Raman devices abound but are more suited toward spectral acquisition than HSI acquisition. Currently, there are no handheld devices commercially available for large-scale tissue imaging in vivo. Label-free systems also suffer at the hands of reproducibility in diagnostic applications. A system that requires extensive training and supervision in each instance of use is not robust and inspires little value in a clinical setting. It is critical to centralize or standardize classification learners to interpret biological SRS data from new test sets with the same accuracy and precision as test sets derived from training set populations. The reproducibility and robustness of classification learners is often improved by labeling the tissues with Raman active tags or stable isotope probes (100), but there has also been recent development in transformation learners for image processing (101-103). The incorporation of tailored sample preparation and analytical methods are prominent research areas in Raman.
Labeled SRS imaging
Label-free SRS imaging of chemical bond vibration mainly focuses on the C-H stretching region (2,800–3,100 cm−1) due to the strong signals of lipids and proteins. However, these chemical bonds are usually shared by different endogenous molecules, which limits molecular specificity and sensitivity of label-free SRS imaging. A variety of special vibrational probes have been developed to replace the C-H bonds by other bonds (such as C-D, C-N) in molecules, with characteristic vibrational frequencies in the cell-silent region (1,800–2,800 cm−1). Raman probes include stable isotope tags, small molecules, and nanomaterials. Although the typical sensitivity of SRS imaging is at the mM level, labeled SRS imaging can not only enhance the visualization and spatial distribution of targeted biomolecules, but also further visualize the metabolic dynamics of labeled biomolecules that are impossible with label-free imaging. Coupling alkyne tags with SRS microscopy could obtain the detection sensitivity at about 15 mM for typical chemical bonds (such as C-H) and 200 µM (with pixel dwell time 100 µs) for alkyne bonds (51,52). The advances of labeled SRS imaging in tissues and animals are reviewed below according to the type of Raman labels.
Imaging using stable isotope tags
Stable isotopes (such as deuterium and 13C) have been widely used for imaging various biomolecules including fatty acids (FAs), amino acids, and glucose bio-orthogonally. For deuterium-labeling, certain C-H bonds in the molecule are replaced by C-D bonds, changing the corresponding characteristic frequencies from ~2,900 to ~2,100 cm−1 (falling in the cell-silent region), allowing SRS microscopy for metabolic imaging of a variety of biomolecules in tissues, organisms, and animals, in addition to live cell imaging.
Deuterium can be incorporated in many biomolecules of interest, either by the researcher to visualize a biomolecule’s fate and activity, or by the animal subject itself to visualize de novo synthesis. For instance, with deuterated (trimethyl-D9)-choline, the distribution of choline in live C. elegans during embryonic and early larval development was mapped (44). Most recently, Zhang et al. (46) reported an SRS microscopy technique, spectral tracing of deuterium (STRIDE), for tracing glucose metabolism by multichannel imaging of the dynamics of newly synthesized macromolecules (proteins, lipids, DNA, and glycogen). Using deuterium-labeled glucose (D7-glucose), STRIDE can distinguish the enrichment of C-D bonds and their spectral signatures in glucose-derived macromolecules. Through administration of D7-glucose in drinking water, glucose metabolism was visualized in brain and intestine tissues of neonatal mice during development, and in xenograft tumor mouse models during tumor progression (Figure 4).
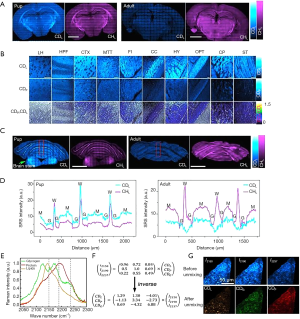
Coupling SRS microscopy with deuterated amino acids (dAAs), Wei et al. (104) developed a platform for labeling and imaging complex protein metabolism with high spatial and temporal resolution. By applying this platform, the newly synthesized proteins were successfully visualized in cultured mouse brain tissue, embryonic zebrafish, and live mouse liver and intestine tissues from mice that were administered dAA via drinking water (105). To enhance labeling efficacy and reduce possible organ bias, Shi et al. (45) further improved and optimized this technique by in vivo intra-carotid artery injection of dAA into mice. Intra-artery injection would quickly and more evenly distribute dAA into every organ with blood vessels. This fast delivery of dAA also allowed for time dependent imaging of the dynamics of protein synthesis. Using this method, they visualized newly synthesized proteins in more mouse organs (such as pancreas) and tissues (cerebral, cerebellar cortex, and hippocampus); they also imaged and quantified the newly synthesized protein in the choroid plexus and pancreas at different time points. Furthermore, the metabolic heterogeneity of protein synthesis in liver and xenograft colon tumor tissues was also observed, proving its potential for distinguishing tumors (Figure 5).
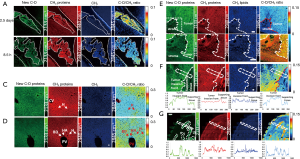
High throughput screening (HTS) has been frequently used for bioimaging, which combines the automated microscopy with image analysis. However, few studies have coupled HTS with SRS imaging. Recently, Yu et al. (106) integrated HTS with SRS microscopy to track lipid synthesis and mobilization in live C. elegans, which were fed with bacteria co-cultured with deuterated oleic acid. They revealed that bone morphogenetic protein signaling plays a crucial role in lipid metabolism.
In addition to the direct use of deuterated labels, Shi et al. (18) developed a novel platform that uses deuterium oxide (D2O) probing and SRS microscopy (DO-SRS) to image metabolic activities of lipids, proteins, and DNA in animals in situ. They visualized de novo lipogenesis in live C. elegans growing in D2O; further, by adding D2O in drinking water for mice, they imaged de novo lipogenesis and tracked de novo protein biosynthesis in situ without tissue bias in a variety of mouse organs such as the intestine, liver, pancreas, hippocampus, and cortex. More importantly, applying DO-SRS allows for simultaneous visualization of lipid and protein metabolism in animals, and for distinguishing tumor margins and metabolic heterogeneity by tumors’ inherently high metabolic activities (Figure 6). D2O probing proves more advantageous than deuterium-labeled carbon substrates in observing metabolic activities. It is nondestructive, does not perturb native metabolism in the host organism, and is a non-carbon tracer that allows for probing de novo biosynthesis. These advantages poise DO-SRS microscopy as an ideal and powerful imaging technique for development, aging, and cancer research. In addition, DO-SRS microscopy shows high sensitivity even at low D2O concentrations that are non-toxic to humans, which allows it to be potentially applied in clinic to track human metabolism.
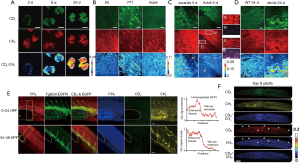
Carbon isotope 13C-labeling has also been applied for SRS imaging in tissues. Long et al. (107) recently reported a new two-color SRS imaging method, by synthesizing 13C-labeled 3-O-propargyl-D-glucose (3-OPG-13C) and combining it with deuterium-labeled glucose (D7-glucose), to study glucose uptake and incorporation activity simultaneously in mouse choroid plexus ex vivo. This newly designed 3-OPG-13C probe not only maintained the same features as the original 3-OPG (49) for visualizing glucose uptake, but more importantly displayed a new Raman frequency (at 2,053 cm−1) that can be spectrally resolved from the D7-glucose that is used for visualizing incorporation activity (at 2,133 cm−1).
Isotope-labeled FA probes have also been used to study metabolism in animals. Lipid signatures are critical to better understanding several pathologies including cancers and neurodegenerative diseases (88,108,109). From a metabolic perspective, however, certain isotope-labeled FAs have a lower rate of incorporation in the macromolecular biomass, and their Raman peaks are conserved in the cell and in solution (100). From a structural perspective, lipid probes may be used to study membrane dynamics, microenvironments, and much more. Isotope-labeled FAs are also incorporated through scavenger pathways, as they cannot freely diffuse through the cell membrane, and affect lipid metabolism uniquely as compared to DO-SRS (18). Other lipid probes are overviewed below.
Imaging using triple-bond based tags and small molecule probes
Albeit weakly detectable, stable isotope labels are small and bio-orthogonal making them ideal for imaging small and highly abundant biomolecules. Compared to isotope labels, triple-bond labels are larger in size but have stronger signals even at low concentration (47), allowing for SRS imaging of less abundant species with high sensitivity and specificity.
Among triple-bond tags, alkyne tags have been most widely used for SRS imaging of a broad range of biomolecules and drugs. Alkyne tags were first employed for spontaneous Raman microscopy by Yamakoshi (47,48), and were soon applied for SRS imaging. Wei et al. (51) and Hong et al. (52) both reported first applications of live-cell SRS imaging using alkyne tags in 2014. By using synthesized alkyne tagged glucose analogue 3-OPG and SRS imaging, Hu et al. (49) visualized glucose uptake in mouse subcutaneous tumor xenograft tissues and ex vivo mouse hippocampus tissues cultured with 3-OPG. Varied metabolic activities in tumors were discriminated, and the heterogeneity of glucose uptake in tumors and mouse hippocampus tissues were revealed. In their following study, Hu et al. (50) for the first time developed a platform that rationally integrated alkyne and deuterium labeling on the same tissue, and combined this platform with SRS microscopy for bio-orthogonal imaging of DNA, RNA, protein, and lipid metabolism in live rat hippocampal tissues under normal conditions as well as after a traumatic brain injury (TBI). Heterogeneous metabolic activities of these biomolecules were observed, and newly synthesized DNA during cell division was visualized inside the nucleus. In the TBI model, great increases in protein and lipid syntheses and metabolism were observed in the hilar region of the dentate gyrus.
Diyne tags have been developed by conjugating two triple bonds to further increase Raman signals. Lee et al. (110) designed a diyne tagged probe, phenyl-diyne cholesterol (PhDY-Chol), and coupled it with SRS imaging to observe cholesterol esterification, storage, and trafficking in live C. elegans (as well as in live cells). SRS imaging shows cholesterol uptake and storage in the intestinal cells of C. elegans.
Simultaneous visualization of many molecular species in situ is desired but remains challenging. Multiplex imaging can achieve no more than 9 colors simultaneously (1,53,54), even by using sophisticated fluorescence imaging instrumentation and complicated analysis. To overcome this barrier, Wei et al. (74) developed the EPR-SRS imaging technology, and created a vibrational palette for super-multiplex imaging by conjugating alkynes and nitriles to near-IR dyes, so-called MAnhattan Raman Scattering (MARS) dyes. They achieved 14 resolvable colors in the cell-silent Raman region, and combining it with 4 more fluorescent channels, obtained 24 resolvable colors. Employing this platform for imaging mouse organotypic cerebellar tissues ex vivo and hippocampal neuronal cultures, they revealed cell type-dependent heterogeneous DNA synthesis and protein metabolism. In another study, Hu et al. (111) developed a supermultiplexed palette based on polyyne, achieved 20 resolvable Raman frequencies (named Carbon rainbow, “Carbow”) in the cell-silent region, and displayed 10-color live-cell imaging. The recent developments and guidelines for future development of vibrational probes for super-multiplex SRS imaging have been timely reviewed and discussed in (112).
A variety of alkyne and diyne tags have been developed and employed for SRS imaging to track drug distribution and uptake in live cells, such as N-5-phenyl-2,4-pentadiyn-1-yl anisomycin (PhDY-ANS) and N-4-(4-phenyl-1,3-butadiyn-1-yl)benzyl anisomycin (BADY-ANS) (113) and diyne ferrostatin analog-2 (114). The applications of SRS imaging in drug delivery are reviewed in (115).
Imaging using nanomaterials
Polymer-based probes have shown great potential for SRS imaging with high stability and low cytotoxicity. Vanden-Hehir et al. (116) appended deuterium and alkyne labels to poly(lactic acid-co-glycolic acid) (PLGA) and synthesized them into nanoparticles (NPs) for SRS imaging. They showed that both NPs could be imaged in rat microglia, and alkyne tagged NPs could be visualized in ex vivo mouse cortical tissue. Hu et al. (55) developed a series of polymer dots by incorporating different vibrational tags (alkyne, nitrile, and carbon-deuterium) to styrene monomers for multiplexed live-cell imaging. Based on this study, Jin et al. (56) synthesized a variety of poly(methacrylate) beads (Raman beads) for multicolor SRS imaging, and successfully targeted tumors in living mice by injecting the Raman beads via the tail vein.
A water soluble and functionalizable master polydiacetylene, poly(deca-4,6-diynedioic acid) (PDDA), was reported most recently (57), and showed significant Raman signal enhancement (up to ~104 fold) compared with existing alkynes. By modifying the side chains of PDDA with functional Raman vibrational tags, a variety of polydiacetylene-based ultra-strong Raman-active nanomaterials could be obtained. Using the probes, hsSRS live-cell imaging was achieved with high spatiotemporal resolution at low laser power.
Volumetric 3D imaging of metabolic activities
The relationship between structure and function in a biological entity is clearer with volumetric 3D imaging. In this regard, greater imaging depth is desired for volumetric SRS imaging since imaging depth of SRS is usually around 100 µm in high scattering tissues and 300–500 µm in less scattering tissues (64). Most recently, Wei et al. (117) developed a tissue-clearing recipe (8 M urea with 0.2% Triton X-100) for SRS imaging and achieved an imaging depth of 10-fold increase (~1 mm) in mouse brain and tumor tissues. In addition, a volumetric phasor analysis method was developed for 3D clustering and segmentation and was coupled with multispectral SRS imaging to quantify the distributions of blood vessel, axon fibers, and cell bodies in a variety of mouse brain regions. Combining this phasor analysis method and metabolic D2O-SRS imaging, they successfully delineated tumor margins and revealed 3D structures of glioblastoma xenograft mouse brains (Figure 7).
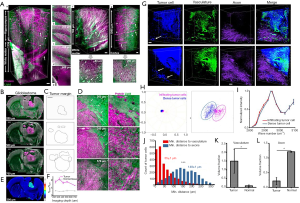
Another rapid tissue clearing method was also reported recently (118) that used formamide for volumetric SRS imaging. The imaging depth reached ~500 µm in mouse brain, kidney, liver and lung tissues. Furthermore, 3D histology-like images of brain tissues were obtained by applying two-color SRS, demonstrating the potential of using SRS based 3D tissue histopathology to improve diagnostic accuracy.
To image blood vessels, off-resonance signal detection at 2,700 cm−1 may be used. Suo et al. (119) used this Raman shift along with the typical protein and lipid C-H stretching channels to study the 3D structure of mouse lymph nodes. The group was able to visualize the capsule, subcapsular, and germinal center regions using SRS imaging.
Conclusion and outlook
The rapid growth of SRS microscopy imaging has seen numerous applications in life science and warrants continuing development. Improvements in SRS detection sensitivity and spatial resolution are always desired, but the path towards super-resolution SRS is long and rife with opportunities of exploration. Instrumentation advances in various filters, modulators, and amplifiers work tirelessly to improve the resolution of SRS imaging. Gong et al. (120) demonstrated a virtual sinusoidal modulation (VSM) as an experimental method for label-free far-field super-resolution saturated SRS (SSRS) in biological cells. Implementing this method in tissues and animals presents yet another exciting opportunity to explore the tremendous potential of SRS. Moreover, conjugation of SRS with other techniques such as fluorescence microscopy, photothermal microscopy (121), and atomic force microscopy (122) may also enhance sensitivity and spatial resolution, as well as improve diagnostic outcomes in clinical applications. These SRS platforms have afforded unique biomolecular insight in many fields such as tumor biology, neurodegenerative diseases, and more. Various labeling techniques have invaded the biomedical space as well. Isotopes, small molecules, and NPs have demonstrated comparable, and in some cases, exceptional utility when compared to other optical methods such as fluorescence imaging.
Downstream of the spectral acquisition, the processing of the acquired data is another developmental front. The large amount of Raman spectral data needs powerful analytical methods and tools for dimensionality reduction, clustering, and correlation (31). There are many multivariate analysis tools available such as principal component analysis, k-means clustering, spectral phasor analysis, support vector machines, etc. Machine learning will undoubtedly play an increasingly important role in these analyses.
For applications, SRS imaging has been coupled with HTS for imaging cells and multicellular organisms (106). Multiple platforms have been developed that couple HTS with Raman spectroscopy for cellomics, drug discovery, and more (123-126), which can also be translated to SRS. We envision tremendous demand for employing HTS-SRS in biological imaging in the years to come due to the convenience of reduced sample preparation, sample preservation, and demonstrated utility in diagnoses. Additionally, multicolor SRS imaging has been used to study NP behavior in cells. We believe by coupling small labels to nanocarriers, SRS microscopy will show huge potential in studying nanocarrier dynamics in living biological systems at subcellular levels. We anticipate more applications of SRS imaging, coupled with other technology and methods, in both basic scientific research and clinics very soon. While several handheld spontaneous Raman systems have emerged at diagnostic capacities in vivo, SRS in the clinic lags slightly. Since SRS has several advantages over spontaneous Raman in terms of imaging ability, to capitalize on this would mean the imaging area, depth, and topography of the subject pose additional hurtles. Fiber-delivered spontaneous Raman systems allow the direct spectral acquisition of tissues in vivo, however, to acquire an image would be much more difficult in vivo. The NIO laser (Invenio Inc.) used by Hollon et al. (86) requires sample excision, which is great at aiding tissue diagnosis in brain cancer, head and neck cancer, and others, but less suited for near-real time tumor boundary identification in vivo. The power of SRH in tissue delineation can be a powerful tool to aid surgical operations, but, the NIO imaging system is currently outfitted with deep learning diagnostic capabilities for research purposes only.
Novel approaches, such as laser source and probe development may allow for deeper SRS imaging capabilities, allowing for more SRH development in vivo. This is because certain wavelengths have demonstrated less scattering in biological tissue, such as the “golden window” between 1,600–1,870 nm (127). Most recently, a new nonlinear process, named enhanced stimulated Raman scattering (ESRS) was reported (128) from resonance Raman in β-carotene methanol solution, where enhanced stimulated Raman peaks were observed at the first and second Stokes. This ESRS effect is due to the energy transfer from excess vibrations of solute to the solvent. This novel process proposes a new potential approach for improving SRS signals in bioimaging.
Along with so many developments and exciting opportunities comes a counter-intuitive bottleneck. As many labs around the world begin to furnish their own Raman systems, the paucity of standardization in the name of ingenuity poses a cost-prohibitive battle for universal acceptance. SRS platforms are relatively expensive and may be outfitted with equipment from numerous suppliers. Custom parts and unique algorithms abound as well. We believe that although there is merit, the sheer diversity of SRS instrumentation, computational algorithms, analytical software, and experimental methodology slows the adoption of SRS as a universal tool. Perhaps with continued demonstration of analytical methods and more affordable instrumentation, SRS development might converge into a more combined effort.
Acknowledgments
Funding: LS acknowledges support from startup funds from UCSD, NIH U54 pilot (grant # CA132378), and 2U54CA132378-11A1.
Footnote
Provenance and Peer Review: With the arrangement by the Guest Editors and the editorial office, this article has been reviewed by external peers.
Conflicts of Interest: All authors have completed the ICMJE uniform disclosure form (available at http://dx.doi.org/10.21037/qims-20-712). The special issue “Advanced Optical Imaging in Biomedicine” was commissioned by the editorial office without any funding or sponsorship. The authors have no other conflicts of interest to declare.
Open Access Statement: This is an Open Access article distributed in accordance with the Creative Commons Attribution-NonCommercial-NoDerivs 4.0 International License (CC BY-NC-ND 4.0), which permits the non-commercial replication and distribution of the article with the strict proviso that no changes or edits are made and the original work is properly cited (including links to both the formal publication through the relevant DOI and the license). See: https://creativecommons.org/licenses/by-nc-nd/4.0/.
References
- Valm AM, Cohen S, Legant WR, Melunis J, Hershberg U, Wait E, Cohen AR, Davidson MW, Betzig E, Lippincott-Schwartz J. Applying systems-level spectral imaging and analysis to reveal the organelle interactome. Nature 2017;546:162-7. [Crossref] [PubMed]
- You S, Tu H, Chaney EJ, Sun Y, Zhao Y, Bower AJ, Liu YZ, Marjanovic M, Sinha S, Pu Y, Boppart SA. Intravital imaging by simultaneous label-free autofluorescence-multiharmonic microscopy. Nat Commun 2018;9:2125. [Crossref] [PubMed]
- Finlayson D, Rinaldi C, Baker MJ. Is Infrared Spectroscopy Ready for the Clinic? Anal Chem 2019;91:12117-28. [Crossref] [PubMed]
- Auner GW, Koya SK, Huang C, Broadbent B, Trexler M, Auner Z, Elias A, Mehne KC, Brusatori MA. Applications of Raman spectroscopy in cancer diagnosis. Cancer Metastasis Rev 2018;37:691-717. [Crossref] [PubMed]
- Kumamoto Y, Harada Y, Takamatsu T, Tanaka H. Label-free Molecular Imaging and Analysis by Raman Spectroscopy. Acta Histochem Cytochem 2018;51:101-10. [Crossref] [PubMed]
- Movasaghi Z, Rehman S, Rehman IU. Raman Spectroscopy of Biological Tissues. Appl Spectrosc Rev 2007;42:493-541. [Crossref]
- Kalkanis SN, Kast RE, Rosenblum ML, Mikkelsen T, Yurgelevic SM, Nelson KM, Raghunathan A, Poisson LM, Auner GW. Raman spectroscopy to distinguish grey matter, necrosis, and glioblastoma multiforme in frozen tissue sections. J Neurooncol 2014;116:477-85. [Crossref] [PubMed]
- Schie IW, Huser T. Methods and applications of Raman microspectroscopy to single-cell analysis. Appl Spectrosc 2013;67:813-28. [Crossref] [PubMed]
- Yue S, Cheng JX. Deciphering single cell metabolism by coherent Raman scattering microscopy. Curr Opin Chem Biol 2016;33:46-57. [Crossref] [PubMed]
- Woodbury EJ, Ng WK. Ruby laser operation in the near IR. Proc Inst Radio Eng 1962;50:2347-8.
- Owyoung A, Jones ED. Stimulated Raman spectroscopy using low-power cw lasers. Opt Lett 1977;1:152-4. [Crossref] [PubMed]
- Ploetz E, Laimgruber S, Berner S, Zinth W, Gilch P. Femtosecond stimulated Raman microscopy. Appl Phys B 2007;87:389-93. [Crossref]
- Freudiger CW, Min W, Saar BG, Lu S, Holtom GR, He C, Tsai JC, Kang JX, Xie XS. Label-free biomedical imaging with high sensitivity by stimulated Raman scattering microscopy. Science 2008;322:1857-61. [Crossref] [PubMed]
- Ozeki Y, Dake F, Kajiyama S, Fukui K, Itoh K. Analysis and experimental assessment of the sensitivity of stimulated Raman scattering microscopy. Opt Express 2009;17:3651-8. [Crossref] [PubMed]
- Nandakumar P, Kovalev A, Volkmer A. Vibrational imaging based on stimulated Raman scattering microscopy. New J Phys 2009;11:033026. [Crossref]
- Harzing AW. Publish or Perish. 2007. Available online: https://harzingcom/resources/publish-or-perish
- Min W, Freudiger CW, Lu S, Xie XS. Coherent nonlinear optical imaging: beyond fluorescence microscopy. Annu Rev Phys Chem 2011;62:507-30. [Crossref] [PubMed]
- Shi L, Zheng C, Shen Y, Chen Z, Silveira ES, Zhang L, Wei M, Liu C, de Sena-Tomas C, Targoff K, Min W. Optical imaging of metabolic dynamics in animals. Nat Commun 2018;9:2995. [Crossref] [PubMed]
- Chen AJ, Li J, Jannasch A, Mutlu AS, Wang MC, Cheng JX. Fingerprint Stimulated Raman Scattering Imaging Reveals Retinoid Coupling Lipid Metabolism and Survival. Chemphyschem 2018;19:2500-6. [Crossref] [PubMed]
- Saar BG, Freudiger CW, Reichman J, Stanley CM, Holtom GR, Xie XS. Video-rate molecular imaging in vivo with stimulated Raman scattering. Science 2010;330:1368-70. [Crossref] [PubMed]
- Fu D, Holtom G, Freudiger C, Zhang X, Xie XS. Hyperspectral imaging with stimulated Raman scattering by chirped femtosecond lasers. J Phys Chem B 2013;117:4634-40. [Crossref] [PubMed]
- He R, Liu Z, Xu Y, Huang W, Ma H, Ji M. Stimulated Raman scattering microscopy and spectroscopy with a rapid scanning optical delay line. Opt Lett 2017;42:659-62. [Crossref] [PubMed]
- Zhang D, Wang P, Slipchenko MN, Ben-Amotz D, Weiner AM, Cheng JX. Quantitative vibrational imaging by hyperspectral stimulated Raman scattering microscopy and multivariate curve resolution analysis. Anal Chem 2013;85:98-106. [Crossref] [PubMed]
- Ozeki Y, Umemura W, Sumimura K, Nishizawa N, Fukui K, Itoh K. Stimulated Raman hyperspectral imaging based on spectral filtering of broadband fiber laser pulses. Opt Lett 2012;37:431-3. [Crossref] [PubMed]
- Alfonso-García A, Mittal R, Lee ES, Potma EO. Biological imaging with coherent Raman scattering microscopy: a tutorial. J Biomed Opt 2014;19:71407. [Crossref] [PubMed]
- Cheng JX, Xie XS. Vibrational spectroscopic imaging of living systems: An emerging platform for biology and medicine. Science 2015;350:aaa8870. [Crossref] [PubMed]
- Fu D, Lu FK, Zhang X, Freudiger C, Pernik DR, Holtom G, Xie XS. Quantitative chemical imaging with multiplex stimulated Raman scattering microscopy. J Am Chem Soc 2012;134:3623-6. [Crossref] [PubMed]
- Liao CS, Slipchenko MN, Wang P, Li J, Lee SY, Oglesbee RA, Cheng JX. Microsecond scale vibrational spectroscopic imaging by multiplex stimulated Raman scattering microscopy. Light Sci Appl 2015;4:e265. [Crossref] [PubMed]
- Zhang C, Huang KC, Rajwa B, Li J, Yang S, Lin H, Liao CS, Eakins G, Kuang S, Patsekin V, Robinson JP, Cheng JX. Stimulated Raman scattering flow cytometry for label-free single-particle analysis. Optica 2017;4:103-9. [Crossref]
- Zhang D, Wang P, Slipchenko MN, Cheng JX. Fast vibrational imaging of single cells and tissues by stimulated Raman scattering microscopy. Acc Chem Res 2014;47:2282-90. [Crossref] [PubMed]
- Hu F, Shi L, Min W. Biological imaging of chemical bonds by stimulated Raman scattering microscopy. Nat Methods 2019;16:830-42. [Crossref] [PubMed]
- Shen Y, Hu F, Min W. Raman Imaging of Small Biomolecules. Annu Rev Biophys 2019;48:347-69. [Crossref] [PubMed]
- Fu D, Yu Y, Folick A, Currie E, Farese RV, Tsai T-H, Xie XS, Wang MC. In Vivo Metabolic Fingerprinting of Neutral Lipids with Hyperspectral Stimulated Raman Scattering Microscopy. J Am Chem Soc 2014;136:8820-8. [Crossref] [PubMed]
- Wang MC, Min W, Freudiger CW, Ruvkun G, Xie XS. RNAi screening for fat regulatory genes with SRS microscopy. Nat Methods 2011;8:135-8. [Crossref] [PubMed]
- Zhang C, Li J, Lan L, Cheng JX. Quantification of Lipid Metabolism in Living Cells through the Dynamics of Lipid Droplets Measured by Stimulated Raman Scattering Imaging. Anal Chem 2017;89:4502-7. [Crossref] [PubMed]
- Ji M, Arbel M, Zhang L, Freudiger CW, Hou SS, Lin D, Yang X, Bacskai BJ, Xie XS. Label-free imaging of amyloid plaques in Alzheimer's disease with stimulated Raman scattering microscopy. Sci Adv 2018;4:eaat7715. [Crossref] [PubMed]
- Ji M, Orringer DA, Freudiger CW, Ramkissoon S, Liu X, Lau D, Golby AJ, Norton I, Hayashi M, Agar NY, Young GS, Spino C, Santagata S, Camelo-Piragua S, Ligon KL, Sagher O, Xie XS. Rapid, label-free detection of brain tumors with stimulated Raman scattering microscopy. Sci Transl Med 2013;5:201ra119. [Crossref] [PubMed]
- Lu FK, Basu S, Igras V, Hoang MP, Ji M, Fu D, Holtom GR, Neel VA, Freudiger CW, Fisher DE, Xie XS. Label-free DNA imaging in vivo with stimulated Raman scattering microscopy. Proc Natl Acad Sci U S A 2015;112:11624-9. [Crossref] [PubMed]
- Wang P, Li J, Wang P, Hu CR, Zhang D, Sturek M, Cheng JX. Label-free quantitative imaging of cholesterol in intact tissues by hyperspectral stimulated Raman scattering microscopy. Angew Chem Int Ed Engl 2013;52:13042-6. [Crossref] [PubMed]
- Lee HJ, Zhang D, Jiang Y, Wu X, Shih PY, Liao CS, Bungart B, Xu XM, Drenan R, Bartlett E, Cheng JX. Label-free vibrational spectroscopic imaging of neuronal membrane potential. J Phys Chem Lett 2017;8:1932-6. [Crossref] [PubMed]
- Tian F, Yang W, Mordes DA, Wang JY, Salameh JS, Mok J, Chew J, Sharma A, Leno-Duran E, Suzuki-Uematsu S, Suzuki N, Han SS, Lu FK, Ji M, Zhang R, Liu Y, Strominger J, Shneider NA, Petrucelli L, Xie XS, Eggan K. Monitoring peripheral nerve degeneration in ALS by label-free stimulated Raman scattering imaging. Nat Commun 2016;7:13283. [Crossref] [PubMed]
- Lu FK, Calligaris D, Olubiyi OI, Norton I, Yang W, Santagata S, Xie XS, Golby AJ, Agar NY. Label-Free Neurosurgical Pathology with Stimulated Raman Imaging. Cancer Res 2016;76:3451-62. [Crossref] [PubMed]
- Egawa M, Iwanaga S, Hosoi J, Goto M, Yamanishi H, Miyai M, Katagiri C, Tokunaga K, Asai T, Ozeki Y. Label-free stimulated Raman scattering microscopy visualizes changes in intracellular morphology during human epidermal keratinocyte differentiation. Sci Rep 2019;9:12601. [Crossref] [PubMed]
- Hu F, Wei L, Zheng C, Shen Y, Min W. Live-cell vibrational imaging of choline metabolites by stimulated Raman scattering coupled with isotope-based metabolic labeling. Analyst 2014;139:2312-7. [Crossref] [PubMed]
- Shi L, Shen Y, Min W. Visualizing protein synthesis in mice with in vivo labeling of deuterated amino acids using vibrational imaging. APL Photonics 2018;3:092401. [Crossref]
- Zhang L, Shi L, Shen Y, Miao Y, Wei M, Qian N, Liu Y, Min W. Spectral tracing of deuterium for imaging glucose metabolism. Nat Biomed Eng 2019;3:402-13. [Crossref] [PubMed]
- Yamakoshi H, Dodo K, Okada M, Ando J, Palonpon A, Fujita K, Kawata S, Sodeoka M. Imaging of EdU, an alkyne-tagged cell proliferation probe, by Raman microscopy. J Am Chem Soc 2011;133:6102-5. [Crossref] [PubMed]
- Yamakoshi H, Dodo K, Palonpon A, Ando J, Fujita K, Kawata S, Sodeoka M. Alkyne-Tag Raman Imaging for Visualization of Mobile Small Molecules in Live Cells. J Am Chem Soc 2012;134:20681-9. [Crossref] [PubMed]
- Hu F, Chen Z, Zhang L, Shen Y, Wei L, Min W. Vibrational Imaging of Glucose Uptake Activity in Live Cells and Tissues by Stimulated Raman Scattering. Angew Chem Int Ed Engl 2015;54:9821-5. [Crossref] [PubMed]
- Hu F, Lamprecht MR, Wei L, Morrison B, Min W. Bioorthogonal chemical imaging of metabolic activities in live mammalian hippocampal tissues with stimulated Raman scattering. Sci Rep 2016;6:39660. [Crossref] [PubMed]
- Wei L, Hu F, Shen Y, Chen Z, Yu Y, Lin CC, Wang MC, Min W. Live-cell imaging of alkyne-tagged small biomolecules by stimulated Raman scattering. Nat Methods 2014;11:410-2. [Crossref] [PubMed]
- Hong S, Chen T, Zhu Y, Li A, Huang Y, Chen X. Live-cell stimulated Raman scattering imaging of alkyne-tagged biomolecules. Angew Chem Int Ed Engl 2014;53:5827-31. [Crossref] [PubMed]
- Tsurui H, Nishimura H, Hattori S, Hirose S, Okumura K, Shirai T. Seven-color fluorescence imaging of tissue samples based on Fourier spectroscopy and singular value decomposition. J Histochem Cytochem 2000;48:653-62. [Crossref] [PubMed]
- Niehörster T, Loschberger A, Gregor I, Kramer B, Rahn HJ, Patting M, Koberling F, Enderlein J, Sauer M. Multi-target spectrally resolved fluorescence lifetime imaging microscopy. Nat Methods 2016;13:257-62. [Crossref] [PubMed]
- Hu F, Brucks SD, Lambert TH, Campos LM, Min W. Stimulated Raman scattering of polymer nanoparticles for multiplexed live-cell imaging. Chem Commun (Camb) 2017;53:6187-90. [Crossref] [PubMed]
- Jin Q, Fan X, Chen C, Huang L, Wang J, Tang X. Multicolor Raman Beads for Multiplexed Tumor Cell and Tissue Imaging and in Vivo Tumor Spectral Detection. Anal Chem 2019;91:3784-9. [Crossref] [PubMed]
- Tian S, Li H, Li Z, Tang H, Yin M, Chen Y, Wang S, Gao Y, Yang X, Meng F, Lauher JW, Wang P, Luo L. Polydiacetylene-based ultrastrong bioorthogonal Raman probes for targeted live-cell Raman imaging. Nat Commun 2020;11:81. [Crossref] [PubMed]
- Hill AH, Fu D. Cellular Imaging Using Stimulated Raman Scattering Microscopy. Anal Chem 2019;91:9333-42. [Crossref] [PubMed]
- Lee HJ, Cheng JX. Imaging chemistry inside living cells by stimulated Raman scattering microscopy. Methods 2017;128:119-28. [Crossref] [PubMed]
- Le TT, Yue S, Cheng JX. Shedding new light on lipid biology with coherent anti-Stokes Raman scattering microscopy. J Lipid Res 2010;51:3091-102. [Crossref] [PubMed]
- Schie IW, Krafft C, Popp J. Applications of coherent Raman scattering microscopies to clinical and biological studies. Analyst 2015;140:3897-909. [Crossref] [PubMed]
- Cicerone MT, Camp CH. Histological coherent Raman imaging: a prognostic review. Analyst 2017;143:33-59. [Crossref] [PubMed]
- Camp CH Jr, Cicerone MT. Chemically sensitive bioimaging with coherent Raman scattering. Nat Photonics 2015;9:295-305. [Crossref]
- Hill AH, Manifold B, Fu D. Tissue imaging depth limit of stimulated Raman scattering microscopy. Biomed Opt Express 2020;11:762-74. [Crossref] [PubMed]
- Sarri B, Canonge R, Audier X, Simon E, Wojak J, Caillol F, Cador C, Marguet D, Poizat F, Giovannini M, Rigneault H. Fast stimulated Raman and second harmonic generation imaging for intraoperative gastro-intestinal cancer detection. Sci Rep 2019;9:10052. [Crossref] [PubMed]
- Yang W, Li A, Suo Y, Lu FK, Sunney Xie X. Simultaneous two-color stimulated Raman scattering microscopy by adding a fiber amplifier to a 2 ps OPO-based SRS microscope. Opt Lett 2017;42:523-6. [Crossref] [PubMed]
- Fu D, Yang W, Xie XS. Label-free Imaging of Neurotransmitter Acetylcholine at Neuromuscular Junctions with Stimulated Raman Scattering. J Am Chem Soc 2017;139:583-6. [Crossref] [PubMed]
- Hill AH, Munger E, Francis AT, Manifold B, Fu D. Frequency Modulation Stimulated Raman Scattering Microscopy through Polarization Encoding. J Phys Chem B 2019;123:8397-404. [Crossref] [PubMed]
- Xiong H, Qian N, Zhao Z, Shi L, Miao Y, Min W. Background-free imaging of chemical bonds by a simple and robust frequency-modulated stimulated Raman scattering microscopy. Opt Express 2020;28:15663-77. [Crossref] [PubMed]
- Hellerer T, Enejder AMK, Zumbusch A. Spectral focusing: High spectral resolution spectroscopy with broad-bandwidth laser pulses. Appl Phys Lett 2004;85:25-7. [Crossref]
- Weiner AM. Femtosecond optical pulse shaping and processing. Prog Quantum Electron 1995;19:161-237. [Crossref]
- Audier X, Forget N, Rigneault H. High-speed chemical imaging of dynamic and histological samples with stimulated Raman micro-spectroscopy. Opt Express 2020;28:15505-14. [Crossref] [PubMed]
- Ito T, Obara Y, Misawa K. Invited Article: Spectral focusing with asymmetric pulses for high-contrast pump–probe stimulated Raman scattering microscopy. APL Photonics 2018;3:092405. [Crossref]
- Wei L, Chen Z, Shi L, Long R, Anzalone AV, Zhang L, Hu F, Yuste R, Cornish VW, Min W. Super-multiplex vibrational imaging. Nature 2017;544:465-70. [Crossref] [PubMed]
- Bi Y, Yang C, Chen Y, Yan S, Yang G, Wu Y, Zhang G, Wang P. Near-resonance enhanced label-free stimulated Raman scattering microscopy with spatial resolution near 130 nm. Light Sci Appl 2018;7:81. [Crossref] [PubMed]
- Wang Z, Zheng W, Huang Z. Lock-in-detection-free line-scan stimulated Raman scattering microscopy for near video-rate Raman imaging. Opt Lett 2016;41:3960-3. [Crossref] [PubMed]
- Liao CS, Wang P, Wang P, Li J, Lee HJ, Eakins G, Cheng JX. Spectrometer-free vibrational imaging by retrieving stimulated Raman signal from highly scattered photons. Sci Adv 2015;1:e1500738. [Crossref] [PubMed]
- Alshaykh MS, Liao CS, Sandoval OE, Gitzinger G, Forget N, Leaird DE, Cheng JX, Weiner AM. High-speed stimulated hyperspectral Raman imaging using rapid acousto-optic delay lines. Opt Lett 2017;42:1548-51. [Crossref] [PubMed]
- Xiong H, Shi L, Wei L, Shen Y, Long R, Zhao Z, Min W. Stimulated Raman excited fluorescence spectroscopy and imaging. Nat Photonics 2019;13:412-7. [Crossref] [PubMed]
- Xiong H, Qian N, Miao Y, Zhao Z, Min W. Stimulated Raman Excited Fluorescence Spectroscopy of Visible Dyes. J Phys Chem Lett 2019;10:3563-70. [Crossref] [PubMed]
- Adams WR, Mehl B, Leiser E, Wang M, Patton S, Throckmorton GA, Jenkins JL, Ford JB, Gautam R, Brooker J, Jansen ED, Mahadevan-Jansen A. Multimodal Nonlinear Optical and Thermal Imaging Platform for Label-Free Characterization of Biological Tissue. bioRxiv 2020. doi: https://doi.org/. [Crossref]
- Francis A, Berry K, Chen Y, Figueroa B, Fu D. Label-free pathology by spectrally sliced femtosecond stimulated Raman scattering (SRS) microscopy. PLoS One 2017;12:e0178750. [Crossref] [PubMed]
- Ji M, Lewis S, Camelo-Piragua S, Ramkissoon SH, Snuderl M, Venneti S, Fisher-Hubbard A, Garrard M, Fu D, Wang AC, Heth JA, Maher CO, Sanai N, Johnson TD, Freudiger CW, Sagher O, Xie XS, Orringer DA. Detection of human brain tumor infiltration with quantitative stimulated Raman scattering microscopy. Sci Transl Med 2015;7:309ra163. [Crossref] [PubMed]
- Bae K, Zheng W, Lin K, Lim SW, Chong YK, Tang C, King NK, Ti Ang CB, Huang Z. Epi-Detected Hyperspectral Stimulated Raman Scattering Microscopy for Label-Free Molecular Subtyping of Glioblastomas. Anal Chem 2018;90:10249-55. [Crossref] [PubMed]
- Zhang L, Wu Y, Zheng B, Su L, Chen Y, Ma S, Hu Q, Zou X, Yao L, Yang Y, Chen L, Mao Y, Chen Y, Ji M. Rapid histology of laryngeal squamous cell carcinoma with deep-learning based stimulated Raman scattering microscopy. Theranostics 2019;9:2541-54. [Crossref] [PubMed]
- Hollon TC, Pandian B, Adapa AR, Urias E, Save AV, Khalsa SSS, Eichberg DG, D’Amico RS, Farooq ZU, Lewis S, Petridis PD, Marie T, Shah AH, Garton HJL, Maher CO, Heth JA, McKean EL, Sullivan SE, Hervey-Jumper SL, Patil PG, Thompson BG, Sagher O, McKhann GM, Komotar RJ, Ivan ME, Snuderl M, Otten ML, Johnson TD, Sisti MB, Bruce JN, Muraszko KM, Trautman J, Freudiger CW, Canoll P, Lee H, Camelo-Piragua S, Orringer DA. Near real-time intraoperative brain tumor diagnosis using stimulated Raman histology and deep neural networks. Nat Med 2020;26:52-8. [Crossref] [PubMed]
- Lin H, Lee HJ, Tague N, Lugagne JB, Zong C, Deng F, Wong W, Dunlop MJ, Cheng JX. Fingerprint Spectroscopic SRS Imaging of Single Living Cells and Whole Brain by Ultrafast Tuning and Spatial-Spectral Learning. arXiv: Medical Physics 2020:43.
- Ding X, Zhang W, Li S, Yang H. The role of cholesterol metabolism in cancer. Am J Cancer Res 2019;9:219-27. [PubMed]
- Figueroa B, Fu W, Nguyen T, Shin K, Manifold B, Wise F, Fu D. Broadband hyperspectral stimulated Raman scattering microscopy with a parabolic fiber amplifier source. Biomed Opt Express 2018;9:6116-31. [Crossref] [PubMed]
- Hu CR, Zhang D, Slipchenko MN, Cheng JX, Hu B. Label-free real-time imaging of myelination in the Xenopus laevis tadpole by in vivo stimulated Raman scattering microscopy. J Biomed Opt 2014;19:086005. [Crossref] [PubMed]
- Freudiger CW, Yang W, Holtom GR, Peyghambarian N, Xie XS, Kieu KQ. Stimulated Raman Scattering Microscopy with a Robust Fibre Laser Source. Nat Photonics 2014;8:153-9. [Crossref] [PubMed]
- Liao CS, Wang P, Huang CY, Lin P, Eakins G, Bentley RT, Liang R, Cheng JX. In Vivo and in Situ Spectroscopic Imaging by a Handheld Stimulated Raman Scattering Microscope. ACS Photonics 2018;5:947-54. [Crossref]
- Žuvela P, Lin K, Shu C, Zheng W, Lim CM, Huang Z. Fiber-Optic Raman Spectroscopy with Nature-Inspired Genetic Algorithms Enhances Real-Time in Vivo Detection and Diagnosis of Nasopharyngeal Carcinoma. Anal Chem 2019;91:8101-8. [Crossref] [PubMed]
- He R, Xu Y, Zhang L, Ma S, Wang X, Ye D, Ji M. Dual-phase stimulated Raman scattering microscopy for real-time two-color imaging. Optica 2017;4:44-7. [Crossref]
- Orringer DA, Pandian B, Niknafs YS, Hollon TC, Boyle J, Lewis S, Garrard M, Hervey-Jumper SL, Garton HJL, Maher CO, Heth JA, Sagher O, Wilkinson DA, Snuderl M, Venneti S, Ramkissoon SH, McFadden KA, Fisher-Hubbard A, Lieberman AP, Johnson TD, Xie XS, Trautman JK, Freudiger CW, Camelo-Piragua S. Rapid intraoperative histology of unprocessed surgical specimens via fibre-laser-based stimulated Raman scattering microscopy. Nat Biomed Eng 2017;1:0027.
- Hollon TC, Lewis S, Pandian B, Niknafs YS, Garrard MR, Garton H, Maher CO, McFadden K, Snuderl M, Lieberman AP, Muraszko K, Camelo-Piragua S, Orringer DA. Rapid Intraoperative Diagnosis of Pediatric Brain Tumors Using Stimulated Raman Histology. Cancer Res 2018;78:278-89. [Crossref] [PubMed]
- Shin KS, Francis AT, Hill AH, Laohajaratsang M, Cimino PJ, Latimer CS, Gonzalez-Cuyar LF, Sekhar LN, Juric-Sekhar G, Fu D. Intraoperative assessment of skull base tumors using stimulated Raman scattering microscopy. Sci Rep 2019;9:20392. [Crossref] [PubMed]
- Shin KS, Laohajaratsang M, Men S, Figueroa B, Dintzis SM, Fu D. Quantitative chemical imaging of breast calcifications in association with neoplastic processes. Theranostics 2020;10:5865-78. [Crossref] [PubMed]
- van Haasterecht L, Zada L, Schmidt RW, de Bakker E, Barbe E, Leslie HA, Vethaak AD, Gibbs S, de Boer JF, Niessen FB, van Zuijlen PPM, Groot ML, Ariese F. Label-free stimulated Raman scattering imaging reveals silicone breast implant material in tissue. J Biophotonics 2020;13:e201960197. [Crossref] [PubMed]
- Zhao Z, Chen C, Xiong H, Ji J, Min W. Metabolic Activity Phenotyping of Single Cells with Multiplexed Vibrational Probes. Anal Chem 2020;92:9603-12. [Crossref] [PubMed]
- He J, Zhao L, Yang H, Zhang M, Li W. HSI-BERT: Hyperspectral Image Classification Using the Bidirectional Encoder Representation From Transformers. IEEE Transactions on Geoscience and Remote Sensing 2020;58:165-78. [Crossref]
- Mei X, Pan E, Ma Y, Dai X, Huang J, Fan F, Du Q, Zheng H, Ma J. Spectral-Spatial Attention Networks for Hyperspectral Image Classification. Remote Sens 2019;11:963. [Crossref]
- He X, Chen Y. Optimized Input for CNN-Based Hyperspectral Image Classification Using Spatial Transformer Network. IEEE Geoscience and Remote Sensing Letters 2019;16:1884-8. [Crossref]
- Wei L, Yu Y, Shen Y, Wang MC, Min W. Vibrational imaging of newly synthesized proteins in live cells by stimulated Raman scattering microscopy. Proc Natl Acad Sci U S A 2013;110:11226-31. [Crossref] [PubMed]
- Wei L, Shen Y, Xu F, Hu F, Harrington JK, Targoff KL, Min W. Imaging complex protein metabolism in live organisms by stimulated Raman scattering microscopy with isotope labeling. ACS Chem Biol 2015;10:901-8. [Crossref] [PubMed]
- Yu Y, Mutlu AS, Liu H, Wang MC. High-throughput screens using photo-highlighting discover BMP signaling in mitochondrial lipid oxidation. Nat Commun 2017;8:865. [Crossref] [PubMed]
- Long R, Zhang L, Shi L, Shen Y, Hu F, Zeng C, Min W. Two-color vibrational imaging of glucose metabolism using stimulated Raman scattering. Chem Commun (Camb) 2018;54:152-5. [Crossref] [PubMed]
- Yi M, Li J, Chen S, Cai J, Ban Y, Peng Q, Zhou Y, Zeng Z, Peng S, Li X, Xiong W, Li G, Xiang B. Emerging role of lipid metabolism alterations in Cancer stem cells. J Exp Clin Cancer Res 2018;37:118. [Crossref] [PubMed]
- Bukiya AN, Blank PS, Rosenhouse-Dantsker A. Cholesterol intake and statin use regulate neuronal G protein-gated inwardly rectifying potassium channels. J Lipid Res 2019;60:19-29. [Crossref] [PubMed]
- Lee HJ, Zhang W, Zhang D, Yang Y, Liu B, Barker EL, Buhman KK, Slipchenko LV, Dai M, Cheng JX. Assessing cholesterol storage in live cells and C. elegans by stimulated Raman scattering imaging of phenyl-Diyne cholesterol. Sci Rep 2015;5:7930. [Crossref] [PubMed]
- Hu F, Zeng C, Long R, Miao Y, Wei L, Xu Q, Min W. Supermultiplexed optical imaging and barcoding with engineered polyynes. Nat Methods 2018;15:194-200. [Crossref] [PubMed]
- Miao Y, Shi L, Hu F, Min W. Probe design for super-multiplexed vibrational imaging. Phys Biol 2019;16:041003. [Crossref] [PubMed]
- Tipping WJ, Lee M, Serrels A, Brunton VG, Hulme AN. Imaging drug uptake by bioorthogonal stimulated Raman scattering microscopy. Chem Sci 2017;8:5606-15. [Crossref] [PubMed]
- Gaschler MM, Hu F, Feng H, Linkermann A, Min W, Stockwell BR. Determination of the Subcellular Localization and Mechanism of Action of Ferrostatins in Suppressing Ferroptosis. ACS Chem Biol 2018;13:1013-20. [Crossref] [PubMed]
- Tipping WJ, Lee M, Serrels A, Brunton VG, Hulme AN. Stimulated Raman scattering microscopy: an emerging tool for drug discovery. Chem Soc Rev 2016;45:2075-89. [Crossref] [PubMed]
- Vanden-Hehir S, Cairns SA, Lee M, Zoupi L, Shaver MP, Brunton VG, Williams A, Hulme AN. Alkyne-Tagged PLGA Allows Direct Visualization of Nanoparticles In Vitro and Ex Vivo by Stimulated Raman Scattering Microscopy. Biomacromolecules 2019;20:4008-14. [Crossref] [PubMed]
- Wei M, Shi L, Shen Y, Zhao Z, Guzman A, Kaufman LJ, Wei L, Min W. Volumetric chemical imaging by clearing-enhanced stimulated Raman scattering microscopy. Proc Natl Acad Sci U S A 2019;116:6608-17. [Crossref] [PubMed]
- Li J, Lin P, Tan Y, Cheng JX. Volumetric stimulated Raman scattering imaging of cleared tissues towards three-dimensional chemical histopathology. Biomed Opt Express 2019;10:4329-39. [Crossref] [PubMed]
- Suo Y, Yang W, Lu F, Xie XS. editors. Label-free imaging of lymph nodes with stimulated Raman scattering microscopy. Proc. SPIE 11190, Optics in Health Care and Biomedical Optics IX, 111900J. SPIE, 2019.
- Gong L, Zheng W, Ma Y, Huang Z. Saturated Stimulated-Raman-Scattering Microscopy for Far-Field Superresolution Vibrational Imaging. Phys Rev Applied 2019;11:034041. [Crossref]
- Zhang D, Li C, Zhang C, Slipchenko MN, Eakins G, Cheng JX. Depth-resolved mid-infrared photothermal imaging of living cells and organisms with submicrometer spatial resolution. Sci Adv 2016;2:e1600521. [Crossref] [PubMed]
- Dufrêne YF, Ando T, Garcia R, Alsteens D, Martinez-Martin D, Engel A, Gerber C, Muller DJ. Imaging modes of atomic force microscopy for application in molecular and cell biology. Nat Nanotechnol 2017;12:295-307. [Crossref] [PubMed]
- Medipally DK, Maguire A, Bryant J, Armstrong J, Dunne M, Finn M, Lyng FM, Meade AD. Development of a high throughput (HT) Raman spectroscopy method for rapid screening of liquid blood plasma from prostate cancer patients. Analyst 2017;142:1216-26. [Crossref] [PubMed]
- Schie IW, Ruger J, Mondol AS, Ramoji A, Neugebauer U, Krafft C, Popp J. High-Throughput Screening Raman Spectroscopy Platform for Label-Free Cellomics. Anal Chem 2018;90:2023-30. [Crossref] [PubMed]
- Mondol AS, Patel MD, Rüger J, Stiebing C, Kleiber A, Henkel T, Popp J, Schie IW. Application of High-Throughput Screening Raman Spectroscopy (HTS-RS) for Label-Free Identification and Molecular Characterization of Pollen. Sensors (Basel) 2019;19:4428. [Crossref] [PubMed]
- Chen X, Stoneburner K, Ladika M, Kuo TC, Kalantar TH. High-Throughput Raman Spectroscopy Screening of Excipients for the Stabilization of Amorphous Drugs. Appl Spectrosc 2015;69:1271-80. [Crossref] [PubMed]
- Shi L, Sordillo LA, Rodríguez-Contreras A, Alfano R. Transmission in near-infrared optical windows for deep brain imaging. J Biophotonics 2016;9:38-43. [Crossref] [PubMed]
- Shi L, Gayen T, Budansky Y, Yoo K, Secor J, Harvey T, Harvey G, Shumyatsky P, Nolan D, Alfano R. Enhanced stimulated raman scattering of solvent due to anharmonic energy transfer from resonance raman solute molecules. Opt Express 2020;28:21792-804. [Crossref] [PubMed]