Real-time three-dimensional histology-like imaging by label-free nonlinear optical microscopy
Introduction
Conventional formalin-fixed and paraffin-embedded (FFPE) histology has been broadly accepted for decades as the gold standard for cancer diagnostics. Despite the evident contrast provided by hematoxylin and eosin (H&E) and immunohistochemistry staining, there are several major limitations to the current gold-standard FFPE histology. First, the histological processing is labor-intensive and cost-ineffective. In the U.S. alone, FFPE histopathology involves around 27,000 histotechnicians and histotechnologists to produce 40 million tissue blocks annually, and approximately 15,000 pathologists using histological slides for diagnosis (1). Second, the complicated tissue processing procedures, such as fixation, sectioning, staining, and slide making, consume a substantial amount of time (on the order of days), potentially causing delays in cancer diagnosis (2,3).
In particular, the FFPE histological treatment of tissue often causes distortion artifacts and the loss of in vivo biological information (3), making it difficult for accurate 3D image reconstruction and real-time in vivo tissue monitoring (4). Compared to conventional cancer diagnoses using FFPE histology, the 3D volumetric imaging of intact cancer tissues can potentially help pathologists determine the size, dimensions, and margins of the tumor in a more straightforward manner. Moreover, in vivo information and dynamic events in cancer tissues, such as the distribution and dynamics of extracellular vesicles (5-7), metabolic activities (8-10), and immune cell migration (11), could reveal cancer aggressiveness and progression at earlier stages, prior to morphological changes in histologically-recognized features (5), thus increasing diagnostic sensitivity and accuracy.
Aiming to improve conventional FFPE histopathology, various optical imaging methods have been explored. Performing microscopy with ultraviolet surface excitation (MUSE) improved the efficiency of FFPE histology by using fluorescent dyes to label fresh or formalin-fixed tissues (12). With a similar optical spectrum, ultraviolet (UV) photoacoustic microscopy (PAM) realized label-free multilayered histology-like imaging, but with a poor acoustic axial resolution of 48 µm (13). However, because of the phototoxicity of the UV light, these two methods cannot be used for real-time monitoring of in vivo biological events. With the help of ultrafast pulsed laser sources, nonlinear optical imaging methods, such as multi-harmonic generation (2), multiphoton fluorescence (2), and coherent Raman scattering (CRS) (14), have also been extensively studied to provide endogenous contrasts of cancer tissues for virtual histology staining. Another approach toward virtual histology is by training a deep neural network to identify the color correlation between grey-scale autofluorescence images and the strictly co-localized FFPE histology (15). This method produces virtual histology images that closely resemble the corresponding real histology. However, this method has not been applied for fresh tissue samples due to the requirement for tissue sectioning and slide making. Therefore, none of these methods have achieved real-time histology-like tissue monitoring and 3D imaging at the same time.
In this study, we employ an advanced imaging technique, simultaneous label-free autofluorescence multiharmonic (SLAM) microscopy (11), to realize real-time 3D histology-like imaging of fresh untreated, unstained tissues. The correlations between histology and SLAM images demonstrate the capability of SLAM microscopy for visualizing essential histopathological tissue features. A deep neural network is trained using SLAM image data to segment the nuclei in SLAM microscopy images. The segmented tissue features were color-transformed to have H&E histology-like contrast. The achieved real-time 3D histology-like imaging can be developed as a potential tool to complement current FFPE histology for future studies in cancer detection and diagnosis.
Methods
Simultaneous label-free autofluorescence multiharmonic (SLAM) microscopy
A custom-built SLAM microscope integrating SHG, 2PF, THG, and 3PF imaging modalities was used in this study (3,6,9,11). These four modalities can visualize different biological tissue features based on their nonlinear optical signatures. For instance, 2PF and 3PF signals come from specific autofluorescence molecules, predominantly flavin adenine dinucleotide (FAD) with 2PF and nicotinamide adenine dinucleotide (NADH) with 3PF (16,17). In addition, SHG and THG signals come from certain nanoscale and microscale structures in biological tissues, such as periodic collagen fibers (18) and optical interfaces between different refractive indices, such as those formed by lipids and water (19). The visualized tissue features by the four modalities are summarized in Table 1.
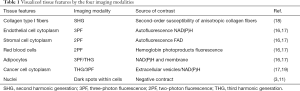
Full table
These four imaging modalities were simultaneously excited by a custom-developed programmable laser source. As shown in Figure 1A, the programmable laser source was realized by the combination of a pump laser, a photonic crystal fiber (PCF) and a pulse shaper. The original pump laser pulses (Satsuma, Amplitude Systemes SA) were spectrally broadened by passing through the PCF to become a supercontinuum laser source. A part of the supercontinuum spectrum from 1,080 to 1,140 nm was chosen and temporally compressed by a pulse shaper (MIIPS Box640, BioPhotonic Solutions Inc.) (11). This excitation source of approximately 10 mW was sent into a laser-scanning inverted microscope equipped with a broadband high-numerical-aperture (NA=1.05) objective lens (XLPLN25XWMP2, Olympus). The mixed emission signals generated from the four imaging modalities were isolated and filtered by a set of dichroic mirrors and bandpass filters, whose parameters are shown in Table 2. Four photomultiplier tubes (PMTs, H7421-40, Hamamatsu K.K.) were used to detect the imaging signals simultaneously. The SLAM microscope was first set up on an optical table in the laboratory environment (Figure 1B) to reach optimal imaging performance, and was employed in the design of a portable imaging system capable of conducting intraoperative imaging of fresh ex vivo human samples in the operating room (Figure 1C) (6). As shown in Figure 1D, the acquired SLAM image of rat mammary tissue is presented by the composite of the four imaging modalities assigned with different colors, which approximately correspond to the emission wavelengths. The colors assigned to the imaging modalities are yellow for 2PF, green for SHG, cyan for 3PF, and magenta for THG. Several tissue features can be easily identified from Figure 1D, such as the NADH-rich cyan adipocytes, FAD-rich yellow stromal cells, magenta-colored adipocytes membranes, and green collagen fibers.
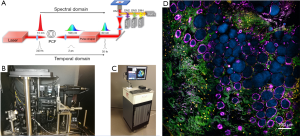
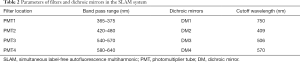
Full table
Ex vivo and in vivo imaging of human and rat tissues
This study of human tissue imaging was conducted under a protocol approved by the institutional review boards of Carle Foundation Hospital and the University of Illinois at Urbana-Champaign (No: 18CCC1708) and informed consent was acquired from the human subjects who participated in this study. A portable SLAM imaging system (Figure 1C) was developed to conduct imaging in the operating room during breast cancer surgeries (6). Immediately after surgical removal, resected tissue specimens were directly transferred to the imaging system for ex vivo imaging, prior to any fixation or treatment. After 20 minutes of imaging, the tissue specimens were immersed in saline and sent to the pathology laboratory. In the pathology lab, specimens were further sectioned grossly for standard histological processing, and excess tissues were made available to the research team for additional imaging experiments. The excess tissues were again immersed in saline before being imaged by the bench-top SLAM imaging system. The time interval between surgical tissue removal and the bench-top SLAM imaging was kept within 2 hours to avoid severe tissue perturbation. Imaged tissue sites were marked with surgical ink, prepared for standard FFPE histology, and used for later correlations.
In vivo rat tissue imaging was conducted under a protocol approved by the Institutional Animal Care and Use Committee at the University of Illinois at Urbana-Champaign (No: 18043) (11). Mammary tumors were induced in female Wistar-Furth rats (Harlan, IN, USA) by injecting diluted NMU (N-Nitroso-N-methylurea) (Sigma, St. Louis, MO, USA) at a concentration of 55 mg·kg−1 (11). The in vivo imaging experiments were carried out in rats approximately 4-6 weeks later when mammary tumors became palpable. For in vivo imaging, animals were anesthetized with 1% isoflurane mixed with O2 (1 L·min−1 flow rate). The skin of the animals was surgically opened and reflected back for imaging, and images were collected from the mammary tissue attached to the inner side of the reflected skin. The time spent for the imaging procedures was less than 3 hours. After imaging, rats were euthanized. The imaged tissue sites were resected for standard FFPE histology and later correlations.
Nuclei segmentation by deep learning
As an essential tissue feature for cancer diagnosis, nuclei are effectively visualized using SLAM microscopy via negative contrast against the autofluorescent cytoplasm (3,6,11). The cytoplasm appears bright in 2PF, 3PF, and THG images due to the nonlinear optical signals generated from the intracellular molecules and structures. Although the nuclei from the SLAM images in Figures 1D,2A can be readily identified by the human eye, they still need to be computationally recognized and segmented before virtual H&E staining due to the lack of positive contrast.
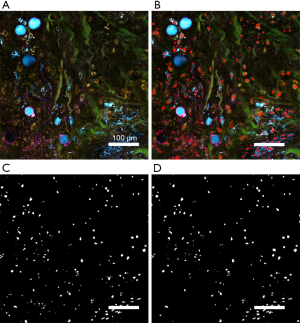
Because of the limited image signal-to-noise ratio (SNR) and the heterogeneity in shape and size of the nuclei, conventional algorithms of feature extraction and segmentation cannot accurately segment the nuclei from SLAM images. To achieve a higher performance, we developed a deep neural network (DNN) for nuclei segmentation (You et al., Label-free deep profiling of the tumor microenvironment. To be submitted). The DNN architecture was based on ResNet-2019 and U-Net20 (20,21). We acquired a large volume of SLAM image data (~300 Gigabytes) that was sufficient to be partitioned to training, validation, and test datasets. The DNN was trained by the four-channel SLAM images and nuclei labels. The nuclei labels were manually segmented from the SLAM images, serving as the ground truth for the training data. The parameters for DNN training followed our previously to-be-submitted work in cell segmentation (You et al., Label-free deep profiling of the tumor microenvironment. To be submitted). After training, the optimal model was chosen by evaluating the loss function of image segmentation using the validation dataset. Finally, the trained DNN was used to segment nuclei in the SLAM images from the test dataset. As shown in Figure 2B, the red shapes overlaid on the original SLAM image represent the nuclei segmented by the trained DNN. Furthermore, the DNN-segmented nuclei mask in Figure 2C was compared to the manually segmented ground truth (Figure 2D). From the comparison, it is noticed that the DNN-segmented nuclei mask included some small dark spots in the lower left corner as false-positive nuclei. The quantitative analysis yields a pixel-by-pixel false positive rate of 8% and a false negative rate of 4%. Quantitative analysis of nuclei segmentation quality was not performed on all the image data included in this study due to the labor-intensive producing of ground truth by manual segmentation.
In practice, the performance of the deep-learning-based segmentation algorithm varies among different SLAM images. For the images collected from deeper tissue regions, the deep-learning-based segmentation does not work as well due to the low image SNR. For instance, the performance of the deep-learning-based nuclei segmentation in rat mammary tissues starts to decrease beyond the imaging depth of around 100 µm. Therefore, to compensate for this, we also manually segment nuclei from those images where deep-learning-based segmentation does not perform well, especially the images with relatively low SNR.
Virtual H&E staining by colormap conversion
The formation of SLAM microscopy images relies on the generation of nonlinear optical signals from the tissue sample, so gray-scale digital images were produced where samples appear bright in contrast to a dark background. The recorded intensity values in each SLAM image channel are related to the concentrations of the targeted molecules or structures. On the other hand, the FFPE histology slides are typically observed under a bright-field illuminated microscope. Therefore, the background of H&E histology images is bright, while the tissue components are purple- and pink-colored because of the light absorption by the staining agents. As previously studied (2), the relation between the concentration of N types of staining agents ni (i=1, …, N) and the intensity of the H&E histology image Ix at a specific color channel x is expressed as:
[1]
where I0 is the wavelength-independent intensity from the illumination and σx,i is the absorption cross-section of the ith staining agent at the color channel x. As discussed above, the SLAM image intensity is related to the concentration of the tissue features that are approximately proportional to the concentration of the staining agents. Therefore, we can use the intensity of a SLAM image to express the staining concentration ni. Taking into account the quadratic and cubic power dependence of the nonlinear optical imaging modalities and the effects of detection loss, Eq. [1] can be rewritten in terms of the SLAM imaging signal intensity ISLAM,i:
[2]
where ki is the factor to represent the detection loss and mi is the power of the nonlinear dependence of the concentration ni on the SLAM imaging intensity ISLAM,i.
In H&E-stained histology, hematoxylin (H) and eosin (E) are used as the two staining agents. If we consider the red (R), green (G), and blue (B) channels (2), which are the basic display colors in most digital screens, Eq. [2] can be expanded to be:
[3]
[4]
[5]
The RGB values of σ are determined by the RGB decomposition of the color of hematoxylin and eosin in H&E histology images.
Hematoxylin primarily stains the DNA in nuclei. Shown as the dark voids in SLAM microscopy images, the nuclei are segmented based on their morphological shape and the negative contrast formed against the bright cytoplasm, but not the DNA within the nuclei. As a result, the SLAM image intensity
Another staining agent in H&E histology, eosin, is mostly bound to collagen fibers. The collagen fibers are present in the SHG imaging channel with a positive contrast. Therefore, the virtual staining of collagen fibers can be easily done by substituting the SHG imaging intensity
[6]
[7]
[8]
Figure 3 demonstrates the image processing procedure for producing histology-like images from the composite SLAM images. From the original SLAM image (Figure 3A), the essential tissue features of collagen fibers, nuclei, and red blood cells were segmented (Figure 3B). By creating RGB-colored images based on the intensities in Eqs. [6-8], the color-converted tissue features under virtual H&E staining are separately shown in Figure 3C, and the composite histology-like image combining these three essential tissue features is shown in Figure 3D.
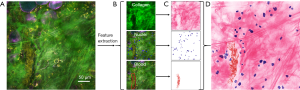
Analysis of nuclear-cytoplasmic ratio
Typically, a high N/C ratio indicates a high likelihood of malignancy (22). As a well-defined diagnostic measure in cancer, the N/C ratio of the cells within tissue samples was quantified from the SLAM imaging data. First, cells were manually segmented to determine the area of cytoplasm. Next, each cell was co-located with its corresponding nuclei. Finally, the N/C ratio was therefore calculated by quantifying the number of pixels within the nucleus and cytoplasm of each cell using a MATLAB program. A total of 61 normal stromal cells and 55 cancer cells from a rat mammary tumor were included to represent this analysis. Following this analysis, and for visualization, the boundaries of the cells were delineated around the nuclei. To indicate the N/C ratio of each cell, the delineated cell boundaries were subsequently colored according to the value of the calculated N/C ratio. The color scale of the cell boundaries is based on the “jet” colormap in MATLAB, with red indicating a high N/C ratio and blue indicating a low ratio. The exact imaged tissue site in this analysis could not be located in the conventional H&E histological images due to the artifacts introduced in the histological processing.
Results
Correlations with co-located FFPE histology
The acquired SLAM images and converted histology-like images were compared with co-located conventional FFPE histology to identify the correlated tissue features. As shown in Figure 4, multiple correlations of essential tissue features were found with a high degree of similarity.
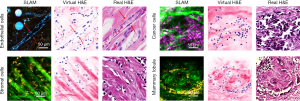
First, the rat endothelial cells within the mammary tumor microenvironment are effectively visualized in the SLAM image, featured by the elongated cytoplasm and nuclei (red arrows, upper-left image in Figure 4). In addition, an adipose cell of the same size is identified at the same location in all images (blue and white arrows, upper-left image in Figure 4).
The upper-right image in Figure 4 shows the correlation of cancer cells. The images of cancer cells were acquired from a site of human invasive lobular carcinoma. In the SLAM image, the magenta-colored THG signal intensities represent the membrane-rich cytoplasm of the cancer cells. These membrane structures can be attributed mainly to the extracellular vesicles, which were more actively produced and secreted by cancer cells based on previous studies (5,6). The SLAM image was acquired intraoperatively from a freshly resected human breast tissue specimen, and the tumor was buried hundreds of micrometers deep below the specimen surface, resulting in a reduced image SNR that makes it difficult to segment all the nuclei from the low-SNR SLAM image. Therefore, although the SLAM image shows many more cells characterized by their THG-intense cytoplasm, only a few of their nuclei can be segmented, resulting in a lower density.
The stromal cells in the SLAM image were correlated with the conventional FFPE histology (lower-left, Figure 4). The yellow 2PF signal is generated from the FAD within the cell cytoplasm. These stromal cells reside in the space between the straightened collagen fibers. A clear difference is noticed that the collagen fibers in the SLAM image are straighter than in the corresponding FFPE histology due to distortion artifacts induced during histology processing (4).
Finally, the histological correlation of a human mammary lobule is shown in the lower-right images of Figure 4. In the mammary lobule, the collagen fibers form several lobular structures for the lobular epithelial cells to reside in, and there are also a few epithelial cells surrounding the lobular structure. These features are identified with a high degree of similarity in both the SLAM and FFPE histology images.
Real-time three-dimensional histology-like imaging
Real-time 3D histology-like imaging was realized by the simultaneous detection and nonlinear optical sectioning capability of the SLAM microscope. Figure 5 and Video 1 present the time-lapse image sequence that captures the motion of blood flow. At this imaging site near a rat mammary tumor, the red blood cells flow through several blood vessels that are surrounded by collagen fibers with scattered stromal cells. With a 2-second acquisition time per frame, the flowing motion of the red blood cells in the unperturbed in vivo tissue can be readily identified in the SLAM and converted histology-like image sequence (Figure 5 and Video 1). In the histology-like images of Figure 5, the trajectories of four blood cells in eight continuous frames are delineated by black, blue, and green solid lines. The initial positions of the first three blood cells are represented by the small crosses in the first image (t =0 s). Then, the solid lines in each image record the trajectories of the blood cells by the time point shown above the image. These time-lapse trajectories provided a quantitative estimation of the blood flow velocity. In Figure 5, the lengths of the three trajectories at t =6 s are 21.2, 22.1, and 21.6 µm, separately, giving an averaged blood flow velocity of 3.6 µm/s, with a standard deviation of 0.1 µm/s. The original SLAM images without the marked blood cell trajectories are shown below each histology-like image for reference. As time goes by, the cell marked by the blue trajectory disappeared in the field-of-view at t =8 s. In the meantime, the trajectory of the fourth cell began, which is marked by the blue solid line again. This result demonstrates the capability of real-time histology-like imaging and its potential application.
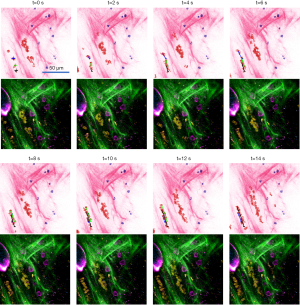
Video 2 and Figure 6 show the 3D visualization of the rat mammary tumor microenvironment, where many stromal cells are distributed at different depths within the extracellular matrix. The scanning of the image plane was realized by a piezo stage to assure the precise increment of 1.5 µm between each z-stack image in depth. As the focal plane changes, each cell nucleus slightly changes size and shape. After a certain depth, the nuclei become out-of-focus and disappear from the image. The depth-resolved information of nuclei can be used to implement 3D reconstructions. As shown in Video 3, a selected area (green dashed box in the first image of Figure 6) was selected to reconstruct the 3D tissue structure. The 3D structure of collagen fibers and 3D distribution of nuclei can be observed in Video 3. For the complex extracellular matrix composed of collagen fibers, the 3D orientation of the collagen fibers can be readily identified from the histology-like image stacks. Without physically sectioning the tissue specimen, the cell nuclei and collagen fibers remain in their natural states throughout the imaging process, free of any form of distortion artifacts. Utilizing this approach, the 3D distribution and spatial profile of the nuclei and collagen fibers can be visualized with much higher accuracy compared to conventional FFPE histology as well as other virtual histology techniques that still require tissue sectioning (14,15).
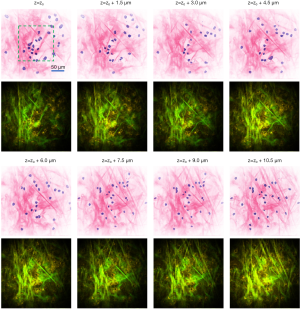
Quantification of N/C ratio
After segmenting the cells and nuclei from the SLAM image, the N/C ratio of each cell is quantified. Figure 7A shows the SLAM image acquired from the microenvironment of a rat mammary tumor. Around the tumor boundary, cancer and stromal cells were included for N/C ratio analysis. In Figure 7A, the cancer cells are densely distributed within the mammary tumor at the lower-right of the image, and 3PF signals are generated from their cytoplasm. On the other hand, stromal cells, with 2PF-intense cytoplasm, are sparsely distributed within the extracellular matrix. The boundaries of these cells are delineated and overlaid on the SLAM (Figure 7A) and histology-like (Figure 7B) images to demonstrate the size and shape of each cell. By quantifying the number of pixels for each cell nucleus and cytoplasm, the N/C ratio was subsequently calculated for each cell. The N/C ratios of the two types of cells are separately plotted in Figure 7C. In each cell group, the N/C ratios that are remarkably deviated from the rest of the group are identified as outliers (red circles in Figure 7C), and they are excluded from the statistical analysis. These outliers are probably the result from the limited spatial resolution and sampling frequency of SLAM microscopy. The inliers represented by the blue circles in Figure 7C give a statistical result of 4.08±2.06 for cancer cells, and 1.12±0.68 for stromal cells. Therefore, as shown in Figure 7D, the N/C ratios of cancer cells are markedly higher than the stromal cells, correlating well with pathological findings (22). The color of the delineated boundaries represents the N/C ratio of each cell, and it is observed that the boundaries of the stromal cells are generally bluer than the cancer cells. These colored cell boundaries indicating the N/C ratio are overlaid on the SLAM (Figure 7A) and converted histology-like images (Figure 7B). The computationally quantified N/C ratio and this presentation method of overlaying cell boundaries with color scales serve as useful tools in addition to the histology-like imaging to assist in cancer diagnosis.
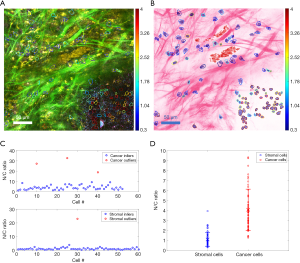
Discussion
This work presents real-time 3D histology-like imaging that is achieved by SLAM microscopy followed by feature segmentation and color transformation. By bypassing all the tissue processing procedures involved in conventional FFPE histology, this new imaging technique can provide histology-like color contrasts directly from even untreated in vivo tissues, enabling the observation of unperturbed tissue features and dynamics, such as blood flow, as well as 3D cell distribution and tissue morphology. The histology-like contrast makes these in vivo image features more interpretable by pathologists, promoting the clinical translation of nonlinear optical microscopy. The biological information available by this new histology-like imaging technique will not only give more insight into cancer investigations, but also potentially supplement current cancer diagnostics by providing new biomarkers or quantitative metrics, such as the computationally quantified N/C ratio and blood flow velocity. In particular, the unique features in SLAM images can be presented as new layers of contrast to overlay on the histology-like image. The overlaying of the cell boundaries colored according to the corresponding N/C ratio serves as one example of how the additional virtual imaging contrast provided by SLAM microscopy can improve and supplement histological imaging.
With these demonstrated merits and advantages, there still remain several improvements to be made on this imaging technique. The most evident limitation in this work is the absence of positive image contrast from the DNA within the cell nuclei. Therefore, the histology-like images only provide the location, shape, and size of the nuclei, while the actual DNA distribution within the nuclei cannot be retrieved and presented. Label-free visualization of cell nuclei, nucleoli, and DNA has been a long-standing challenge in the optical imaging community. The most promising approach is to use coherent Raman imaging techniques, such as coherent anti-Stokes Raman scattering (CARS) and stimulated Raman scattering (SRS) to identify the Raman signatures of the DNA (14,23). We are currently working on integrating coherent Raman imaging modalities into SLAM microscopy, without compromising system performance, to provide positive contrast for nuclei. In addition to CARS and SRS, we are also working on integrating fluorescence lifetime imaging (FLIM) into the SLAM imaging system to offer more diagnostic biomarkers.
Furthermore, it is noticed that the similarity between the real H&E histology images and the virtual H&E images is not as high as some previously demonstrated virtual H&E imaging approaches (12,13,15). This is mainly attributed to the fact that we used fresh untreated tissues to conduct in vivo or ex vivo imaging in real time. The tissues used to make the conventional histology were fixed and distorted after imaging and during the tissue processing. We believe the reduced similarity is the compromise we have to make to achieve these unique histology imaging capabilities like real-time virtual H&E video recordings and 3D histology imaging.
It was mentioned earlier that the DNN-based nuclei segmentation does not work well for low-SNR images, such as the images collected from deep tissue. This issue mainly resulted from the limited training data that relies on the labor-intensive and time-consuming manual segmentation. Therefore, the DNN model trained by the limited data was not very robust to noise. There are three future directions that might lead to the solution of this issue, including deep-learning-based image denoising, a larger amount of training data, and a refined design of the deep neural network.
Potential improvements can also be made on the imaging speed and FOV. The current image acquisition time is about 2 seconds per 350×350 µm2 FOV, making the assessment of the entire tissue specimen rather time-consuming for routine clinical diagnosis. To address this, our lab is currently developing next-generation SLAM microscopy that can increase the current imaging speed by at least an order-of-magnitude. In addition, fast imaging techniques like optical coherence tomography (OCT) can be integrated to grossly scan the entire specimen (24) and identify areas suspicious of cancer, followed by SLAM microscopy of these areas to provide the detailed local tissue contrasts necessary for generating the histology-like images.
Acknowledgments
We thank Drs. Anna Higham and Kimberly Cradock for providing human surgical tissue specimens as part of their standard practice, and we thank the human subjects for volunteering to contribute tissue specimens for this research. We also thank the study coordinators and Research Office at Carle Foundation Hospital. Additional information can be found at http://biophotonics.illinois.edu.
Funding: This research was supported in part by grants from the National Institutes of Health (R01CA213149, R01EB023232) and the National Science Foundation (CBET 18-41539). Allison Spaulding received support from the NSF REU Program when conducting this study.
Footnote
Conflicts of Interest: All authors have completed the ICMJE uniform disclosure form (available at http://dx.doi.org/10.21037/qims-20-381). YS, SY, EJC, DRS, MM, SAB report grants from the National Institutes of Health during the conduct of this study; SAB also reports grants from the National Science Foundation during the conduct of the study; HT reports grants from LiveBx, LLC outside the submitted work; in addition, HT has a patent Molecular Imaging Biomarkers issued, and a patent Clipping-Assisted Dual-Fluorophore Sensing pending; SY has a patent No. 10,445,880 issued. The other authors have no conflicts of interest to declare.
Ethical Statement: The study of human tissue imaging was conducted under a protocol approved by the institutional review boards of Carle Foundation Hospital and the University of Illinois at Urbana-Champaign (No: 18CCC1708) and informed consent was acquired from the human subjects who participated in this study. In vivo rat tissue imaging was conducted under a protocol approved by the Institutional Animal Care and Use Committee at the University of Illinois at Urbana-Champaign (No: 18043).
Open Access Statement: This is an Open Access article distributed in accordance with the Creative Commons Attribution-NonCommercial-NoDerivs 4.0 International License (CC BY-NC-ND 4.0), which permits the non-commercial replication and distribution of the article with the strict proviso that no changes or edits are made and the original work is properly cited (including links to both the formal publication through the relevant DOI and the license). See: https://creativecommons.org/licenses/by-nc-nd/4.0/.
References
- Buesa RJ. Productivity standards for histology laboratories. Ann Diagn Pathol 2010;14:107-24. [Crossref] [PubMed]
- Giacomelli MG, Husvogt L, Vardeh H, Faulkner-Jones BE, Hornegger J, Connolly JL, Fujimoto JG. Virtual hematoxylin and eosin transillumination microscopy using epi-fluorescence imaging. PLoS One 2016;11:e0159337. [Crossref] [PubMed]
- Tu H, Liu Y, Turchinovich D, Marjanovic M, Lyngsø JK, Lægsgaard J, Chaney EJ, Zhao Y, You S, Wilson WL, Xu B, Dantus M, Boppart SA. Stain-free histopathology by programmable supercontinuum pulses. Nat Photonics 2016;10:534-40. [Crossref] [PubMed]
- Taqi SA, Sami SA, Sami LB, Zaki SA. A review of artifacts in histopathology. J Oral Maxillofac Pathol 2018;22:279. [Crossref] [PubMed]
- Tu H, Liu Y, Marjanovic M, Chaney EJ, You S, Zhao Y, Boppart SA. Concurrence of extracellular vesicle enrichment and metabolic switch visualized label-free in the tumor microenvironment. Sci Adv 2017;3:e1600675. [Crossref] [PubMed]
- Sun Y, You S, Tu H, Spillman DR Jr, Chaney EJ, Marjanovic M, Li J, Barkalifa R, Wang J, Higham AM, Luckey NN, Cradock KA, Liu ZG, Boppart SA. Intraoperative visualization of the tumor microenvironment and quantification of extracellular vesicles by label-free nonlinear imaging. Sci Adv 2018;4:eaau5603.
- You S, Barkalifa R, Chaney EJ, Tu H, Park J, Sorrells JE, Sun Y, Liu YZ, Yang L, Chen DZ, Marjanovic M, Sinha S, Boppart SA. Label-free visualization and characterization of extracellular vesicles in breast cancer. Proc Natl Acad Sci 2019;116:24012-18. [Crossref] [PubMed]
- You S, Sun Y, Chaney EJ, Zhao Y, Chen J, Boppart SA, Tu H. Slide-free virtual histochemistry (Part I): development via nonlinear optics. Biomed Opt Express 2018;9:5240-52. [Crossref] [PubMed]
- You S, Sun Y, Chaney EJ, Zhao Y, Chen J, Boppart SA, Tu H. Slide-free virtual histochemistry (Part II): detection of field cancerization. Biomed Opt Express 2018;9:5253-68. [Crossref] [PubMed]
- Bower AJ, Li J, Chaney EJ, Marjanovic M, Spillman DR, Boppart SA. High-speed imaging of transient metabolic dynamics using two-photon fluorescence lifetime imaging microscopy. Optica 2018;5:1290-6. [Crossref] [PubMed]
- You S, Tu H, Chaney EJ, Sun Y, Zhao Y, Bower AJ, Liu YZ, Marjanovic M, Sinha S, Pu Y, Boppart SA. Intravital imaging by simultaneous label-free autofluorescence-multiharmonic microscopy. Nat Commun 2018;9:2125. [Crossref] [PubMed]
- Fereidouni F, Harmany ZT, Tian M, Todd A, Kintner JA, McPherson JD, Borowsky AD, Bishop J, Lechpammer M, Demos SG, Levenson R. Microscopy with ultraviolet surface excitation for rapid slide-free histology. Nat Biomed Eng 2017;1:957-66. [Crossref]
- Wong TTW, Zhang R, Hai P, Zhang C, Pleitez MA, Aft RL, Novack DV, Wang L V. Fast label-free multilayered histology-like imaging of human breast cancer by photoacoustic microscopy. Sci Adv 2017;3:e1602168. [Crossref] [PubMed]
- Orringer DA, Pandian B, Niknafs YS, Hollon TC, Boyle J, Lewis S, Garrard M, Hervey-Jumper SL, Garton HJL, Maher CO, Heth JA, Sagher O, Wilkinson DA, Snuderl M, Venneti S, Ramkissoon SH, McFadden KA, Fisher-Hubbard A, Lieberman AP, Johnson TD, Xie XS, Trautman JK, Freudiger CW, Camelo-Piragua S. Rapid intraoperative histology of unprocessed surgical specimens via fibre-laser-based stimulated Raman scattering microscopy. Nat Biomed Eng 2017;1:0027.
- Rivenson Y, Wang H, Wei Z, de Haan K, Zhang Y, Wu Y, Günaydın H, Zuckerman JE, Chong T, Sisk AE, Westbrook LM, Wallace WD, Ozcan A. Virtual histological staining of unlabelled tissue-autofluorescence images via deep learning. Nat Biomed Eng 2019;3:466-77. [Crossref] [PubMed]
- Skala MC, Riching KM, Gendron-Fitzpatrick A, Eickhoff J, Eliceiri KW, White JG, Ramanujam N. In vivo multiphoton microscopy of NADH and FAD redox states, fluorescence lifetimes, and cellular morphology in precancerous epithelia. Proc Natl Acad Sci 2007;104:19494-99. [Crossref] [PubMed]
- Huang S, Heikal AA, Webb WW. Two-photon fluorescence spectroscopy and microscopy of NAD(P)H and flavoprotein. Biophys J 2002;82:2811-25. [Crossref] [PubMed]
- Chen X, Nadiarynkh O, Plotnikov S, Campagnola PJ. Second harmonic generation microscopy for quantitative analysis of collagen fibrillar structure. Nat Protoc 2012;7:654-69. [Crossref] [PubMed]
- Débarre D, Supatto W, Pena AM, Fabre A, Tordjmann T, Combettes L, Schanne-Klein MC, Beaurepaire E. Imaging lipid bodies in cells and tissues using third-harmonic generation microscopy. Nat Methods 2006;3:47-53. [Crossref] [PubMed]
- Wu S, Zhong S, Liu Y. Deep residual learning for image steganalysis. Multimed Tools Appl 2017;77:1-17.
- Ronneberger O, Fischer P, Brox T. U-net: Convolutional networks for biomedical image segmentation. arXiv 2015;9351:234-41.
- Malhotra S, Kazlouskaya V, Andres C, Gui J, Elston D. Diagnostic cellular abnormalities in neoplastic and non-neoplastic lesions of the epidermis: A morphological and statistical study. J Cutan Pathol 2013;40:371-78. [Crossref] [PubMed]
- Camp CH Jr, Cicerone MT. Chemically sensitive bioimaging with coherent Raman scattering. Nat Photonics 2015;9:295-305. [Crossref]
- Erickson-Bhatt SJ, Nolan RM, Shemonski ND, Adie SG, Putney J, Darga D, Mccormick DT, Cittadine AJ, Zysk AM, Marjanovic M, Chaney EJ, Monroy GL, South FA, Cradock KA, Liu ZG, Sundaram M, Ray PS, Boppart SA. Real-time imaging of the resection bed using a handheld probe to reduce incidence of microscopic positive margins in cancer surgery. Cancer Res 2015;75:3706-12. [Crossref] [PubMed]