A CT-based logistic regression model to predict spread through air space in lung adenocarcinoma
Introduction
Spread through air space (STAS) is defined as a detachment of micropapillary clusters, solid nests, or single cells beyond the edge of the tumor into the air spaces in surrounding lung parenchyma (1). This detachment is considered a novel invasion pattern of lung adenocarcinoma after or beyond the infiltration of the myofibroblast stroma, lymph vascular, and pleura (2,3). Onozato and colleagues first reported that the tumor island is detached to a collection of tumor cells that can be observed within the alveolar space from the primary tumor mass by a distance of at least a few alveoli (4). After this phenomenon was verified by two substantial studies and correlated with recurrence-free survival (not related to the stage), STAS was introduced into the 2015 World Health Organization (WHO) classification (5). Even though STAS is a worse prognosticator, if patients with STAS-positive tumors undergo lobectomy resection instead of limited local resection, patients’ prognosis can be significantly improved (6-8) Therefore, knowledge of STAS status before operation can facilitate surgeons in choosing an appropriate operation pattern for patients. Unfortunately, STAS is a histopathological finding, which can only be obtained after the operation. Recently, several reports have shown that several computed tomography (CT) characteristics were associated with STAS status (9-11). In one study, de Margerie-Mellon et al. studied 40 STAS-positive nodules and 40 STAS-negative nodules. From them, 203 subsolid nodules were identified; they found that the nodule diameter and the direct and relative diameters of the solid component were positively associated with STAS (9). In another study, Toyokawa et al. not only showed that a larger radiologic tumor diameter, vascular convergence, notch, pleural indentation, speculation, and the absence of ground-glass opacity (GGO) were associated with STAS in univariable analysis. They also found that the notch and the absence of GGO were risk factors of STAS with a combined odds ratio (OR) of 5.01 (10). Kim et al. analyzed CT features of 92 STAS-positive nodules and 184 STAS-negative nodules and found that the percentage of solid components was an independent predictor of STAS, and could obtain a sensitivity of 89.2% and a specificity of 60.3% using a cutoff value of 90% (11). Previously, we developed a CT-based radiomics machine learning model to predict STAS (12), and the model achieved an AUC of 0.754 (a sensitivity of 0.880 and a specificity of 0.588) for predicting STAS. This model showed that the CT-based radiomics could preoperatively predict STAS in lung adenocarcinoma with excellent diagnosis performance. Therefore, this study aimed to evaluate the value of the CT characteristics logistic regression model for the preoperative assessment of lung adenocarcinoma STAS status.
Methods
Patients
This retrospective study was approved by the institutional review board of both centers, and the requirement for informed consent was waived. From April 2015 to April 2019, information from 695 consecutive patients (531 from the center I and 164 from center II) with surgical histopathologically confirmed lung adenocarcinoma were located on the two centers’ electronic databases. We excluded patients who underwent CT examinations 3 months before the operation (n=19), received preoperative neoadjuvant chemotherapy (n=27) or preoperative biopsy with the theoretical possibility of infiltration or contamination through needles (n=32), or whose pathological sections were unsuitable for STAS detection (n=24), or who had more than one pathological confirmed tumor resected (n=15). The final study cohort included 578 patients (462 from center I and 116 from center II). Figure 1 shows the patient cohort’s workflow.
Histopathologic evaluation
For this study, two experienced pathologists who were blinded to the patient’s clinical outcomes were asked to review the hematoxylin and eosin (HE) tissue section again according to the WHO definitions of STAS in consensus to determine the STAS status. STAS positive is defined as the discovery of tumor cells in the lung air spaces beyond the edge of the primary tumor. It mainly consists of the following three forms: (I) an air space filled by micro-nipple structure without central fibrovascular cores; (II) a solid nest, with air spaces filled by the solid component of the tumor; (III) air spaces filled by multiple discrete and discontinuous single cells (5).
CT image acquisition and assessment
CT examinations were performed using 16-detector CT scanners (Philips Brilliance 16, Philips Medical Systems, or Toshiba Aquilion 16, Toshiba Medical Systems); 1.5 mm × 16 collimations were used, and images with a slice thickness of 2 mm and a gap of 1 mm were reconstructed using a standard reconstruction algorithm. The tube voltage was 120 kV, and the tube current was automatically adjusted. Two experienced radiologists analyzed the CT images independently on the picture archiving and communication system (PACS) with a lung window (1,500 HU window width and −600 HU window level) and mediastinum window (250 HU window width and 40 HU window level). The interpretations included 3 measurements (maximum diameter, maximum area, and area of the solid components of the tumor) and 20 semantic characteristics (nodule type, pleural thickening, pleural retraction, mediastinal lymph node enlargement, hilar lymphatic enlargement, vascular cluster sign, lobulation, specula, air bronchogram, satellite lesions, vacuolar sign, void sign, pleural effusion, distribution within the lobe, nodules location, low central attenuation, other pulmonary nodules, emphysema, pulmonary fibrosis, and calcification). Measurements were performed on the transverse section that displayed the largest nodule using the lung window setting. The nodule area was measured by a manual drawing of the region of interest. For part solid nodules, the area of the solid component was measured, and the ratio of the solid components was calculated as the area of the solid component divided by the nodule area. Any disagreement was resolved through consensus, and the measurements were averaged.
Statistical analysis
The statistical analysis was performed in SPSS and R version 3.5.1 (R Foundation for Statistical Computing). A P value of less than 0.05 indicated statistical significance. Non-normal distributed continuous variables are presented as medians and interquartiles. Categorical variables are presented as frequencies and percentages. At first, patients from center I were randomly divided into a training cohort (n=323) and a validation cohort (n=139) with a ratio of 0.7:0.3. Univariate analysis was used to select the CT characteristics associated with STAS in the patient cohort from center I. Measurements were compared with the Student’s t-test or Mann-Whitney U test. Proportions were compared using either the χ2 test or Fisher’s exact test. Then, multivariable logistic regression was used to develop the CT characteristics on those variables with statistical significance. The model was validated in the validation cohort and then tested in the external test cohort (patients from center II). During the development of the model, we used the ‘bestglm’ package in R for automated parameter tuning with ten-fold cross-validation to find the optimal model. The diagnostic performance of the model was measured by area under the curve (AUC) of receiver operating characteristic (ROC). The AUC of the model for the validation cohort and the external test cohort was compared with the DeLong test using the ‘pROC’ package in R (13).
Results
Of the 462 tumors from the center I, 90 (19.5%) were found to be STAS positive. In the patient cohort from center II, STAS-positive nodules were identified in 28 patients (24.1%). The difference in the prevalence of STAS-positive nodules between the two centers was not statistically significant (χ2=1.238, P=0.266). In center I cohort patients with STAS-positive tumors, it was found that the individuals were significantly younger than those with STAS-negative tumors. However, the differences in gender and smoking status between STAS-positive and STAS-negative tumors were not statistically significant (Table 1). Univariate analysis of the CT characteristics of STAS-positive and -negative nodules from centers I and II are summarized in Table 2. Eleven CT characteristics were associated with statistical significance. These factors included the maximum diameter of the tumor, the maximum area of the tumor, the area and ratio of the solid component, nodule type, pleural thickening, pleural retraction, mediastinal lymph node enlargement, vascular cluster sign, lobulation, and specula.
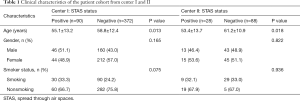
Full table
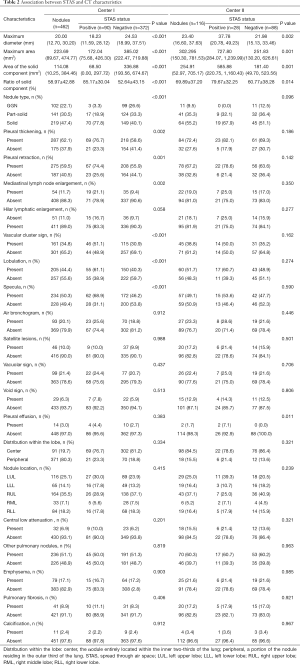
Full table
Regarding measurements, STAS-positive tumors tended to be larger in maximum diameter [18.23 mm (11.59, 28.12) vs. 24.53 mm (18.99, 37.51)], maximum area [172.04 (75.68, 426.30) vs. 385.02 (222.47, 719.88) mm2], areas of solid components [68.50 (0.00, 297.72) vs. 336.88 (193.56, 674.67) mm2], and had a higher ratio of solid components (85.17%±30.04% vs. 52.64%±43.15%).
As for the semantic characteristics, STAS-positive nodules tended to be solid or part-solid [ground glass nodules (GGNs), part solid, and solid were 3.3%, 18.9%, and 77.8% respectively, in STAS-positive nodules, and were 26.6%, 33.3%, and 40.1% respectively, in STAS-negative nodules]. STAS was also significantly associated with pleural thickening, pleural retraction, mediastinal lymph node enlargement, vascular cluster sign, lobulation, and specula.
CT images and histopathological photos of STAS-positive and STAS-negative nodules are shown in Figures 2 and 3.
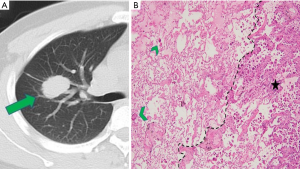
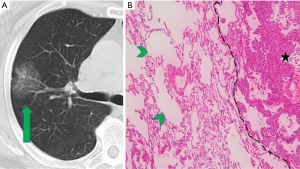
The optimal logistic regression model included age, maximum diameter, and ratio of solid component with OR value of 0.967 (95% CI: 0.944–0.988), 1.027 (95% CI: 1.008–1.046), and 5.14 (95% CI: 2.180–13.321), respectively. This model obtained the AUC of 0.801 (95% CI: 0.709–0.892) and 0.692 (95% CI: 0.518–0.866) in the validation cohort and the external test cohort, respectively (Figure 4). The difference was not statistically significant (P=0.280).
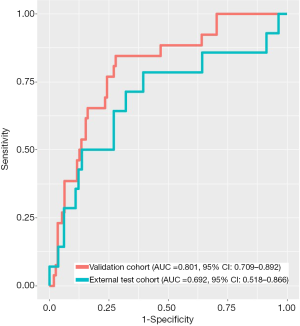
Discussion
Our study showed that several CT characteristics of lung adenocarcinoma were associated with STAS, which implies that the radiologists’ visual interpretation might have the potential to assess the STAS status preoperatively. Using automated parameter tuning, an optimal model which includes age, maximum diameter, and the ratio of the solid component can achieve high diagnostic performance for preoperative prediction of STAS in both the internal validation cohort and the external test cohort with AUC of 0.801 (95% CI: 0.709–0.892) and 0.692 (95% CI: 0.518–0.866) in the validation cohort and the external test cohort, respectively.
Widespread usage of low dose CT screening and micro intervention operation leads to the early detection of lung cancer and limited resection which preserves more lung parenchyma and improves patients’ prognosis in turn (14). With the aid of artificial intelligence, CT scanning can be used to predict the invasive patterns of lung cancer (15). The newly defined invasion manner of lung adenocarcinoma, STAS, was shown to be a significant prognosticator for locoregional recurrence when patients with STAS-positive nodules received limited resection. However, if these patients underwent lobectomy, the association between STAS and tumor recurrence and overall patient survival was not observed (5). Therefore, preoperative knowledge of STAS status is essential for surgeons to choose the optimal operative type. STAS is a histopathological finding which can only be discerned after operation. Before the operation, the surgeons cannot obtain information about the STAS status of lung nodules.
Several studies, including our previous research, attempted to investigate the association between CT features and STAS status or to predict STAS status using CT-based radiomics machine learning model (9-12). de Margerie-Mellon and colleagues noticed that size and a high proportion of solid components were associated with STAS (9). Using CT imaging features to develop a multivariable prediction model, Kim et al. found that the percentage of solid components was an independent predictor of STAS, which could achieve a sensitivity of 89.2% and a specificity of 60.3% when the cutoff value was set at 90% (11). Due to the low prevalence of STAS in early stage lung adenocarcinomas, both studies selected STAS-negative nodules with matched age, gender, and smoking status for analysis. Therefore, selection bias was introduced. In the present study, age was found to be an independent risk factor, and young patients tended to have STAS positive tumors. In the study performed by Toyokawa et al., presence of notch and the absence of GGO were demonstrated to be independent risk factors for the STAS phenomenon. Although Toyokawa et al. proved that the ratio of the solid components at four levels was different between STAS-positive and -negative tumors, they did not quantify the solid components in the multivariate analysis. Therefore, they might have exaggerated the contribution of these nodule types. In this study, the univariate analysis showed that both the nodule type and the ratio of the solid components were associated with STAS status, while multivariate analysis revealed that only the ratio of solid components was the risk factor. Nodule type and the ratio of solid components were related to the variables, and the ratio of solid components could quantify the sub-solid nodules. Our previous study showed that the median, maximum, age, maximum 3D diameter, and size-zone non-uniformity normalized scale were listed as the top five critical radiomics features with a random forest machine learning model, which obtained an AUC of 0.75 (12). Our study is the first to develop and validate a model to predict STAS on CT characteristics. Three variables, which consisted of age, maximum diameter, and the ratio of solid components, were selected to develop an optimal model using the leveraging automated parameter tuning R package, ‘bestglm’. The two CT characteristics included in the model were concordant with what had been reported by de Margerie-Mellon and Kim. Nodules with larger size and more solid components tended to be STAS positive. Adding age, our model performed as well as the radiomics model.
Furthermore, we added an external test cohort, which was unseen by the model. Although the only moderate performance was obtained in the test cohort, it reflected the model’s ability to predict STAS in the real world. Therefore, our study addressed not only the association between CT characteristics and STAS status of lung adenocarcinomas, but the possibility to harness perceptible CT characteristics to predict STAS, which allows it to be a routinely available modality in the real world and manages to eliminate the need for time-consuming segmentation.
There are some limitations to this study. First, STAS is a histopathological finding, and so we could only enroll those patients who had operation to resect tumors. The nodules tended to be large, and had more solid components or a greater likelihood of being malignant at visual interpretation. Therefore, selection bias might have been introduced. Second, only 19.5% nodules in the center I cohort and 24.1% nodules in the center II cohort were STAS positive. This imbalanced data could have decreased the accuracy of the model. Augmentation or down-sampling was conducted to improve the model’s performance and prevent overfitting. Third, the lack of follow-up prevented us from evaluating the effects of STAS on patients’ prognosis.
In conclusion, at univariate analysis, several CT characteristics were associated with the STAS status of lung adenocarcinomas. Age, maximum diameter, and the ratio of solid components were selected by the ‘bestglm’ package in R to develop an optimal model for predicting STAS status. The model showed good diagnostic performance in both the internal validation cohort and the external test cohort, which demonstrated that routine visual interpretation of CT images could be useful for preoperative assessment of STAS status for lung adenocarcinomas.
Acknowledgments
Funding: The Shenzhen Science and Technology Project supported this study (No. GJHZ20180928172002087).
Footnote
Conflicts of Interest: All authors have completed the ICMJE uniform disclosure form (available at http://dx.doi.org/10.21037/qims-20-724). JG serves as an unpaid editorial board member of Quantitative Imaging in Medicine and Surgery. The other authors have no conflicts of interest to declare.
Ethical Statement: This retrospective study was given approval by the institutional review boards of both centers, and the requirement for informed consent was waived.
Open Access Statement: This is an Open Access article distributed in accordance with the Creative Commons Attribution-NonCommercial-NoDerivs 4.0 International License (CC BY-NC-ND 4.0), which permits the non-commercial replication and distribution of the article with the strict proviso that no changes or edits are made and the original work is properly cited (including links to both the formal publication through the relevant DOI and the license). See: https://creativecommons.org/licenses/by-nc-nd/4.0/.
References
- Travis WD, Brambilla E, Nicholson AG, Yatabe Y, Austin JHM, Beasley MB, Chirieac LR, Dacic S, Duhig E, Flieder DB, Geisinger K, Hirsch FR, Ishikawa Y, Kerr KM, Noguchi M, Pelosi G, Powell CA, Tsao MS, Wistuba I, Panel WHO. The 2015 World Health Organization Classification of Lung Tumors: Impact of Genetic, Clinical and Radiologic Advances Since the 2004 Classification. J Thorac Oncol 2015;10:1243-60. [Crossref] [PubMed]
- Amin MB, Tamboli P, Merchant SH, Ordóñez NG, Ro J, Ayala AG, Ro JY. Micropapillary component in lung adenocarcinoma: a distinctive histologic feature with possible prognostic significance. Am J Surg Pathol 2002;26:358-64. [Crossref] [PubMed]
- Blaauwgeers H, Flieder D, Warth A, Harms A, Monkhorst K, Witte B, Thunnissen E. A Prospective Study of Loose Tissue Fragments in Non-Small Cell Lung Cancer Resection Specimens: An Alternative View to "Spread Through Air Spaces". Am J Surg Pathol 2017;41:1226-30. [Crossref] [PubMed]
- Onozato ML, Kovach AE, Yeap BY, Morales-Oyarvide V, Klepeis VE, Tammireddy S, Heist RS, Mark EJ, Dias-Santagata D, Iafrate AJ, Yagi Y, Mino-Kenudson M. Tumor islands in resected early-stage lung adenocarcinomas are associated with unique clinicopathologic and molecular characteristics and worse prognosis. Am J Surg Pathol 2013;37:287-94. [Crossref] [PubMed]
- Kadota K, Nitadori JI, Sima CS, Ujiie H, Rizk NP, Jones DR, Adusumilli PS, Travis WD. Tumor Spread through Air Spaces is an Important Pattern of Invasion and Impacts the Frequency and Location of Recurrences after Limited Resection for Small Stage I Lung Adenocarcinomas. J Thorac Oncol 2015;10:806-14. [Crossref] [PubMed]
- Dai C, Xie H, Su H, She Y, Zhu E, Fan Z, Zhou F, Ren Y, Xie D, Zheng H, Kadeer X, Chen D, Zhang L, Jiang G, Wu C, Chen C. Tumor Spread through Air Spaces Affects the Recurrence and Overall Survival in Patients with Lung Adenocarcinoma >2 to 3 cm. J Thorac Oncol 2017;12:1052-60. [Crossref] [PubMed]
- Berfield KS, Wood DE. Sublobar resection for stage IA non-small cell lung cancer. J Thorac Dis 2017;9:S208-S210. [Crossref] [PubMed]
- Warth A, Muley T, Kossakowski CA, Goeppert B, Schirmacher P, Dienemann H, Weichert W. Prognostic Impact of Intra-alveolar Tumor Spread in Pulmonary Adenocarcinoma. Am J Surg Pathol 2015;39:793-801. [Crossref] [PubMed]
- de Margerie-Mellon C, Onken A, Heidinger BH, VanderLaan PA, Bankier AA. CT Manifestations of Tumor Spread Through Airspaces in Pulmonary Adenocarcinomas Presenting as Subsolid Nodules. J Thorac Imaging 2018;33:402-8. [Crossref] [PubMed]
- Toyokawa G, Yamada Y, Tagawa T, Kamitani T, Yamasaki Y, Shimokawa M, Oda Y, Maehara Y. Computed tomography features of resected lung adenocarcinomas with spread through air spaces. J Thorac Cardiovasc Surg 2018;156:1670-1676.e4. [Crossref] [PubMed]
- Kim SK, Kim TJ, Chung MJ, Kim TS, Lee KS, Zo JI, Shim YM. Lung Adenocarcinoma: CT Features Associated with Spread through Air Spaces. Radiology 2018;289:831-40. [Crossref] [PubMed]
- Jiang C, Luo Y, Yuan J, You S, Chen Z, Wu M, Wang G, Gong J. CT-based radiomics and machine learning to predict spread through air space in lung adenocarcinoma. Eur Radiol 2020;30:4050-7. [Crossref] [PubMed]
- DeLong ER, DeLong DM, Clarke-Pearson DL. Comparing the areas under two or more correlated receiver operating characteristic curves: a nonparametric approach. Biometrics 1988;44:837-45. [Crossref] [PubMed]
- Mao L, Chen H, Liang M, Li K, Gao J, Qin P, Ding X, Li X, Liu X. Quantitative radiomic model for predicting malignancy of small solid pulmonary nodules detected by low-dose CT screening. Quant Imaging Med Surg 2019;9:263-72. [Crossref] [PubMed]
- Wang S, Wang R, Zhang S, Li R, Fu Y, Sun X, Li Y, Sun X, Jiang X, Guo X, Zhou X, Chang J, Peng W. 3D convolutional neural network for differentiating pre-invasive lesions from invasive adenocarcinomas appearing as ground-glass nodules with diameters ≤3 cm using HRCT. Quant Imaging Med Surg 2018;8:491-9. [Crossref] [PubMed]