Differential artery-vein analysis in quantitative retinal imaging: a review
Introduction
The retina is a neurovascular complex network that can be frequently targeted by eye diseases. As one part of the central nervous system, the retina can also be a window for assessing brain function and cardiovascular conditions. Systemic conditions such as diabetes, can also cause retinal neurovascular abnormalities, including diabetic retinopathy (DR) and diabetic macular edema (DME). Long term diabetes induces hyperglycemia-induced vascular damage (1), hypertension and hyperlipidemia. Furthermore, blood clots and atherosclerosis can develop into pathologies such as retinal vein occlusion (RVO) (2). Retinal diseases, such as DR and RVO, often affect arteries and veins differently. One of the key observations of RVO is the arteriovenous AV crossings (also called AV nicking), in which a small artery is observed to cross a small vein, resulting in the compression of the vein. In patients with atherosclerosis, vein narrowing has been observed at the site of AV crossing (3). Similarly, retinal arterial narrowing (4,5) and venous beading (6,7) have been observed in patients with DR and other diseases. Therefore, differential AV analysis of vascular abnormalities is valuable for disease screening, diagnosis, and treatment assessment.
Different imaging modalities, such as fundus photography (8-11), optical coherence tomography (OCT) (12,13) and OCT angiography (OCTA) (14-18), have been demonstrated for quantitative assessment of retinal vasculatures. It is known that arteries and veins are often affected differently, either at the early stage or throughout the disease progression. Furthermore, the interactions between arteries and veins are also prominent microvascular abnormalities in certain diseases such as the compressive nature of AV nicking in RVO (19). However, a recent study that employed adaptive optics technology, has revealed that without detectable arteriovenous contact, there is still a change in morphology of the vasculature, i.e., nicking, narrowing, opacification, and dragging (20). Therefore, differential artery-vein (AV) analysis can provide enhanced performance for quantitative retinal imaging. Differential AV analysis has been demonstrated to be valuable for evaluating diabetes, hypertension, stroke and cardiovascular diseases (21-23) along with common retinopathies (24,25). For differential AV analysis, the first step is to perform AV classification in retinal images. This step consists of several processing procedures, such as image intensity normalization, fovea/optic disc localization to find region of interest (ROI), vessel segmentation, and feature extraction. The second step is to use quantitative feature analyses or machine learning algorithms for robust AV classification. The final step is to use the AV maps to quantify the artery and vein features, for eye disease detection and treatment assessment. In order to achieve robust AV measurement, extensive efforts have been explored to address technical issues relative to these three steps of differential AV analysis.
In this article, we provide a brief review of differential AV classification and analysis in quantitative retinal imaging. Following section 2 describes clinical importance of differential AV analysis. Section 3 summarizes differential AV in traditional color fundus photography. Section 4 provides recent developments of differential AV analysis in the relatively new OCTA. Section 5 discusses current limits and prospective developments of differential AV analysis.
Clinical importance of differential AV analysis
Differential AV analysis has been a topic of widespread research for clinicians for a long time. Several large-scale clinical studies have been conducted both prospectively and longitudinally to evaluate AV abnormalities in different diseases. This section describes some of the studies that focused on quantitative AV features. Most of the clinical studies relied on fundus imaging, however, did not utilize automated AV classification techniques. Most studies have attempted manual or semi-automated approaches to identify artery and vein retinal vessels and observed significant AV changes for different diseases. Hence, it is even more evident why integration of automated and robust AV classification techniques is required in clinical imaging devices. Differential AV feature analysis for different diseases are summarized as follows.
Diabetes and diabetic retinopathy (DR)
DR is diabetic complication that can be broadly divided into non-proliferative and proliferative stages. The prevalence of DR symptoms increases with duration of diabetes, from non-proliferative to proliferative stage. The two main risk factors associated with DR are hyperglycemia and hypertension (26). Recent studies have shown evidence that dyslipidemia is also a major risk factor (26). In hypertension patients, clinical signs are arteriolar narrowing, arterio-venous nicking, increased arteriolar light reflex, retinal hemorrhages, and cotton wool spots (27,28). Hypertension has also been associated with decrease in branching angle at arteriole bifurcations (29), and microvascular density in the retina (29-31). These retinal vascular abnormalities have been observed in both adults (32-42) and children (27). Historically, arteriolar caliber which is an early sign of hypertension in DR has been difficult to measure clinically (43). Hubbard et al. (44-46) developed several techniques to measure AV ratio (AVR) features for clinical research. These features have substantial reproducibility and have been validated in different epidemiological studies (40,44,47-49).
Recent studies have suggested that both narrowed arterioles and widened venules contribute to the sensitivity of AVR features (50,51). Arteriolar and venular widths or calibers appear to reflect different pathophysiological processes (50,52). Three large population based studies, i.e., the Atherosclerosis Risk in Communities (ARIC) study (53), the Beaver Dam study (54) and Rotterdam study (22), have demonstrated that the AVR features have association with the development of type 2 diabetes and DR. The ARIC and Beaver dam study observed decreased AVR diameter associated with incident diabetes whereas the Rotterdam study observed increased overall retinal vascular caliber and venular caliber associated with chronic hyperglycemia and pre-diabetes. Apart from DR, wider venular caliber has been found to be associated with other microvascular complications of diabetes, such as diabetic nephropathy (55). It has been also associated with several metabolic syndrome and obesity (50,56). The Blue Mountain study found that a wider venular caliber was linked to 5-year incidence of obesity and higher body-mass-index (BMI) in subjects of normal weight at baseline as well as children aged 6–8 years (57). It has been speculated that the venular widening could be the result of increased blood flow associated with retinal hypoxia (58) and hyperglycemia (59). Alternative theories behind the pathophysiological reasons of venular widening include inflammatory processes (35,40,60,61), or endothelial dysfunctions (62,63) associated with impaired glucose metabolism and diabetes.
Cardiovascular and other diseases
New imaging developments and AV classification capabilities have allowed the measurement of other architectural changes in the retinal microvasculature. Some of these changes in retina have also been observed to be associated with cardiovascular risk. The Beaver Dam Study (64) demonstrated decreased arteriolar tortuosity and suboptimal arteriolar bifurcation associated with coronary heart mortality. The ARIC study demonstrated a semi-automated system to measure the vessel calibers on fundus photograph (44-46) and observed a lower AVR of caliber and generalized arteriolar narrowing were able to provide information that could predict incident cardiovascular diseases without knowing other cardiovascular risk factors (36,38,41,44,53). Compared with other imaging biomarkers in fundus image such as AV nicking, hemorrhages, and focal arteriolar narrowing, the AVR caliber has been more reliable and commonly used to quantify vascular damage (38,41,44,53). However, this study states that it remains unclear, how artery and venular calibers contribute to the change in AVR measures. Some studies were explored to quantify the correlation between AVR features and blood pressure in the other parts of the body (36,65).
With increasing pulse and blood pressures, the arteriolar diameters decreased in a linear pattern. The relationship was observed to be the strongest in the younger age category and became nonsignificant above 80 years (50). The venular diameters on the other hand, showed a smaller decrease. There was a linear decrease of AVR diameter with pulse and blood pressures. The relationship with AVR caliber was most distinct for patients in the category of age 55 to 60 years. Also, associations of AVR caliber to atherosclerosis was found inconclusive. The arteriolar calibers did not show an association with atherosclerosis except for the intima–media thickness. After statistical adjustment for blood pressure, the association became weaker. The venular calibers were linearly correlated to some biomarkers of atherosclerosis. Larger venular calibers were associated with a higher carotid plaque score, lower ankle–arm index, aortic calcifications. Furthermore, a lower AVR caliber was found to be related to a lower ankle–arm index, higher carotid plaque score and increased intima–media thickness, but not to aortic calcifications. Higher leukocyte count, lower serum HDL levels, higher erythrocyte sedimentation rate, smoking, higher hip to waist ratio and total serum cholesterol, were related to larger venular diameters and a lower AVR caliber.
In certain retinal diseases such as RVO, a common microvascular abnormality is AV nicking. There is a consensus that in AV nicking the vein is compressed by the bypassing artery. However, a recent study revealed that arteriovenous contact may not be necessary for abnormal venous changes, i.e., nicking, narrowing, and dragging (20).
Differential AV analysis in traditional color fundus photography
Almost all the research work conducted for AV classification and differential AV analysis have been based on fundus images. Fundus photography is widely used in clinics and provides a non-invasive solution to look at the retinal vasculature with color and intensity information. A number of algorithms have been proposed to explore computer-aided classification of A-V vessels (66-75). Some of these proposed algorithms for automated AV classification are representatively summarized in this section by dividing them into two categories: (I) feature extraction and vessel tracking based; and (II) machine learning (ML) based methods.
Feature extraction-based methods
A majority of the AV classification algorithms are based on color and intensity information from arteries and veins (68-71,73-75). In general speaking, the arteries have lighter color intensity due to the presence of oxygenated blood, compared to deoxygenated blood in the veins. Therefore, this information provides a general thresholding factor to separate arteries and veins. Vázquez et al. (76) used a “snake” model to extract various feature points in two color spaces of RGB and HSL from the blood vessels near the optic disc and selected multiple sets of features for an AV classification method based on a K-means clustering algorithm. They further improved the AV classification performance using a minimal path approach in a follow up study (75). Niemeijer et al. (71) proposed an AV classification method based on intensity and derivative information in retinal vessels. Relan et al. (77-79) automatically classified AV in retinal vessels based on color features using GMM-EM (Gaussian Mixture Model, Expectation-Maximization) unsupervised classifier, utilizing quadrant-pairwise approach. Some researchers have also tried to incorporate functional features, such as optical density ratio (ODR) in red and green channels, to identify arteries and veins (80-82). Mirsharif et al. (83) divided the retinal vessel tree into few subsets, and then integrated a vascular tracking technique along with color information to classify the vessels into artery and vein. Estrada et al. (84) proposed a global AV classification method based on graph theory, considering the topological structure of retinal fundus vessels. Vessel tracking is also a quite common technique used for AV classification (75,85-87). Alam et al. (23) introduced a method that incorporates both ODR based feature extraction and vessel tracking for AV classification. Before AV classification, they used matched filtering and bottom hat thresholding to enhance and extract a detailed vessel map from the green channel of color fundus image. They used ODR based features to identify AV source nodes near optic disc, since the difference in ODR value is more prevalent between artery and vein in that area. After identifying the AV source nodes, a vessel tracking algorithm was used to classify AV in whole vessel map. Figures 1,2 illustrate the vessel extraction and tacking procedures for AV classification.
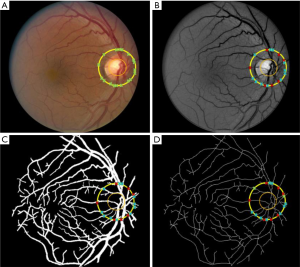
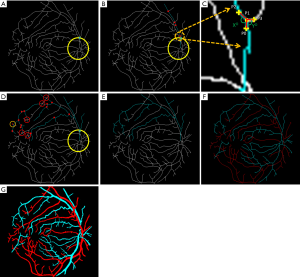
Machine learning (ML) based approaches
Both supervised and unsupervised ML algorithms have been explored for AV classification of retinal vasculatures. General methodology of these strategies is to train a traditional (i.e., supervised) or deep (i.e., unsupervised) ML classifier to identify artery and vein. In traditional supervised classifiers, the features are usually pre-extracted. These features could be color, intensity based, functional features or any features engineered to distinguish artery and vein by the researchers. The classifiers are trained to learn the trend of the features to distinguish artery and vein vessels based on the ground truths prepared by physicians. In deep ML, also termed as deep learning (DL), based approaches, the deep neural network learns to extract similar features, such as color, intensity, and morphological features, based on provided ground truths for automated AV classification. Although DL classifiers are quite powerful, most DL approaches require a large dataset, with annotated AV ground truths, for the reliable training process.
Semiautomatic (66,67,72) and automatic approaches (71,77-80,83,88-91) for supervised AV classification have been demonstrated. Vijayakumar et al. (92) proposed a color feature-based AV classification method utilizing Random Forest and support vector machine (SVM) classifier. Yang et al. (93) also described a method based on SVM and CNN. Jelinek et al. (69) presented eight features and tested different classifier for AV classification. Niemeijer et al. (94) also investigated different classifiers, i.e., K-nearest-neighbor (KNN), Fisher linear discriminant analysis (LDA) and SVM for AV classification. In their analysis, SVM generated the best performance (true positive rate of 97% for arteries and 90% for veins) utilizing both structural and functional features.
Kondermann et al. (88) examined two profile and ROI feature extraction methods, and two classifiers based on SVM and neural networks for AV classification. Profile-based features were RGB color space values for each centerline pixel (after subtracting their mean) and ROI-based features were obtained around each centerline pixel. The extracted feature vectors were reduced by using multiclass principle component analysis before applying them to the classifiers. Muramatsu et al. (95) demonstrated an accuracy of 92.8% using LDA classifier with RGB and contrast features. Vázquez et al. (74,75,87) combined a color-based K-means clustering approach (unsupervised classifier) and vessel tracking for AV classification. Their method achieved about 88% accuracy. Another study by Rothaus and Jiang (96) also utilized K-means clustering for AV classification. However, one of the disadvantages of the K-means clustering method is its initialization problem and that the iterative method can get stuck in local minima. Niemeijer et al. (71,94,97) demonstrated an automatic supervised method using LDA, SVM and KNN for AV classification and observed good AV classification accuracy up to 88%. Fraz et al. (98) proposed an ensemble classifier of decision tress for AV classification that lead to an 83% accuracy rate. Recent works have also demonstrated feasibility of DL based methods for AV classification (99-103). Girard et al. (99) used a convolutional neural network (CNN) for AV classification and obtained about 94% accuracy on publicly available DRIVE dataset (104). A representative DL approach is illustrated in Figure 3 and representative AV classification performance on publicly available dataset (99) is shown in Figure 4.
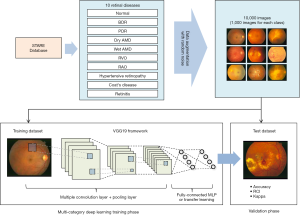
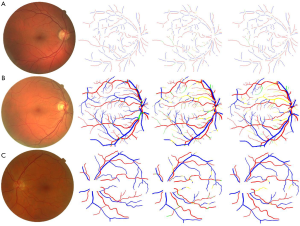
Differential AV analysis in OCTA
As aforementioned, traditional color fundus images have been widely used for AV classification and differential AV analysis of eye conditions. However, fundus images have limited resolution to reveal microvascular abnormalities in the retina, particularly difficult for evaluating smaller capillary level blood vessels around the fovea. In contrary, relatively new OCTA can provide depth-resolved capability to visualize multiple vascular layers in the retina with capillary-level resolution. By providing unprecedented morphological details of retinal vasculatures, OCTA has been rapidly adopted for clinical management of DR (14), age-related macular degeneration (AMD) (15), glaucoma (16), sickle cell retinopathy (SCR) (17,18,24), etc. However, clinical OCT and OCTA don’t have the function for differential AV classification and analysis. In order to achieve differential AV analysis in OCTA, color fundus image analysis and OCT information processing guided methods are developed (17,24,25,105-107).
Color fundus image analysis guided AV classification in OCTA
Alam et al. (24) demonstrated a fundus image guided AV classification technique in OCTA. In this study, both fundus and OCTA images are obtained from subjects. A fundus AV map was first generated using ODR and vessel tracking (23) algorithm. In the next step, parafoveal region of fundus image was aligned with corresponding OCTA image. The fovea was used as an anchor point and a geometric-affine based image registration method was used to align the vessel maps of two images. Upon registration the AV classification from fundus image was mapped on to the OCTA image. The additional vascular branches in OCTA were tracked back to source nodes accordingly. Figure 5 demonstrates the methodology of this AV classification technique. Figure 6 shows different AV map generated for control and SCR subjects.
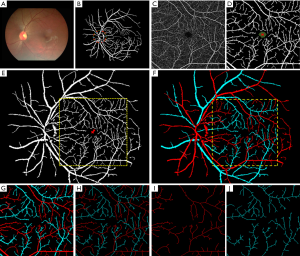
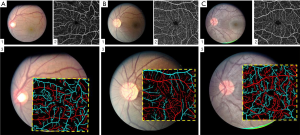
Based on these AV classification maps, two AVR features were demonstrated: AVR-Blood vessel caliber (BVC) and tortuosity (AVR-BVT) for quantifying DR (25) (Figure 7) and SCR patients (24) (Figure 8).
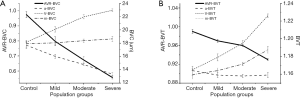
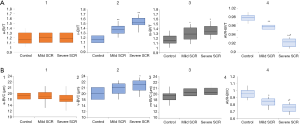
For DR study, AVR-BVC and AVR-BVT provided significant (P<0.001) and moderate (P<0.05) improvements, respectively, in detecting and classifying NPDR stages, compared with traditional m-BVC. The opposite polarity of BVC in artery (narrow) and vein (dilated) caused AVR-BVC to be highly significant. For SCR study, the BVT was prevalent mostly in vein, making vein BVT the most significant feature for SCR classification.
OCT information processing guided AV classification in OCTA
Ouyang et al. (108) demonstrated a spectral dimension (SD) OCT based technique or AV classification in OCT. In this semi-automatic approach, the ground truth AV notations are generated around the optic disc using infrared reflectance (IR) or fluorescence angiography (FA) imaging. The presence or absence of hyperreflective lower border reflectivity features in OCT was used to differentiate artery and vein. This method was applied to larger vessels around optic disc and generated sensitivity of 0.88/0.93 and specificity of 0.93/0.88 for arteries and veins, respectively. We found two recent studies that attempted manual approaches for AV classification in en face OCTA based on some empirical rules (109,110). In the first study by Ishibazawa et al. (109), two masked readers were instructed to identify artery and vein in en face OCTA using following rules: (I) the presence of surrounding hypointense areas that represent the capillary-free zones, are associated with arteries; (II) arteries and veins do not cross each other, physiologically; (III) vessels could be traced back proximally and distally for identification of source nodes. The AV classification in en face OCTA was compared with AV maps from corresponding fundus images. The average accuracies identifying all AV vessels, first-, second-, and third-order AV vessels were 98.61%, 99.16%, 100%, and 98.06%, respectively. In another study by Xu et al. (110) used similar manual strategy but utilized four default en face slabs that included color depth encoded retina, grayscale full-thickness retina, superficial OCTA, and deep layer OCTA. Manual graders identified AV maps with an accuracy of 96.9% and 93.2% respectively for 3 mm and 6 mm OCTA scans.
Son et al. (107) reported an automated approach for AV classification in OCTA using near-infrared OCT oximetry to guide AV classification in macular OCTA scans. This study developed a custom-built OCT/OCTA device with an oxygen sensitive wavelength of 765 nm which provided 2.8 times higher oxygen extinction coefficient between artery and vein (Figure 9).
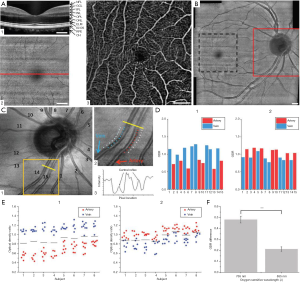
Alam et al. (105) also presented a study where they utilized en face OCT to guide AV classification in OCTA. Using OCT en face to guide AV classification in OCTA could improve efficiency for clinical deployment of AV classification and differential AV analysis. Since OCT and OCTA are intrinsically reconstructed from the same raw spectrogram, it removes the requirement for image registration. This study employed K-means clustering using four OCT intensity profile features, i.e., (I) ratio of vessel width to central reflex, (II) average of maximum profile brightness, (III) average of median profile intensity, and (IV) optical density of boundary intensity compared to background intensity, for classifying AV source nodes around optic disc. After the identification of AV source nodes, vessel tracking is employed to generate en face OCT AV map and consequent mapping of AV information into OCTA (Figure 10). This study reported a 96% accuracy for classifying artery and vein in OCTA image.
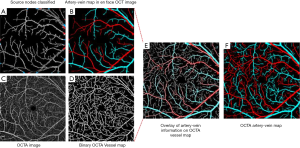
Discussion
Technical rationales and clinical applications for quantitative AV analysis in traditional color fundus photography and relatively new OCTA have been briefly summarized in the article. Retinal vascular abnormalities are frequently observed in eye diseases. As one part of the central nervous system, the retinal neurovascular network can also be targeted by neurodegenerative diseases such as Alzheimer’s, Parkinson’s and others. It is known the artery and vein systems can be affected in different ways by diseased types and stages. Therefore, quantitative AV analysis are valuable for improved disease detection and treatment managements. Although a variety of approaches have been developed for AV classification and quantitative AV analysis, there are still major problems to be addressed, especially for clinical deployments of differential AV analysis. Most of current AV classification works utilize color and intensity-based features in traditional color fundus images. The absolute spectral information varies greatly among images from different devices and even from images within the same subject. Some reasons of variability are oxygen saturation, imaging artifacts, optical distortions, motion artifacts, development of cataract, aging effect, etc. A solution to this variability is color and contrast normalization, which has been employed by some studies (23). Some studies also rely on the difference of artery and vein vessel calibers for AV classification near the optic disc. Compared to vessel caliber, a more reliable feature is central reflex which quantifies the inner wall of artery or vein and the actual path of blood flow within vessels. Combination or ratio of central reflex and vessel caliber could be a more reliable strategy for AV classification near the optic disc (111). Presence of micro-aneurysms, exudates, or hemorrhages can also incur further challenges in AV classification. All these challenges can make reliable AV classification difficult, especially for pursuing fully automates processing. Some approaches proposed the use of clustering or semantic segmentation which were successful in AV classification, but primarily for larger blood vessels. ML based approaches however are limited to ground truth quality and require a large number of training data. Miri et al. (85) showed that some studies simplified AV classification process and included only large vessels for differential AV analysis since the AV classification performance is more robust for larger vessels. It was argued that the AVR calculation could be sufficient using the larger vessels. However, it has been shown that fundus images are not able to show subtle micro-vascular changes in retina. Early stages of retinal diseases often manifest in the perifoveal and parafoveal retinal blood vessels. Therefore, conducting differential AV analysis in such detailed vasculature may significantly improve the quantitative efficiency. OCTA as an imaging modality is perfectly suitable for such analysis since it provides high resolution microvasculature information around fovea. However, a major challenge for AV classification in OCTA is the absence of spectral information. Therefore, the studies that have attempted AV classification in OCTA, have utilized either fundus or corresponding en face OCT images for guiding AV information to OCTA. It was observed that the differential analysis of vessel caliber and tortuosity improved the features sensitivity for objective classification of different retinal diseases. It was also interesting to see that the use of lower wavelength for OCT imaging, the oxygen extinction coefficient difference between artery and vein was enhanced multiple times.
Despite promising results in differentiating AV in OCTA, it is still challenging to establish robust end-to-end framework for reliable AV analysis. OCTA suffers from different artifacts such as projection artifacts, shadow artifacts and segmentation errors, which can affect the accuracy of AV classification. The demonstrated AV differentiation techniques in OCTA typically excluded images with severe projection and shadow artifacts. However, it was observed that, as long as the vessel connection was retained for the vessel tracking, the AV classification was successful. One reason is the classification of source nodes is first based on multiple features around optic disk, that are not severely distorted or affected by OCTA artifacts. Furthermore, the AV classifications have been only demonstrated in superficial layer since the algorithms depends on the connectivity and tracking of the vessel map. Segmentation of the superficial layers are also feasible with clinical devices. Therefore, the AV classification accuracy may not be affected by OCTA segmentation errors. One limitation of current methods is the incapability to identify AV in deep retinal and choroidal layers, which consists of a more densely connected mesh-like vessel network. At the capillary level, it is still difficult to distinguish artery or vein reliably. Therefore, to date the differential AV analysis is typically limited at superficial layer.
In conclusion, differential AV analysis has been demonstrated in both traditional color fundus photography and relatively new OCT angiography. Differential AV analysis has the potential to improve clinical managements of not only eye diseases but also systematic and cardiovascular diseases. However, clinical deployment of quantitative AV analysis is still at a very early stage. There seems to be a gap between the technological development and proper clinical application. Further development of DL based fully automated platform for differential AV analysis may foster its clinical deployments to advance the eye disease detection and management.
Acknowledgments
Funding: This research was supported in part by NIH grants R01 EY030101, R01EY029673, R01 EY023522, R01EY030842, P30 EY001792; by unrestricted grant from Research to Prevent Blindness; by Richard and Loan Hill endowment; by Illinois society to prevent blindness grant.
Footnote
Provenance and Peer Review: With the arrangement by the Guest Editors and the editorial office, this article has been reviewed by external peers.
Conflicts of Interest: All authors have completed the ICMJE uniform disclosure form (available at http://dx.doi.org/10.21037/qims-20-557). The special issue “Advanced Optical Imaging in Biomedicine” was commissioned by the editorial office without any funding or sponsorship. MNA and XY have a pending patent US2020/022774. The authors have no other conflicts of interest to declare.
Open Access Statement: This is an Open Access article distributed in accordance with the Creative Commons Attribution-NonCommercial-NoDerivs 4.0 International License (CC BY-NC-ND 4.0), which permits the non-commercial replication and distribution of the article with the strict proviso that no changes or edits are made and the original work is properly cited (including links to both the formal publication through the relevant DOI and the license). See: https://creativecommons.org/licenses/by-nc-nd/4.0/.
References
- Wang W, Lo AC. Diabetic retinopathy: pathophysiology and treatments. Int J Mol Sci 2018;19:1816. [Crossref] [PubMed]
- Karia N. Retinal vein occlusion: pathophysiology and treatment options. Clin Ophthalmol 2010;4:809-16. [Crossref] [PubMed]
- Fraenkl SA, Mozaffarieh M, Flammer J. Retinal vein occlusions: the potential impact of a dysregulation of the retinal veins. EPMA J 2010;1:253-61. [Crossref] [PubMed]
- Pedersen L, Jeppesen P, Knudsen ST, Poulsen PL, Bek T. Improvement of mild retinopathy in type 2 diabetic patients correlates with narrowing of retinal arterioles. A prospective observational study. Graefes Arch Clin Exp Ophthalmol 2014;252:1561-7. [Crossref] [PubMed]
- Cheung N, Bluemke DA, Klein R, Sharrett AR, Islam FA, Cotch MF, Klein BE, Criqui MH, Wong TY. Retinal arteriolar narrowing and left ventricular remodeling: the multi-ethnic study of atherosclerosis. J Am Coll Cardiol 2007;50:48-55. [Crossref] [PubMed]
- Fonseca RA, Dantas MA. Retinal venous beading associated with recurrent branch vein occlusion. Can J Ophthalmol 2002;37:182-3. [Crossref] [PubMed]
- Piguet B, Gross-Jendroska M, Holz FG, Bird AC. Inherited venous beading. Eye 1994;8:84-8. [Crossref] [PubMed]
- Faust O, Acharya R, Ng EYK, Ng KH, Suri JS. Algorithms for the automated detection of diabetic retinopathy using digital fundus images: a review. J Med Syst 2012;36:145-57. [Crossref] [PubMed]
- Niemeijer M, Van Ginneken B, Staal J, Suttorp-Schulten MS, Abràmoff MD. Automatic detection of red lesions in digital color fundus photographs. IEEE Trans Med Imaging 2005;24:584-92. [Crossref] [PubMed]
- Sinthanayothin C, Boyce JF, Williamson TH, Cook HL, Mensah E, Lal S, Usher D. Automated detection of diabetic retinopathy on digital fundus images. Diabet Med 2002;19:105-12. [Crossref] [PubMed]
- Walter T, Klein J-C, Massin P, Erginay A. A contribution of image processing to the diagnosis of diabetic retinopathy-detection of exudates in color fundus images of the human retina. IEEE Trans Med Imaging 2002;21:1236-43. [Crossref] [PubMed]
- ElTanboly AH, Palacio A, Shalaby AM, Switala AE, Helmy O, Schaal S, El-Baz A. An automated approach for early detection of diabetic retinopathy using sd-oct images. Front Biosci (Elite Ed) 2018;10:197-207. [Crossref] [PubMed]
- de Carlo TE, Chin AT, Bonini Filho MA, Adhi M, Branchini L, Salz DA, Baumal CR, Crawford C, Reichel E, Witkin AJ. Detection of microvascular changes in eyes of patients with diabetes but not clinical diabetic retinopathy using optical coherence tomography angiography. Retina 2015;35:2364-70. [Crossref] [PubMed]
- Kim AY, Chu Z, Shahidzadeh A, Wang RK, Puliafito CA, Kashani AH. Quantifying Microvascular Density and Morphology in Diabetic Retinopathy Using Spectral-Domain Optical Coherence Tomography Angiography. Invest Ophthalmol Vis Sci 2016;57:OCT362-70. [Crossref] [PubMed]
- Palejwala NV, Jia Y, Gao SS, Liu L, Flaxel CJ, Hwang TS, Lauer AK, Wilson DJ, Huang D, Bailey ST. Detection of non-exudative choroidal neovascularization in age-related macular degeneration with optical coherence tomography angiography. Retina 2015;35:2204. [Crossref] [PubMed]
- Holló G. Vessel density calculated from OCT angiography in 3 peripapillary sectors in normal, ocular hypertensive, and glaucoma eyes. Eur J Ophthalmol 2016;26:e42-5. [Crossref] [PubMed]
- Alam M, Thapa D, Lim JI, Cao D, Yao X. Quantitative characteristics of sickle cell retinopathy in optical coherence tomography angiography. Biomed Opt Express 2017;8:1741-53. [Crossref] [PubMed]
- Alam M, Thapa D, Lim JI, Cao D, Yao X. Computer-aided classification of sickle cell retinopathy using quantitative features in optical coherence tomography angiography. Biomed Opt Express 2017;8:4206-16. [Crossref] [PubMed]
- Nguyen UT, Bhuiyan A, Park LA, Kawasaki R, Wong TY, Wang JJ, Mitchell P, Ramamohanarao K. An automated method for retinal arteriovenous nicking quantification from color fundus images. IEEE Trans Biomed Eng 2013;60:3194-203. [Crossref] [PubMed]
- Paques M, Brolly A, Benesty J, Lermé N, Koch E, Rossant F, Bloch I, Girmens J-F. Venous nicking without arteriovenous contact: the role of the arteriolar microenvironment in arteriovenous nickings. JAMA ophthalmology 2015;133:947-50. [Crossref] [PubMed]
- Hatanaka Y, Nakagawa T, Aoyama A, Zhou X, Hara T, Fujita H, Kakogawa M, Hayashi Y, Mizukusa Y, Fujita A, editors. Automated detection algorithm for arteriolar narrowing on fundus images. 2005 IEEE Engineering in Medicine and Biology 27th Annual Conference, 2006.
- Ikram MK, Janssen JA, Roos AM, Rietveld I, Witteman JC, Breteler MM, Hofman A, Van Duijn CM, de Jong PT. Retinal vessel diameters and risk of impaired fasting glucose or diabetes: the Rotterdam study. Diabetes 2006;55:506-10. [Crossref] [PubMed]
- Alam M, Son T, Toslak D, Lim J, Yao X, Tech TVS. Combining optical density ratio and blood vessel tracking for automated artery-vein classification and quantitative analysis in color fundus images. Transl Vis Sci Technol 2018;7:23. [Crossref] [PubMed]
- Alam M, Lim JI, Toslak D, Yao X. Differential Artery–Vein Analysis Improves the Performance of OCTA Staging of Sickle Cell Retinopathy. Transl Vis Sci Technol 2019;8:3. [Crossref] [PubMed]
- Alam M, Toslak D, Lim JI, Yao X. Color fundus image guided artery-vein differentiation in optical coherence tomography angiography. Invest Ophthalmol Vis Sci 2018;59:4953-62. [Crossref] [PubMed]
- Nguyen TT, Wang JJ, Wong TY. Retinal vascular changes in pre-diabetes and prehypertension: new findings and their research and clinical implications. Diabetes Care 2007;30:2708-15. [Crossref] [PubMed]
- Wong TY, Mitchell P. The eye in hypertension. Lancet 2007;369:425-35. [Crossref] [PubMed]
- Wong TY, Mitchell P. Hypertensive retinopathy. N Engl J Med 2004;351:2310-7. [Crossref] [PubMed]
- Stanton AV, Wasan B, Cerutti A, Ford S, Marsh R, Sever PP, Thom SA, Hughes AD. Vascular network changes in the retina with age and hypertension. J Hypertens 1995;13:1724-8. [PubMed]
- Hughes AD, Martinez-Perez E, Jabbar AS, Hassan A, Witt NW, Mistry PD, Chapman N, Stanton AV, Beevers G, Pedrinelli R. Quantification of topological changes in retinal vascular architecture in essential and malignant hypertension. J Hypertens 2006;24:889-94. [Crossref] [PubMed]
- Kutschbach P, Wolf S, Sieveking M, Ittel TH, Schulte K, Reim M. Retinal capillary density in patients with arterial hypertension: 2-year follow-up. Graefes Arch Clin Exp Ophthalmol 1998;236:410-4. [Crossref] [PubMed]
- Duncan BB, Wong T, Tyroler H, Davis C, Fuchs F. Hypertensive retinopathy and incident coronary heart disease in high risk men. Br J Ophthalmol 2002;86:1002-6. [Crossref] [PubMed]
- Klein R, Klein B, Moss SE. The relation of systemic hypertension to changes in the retinal vasculature: the Beaver Dam Eye Study. Trans Am Ophthalmol Soc 1997;95:329. [PubMed]
- Klein R, Klein BE, Moss SE, Wang Q. Hypertension and retinopathy, arteriolar narrowing, and arteriovenous nicking in a population. Arch Ophthalmol 1994;112:92-8. [Crossref] [PubMed]
- Klein R, Sharrett AR, Klein BE, Chambless LE, Cooper LS, Hubbard LD, Evans G. Are retinal arteriolar abnormalities related to atherosclerosis? Arterioscler Thromb Vasc Biol 2000;20:1644-50. [Crossref] [PubMed]
- Leung H, Wang JJ, Rochtchina E, Tan AG, Wong TY, Klein R, Hubbard LD, Mitchell P. Relationships between age, blood pressure, and retinal vessel diameters in an older population. Invest Ophthalmol Vis Sci 2003;44:2900-4. [Crossref] [PubMed]
- Sharp PS, Chaturvedi N, Wormald R, McKeigue PM, Marmot M, Young SM. Hypertensive retinopathy in Afro-Caribbeans and Europeans: prevalence and risk factor relationships. Hypertension 1995;25:1322-5. [Crossref] [PubMed]
- Sharrett AR, Hubbard LD, Cooper LS, Sorlie PD, Brothers RJ, Nieto FJ, Pinsky JL, Klein R. Retinal arteriolar diameters and elevated blood pressure: the Atherosclerosis Risk in Communities Study. Am J Epidemiol 1999;150:263-70. [Crossref] [PubMed]
- Wang JJ, Mitchell P, Leung H, Rochtchina E, Wong TY, Klein R. Hypertensive retinal vessel wall signs in a general older population: the Blue Mountains Eye Study. Hypertension 2003;42:534-41. [Crossref] [PubMed]
- Wong TY, Islam FA, Klein R, Klein BE, Cotch MF, Castro C, Sharrett AR, Shahar E. Retinal vascular caliber, cardiovascular risk factors, and inflammation: the multi-ethnic study of atherosclerosis (MESA). Invest Ophthalmol Vis Sci 2006;47:2341-50. [Crossref] [PubMed]
- Wong TY, Klein R, Sharrett AR, Manolio TA, Hubbard LD, Marino EK, Kuller L, Burke G, Tracy RP, Polak JF. The prevalence and risk factors of retinal microvascular abnormalities in older persons: The Cardiovascular Health Study. Ophthalmology 2003;110:658-66. [Crossref] [PubMed]
- Yu T, Mitchell P, Berry G, Li W, Wang JJ. Retinopathy in older persons without diabetes and its relationship to hypertension. Arch Ophthalmol 1998;116:83-9. [Crossref] [PubMed]
- Wagener HP, Clay GE, Gipner JF. Classification of Retinal Lesions in the Presence of Vascular Hypertension: Report submitted to the American Ophthalmological Society by the committee on Classification of Hypertensive Disease of the Retina. Trans Am Ophthalmol Soc 1947;45:57. [PubMed]
- Hubbard LD, Brothers RJ, King WN, Clegg LX, Klein R, Cooper LS, Sharrett AR, Davis MD, Cai J. Group ARiCS. Methods for evaluation of retinal microvascular abnormalities associated with hypertension/sclerosis in the Atherosclerosis Risk in Communities Study. Ophthalmology 1999;106:2269-80. [Crossref] [PubMed]
- Parr JC, Spears G. General caliber of the retinal arteries expressed as the equivalent width of the central retinal artery. Am J Ophthalmol 1974;77:472-7. [Crossref] [PubMed]
- Parr JC, Spears G. Mathematic relationships between the width of a retinal artery and the widths of its branches. Am J Ophthalmol 1974;77:478-83. [Crossref] [PubMed]
- Couper DJ, Klein R, Hubbard LD, Wong TY, Sorlie PD, Cooper LS, Brothers RJ, Nieto FJ. Reliability of retinal photography in the assessment of retinal microvascular characteristics: the Atherosclerosis Risk in Communities Study. Am J Ophthalmol 2002;133:78-88. [Crossref] [PubMed]
- Li H, Hsu W, Lee ML, Wong TY. Automatic grading of retinal vessel caliber. IEEE Trans Biomed Eng 2005;52:1352-5. [Crossref] [PubMed]
- Wong TY, Knudtson MD, Klein R, Klein BE, Meuer SM, Hubbard LD. Computer-assisted measurement of retinal vessel diameters in the Beaver Dam Eye Study: methodology, correlation between eyes, and effect of refractive errors. Ophthalmology 2004;111:1183-90. [Crossref] [PubMed]
- Ikram MK, de Jong FJ, Vingerling JR, Witteman JC, Hofman A, Breteler MM, de Jong PT. Are retinal arteriolar or venular diameters associated with markers for cardiovascular disorders? The Rotterdam Study. Invest Ophthalmol Vis Sci 2004;45:2129-34. [Crossref] [PubMed]
- Patton N, Aslam T, MacGillivray T, Dhillon B, Constable I. Asymmetry of retinal arteriolar branch widths at junctions affects ability of formulae to predict trunk arteriolar widths. Invest Ophthalmol Vis Sci 2006;47:1329-33. [Crossref] [PubMed]
- Liew G, Sharrett AR, Kronmal R, Klein R, Wong TY, Mitchell P, Kifley A, Wang JJ. Measurement of retinal vascular caliber: issues and alternatives to using the arteriole to venule ratio. Invest Ophthalmol Vis Sci 2007;48:52-7. [Crossref] [PubMed]
- Wong TY, Klein R, Sharrett AR, Schmidt MI, Pankow JS, Couper DJ, Klein BE, Hubbard LD, Duncan BB, Investigators A. Retinal arteriolar narrowing and risk of diabetes mellitus in middle-aged persons. JAMA 2002;287:2528-33. [Crossref] [PubMed]
- Wong TY, Shankar A, Klein R, Klein BE, Hubbard LD. Retinal arteriolar narrowing, hypertension, and subsequent risk of diabetes mellitus. Arch Intern Med 2005;165:1060-5. [Crossref] [PubMed]
- Wong TY, Shankar A, Klein R, Klein BE. Retinal vessel diameters and the incidence of gross proteinuria and renal insufficiency in people with type 1 diabetes. Diabetes 2004;53:179-84. [Crossref] [PubMed]
- Wang JJ, Taylor B, Wong TY, Chua B, Rochtchina E, Klein R, Mitchell P. Retinal vessel diameters and obesity: a population‐based study in older persons. Obesity 2006;14:206-14. [Crossref] [PubMed]
- Mitchell P, Cheung N, de Haseth K, Taylor B, Rochtchina E, Islam FA, Wang JJ, Saw SM, Wong TY. Blood pressure and retinal arteriolar narrowing in children. Hypertension 2007;49:1156-62. [Crossref] [PubMed]
- Saldívar E, Cabrales P, Tsai AG, Intaglietta M. Microcirculatory changes during chronic adaptation to hypoxia. Am J Physiol Heart Circ Physiol 2003;285:H2064-71. [Crossref] [PubMed]
- Grunwald JE, Riva CE, Baine J, Brucker AJ. Total retinal volumetric blood flow rate in diabetic patients with poor glycemic control. Invest Ophthalmol Vis Sci 1992;33:356-63. [PubMed]
- Caballero AE. Metabolic and vascular abnormalities in subjects at risk for type 2 diabetes: the early start of a dangerous situation. Arch Med Res 2005;36:241-9. [Crossref] [PubMed]
- Klein R, Klein BE, Knudtson MD, Wong TY, Tsai MY. Are inflammatory factors related to retinal vessel caliber?: The Beaver Dam Eye Study. Arch Ophthalmol 2006;124:87-94. [Crossref] [PubMed]
- de Rekeneire N, Peila R, Ding J, Colbert LH, Visser M, Shorr RI, Kritchevsky SB, Kuller LH, Strotmeyer ES, Schwartz AV. Diabetes, hyperglycemia, and inflammation in older individuals: the health, aging and body composition study. Diabetes Care 2006;29:1902-8. [Crossref] [PubMed]
- Wilkinson-Berka JL. Vasoactive factors and diabetic retinopathy: vascular endothelial growth factor, cycoloxygenase-2 and nitric oxide. Curr Pharm Des 2004;10:3331-48. [Crossref] [PubMed]
- Witt N, Wong TY, Hughes AD, Chaturvedi N, Klein BE, Evans R, McNamara M, Thom SAM, Klein R. Abnormalities of retinal microvascular structure and risk of mortality from ischemic heart disease and stroke. Hypertension 2006;47:975-81. [Crossref] [PubMed]
- Wong TY, Hubbard L, Klein R, Marino E, Kronmal R, Sharrett A, Siscovick D, Burke G, Tielsch J. Retinal microvascular abnormalities and blood pressure in older people: the Cardiovascular Health Study. Br J Ophthalmol 2002;86:1007-13. [Crossref] [PubMed]
- Aguilar W, Martinez-Perez ME, Frauel Y, Escolano F, Lozano MA, Espinosa-Romero A, editors. Graph-based methods for retinal mosaicing and vascular characterization. Springer: International Workshop on Graph-Based Representations in Pattern Recognition, 2007.
- Chrástek R, Wolf M, Donath K, Niemann H, Michelson G, Appl MV, editors. Automated Calculation of Retinal Arteriovenous Ratio for Detection and Monitoring of Cerebrovascular Disease Based on Assessment of Morphological Changes of Retinal Vascular System. Available online: https://www.cvl.iis.u-tokyo.ac.jp/mva/proceedings/CommemorativeDVD/2002/papers/2002240.pdf
- Grisan E, Ruggeri A, editors. A divide et impera strategy for automatic classification of retinal vessels into arteries and veins. Proceedings of the 25th Annual International Conference of the IEEE Engineering in Medicine and Biology Society, 2003.
- Jelinek H, Depardieu C, Lucas C, Cornforth DJ, Huang W, Cree MJ, editors. Towards vessel characterization in the vicinity of the optic disc in digital retinal images. Image Vis Comput Conf; 2005.
- Li H, Hsu W, Lee ML, Wang H, editors. A piecewise Gaussian model for profiling and differentiating retinal vessels. Proceedings 2003 International Conference on Image Processing (Cat. No. 03CH37429), 2003.
- Niemeijer M, van Ginneken B, Abràmoff MD, editors. Automatic classification of retinal vessels into arteries and veins. Florida, United States: SPIE Medical Imaging, 2009.
- Rothaus K, Jiang X, Rhiem P. Separation of the retinal vascular graph in arteries and veins based upon structural knowledge. Image and Vision Computing 2009;27:864-75. [Crossref]
- Simó A, de Ves E. Segmentation of macular fluorescein angiographies. A statistical approach. Pattern Recognition 2001;34:795-809. [Crossref]
- Vázquez S, Barreira N, Penedo M, Penas M, Pose-Reino A, editors. Automatic classification of retinal vessels into arteries and veins. 7th international conference biomedical engineering (BioMED 2010), 2010.
- Vázquez S, Cancela B, Barreira N, Penedo MG, Rodríguez-Blanco M, Seijo MP, de Tuero GC, Barceló MA, Saez M. Improving retinal artery and vein classification by means of a minimal path approach. Mach Vis Appl 2013;24:919-30. [Crossref]
- Vázquez S, Barreira N, Penedo MG, Ortega M, Pose-Reino A. Improvements in retinal vessel clustering techniques: towards the automatic computation of the arterio venous ratio. Computing 2010;90:197-217. [Crossref]
- Dashtbozorg B, Mendonça AM, Campilho A. An automatic graph-based approach for artery/vein classification in retinal images. IEEE Trans Image Process 2014;23:1073-83. [Crossref] [PubMed]
- Joshi VS, Reinhardt JM, Garvin MK, Abramoff MD. Automated method for identification and artery-venous classification of vessel trees in retinal vessel networks. PLoS One 2014;9:e88061. [Crossref] [PubMed]
- Relan D, MacGillivray T, Ballerini L, Trucco E, editors. Retinal vessel classification: sorting arteries and veins. 2013 35th Annual International Conference of the IEEE Engineering in Medicine and Biology Society (EMBC), 2013.
- Narasimha-Iyer H, Beach JM, Khoobehi B, Roysam B. Automatic identification of retinal arteries and veins from dual-wavelength images using structural and functional features. IEEE Trans Biomed Eng 2007;54:1427-35. [Crossref] [PubMed]
- Gao X, Bharath A, Stanton A, Hughes A, Chapman N, Thom S, editors. A method of vessel tracking for vessel diameter measurement on retinal images. Proceedings 2001 International Conference on Image Processing (Cat. No. 01CH37205), 2001.
- Roberts DA. Analysis of vessel absorption profiles in retinal oximetry. Med Phys 1987;14:124-30. [Crossref] [PubMed]
- Mirsharif Q, Tajeripour F, Pourreza H. Automated characterization of blood vessels as arteries and veins in retinal images. Comput Med Imaging Graph 2013;37:607-17. [Crossref] [PubMed]
- Estrada R, Allingham MJ, Mettu PS, Cousins SW, Tomasi C, Farsiu S. Retinal artery-vein classification via topology estimation. IEEE Trans Med Imaging 2015;34:2518-34. [Crossref] [PubMed]
- Miri M, Amini Z, Rabbani H, Kafieh R. A comprehensive study of retinal vessel classification methods in fundus images. J Med Signals Sens 2017;7:59. [Crossref] [PubMed]
- Tramontan L, Grisan E, Ruggeri A, editors. An improved system for the automatic estimation of the Arteriolar-to-Venular diameter Ratio (AVR) in retinal images. 2008 30th Annual International Conference of the IEEE Engineering in Medicine and Biology Society, 2008.
- Saez M, González-Vázquez S, González-Penedo M, Barceló MA, Pena-Seijo M, de Tuero GC, Pose-Reino A. Development of an automated system to classify retinal vessels into arteries and veins. Comput Methods Programs Biomed 2012;108:367-76. [Crossref] [PubMed]
- Kondermann C, Kondermann D, Yan M, editors. Blood vessel classification into arteries and veins in retinal images. Medical Imaging 2007: Image Processing; 2007: International Society for Optics and Photonics.
- Zamperini A, Giachetti A, Trucco E, Chin KS, editors. Effective features for artery-vein classification in digital fundus images. 2012 25th IEEE International Symposium on Computer-Based Medical Systems (CBMS), 2012.
- CHEUNG CYL. Hsu W, Lee ML, Wang JJ, Mitchell P, Lau QP, Hamzah H, Ho M, Wong TY. A new method to measure peripheral retinal vascular caliber over an extended area. Microcirculation 2010;17:495-503.
- Abramoff MD, Suttorp-Schulten MS. Web-based screening for diabetic retinopathy in a primary care population: the EyeCheck project. Telemed J E Health 2005;11:668-74. [Crossref] [PubMed]
- Vijayakumar V, Koozekanani DD, White R, Kohler J, Roychowdhury S, Parhi KK, editors. Artery/vein classification of retinal blood vessels using feature selection. 2016 38th annual international conference of the IEEE engineering in medicine and biology society (EMBC), 2016.
- Yang Y, Bu W, Wang K, Zheng Y, Wu X, editors. Automated artery-vein classification in fundus color images. Springer: International Conference of Pioneering Computer Scientists, Engineers and Educators, 2016.
- Niemeijer M, Xu X, Dumitrescu A, Gupta P, van Ginneken B, Folk J, Abramoff M. INSPIRE-AVR: Iowa normative set for processing images of the retina-artery vein ratio. Available online: http://webeye.ophth.uuiowa.edu/Component/k2/item/270
- Muramatsu C, Hatanaka Y, Iwase T, Hara T, Fujita H. Automated selection of major arteries and veins for measurement of arteriolar-to-venular diameter ratio on retinal fundus images. Comput Med Imaging Graph 2011;35:472-80. [Crossref] [PubMed]
- Rothaus K, Jiang X, editors. Classification of arteries and veins in retinal images using vessel profile features. American Institute of Physics: AIP Conference Proceedings, 2011.
- Niemeijer M, van Ginneken B, Abràmoff MD, editors. Automatic determination of the artery-vein ratio in retinal images. Medical Imaging 2010. International Society for Optics and Photonics: Computer-Aided Diagnosis, 2010.
- Fraz M, Rudnicka AR, Owen CG, Strachan D, Barman SA, editors. Automated arteriole and venule recognition in retinal images using ensemble classification. 2014 International Conference on Computer Vision Theory and Applications (VISAPP), 2014.
- Girard F, Cheriet F, editors. Artery/vein classification in fundus images using CNN and likelihood score propagation. 2017 IEEE Global Conference on Signal and Information Processing (GlobalSIP), 2017.
- Welikala RA, Foster P, Whincup P, Rudnicka AR, Owen CG, Strachan D, Barman S. Automated arteriole and venule classification using deep learning for retinal images from the UK Biobank cohort. Comput Biol Med 2017;90:23-32. [Crossref] [PubMed]
- Choi JY, Yoo TK, Seo JG, Kwak J, Um TT, Rim TH. Multi-categorical deep learning neural network to classify retinal images: a pilot study employing small database. PLoS One 2017;12:e0187336. [Crossref] [PubMed]
- Huang F, Dashtbozorg B, Tan T, ter Haar Romeny BM. Retinal artery/vein classification using genetic-search feature selection. Comput Methods Programs Biomed 2018;161:197-207. [Crossref] [PubMed]
- Meyer MI, Galdran A, Costa P, Mendonça AM, Campilho A, editors. Deep convolutional artery/vein classification of retinal vessels. Springer: International Conference Image Analysis and Recognition, 2018.
- Hu Q, Abràmoff MD, Garvin MK, editors. Automated separation of binary overlapping trees in low-contrast color retinal images. Springer: International conference on medical image computing and computer-assisted intervention, 2013.
- Alam M, Toslak D, Lim JI, Yao X. OCT feature analysis guided artery-vein differentiation in OCTA. Biomed Opt Express 2019;10:2055-66. [Crossref] [PubMed]
- Son T, Alam M, Kim TH, Liu C, Toslak D, Yao X. Near infrared oximetry-guided artery–vein classification in optical coherence tomography angiography. Exp Biol Med (Maywood) 2019;244:813-8. [Crossref] [PubMed]
- Son T, Alam M, Liu C, Toslak D, Yao X, editors. Optical coherence tomography guided artery-vein classification in retinal OCT angiography of macular region. International Society for Optics and Photonics: Ophthalmic Technologies XXIX, 2019.
- Ouyang Y, Shao Q, Scharf D, Joussen AM, Heussen FM. An easy method to differentiate retinal arteries from veins by spectral domain optical coherence tomography: retrospective, observational case series. BMC Ophthalmol 2014;14:66. [Crossref] [PubMed]
- Ishibazawa A, Mehta N, Sorour O, Braun P, Martin S, Alibhai AY, Saifuddin A, Arya M, Baumal CR, Duker JS. Accuracy and Reliability in Differentiating Retinal Arteries and Veins Using Widefield En face OCT Angiography. Transl Vis Sci Technol 2019;8:60. [Crossref] [PubMed]
- Xu X, Yannuzzi NA, Fernández-Avellaneda P, Echegaray JJ, Tran KD, Russell JF, Patel NA, Hussain RM, Sarraf D, Freund KB. Differentiating Veins From Arteries on Optical Coherence Tomography Angiography by Identifying Deep Capillary Plexus Vortices. Am J Ophthalmol 2019;207:363-72. [Crossref] [PubMed]
- Fu D, Liu Y, Huang Z, editors. A Review of Retinal Vessel Segmentation and Artery/Vein Classification. Springer: Chinese Intelligent Systems Conference, 2017.