Influence of feature calculating parameters on the reproducibility of CT radiomic features: a thoracic phantom study
Introduction
Radiomics is an emerging advanced texture analysis technique for identification of the linkage between imaging phenotypes and the underlying disease genotypes and/or clinical manifestations (1). By employing machine learning and statistical analysis, high-dimensional radiomics features that describe the characteristics of lesion intensity (e.g., high or low signal), heterogeneity (e.g., homogeneous or heterogeneous), as well as shapes (e.g., round or spiculated), are extracted in medical images and correlated to the underlying gene expression profiles, histopathological features, and clinical symptoms (2). In addition, radiomics analysis can also improve diagnosis and predict prognosis or therapeutic response (3). Since its groundwork in 2012 (4,5), radiomics has shown its great potentials to improve diagnostic, prognostic, and predictive accuracy in a wide range of clinical research and studies.
However, concerns have been raised about the reproducibility of radiomic features (hereafter, features) for comparing and generalizing study results and conducting multicenter clinical trials (3). Feature reproducibility may be influenced by many factors involved in the entire radiomics pipeline, from image acquisition, reconstruction, segmentation, to feature calculation and analysis (3). Handling feature sensitivity to imaging and calculating parameters is critical in radiomics, as the great potential of this field lies in its utilization of publicly available medical images across institutions, devices, and collection methods. Thus, it is essential to thoroughly understand the effects of these parameters on radiomics features before decisions are made on how to handle them. Existing phantom and patient studies have demonstrated the effects imaging parameters (e.g., manufacturer, scanner, acquisition and reconstruction parameters) can have on radiomic features (3,6-9). However, little is known regarding the influence of feature calculating parameters (e.g., gray-level range and bin size) on radiomic features (10,11).
Therefore, the purpose of this study is to investigate the influence of feature calculating parameters (gray-level range and bin size) on the reproducibility of CT radiomic features in a thoracic phantom.
Methods
Phantom imaging
Thirty-six CT scans from an anthropomorphic thoracic phantom (Kyotokagaku Incorporated, Tokyo, Japan) publicly available at the Cancer Imaging Archive (TCIA) were employed in the study (12). The phantom contains 12 attached synthetic nodules (Kyotokagaku Incorporated, Tokyo, Japan or Computerized Imaging Reference Systems (CIRS), Norfolk, VA) varying in size (10 and 20 mm), shape [elliptical, lobulated and spiculate) and density (−630 and +100 Hounsfield Unit (HU)]. The phantom and the layout of the synthetic lung nodules are shown in Figure 1 (12). Each nodule was labeled by using three digits: the first digit indicating the diameter (1 for 10 mm and 4 for 20 mm), the second digit indicating shape (0 for elliptical, 2 for lobulate and 4 for spiculate) and the third digit indicating density (1 for −630 HU and 5 for 100 HU). This phantom was scanned on a 16-row Philips Mx8000 IDT (Philips Healthcare, Andover, MA) with different acquisition and reconstruction parameters (hereafter, imaging parameters) of effective dose (25, 100 or 200 mAs), pitch (0.9 or 1.2), slice thickness (0.75, 1.5 or 3 mm) and reconstruction kernel (medium or detail).
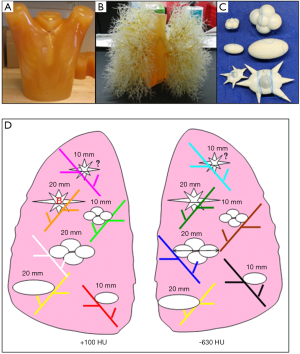
Nodule segmentation
The 36 scans were imported to a volumetric image analysis platform 3DQI (a free software platform for volumetric image analysis developed by the 3D quantitative imaging laboratory at Massachusetts General Hospital and Harvard Medical School) (https://3dqi.mgh.harvard.edu). To minimize the influence of partial volume effects and inter-scan misregistration, we selected six nodules ≥20 mm in this study. To evaluate the inter-observer reproducibility, the nodules were manually delineated by a senior radiologist with 19 years of experience in clinical radiology and a trained intern independently. To evaluate the intro-observer reproducibility, the senior radiologist delineated the nodules twice with a 2-week interval. The resulting volumes of interest (VOIs) of nodules that were delineated in one of the scans were transported to other scans by a rigid registration.
Radiomic feature calculation
A set of 88 radiomic features including shape features (n=11), statistics features [histogram (HIST) features (n=20), moment (n=3) and gradient features (n=2)], run-length (RL) features (n=16), gray-level co-occurrence matrix (GLCM) features (n=22) and gray-level zone-size matrix (GLZSM) features (n=14), were calculated for each nodule in each scan (Table S1 lists the radiomic features in the study) by using varied calculating parameters (gray-level range and bin size). Gray-level range indicates the lower bound and the upper bound of gray-level values (e.g., density in CT or signal intensity in MRI) used in texture calculation. The gray-level range is divided into a series of equal-size intervals, which is referred to bin size (2). We employed three gray-level ranges of 1,000 HU (−800 to 200 HU), 1,400 HU (−700 to 700 HU) and 2,000 HU (−1,000 to 1,000 HU) and eleven gray-level bin sizes ranging from 1 to 50 HU (1, 5, 10, 15, 20, 25, 30, 35, 40, 45, 50 HU). For a specific gray-level range, a pixel was excluded if its density was beyond the range we confined. A total of 1,188 (36×33) feature files were generated, each containing six nodules with 88 radiomic features for each nodule.
Feature reproducibility assessment
To investigate the influence of each parameter on feature reproducibility, we selected a set of reference values of imaging parameters (effective dose of 100 mAs, pitch of 0.9, slice thickness of 3 mm and reconstruction kernel of detail) in terms of the standard thoracic CT imaging protocol, and a set of reference values of calculating parameters (gray-level range of 1,000 HU and the bin size of 20 HU) in terms of the optimal lung nodules feature analysis. The influence of calculating parameters was assessed by fixing the imaging parameters to the reference values, and on the other hand the influence of imaging parameters was assessed by fixing the calculating parameters to the reference values. In our study, all imaging and calculating parameters had only 2–3 discrete values except bin size, which had 11 values. To reduce the variation caused by the extrema value of bin size (such as 1 or 50), we selected the bin sizes of 10, 20 and 40 HU for the influence of feature reproducibility in the comparisons between imaging and calculating parameters.
For each parameter, its influence was assessed by changing its value while keeping other parameters unchanged. For instance, the influence of gray-level range on feature reproducibility was evaluated when the bin size was assigned to 20 HU, whereas bin size was assessed in terms of the fixed gray-level range of 1,000 HU. For a comprehensive assessment of calculating parameters, we repeated this assessment in all 36 scans in addition to the scans with reference imaging parameters.
Feature reproducibility was assessed by the intraclass correlation coefficient (ICC) with the cutoff value of 0.8 and the coefficient of variation (CV) with the cutoff value of 20%. For each parameter, one feature was considered reproducible if more than four out of six nodules showed CV less than 20%. The overall influence of calculating parameters and imaging parameters on the feature reproducibility were compared by using the proportion of reproducible features in both parameters.
In addition, we classified the trendlines between the feature values and the bin sizes into six types: consistent, logarithmic ascending, polynomial ascending, logarithmic descending, polynomial descending and fluctuating. The type of trendline of each feature was determined by the largest correlation coefficient (R2) of the corresponding fitting curve, which ranges from 0 to 1, with 1 representing a perfect fit between the data and the curve, and 0 representing no statistical correlation between the data and the curve.
Statistical analysis
The statistical calculation was performed by using the build-in statistical analysis toolkit at 3DQI platform, which was developed using R (version 3.4.3; R Foundation for Statistical Computing, Vienna, Austria) with the DescTools packages and SPSS (version 24, IBM, Armonk, New York). The trendlines between the feature values (normalized 0 to 1) and the bin sizes were generated using Microsoft Excel (Office 365, Microsoft Software, Redmond, Washington).
Considering the skewed distribution of ICC and CV in most features, we reported the median ICC or CV of each feature, which was calculated by evaluating median values first through all scans and then through all features, represented as Median[scan][feature].
The agreement of reproducible features in different ranges and bin sizes were analyzed by Kendall’s W test and Kappa test (Kappa test was only for range 2,000 and 1,400 HU because their centers of CT values were the same). A Kendall’s W or Kappa coefficient of 0.81–1.00, 0.61–0.80, 0.41–0.60, 0.21–0.40 and 0.0–0.20 indicated perfect, substantial, moderate, fair and no agreement, respectively.
The statistical differences in the proportions of reproducible features, in two feature calculating parameters and four imaging parameters, were evaluated using Cochran’s Q test and Dunn’s test. For all statistical analyses, the P value or the adjusted P value (Bonferroni correction for Dunn’s test) less than 0.05 was considered statistically significant.
Results
For the three gray-level ranges of 1,000, 1,400 and 2,000 HU, the overall median ICCrange and CVrange in 36 scans and 88 features was 0.95 (range, 0.00 to 1.00) and 14% (range, −86% to 105%), respectively. According to the reproducibility criteria of ICCrange >0.8 and CVrange <20%, 50% (44/88) of features were considered reproducible. The proportions of reproducible features throughout 36 scans were 55/88 (62.5%), 52/88 (59.1%) and 51/88 (58%) for three gray-level ranges, respectively, with no statistically significant difference (P=0.420). The agreement was perfect (Kendall’s W coefficient 0.844, P<0.001) among the three ranges, but was substantial (Kappa coefficient 0.649, P<0.001) between ranges of 2,000 HU and 1,400 HU.
In the analysis of the influence of bin size, we excluded 16 features that are independent of bin size by definition, including 11 shape features, GRAD_mean, GRAD_std, MOMENT_j1, MOMENT_j2 and MOMENT_j3. These 16 features were treated as reproducible features when bin size was compared with other parameters.
For the 11 bin sizes, the overall median ICCbin and CVbin in 36 scans and 72 features were 0.75 (range, 0.00 to 1.00) and 39% (range, −328% to 331%), respectively. According to the criteria of feature reproducibility of ICCbin >0.8 and CVbin <20%, 33.3% (24/72) of features were considered reproducible (Table S1 lists the median ICC and CV values of each feature and Table S2 lists the reproducible and non-reproducible features in each group). Figure 2 shows the normalized feature values plotted against bin sizes for 72 features in four groups (HIST, RL, GLCM and GLZSM features).
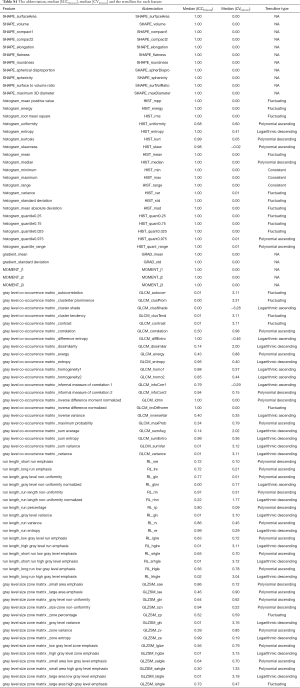
Full table
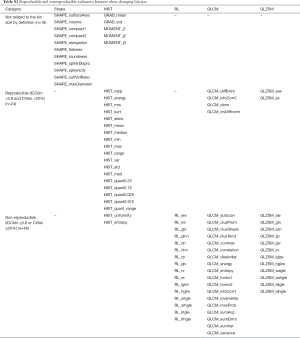
Full table
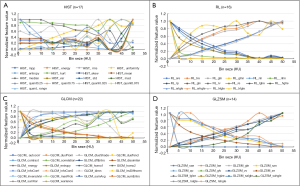
The types of trendlines between the feature values and the bin sizes in the scan of reference imaging parameters were: consistent (n=3), logarithmic ascending (n=7), polynomial ascending (n=19), logarithmic descending (n=17), polynomial descending (n=9) and fluctuating (n=17). Figure 3 shows examples for six types of trendlines. Of 72 features, the trendlines of 59 (81.9%) features were considered similar in 36 scans.
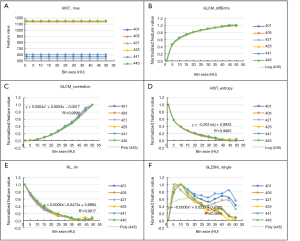
Table 1 lists the proportions of reproducible features of 36 scans in each bin size according to the criteria of ICCscan >0.8, or CVscan <20%, or (ICCscan >0.8 and CVscan <20%) in the reference gray-level range of 1,000 HU. There were statistically significant differences among different bin sizes (P=0.013). Although the overall proportions of reproducible features in bin size of 15, 20, 25, 50 HU were relatively higher than those in other bin sizes, the statistically significant differences were only observed between bin size 1 and 15 HU (adjusted P=0.045), 1 and 20 HU (adjusted P=0.045), 1 and 25 HU (adjusted P=0.045), 1 and 50 HU (adjusted P=0.009). The agreement of reproducible features in 11 bin sizes was perfect (Kendall’s W coefficient 0.879, P<0.001).
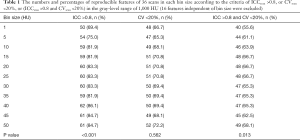
Full table
Table 2 lists the proportions of reproducible features among 36 scans in each bin size for the subgroups of HIST, RL, GLCM and GLZSM. There were no statistically significant differences among different bin sizes for each subgroup (P>0.05) except in RL group (P=0.004), in which the differences were observed between 1 and 25 HU, 1 and 30 HU, 1 and 35 HU, 1 and 40 HU, 1 and 45 HU, 1 and 50 HU (adjusted P<0.05).
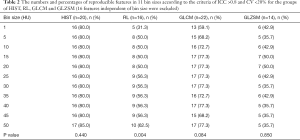
Full table
In the reference calculating parameters with range of 1,000 HU and bin size of 20 HU, 55 features (62.5%) were assessed reproducible, whereas 33 features were nonreproducible in 36 scans (Table S3).
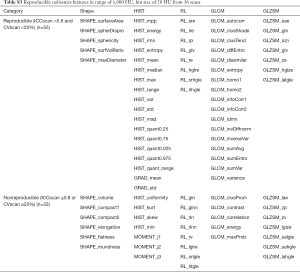
Full table
Table 3 listed the proportions of reproducible features in all the parameters (two calculating, four imaging and two segmentation parameters) involved in this study according to the criteria of ICC >0.8, or CV <20%, or (ICC >0.8 and CV <20%). There were statistically significant differences among six parameters (P<0.001). The proportions of reproducible features in calculating parameters (range, and bin size) were statistically significantly lower than those in imaging parameters (effective dose, pitch, slice thickness and filter) according to the criteria of (ICC >0.8 and CV <20%) (adjusted P<0.05).
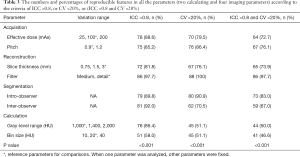
Full table
Discussion
The radiomic features employed in different platforms may be varied, among which statistical based methods have been most commonly applied. First-order (intensity of pixel histogram), second-order (run-length matrix and gray-level co-occurrence matrix) and higher orders (advanced metrics) features are analyzed in these methods. Gray-level range and gray-level bin size are indispensable and the most fundamental parameters applied in almost all radiomics platforms. Thus, we chose these two parameters for our study (2,13-15).
We observed that the proportions of reproducible features in calculating parameters were statistically significantly lower than those in imaging parameters according to the criteria of (ICC >0.8 and CV <20%). This indicates that the calculating parameters may have a greater influence on the reproducibility of CT radiomic features than imaging parameters. This observation is significant since the influence of calculating parameters have been undervalued and simply ignored in the majority previous studies. Most studies discussed the influence of imaging parameters, whereas only a few studies have clarified the parameters used in calculating the features (2,16).
The grey-level range may influence the feature reproducibility in two ways. If the centers of the ranges are different, for instance the 1,000 HU (center −300 HU) and 2,000 HU (center 0 HU) in our study, gray-level range may influence the distribution of the density in each bin. If the centers of two gray-level ranges are the same, such as 1,400 and 2,000 HU, whose centers are 0 HU, gray-level range may determine how many pixels are included, because the pixels are excluded if its density was beyond the range we confined. In our study, the agreement of range 2,000 and 1,400 HU was substantial (Kappa coefficient 0.649), which indicated that the influence of range cannot be ignored even if they have the same center. Range influences all the 88 features including the 16 features which are not affected by bin sizes.
When defining a range in an application, the underlying principle is to reduce the pixels that should not be included when we extract features. In this study, some component which may be included at the edge of an VOI, such as air and bones, were exclude in the range of −200 to 800 HU whereas included in the range of −1,000 to 1,000 HU. An appropriate range depends on the applications, e.g., the range used in a chest CT without contrast should be different with that in a contrast enhanced abdominal CT.
The trendlines revealed that the selection of bin size has a significant influence on the absolute values of the features, as all features except three changed when the bin sizes were changed. These results imply that the selections of bin size may influence the radiomics score or signature, which was built by combining some selected features into a regression model and used as the new biomarker for decision-making (2,14,16).
Bins are usually specified as consecutive, non-overlapping intervals of the intensity values. They must be adjacent, tend to be equal-width (but are not required to be). Wider bins reduce noise due to sampling randomness; whereas narrower bins give greater precision to the density distribution. Experimentation is usually needed to determine an appropriate bin size. An optimal bin size depends on the actual data distribution and the aims of analysis. For instance, if our purpose is to differentiate the malignant lesions from the benign (a higher resolution is preferred) in a chest CT with a lung kernel (which has a relatively higher noise level we intend to reduce), the bin size should be deliberately chosen to balance these two purposes.
The bin size influenced the feature values in different extents in four groups of features in our study. In general, second-order statistics features are more sensitive to the bin size than first-order statistics features. We observe this trend, being that histogram features (which are first-order) were less influenced by the bin size, whereas GLCM, RL and GLZSM features were more sensitive to the change of bin size.
Our research had two improvements in its methodology. Shafiq-ul-Hassan et al. (17) reported 17 out of 51 features were dependent on the number of gray-level using an in-house program and the statistical index coefficient of variation (CV) ≤20%. Larue et al. (18) found almost all features (114 features) changed in value using an in-house program and the statistical index concordance correlation coefficient (CCC) >0.85. Both used the Credence Cartridge phantom scanned on different CT-scanners with different tube currents and slice thicknesses. The proportions of reproducible features differed for three main factors, i.e., the phantoms, the radiomic software and the statistical indices. Some studies observed that the CT number is influenced by the anatomic position (19). The phantom we used was a thoracic phantom which simulated the human anatomy and contained nodules with different sizes, shapes and densities. This phantom was closer to some typical conditions in clinical setting compared with the Credence Cartridge phantom. Second, we combined several statistical indices which were complementary to each other to evaluate the reproducibility because each index has its pros and cons (20). For example, ICC values for a very heterogeneous sample may yield values that are very close to 1.0 based solely on the between-subject variance (20); therefore, we used CV to complement the evaluation of within-subject variation. By combining these statistical metrics, we evaluated the reproducibility of these features as comprehensively as possible.
Larue et al. (18) found the feature values were more similar with a bin size of 25 HU but the total numbers of reproducible features for each bin size were not significantly influenced when comparing a slice thickness of 1.5 mm with 3 mm, an exposure of 60 mA with 80 mA and two different scanners. Other investigators applied different bin sizes in their studies. Aerts et al. (2) applied a bin size of 25 HU for lung and head-and-neck cancers while Sun et al. (16) used a bin size of 10 HU for 15 types of solid tumors, but none of them discussed why they chose those two bin sizes instead of others. Our results were similar to Larue’s because there were no statistically significant differences among different bin sizes in the proportions of reproducible features, except in bin size 1 HU (in which proportion was the lowest). In clinical studies, the bin sizes of 15, 20 and 25 HU may be reasonable since the proportions of reproducible features were relatively higher in these bin sizes than in other bin sizes, and 10 to 20 HU are commonly used as the threshold of enhancement or measurement error because the CT numbers are affected by many factors such as X-ray beam hardening, X-ray scatter, partial volume effects, etc. (19,21,22). Although the proportion of reproducible features was the highest in the bin size of 50 HU, the image resolution is inadequate to distinguish different composition in tissues and lesions. The larger bin sizes may cause volume-confounding effects, such as imaging blurring, deterioration of structures and histogram shape. Thus, we do not recommend choosing the bin size of 50 HU to calculate the features.
Our study had two major contributions. First, we elucidated the important influence of calculating parameters on radiomics features. Second, we suggested to optimize, fix and report the gray-level range and bin size used in studies to guarantee the reproducibility of radiomic features.
Our study has some limitations. First, the variations of imaging parameters were limited, which may not cover all the variations in clinical settings. Second, we haven’t validated our results in patient data, which will be our future work. Third, some solutions, based on resampling images before feature extraction or statistical methods, have been proposed to reduce or compensate the variations caused by imaging parameters (9,23,24). We avoided using these procedures because we intended to compare the original effects of imaging parameters with calculating parameters on the feature reproducibility. Lastly, we didn’t analyze the test-retest and inter-scanner reproducibility because the effects of calculating parameters on them will be the same as on intra-scanner reproducibility.
Conclusions
Feature calculating parameters (range and bin size) may have a greater influence than imaging parameters (effective dose, pitch, slice thickness and filter) on the reproducibility of CT radiomic features, which should be given special attention in clinical applications.
Acknowledgments
Funding: This research was partly supported by Grant R42CA189637 from the National Institute of Health.
Footnote
Conflicts of Interest: All authors have completed the ICMJE uniform disclosure form (available at http://dx.doi.org/10.21037/qims-19-921). WC is the stockholder of IQ Medical Imaging LLC. The other authors have no conflicts of interest to declare.
Ethical Statement: No institutional review board approval was required for this phantom study.
Open Access Statement: This is an Open Access article distributed in accordance with the Creative Commons Attribution-NonCommercial-NoDerivs 4.0 International License (CC BY-NC-ND 4.0), which permits the non-commercial replication and distribution of the article with the strict proviso that no changes or edits are made and the original work is properly cited (including links to both the formal publication through the relevant DOI and the license). See: https://creativecommons.org/licenses/by-nc-nd/4.0/.
References
- Gillies RJ, Kinahan PE, Hricak H. Radiomics: Images Are More than Pictures, They Are Data. Radiology 2016;278:563-77. [Crossref] [PubMed]
- Aerts HJ, Velazquez ER, Leijenaar RT, Parmar C, Grossmann P, Carvalho S, Bussink J, Monshouwer R, Haibe-Kains B, Rietveld D, Hoebers F, Rietbergen MM, Leemans CR, Dekker A, Quackenbush J, Gillies RJ, Lambin P. Decoding tumour phenotype by noninvasive imaging using a quantitative radiomics approach. Nat Commun 2014;5:4006. [Crossref] [PubMed]
- Berenguer R, Pastor-Juan MDR, Canales-Vazquez J, Castro-Garcia M, Villas MV, Mansilla Legorburo F, Sabater S. Radiomics of CT Features May Be Nonreproducible and Redundant: Influence of CT Acquisition Parameters. Radiology 2018;288:407-15. [Crossref] [PubMed]
- Lambin P, Rios-Velazquez E, Leijenaar R, Carvalho S, van Stiphout RG, Granton P, Zegers CM, Gillies R, Boellard R, Dekker A, Aerts HJ. Radiomics: extracting more information from medical images using advanced feature analysis. Eur J Cancer 2012;48:441-6. [Crossref] [PubMed]
- Kumar V, Gu Y, Basu S, Berglund A, Eschrich SA, Schabath MB, Forster K, Aerts HJ, Dekker A, Fenstermacher D, Goldgof DB, Hall LO, Lambin P, Balagurunathan Y, Gatenby RA, Gillies RJ. Radiomics: the process and the challenges. Magn Reson Imaging 2012;30:1234-48. [Crossref] [PubMed]
- Buch K, Li B, Qureshi MM, Kuno H, Anderson SW, Sakai O. Quantitative Assessment of Variation in CT Parameters on Texture Features: Pilot Study Using a Nonanatomic Phantom. AJNR Am J Neuroradiol 2017;38:981-5. [Crossref] [PubMed]
- Caramella C, Allorant A, Orlhac F, Bidault F, Asselain B, Ammari S, Jaranowski P, Moussier A, Balleyguier C, Lassau N, Pitre-Champagnat S. Can we trust the calculation of texture indices of CT images? A phantom study. Med Phys 2018;45:1529-36. [Crossref] [PubMed]
- Lu L, Ehmke RC, Schwartz LH, Zhao B. Assessing Agreement between Radiomic Features Computed for Multiple CT Imaging Settings. PLoS One 2016;11:e0166550. [Crossref] [PubMed]
- Midya A, Chakraborty J, Gonen M, Do RKG, Simpson AL. Influence of CT acquisition and reconstruction parameters on radiomic feature reproducibility. J Med Imaging (Bellingham) 2018;5:011020. [Crossref] [PubMed]
- Shafiq-Ul-Hassan M, Zhang GG, Latifi K, Ullah G, Hunt DC, Balagurunathan Y, Abdalah MA, Schabath MB, Goldgof DG, Mackin D, Court LE, Gillies RJ, Moros EG. Intrinsic dependencies of CT radiomic features on voxel size and number of gray levels. Med Phys 2017;44:1050-62. [Crossref] [PubMed]
- Mackin D, Fave X, Zhang L, Yang J, Jones AK, Ng CS, Court L. Harmonizing the pixel size in retrospective computed tomography radiomics studies. PLoS One 2017;12:e0178524. [Crossref] [PubMed]
- Phantom FDA. Available online: http:// http://doi.org/ [Crossref]
- Zhang L, Fried DV, Fave XJ, Hunter LA, Yang J, Court LE. IBEX: an open infrastructure software platform to facilitate collaborative work in radiomics. Med Phys 2015;42:1341-53. [Crossref] [PubMed]
- Huang YQ, Liang CH, He L, Tian J, Liang CS, Chen X, Ma ZL, Liu ZY. Development and Validation of a Radiomics Nomogram for Preoperative Prediction of Lymph Node Metastasis in Colorectal Cancer. J Clin Oncol 2016;34:2157-64. [Crossref] [PubMed]
- Lubner MG, Smith AD, Sandrasegaran K, Sahani DV, Pickhardt PJ. CT Texture Analysis: Definitions, Applications, Biologic Correlates, and Challenges. Radiographics 2017;37:1483-503. [Crossref] [PubMed]
- Sun R, Limkin EJ, Vakalopoulou M, Dercle L, Champiat S, Han SR, Verlingue L, Brandao D, Lancia A, Ammari S, Hollebecque A, Scoazec JY, Marabelle A, Massard C, Soria JC, Robert C, Paragios N, Deutsch E, Ferté C. A radiomics approach to assess tumour-infiltrating CD8 cells and response to anti-PD-1 or anti-PD-L1 immunotherapy: an imaging biomarker, retrospective multicohort study. Lancet Oncol 2018;19:1180-91. [Crossref] [PubMed]
- Shafiq-Ul-Hassan M, Zhang GG, Latifi K, Ullah G, Hunt DC, Balagurunathan Y, Abdalah MA, Schabath MB, Goldgof DG, Mackin D, Court LE, Gillies RJ, Moros EG. Intrinsic dependencies of CT radiomic features on voxel size and number of gray levels. Med Phys 2017;44:1050-62. [Crossref] [PubMed]
- Larue RT, van Timmeren JE, de Jong EEC, Feliciani G, Leijenaar RTH, Schreurs WMJ, Sosef MN, Raat F, van der Zande FHR, Das M, van Elmpt W, Lambin P. Influence of gray level discretization on radiomic feature stability for different CT scanners, tube currents and slice thicknesses: a comprehensive phantom study. Acta Oncol 2017;56:1544-53. [Crossref] [PubMed]
- Szczykutowicz TP, DuPlissis A, Pickhardt PJ. Variation in CT Number and Image Noise Uniformity According to Patient Positioning in MDCT. AJR Am J Roentgenol 2017;208:1064-72. [Crossref] [PubMed]
- Raunig DL, McShane LM, Pennello G, Gatsonis C, Carson PL, Voyvodic JT, Wahl RL, Kurland BF, Schwarz AJ, Gonen M, Zahlmann G, Kondratovich MV, O'Donnell K, Petrick N, Cole PE, Garra B, Sullivan DC, Group QTPW. Quantitative imaging biomarkers: a review of statistical methods for technical performance assessment. Stat Methods Med Res 2015;24:27-67. [Crossref] [PubMed]
- Goodsitt MM, Chan HP, Way TW, Larson SC, Christodoulou EG, Kim J. Accuracy of the CT numbers of simulated lung nodules imaged with multi-detector CT scanners. Med Phys 2006;33:3006-17. [Crossref] [PubMed]
- Arslanoglu A, Chalian H, Sodagari F, Seyal AR, Tore HG, Salem R, Yaghmai V. Threshold for Enhancement in Treated Hepatocellular Carcinoma on MDCT: Effect on Necrosis Quantification. AJR Am J Roentgenol 2016;206:536-43. [Crossref] [PubMed]
- Ger RB, Zhou S, Chi PM, Lee HJ, Layman RR, Jones AK, Goff DL, Fuller CD, Howell RM, Li H, Stafford RJ, Court LE, Mackin DS. Comprehensive Investigation on Controlling for CT Imaging Variabilities in Radiomics Studies. Sci Rep 2018;8:13047. [Crossref] [PubMed]
- Orlhac F, Frouin F, Nioche C, Ayache N, Buvat I. Validation of A Method to Compensate Multicenter Effects Affecting CT Radiomics. Radiology 2019;291:53-9. [Crossref] [PubMed]