Big data and clinical research: focusing on the area of critical care medicine in mainland China
The 21st century is an era of big data involving all aspects of human life, including economics, biology, and medicine. In Wikipedia, the term big data is defined as any collection of data sets so large and complex that it becomes difficult to process using on-hand data management tools or traditional data processing applications. Big data can be seen in all aspects of our daily life. For instance, most of us hold a VIP card when we go shopping in a supermarket. The card records information on our individual characteristics such as age, gender, career and education. Every time when we use VIP card the supermarket can obtain information on the category of goods that we bought, as well as the time when this good are most likely to be sold. If we go shopping twice a week, there will be more than 100,000 observations in a year for a supermarket with daily volume of 1,000 people. Such a large sample size can provide sufficient statistical power for varieties of complex model fitting (e.g., fractional polynomials, spline function with more than five knots, and complex interactions) (1). In the field of marketing research, it is of particular interest to explore the association between particular characteristics of customers and their preference of buying. If a certain brand of perfume is more likely to be sold between 7 and 8 o’clock at night, promotion activities of this good can be performed at this time period.
Big data has long been found its way into clinical practice since the advent of information technology era. Medical records and follow-up data can be more efficiently stored and extracted with information technology. A patient, immediately after admission, produces a large amount of data including laboratory findings, medications, fluid balance, progressing notes and imaging findings. This is particularly true for critically ill patients requiring intensive care unit (ICU) admission, because vital signs and urine output are recorded on hourly basis for them. In our institution, every bed is equipped with a monitor and a computer so that data on vital signs (e.g., respiratory rate, heart rate, blood pressure and body temperature) and other physiological signals (e.g., extravascular lung water when transpulmonary thermodilution measurement is performed) can be automatically extracted from the monitor and stored in the electronic medical system in a predefined time interval (Figure 1). Furthermore, other clinical observations such as pupil size, Glasgow coma scale (GCS), fluid input and output can be input into the computer on hourly basis. Such “high resolution” data allow extensive studies to explore the effectiveness of certain interventions and independent predictors of clinical outcomes.
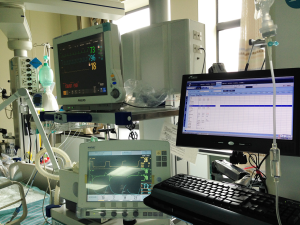
Big data has not been fully utilized yet for clinical research in mainland China, despite the wide use of electronic medical record (EMR) system in the majority of tertiary hospitals. We have previously worked with the department of information technology of our hospital to extract data from electronic record system, and by using these data we carried out several interesting clinical researches (2-4). Researches based on EMR system are feasible and efficient because all data are stored electronically and it saves a lot of time as compared to data extraction by hand. However, our experience is based on single center and the sample size is relatively small (less than 2,000 in our previous studies), which significantly compromised the generalizability of our conclusions. In the future, electronic clinical data may be incorporated from dozens or hundreds of hospitals to form the big data, and studies by using these data will be more generalizable.
However, clinical research based on big data has been widely used in other Western countries (5-8). I personally have been working with some famous databases for a while and herein I would like to share some of my experience on using these databases. The most distinguished database in my experience is the Multiparameter Intelligent Monitoring in Intensive Care database (MIMIC-2), and now it has been updated to version 2.6 (9). This database comprises more than 30,000 ICU patients enrolled between 2001 and 2008 in Beth Israel Deaconess Medical Center (Boston, MA, USA). The information technology required to construct and maintain the database is provided by Massachusetts Institute of Technology (MIT). It is publically available and requires completion of an online training course before one can obtain the full access to the database (10). Access of our team to the database was approved after completion of the NIH web-based training course named “Protecting Human Research Participants” (certification number: 1132877). At first inspection of the dataset, one may be overwhelmed by the large amount of data as it occupied nearly 80 G of the computer disc after decompression. Everything happened during hospital stay is recorded in the database, including demographics, diagnosis based on ICD-9, medications, report of imaging study, vital signs recorded on hourly basis and laboratory findings. Scores for severity of illness such as sequential organ failure assessment (SOFA) and simplified acute physiology score (SAPS) are calculated after secondary analysis of original data.
The exploration of the MIMIC-2 database requires some degree of experience of computer science. One needs a virtual machine to mount the data and extract it from Linux operation system. Data are stored in relational tables that are connected to each other by using the primary key (e.g., icustay_id or hadm_id). Therefore, structure query language (SQL) is mandatory for data extraction which takes the form of “select * from tablename where a condition”. There is a good online material to learn SQL at http://www.w3schools.com/sql/sql_like.asp.
The first work I have done with the MIMIC-2 was to explore the association of urine output and mortality in critically ill patients (11). The idea comes from the fact that while another indicator of renal function serum creatinine has been extensively studied for its association with mortality risk, the urine output has not received so much attention despite the widely accepted notion that urine output should be tightly associated with mortality risk based on clinical experience. The MIMIC-2 database provided a good material to quantitatively establish the association between urine output and mortality risk. After successful completion of the first work, I continued to do several other investigations involving the clinical implications of lactate and ionized calcium (12,13). Up to now, these projects have been completed.
The following section will discuss something about what clinical researches based on big data differ from the randomized controlled trials (RCT). There is no doubt that RCT is at the top of the evidence pyramid and can provide high level evidence on the efficacy of a certain intervention. However, RCT is not a panacea that solves all clinical problems (14). As I have previously mentioned, RCT has at least the following limitations which may be potentially addressed by using big data derived from “real world” setting (15,16). Although some investigators argue that a high quality RCT should be as close to real word as possible, RCT may differ from observational studies in that they provide evidence in biological efficacy of an intervention which may not be translated to clinical effectiveness in “real world” setting (17). Firstly, RCT is performed with strict inclusion/exclusion criteria that maybe only 20% of population of interest is included, and the generalizability of the conclusion to the rest 80% patients is questionable. With aging population, more and more patients encountered in daily clinical practice will have multiple comorbidities that would have been excluded from RCTs. Secondly, interventions performed in RCT may be complex and not applicable to other institutions or routine practice. In contrast, the big data using EMR system provides patients’ information from real world setting and researches based on such design is more applicable to patients encountered in daily practice. Thirdly, some interventions cannot be explored by using RCT due to ethical concerns. Likewise, when an intervention becomes widespread, clinicians are unwilling to “experiment” with alternatives. For instance, the impact of timing of cardiopulmonary resuscitation (CPR) on cerebral function recovery cannot be investigated with controlled trials. However, such studies can be done by using techniques such as propensity score analysis and stratification based on big data. Forth, RCT is generally time consuming and more costly than observational studies based on big data (18). For a novel intervention or diagnostic test whose beneficial effect is largely unknown, preliminary data based on electronic record system or other healthcare data for administration purpose may be needed before large amount of funds can be approved for a well-designed RCT.
In conclusion, clinicians and clinical investigators should make every effort to make full use of the big data that is being continuously generated by EMR system and other healthcare databases. It is totally a waste of resources by leaving electronic data on the disc without exploiting its potential values on revealing mechanisms underlying disease course. Unfortunately, this is a prevailing phenomenon in mainland China. At this stage, more training courses on data management and statistical analysis are required before clinicians and clinical investigators can handle big data and translate them into advances in medical science. I personally feel that it is of much more interests to clinicians to perform clinical research based on big data than to culture cells and feed mice. Of note, China is a large country with a population of 1.3 billion and can contribute greatly to clinical researches by providing reliable and high-quality big data, but now this goal is far from being achieved.
Disclosure: The authors declare no conflict of interest.
References
- Harrell FE Jr, Lee KL, Pollock BG. Regression models in clinical studies: determining relationships between predictors and response. J Natl Cancer Inst 1988;80:1198-202. [PubMed]
- Zhang Z, Xu X, Fan H, Li D, Deng H. Higher serum chloride concentrations are associated with acute kidney injury in unselected critically ill patients. BMC Nephrol 2013;14:235. [PubMed]
- Zhang Z, Xu X, Ni H, Deng H. Red cell distribution width is associated with hospital mortality in unselected critically ill patients. J Thorac Dis 2013;5:730-6. [PubMed]
- Zhang Z, Xu X, Ni H, Deng H. Platelet indices are novel predictors of hospital mortality in intensive care unit patients. J Crit Care 2014;29:885.e1-6.
- Cooke CR, Iwashyna TJ. Using existing data to address important clinical questions in critical care. Crit Care Med 2013;41:886-96. [PubMed]
- Margolis R, Derr L, Dunn M, Huerta M, Larkin J, Sheehan J, Guyer M, Green ED. The National Institutes of Health’s Big Data to Knowledge (BD2K) initiative: capitalizing on biomedical big data. J Am Med Inform Assoc 2014;21:957-8. [PubMed]
- Psaty BM, Breckenridge AM. Mini-Sentinel and regulatory science--big data rendered fit and functional. N Engl J Med 2014;370:2165-7. [PubMed]
- Schneeweiss S. Learning from big health care data. N Engl J Med 2014;370:2161-3. [PubMed]
- Saeed M, Villarroel M, Reisner AT, Clifford G, Lehman LW, Moody G, Heldt T, Kyaw TH, Moody B, Mark RG. Multiparameter Intelligent Monitoring in Intensive Care II: a public-access intensive care unit database. Crit Care Med 2011;39:952-60. [PubMed]
- Scott DJ, Lee J, Silva I, Park S, Moody GB, Celi LA, Mark RG. Accessing the public MIMIC-II intensive care relational database for clinical research. BMC Med Inform Decis Mak 2013;13:9. [PubMed]
- Zhang Z, Xu X, Ni H, Deng H. Urine output on ICU entry is associated with hospital mortality in unselected critically ill patients. J Nephrol 2014;27:65-71. [PubMed]
- Zhang Z, Xu X, Ni H, Deng H. Predictive value of ionized calcium in critically ill patients: an analysis of a large clinical database MIMIC II. PLoS One 2014;9:e95204. [PubMed]
- Zhang Z, Chen K, Ni H, Fan H. Predictive value of lactate in unselected critically ill patients: an analysis using fractional polynomials. J Thorac Dis 2014;6:995-1003. [PubMed]
- Wang SD. Opportunities and challenges of clinical research in the big-data era: from RCT to BCT. J Thorac Dis 2013;5:721-3. [PubMed]
- Zhang Z, Ni H, Xu X. Do the observational studies using propensity score analysis agree with randomized controlled trials in the area of sepsis? J Crit Care 2014;29:886.e9-886.e15.
- Zhang Z, Ni H, Xu X. Observational studies using propensity score analysis underestimated the effect sizes in critical care medicine. J Clin Epidemiol 2014;67:932-9. [PubMed]
- Nallamothu BK, Hayward RA, Bates ER. Beyond the randomized clinical trial: the role of effectiveness studies in evaluating cardiovascular therapies. Circulation 2008;118:1294-303. [PubMed]
- Sibbald B, Roland M. Understanding controlled trials. Why are randomised controlled trials important? BMJ 1998;316:201. [PubMed]