The association of myelination in the internal capsule with iron deposition in the basal ganglia in macaques: a magnetic resonance imaging study
Introduction
Myelin and iron each play an important role in maintaining normal brain function and metabolic activity. In mammalian central nervous systems, myelin is produced by oligodendrocytes (1). Aggregated evidence has shown that myelination of white matter (WM) continues into adulthood, and myelin degeneration occurs with old age (2-4), which brain iron is essential for myelin synthesis throughout the lifespan of mammals (5). Iron deficiency early in life can lead to hypomyelination, while excess iron deposition can cause oxidative stress, inflammation, and demyelination (6). The findings of neuroanatomical studies on brain iron and myelin have been mixed, but have consistently suggested that iron is progressively deposited in the brain with age, and accumulates largely in basal ganglia (BG) regions, where pathological changes often result in a wide range of neurological and psychiatric diseases (7-9). The BG, which notably consists of the globus pallidus (GP), the putamen (PU), the substantia nigra, the subthalamic nucleus, and the caudate nucleus (CN), contains the greatest amount of iron in the brain (10,11). The BG-connecting fiber tract internal capsule (IC) is a highly myelinated WM structure which is anatomically connected to the BG. This structure consists of projection fibers that pass through the BG and is located between the lentiform nucleus (which comprises the PU and GP) laterally, and between the thalamus and CN medially (12,13). The IC can be divided into several structures, including the anterior limb of the internal capsule (ALIC) and the posterior limb of the internal capsule (PLIC). Previous research has demonstrated that there is a close association between myelin and iron (14,15). A relatively high correspondence between iron concentration and myelin content has been observed in most gray matter (GM) regions, whereas there is generally a low correspondence between iron and myelin in WM, the thalamus, and BG regions (11). A previous aging study reported a negative correlation between iron and myelin content in the ventral striatum (16). To date, the relationship between iron concentration and myelin content in the BG and its connecting fiber tracts remains essentially unexplored.
It is technically challenging to perform an in vivo, non-invasive, quantitative measurement of myelin and iron content in the human brain. Magnetic resonance imaging (MRI)-based technologies are considered to be a promising path in finding a resolution for this issue (17,18). Of these MRI-based technologies, calculating myelin water fraction (MWF) using T2* decay signals acquired from a multiple gradient echo (mGRE) sequence is a fast method for whole-brain myelin water imaging (19) in which a three-pool model of WM (20) is used for multi-exponential analysis of T2* decay data. This three-pool model assumes that WM is made up of a myelin water pool (short T2* component), an axonal water pool (medium T2* component), and an extracellular water pool (long T2* component) (21,22). MWF is defined as the ratio of myelin water component to total water component (23). Quantitative susceptibility mapping (QSM), on the other hand, measures the bulk magnetic susceptibility of tissue using phase images of mGRE sequence (24) and reveals the quantity of iron, because iron is the main source of magnetic susceptibility in subcortical GM (25,26). Iron can be transported between different areas of the brain along axons and dendrites (27,28). There are two primary forms of transported iron in the brain: transferrin-bound iron and non-transferrin-bound iron (6). Disorders such as Alzheimer’s disease (AD) and Parkinson’s disease (PD) are known to involve myelin degeneration, which leads to the disruption of brain iron homeostasis (5,29). Nevertheless, the relationship between iron concentration and myelin content in distant but anatomically connected brain regions has rarely been examined, even though an understanding of the connection between iron in the BG and myelin in the IC is crucial for investigating the physiological mechanisms of normal aging and some neurodegenerative diseases further.
In the present study, we measured brain iron concentration in young and old adult macaques using QSM, focusing primarily on BG areas, including the CN, GP, and PU. In addition, using the MWF technique, we measured the myelin content of the BG-connecting fiber tracts, including the ALIC and PLIC. The myelin content of four major WM structures that are anatomically separate from the BG, including the optic tract (OT), and the genu, body, and splenium of the corpus callosum (GCC, BCC, and SCC), were also measured as controls.
Methods
Animal preparation
The subjects of the present study were 16 healthy adult macaques (Macaca fascicularis). The macaques were divided into 2 groups by age: the young group (n=8, 6 males and 2 females), with a mean age of 7.7 (range: 6.7–8.7) years old; and the old group (n=8, 6 males and 2 females), with a mean age of 24.7 (range: 23.4–26) years old. All 16 macaques were prepared similarly to our previous work (30-32) and maintained in a stable physiological state for MRI scanning. Before each scanning session, the animals were anesthetized with an intramuscular injection of ketamine (10 mg/kg) and atropine sulfate (0.05 mg/kg). After intubation, the animals were ventilated with an MRI-compatible ventilator (CWE Inc., Weston, Wisconsin). To ensure a constant respiratory rate (25–35 breaths/min), the macaques were maintained with intermittent positive-pressure ventilation. Local anesthetic (5% lidocaine cream) was applied around the macaques’ ears to block peripheral nerve stimulation. The macaques were then placed in a custom-built MRI-compatible stereotaxic frame in a sphinx position and their heads were secured before being inserted into the center of the scanner. The animals’ vital signs, including blood oxygenation, ECG, rectal temperature (Small Animal Instruments, Inc., Stony Brook, New York), respiratory rate, and end-tidal CO2 (Smiths Medical ASD Inc., Dublin, Ohio) were monitored throughout the experiment. Oxygen saturation was kept at over 95% and body temperature was kept constant (39±1 °C) using a hot water blanket (Gaymar Industries Inc., Orchard Park, New York). During the anesthesia process, lactated Ringer’s solution was given at a maximum rate of 10 mL/kg/hour. All experimental procedures in this study were approved by the Institute of Neuroscience Animal Care and Use Committee and by the Shanghai Institute for Biological Sciences Biomedical Research Ethics Committee, and they all conformed to the National Institutes of Health guidelines for the humane care and use of laboratory animals.
MRI acquisition
MRI images of all subjects were acquired using a 3T whole-body scanner (MAGNETOM Trio, Siemens Healthcare A.G., Erlangen, Germany) with an enhanced gradient insert (AC88; 80 mT/m maximum gradient strength, 800 mT/m/ms maximum slew rate) and a customized eight-channel phased-array coil, which was designed specifically for animal imaging. Image data for QSM and MWF analysis were acquired using a customized 3D mGRE sequence with a bipolar readout gradient. The sequence parameters were as follows: repetition time (TR) 60 ms, the first echo time (TE1) 2.4 ms, echo spacing (ΔTE) 1.42 ms, number of echoes (#Echo) 32, matrix size 128×128×52, acquisition voxel size 1×1×1 mm3, readout bandwidth 930 Hz/pixel, flip angle 25°, 6 averages, and the acquisition time was 40 minutes.
Computation of parametric maps
The processing procedure for the mGRE data was performed in MATLAB (R2014a, MathWorks, Natick, MA, USA). The QSM computation was conducted with the STISuite v3.0 toolbox (https://people.eecs.berkeley.edu/~chunlei.liu/software.html). The 8 echoes of 1, 5, 9, 13, 17, 21, 25, and 29 acquired from the mGRE sequence were chosen to keep the same readout polarity in order to avoid phase fluctuation between even and odd echoes due to echo shift caused by eddy currents. The susceptibility values obtained by this method were verified to strongly correlate with those obtained by traditional QSM protocol (21). Phase images of these echoes were unwrapped using a Laplacian-based phase unwrapping method (33); the V-SHARP method (34) was applied for background field removal; and a fast STAR-QSM algorithm (35) was applied for QSM calculation.
MWF maps were also calculated using mGRE data, employing the proposed method of incorporating local tissue susceptibility to acquire high resolution myelin water imaging (21). A three-component complex model (21) was used to characterize the signal decay in WM as follows:
| [1] |
In this equation, S(t) represents the complex signal as a function of time, and A and f represent signal magnitudes and frequency offsets of each water component, including my (myelin water), ax (axonal water), and ex (extracellular water). φ0 is the initial phase offset. Subsequently, the phase component of the complex data from the mGRE sequence was replaced by the following:
| [2] |
In this equation, χ is the susceptibility value obtained by QSM, γ is the gyromagnetic ratio, and i is the echo number. A complex dataset was created by this new phase component and the magnitude component filtered by 3D anisotropic diffusion filter (ADF), the parameter κ of 3D ADF being 6σ (σ is the noise standard deviation in the 3D volumetric image acquired from the first echo image). This synthesized signal was fitted to Eq. [1]. An iterative nonlinear curve-fitting method (‘lsqcurvefit’ function in MATLAB) was used to estimate the complex model parameters. Table 1 shows the initial values and searching boundaries of each parameter (21,22). Finally, MWF was defined as the fraction of myelin water signal amplitude over the total water signal amplitude; the following equation was used:
| [3] |
Definition of ROIs
Firstly, non-brain tissue was removed using the brain extraction tool (BET) in FSL software (36). To conduct ROI analysis of MWF values in WM and QSM values in the BG, the first echo image acquired from the mGRE sequence was used for segmentation to obtain WM, GM, and cerebrospinal fluid (CSF) tissue maps by means of FSL FAST module (37). To minimize partial volume artifacts, only voxels that had a WM or GM partial volume fraction larger than 0.95 were included in the final binary WM or GM tissue masks.
To measure the MWF and QSM values in well-defined ROIs, the masks of 10 WM ROIs, being the GCC [left (L) and right (R)], BCC (L and R), SCC (L and R), IC (L and R), and OT (L and R), and six BG ROIs, being the CN (L and R), GP (L and R), and PU (L and R), were derived from the INIA19 atlas (38). The IC is V-shaped on the axial (horizontal) sections, with a knee-bend around the apex of the lentiform nucleus. The IC was manually divided into two sections (ALIC and PLIC) using a line at the inflection point (39). All ROIs from the INIA19 atlas were co-registered to the first echo image from the mGRE sequence by SPM8 (Wellcome Trust Centre for Neuroimaging, London, UK). After the ROIs had been inspected for gross registration errors, MWF or QSM values were acquired for all ROIs in each macaque (as shown in Figure 1). Additionally, to further reduce ROI registration errors when applying WM ROIs from the INIA19 atlas, the MWF map was first masked by a WM tissue mask, and when applying BG ROIs from the INIA19 atlas, the QSM was first masked by WM and GM tissue masks, because many regions of BG were attributed as WM by FAST. The mean values of MWF in WM ROIs and the mean values of QSM in BG ROIs were recorded for each macaque.
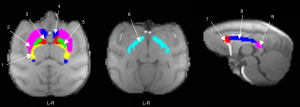
Statistical analysis
Statistical analysis was performed with SPSS Version 20 (IBM Inc., Armonk, NY, USA). After confirming that MWF and susceptibility data in all ROIs were normally distributed, an independent sample t-test was used to investigate differences in MWF and susceptibility between the young and old macaque groups. A paired sample t-test was performed to investigate differences in MWF and susceptibility between the left and right hemispheres. Also, to assess the relationship between myelin in WM ROIs and iron in BG ROIs, Spearman’s rank-order correlation analysis (two-tailed) was performed on the mean susceptibility of BG ROIs and the mean MWF in WM ROIs both in anatomically connected and anatomically separate WM structures. A difference of P<0.05 for any given t-test or Spearman’s correlation was considered statistically significant.
Results
ROI analysis results
Figure 2 shows examples of QSM and MWF maps for two representative macaques, one from the young group and one from the old group. The results of the regional MWF analysis and the regional susceptibility analysis between the young and old groups are summarized in detail in Table 2 and Table 3, respectively. The mean MWF of the old group was larger than that of the young group for each WM ROI, but significant intergroup differences were only found in the ALIC (L: t=3.403, P=0.004; R: t=5.659, P<0.001) and PLIC (R: t=3.634, P=0.003). Looking at the MWF of WM ROIs in the young group, the highest value was in the OT, followed in descending order by the PLIC, BCC, SCC, ALIC, and GCC. This held true for both the left and right hemispheres. However, considering the MWF of WM ROIs in the old group, the highest value was in the ALIC, followed in descending order by the PLIC, OT, BCC, SCC, and GCC. This held true for both left and right hemispheres. Moreover, no significant intergroup differences in MWF values were found between the left and right hemispheres in all ROIs in both the young and old groups. The mean susceptibility values of the old group were significantly higher than those of the young group in the GP (L: t=3.268, P=0.012; R: t=2.698, P=0.029), PU (L: t=4.564, P<0.001; R: t=2.986, P=0.016) and CN (L: t=3.075, P=0.011; R: t=4.860, P=0.001). In both the left and right hemispheres, the mean susceptibility values in the young and the old groups were highest in the GP, followed by the PU and the CN. There were no significant intergroup differences in susceptibility values between the left and right hemispheres in either group.
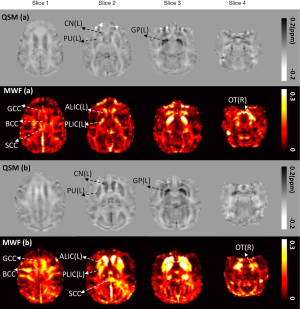
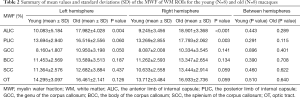
Full table
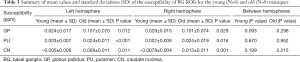
Full table
Correlation analysis results
Spearman’s correlations between the mean MWF of WM ROIs and the mean susceptibility of BG ROIs are presented in Table 4. Figure 3 shows the scatterplots of correlations between the mean MWF of WM ROIs (ALIC and PLIC) and the mean susceptibility of BG ROIs for all macaques.
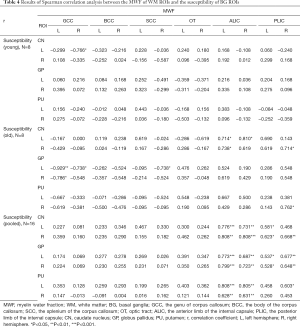
Full table
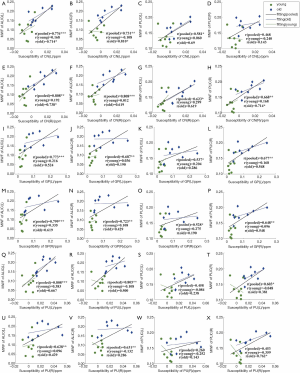
In the young group, a significant correlation (r=−0.766, P=0.027) was only found between the mean MWF of the GCC (R) and mean susceptibility of the CN (L). In the old group, there were significant positive correlations between the mean susceptibility of the CN (L) and the mean MWF of the ALIC (L: r=0.714, P=0.047, Figure 3A; R: r=0.810, P=0.015, Figure 3B), and there were significant positive correlations between the mean susceptibility of the CN (R) and the mean MWF of the ALIC (L: r=0.738, P=0.037, Figure 3E) and the PLIC (R: r=0.714, P=0.047, Figure 3H). There were significant negative correlations between the mean susceptibility of the GP (L) and the mean MWF of the GCC (L: r=−0.929, P=0.001; R: r=−0.738, P=0.037) and the SCC (R: r=−0.738, P=0.037). A significant negative correlation (r=−0.786, P=0.021) was found between the mean susceptibility of the GP (R) and the mean MWF of the GCC (L). There was also a significant positive correlation between mean susceptibility of the PU (R) and mean MWF of the PLIC (R: r=0.762, P=0.028, Figure 3X).
In the pooled group, the MWF of the ALIC (L) was positively correlated with the mean susceptibility of the CN (L: r=0.776, P<0.001, Figure 3A; R: r=0.808, P<0.001, Figure 3E), GP (L: r=0.773, P<0.001, Figure 3I; R: r=0.799, P<0.001, Figure 3M) and PU (L: r=0.808, P<0.001, Figure 3Q; R: r=0.628, P=0.009, Figure 3U), and the MWF of the ALIC (R) was also positively correlated with the mean susceptibility of the CN (L: r=0.731, P=0.001, Figure 3B; R: r=0.808, P<0.001, Figure 3F), GP (L: r=0.687, P=0.003, Figure 3J; R: r=0.723, P=0.002, Figure 3N) and PU (L: r=0.805, P<0.001, Figure 3R; R: r=0.631, P=0.009, Figure 3V). The MWF of the PLIC (L) was positively correlated with the mean susceptibility of the CN (L: r=0.581, P=0.018, Figure 3C); R: r=0.623, P=0.01, Figure 3G) and GP (L: r=0.537, P=0.032, Figure 3K); R: r = 0.528, P=0.035, Fig. 3o), and the MWF of the PLIC (R) was positively correlated with the mean susceptibility of the CN (R: r=0.668, P=0.005, Figure 3H), GP (L: r=0.677, P=0.004, Figure 3L); R: r=0.648, P=0.007, Figure 3P) and PU (L: r=0.603, P=0.013, Figure 3T). Of all the significantly correlated ROIs in the pooled group, the correlation coefficients between the mean MWF of the ALIC and the mean susceptibility of BG ROIs were higher than those between the mean MWF of the PLIC and the mean susceptibility of BG ROIs.
Discussion
To the best of our knowledge, the present study is the first to investigate non-local associations between iron concentration in the BG and myelin content of WM in non-human primates. Our analyses of 16 (8 young and 8 old) macaques demonstrated significant associations between the MWF of the IC and the susceptibility of the CN, the GP, and the PU, while few significant correlations were observed between the susceptibility of the three above-mentioned BG ROIs and the MWF of WM ROIs that are anatomically separate from the BG. These findings deepen the current understanding of the anatomical connection between iron and myelin.
Macaque brains are insusceptible to the psychopathologies that can affect the human brain (40) and are therefore useful models for studying the interactions between myelin and iron under normal conditions, which could better control any biases caused by the potential presence of disease such as AD. Additionally, according to previous human research (2,41), the MWF of WM demonstrates an inverted U-shaped trend with age, with the peak values occurring at approximately the fourth to sixth decade of life. In another study of rhesus macaques (42), the thickness of myelin in layer 4 of the visual cortex was compared between young macaques aged 4 to 9 years and macaques aged 24 years or older, and was found to increase significantly with age. The old macaques, which were comparable to humans of approximately 60 years of age (43), had significantly higher MWF values than the young macaques, which were comparable to humans of approximately 20 years of age (43), in the ALIC (L and R) and PLIC (R). However, no significant differences in MWF were observed between the young and old groups in the GCC, BCC, SCC, and OT, suggesting that myelin is still in the stages of production and maintenance in these WM ROIs. However, previous diffusion tensor imaging studies of brain WM (44) and the optic nerve (45) of macaques showed age-related decreases in fractional anisotropy and increases in radial diffusivity. Another ex vivo diffusion spectrum imaging study (46) found that transcallosal connectivity declined in older macaques, which suggested that brain degeneration had occurred.
Additionally, the mean magnetic susceptibility of the old macaque group was significantly higher than that of the young group in the GP, PU, and CN in both the left and right hemispheres, which suggests that significant deposits of iron occur in these structures with age. This finding is consistent with those of previous human studies (8,9). In the present study, the GP had the highest susceptibility, followed by the PU and CN, in both the young and old macaque groups. This is also consistent with the order in the human brain (10).
Spearman’s correlation analysis demonstrated that the susceptibility of the BG is positively correlated with the MWF of anatomically connected IC structures during myelin production and maintenance. The correlation between the MWF of the ALIC and the susceptibility of the BG is stronger than the correlation between the MWF of the PLIC and the susceptibility of the BG. There was less of a correlation, however, between BG susceptibility and the MWF of anatomically separate WM structures.
The BG is a cluster of neurons located deep beneath the cerebral cortex with a high concentration of iron. The results of this study suggest that iron in the BG is involved in the synthesis and maintenance of myelin in IC structures. A genetic mutant mouse model study found that iron loading influences the expression of myelin-related genes, and that there is preferential iron loading in myelinated tracts of the BG (47). It is interesting to note that the nucleus accumbens, CN, and PU, which are regions with low iron levels, have a high density of transferrin receptors. These regions connect to the GP and the substantia nigra, which are regions with high iron levels. This suggests a possible existing mechanism of iron uptake and delivery through axonal transportation (48). Unfortunately, it is difficult to verify any existing mechanisms between iron in the BG and WM myelin in vivo, and the pathway that iron takes from the BG to regions producing myelin remains unclear. This requires further histological study.
The results of the present study demonstrate no significant relationship between the susceptibility of BG ROIs and the MWF in WM ROIs in the young group. This could be attributed to the small sample size (N=8). The young macaques also exhibited similar or less iron deposition in the BG region than their older counterparts. On the other hand, in the old group there was a significant positive relationship between the susceptibility of the CN and the MWF of the IC (both the ALIC and PLIC), but no significant correlation existed between the MWF values of the IC and the susceptibility of the GP. These results suggest that iron in the CN is more tightly linked with IC myelin than that of the GP and PU, even though iron content in the CN is the least among these three regions. A past tractography study (49) showed that, compared to the GP and PU, most of the CN is connected to the ALIC and has significant connections with the distant PLIC, which might explain the significant correlations between the MWF of IC and the susceptibility of the CN in the present study’s old group.
By using a customized mGRE sequence and proper data post-processing methods, MWF and susceptibility could be measured in the brain of each macaque with a single MRI scan. In the present study, we obtained 32 echoes with 1.42 ms echo spacing. There were 16 echoes with TE
QSM is considered to be a good biomarker for paramagnetic materials in the subcortical GM. However, the susceptibility of WM is partially dependent on the orientation of axons and myelin (52,53); therefore, only the MWF of WM and the susceptibility of the BG were used as valid data sources in the present study, which hindered investigation into the relationship between iron and myelin in local brain regions.
There were several other limitations to this study. First, the sample size was relatively small and the signal-to-noise ratio of images was affected by iron and myelin levels, meaning that subtle changes in the ratio may have been lost in areas with low myelin and iron levels. Also, because the registration tools used are optimized for humans brains and not macaques, when ROIs from the INIA19 atlas were registered to each macaque, registration errors may have occurred, resulting in potential errors in statistical analysis.
Conclusions
In this study, we demonstrated that moderate to high levels of positive correlations exist between the magnetic susceptibility of the BG and the MWF of IC structures that are anatomically connected to the BG. This suggests that future studies should consider the impact of iron concentration in the BG on myelin development in these anatomically connected WM structures. Further physiological studies are needed to understand the underlying processes of iron transportation.
Acknowledgments
Funding: This work was supported by the National Key R&D Program of China (2017YFC1310400; No. 2018YFC1313803), the Strategic Priority Research Program of Chinese Academy of Science (XDB32030000), Shanghai Municipal Science and Technology Major Project (2018SHZDZX05), grants from the National Natural Science Foundation of China (81871428, 81527901, 31771174), Major Scientific Project of Zhejiang Lab (2018DG0ZX01), the Fundamental Research Funds for the Central Universities (2019QNA5026, 2019XZZX001-01-08), and the Zhejiang University Education Foundation Global Partnership Fund.
Footnote
Conflicts of Interest: All authors have completed the ICMJE uniform disclosure form (available at http://dx.doi.org/10.21037/qims-19-1014). The authors have no conflicts of interest to declare.
Ethical Statement: All experimental procedures in this study were approved by the Institute of Neuroscience Animal Care and Use Committee and by the Shanghai Institute for Biological Sciences Biomedical Research Ethics Committee, and they all conformed to the National Institutes of Health guidelines for the humane care and use of laboratory animals.
Open Access Statement: This is an Open Access article distributed in accordance with the Creative Commons Attribution-NonCommercial-NoDerivs 4.0 International License (CC BY-NC-ND 4.0), which permits the non-commercial replication and distribution of the article with the strict proviso that no changes or edits are made and the original work is properly cited (including links to both the formal publication through the relevant DOI and the license). See: https://creativecommons.org/licenses/by-nc-nd/4.0/.
References
- Bradl M, Lassmann H. Oligodendrocytes: biology and pathology. Acta Neuropathol 2010;119:37-53. [Crossref] [PubMed]
- Arshad M, Stanley JA, Raz N. Adult age differences in subcortical myelin content are consistent with protracted myelination and unrelated to diffusion tensor imaging indices. Neuroimage 2016;143:26-39. [Crossref] [PubMed]
- Wang S, Young KM. White matter plasticity in adulthood. Neuroscience 2014;276:148-60. [Crossref] [PubMed]
- Westlye LT, Walhovd KB, Dale AM, Bjornerud A, Due-Tonnessen P, Engvig A, Grydeland H, Tamnes CK, Ostby Y, Fjell AM. Life-span changes of the human brain white matter: diffusion tensor imaging (DTI) and volumetry. Cereb Cortex 2010;20:2055-68. [Crossref] [PubMed]
- Ward RJ, Zucca FA, Duyn JH, Crichton RR, Zecca L. The role of iron in brain ageing and neurodegenerative disorders. The Lancet Neurology 2014;13:1045-60. [Crossref] [PubMed]
- Mills E, Dong XP, Wang F, Xu H. Mechanisms of brain iron transport: insight into neurodegeneration and CNS disorders. Future Med Chem 2010;2:51-64. [Crossref] [PubMed]
- Acosta-Cabronero J, Betts MJ, Cardenas-Blanco A, Yang S, Nestor PJ. In Vivo MRI Mapping of Brain Iron Deposition across the Adult Lifespan. J Neurosci 2016;36:364-74. [Crossref] [PubMed]
- Aquino D, Bizzi A, Grisoli M, Garavaglia B, Bruzzone MG, Nardocci N, Savoiardo M, Chiapparini L. Age-related iron deposition in the basal ganglia: quantitative analysis in healthy subjects. Radiology 2009;252:165-72. [Crossref] [PubMed]
- Bartzokis G, Tishler TA, Lu PH, Villablanca P, Altshuler LL, Carter M, Huang D, Edwards N, Mintz J. Brain ferritin iron may influence age- and gender-related risks of neurodegeneration. Neurobiol Aging 2007;28:414-23. [Crossref] [PubMed]
- Hallgren B, Sourander P. The effect of age on the non-haemin iron in the human brain. J Neurochem 1958;3:41-51. [Crossref] [PubMed]
- Hametner S, Endmayr V, Deistung A, Palmrich P, Prihoda M, Haimburger E, Menard C, Feng X, Haider T, Leisser M, Kock U, Kaider A, Hoftberger R, Robinson S, Reichenbach JR, Lassmann H, Traxler H, Trattnig S, Grabner G. The influence of brain iron and myelin on magnetic susceptibility and effective transverse relaxation - A biochemical and histological validation study. Neuroimage 2018;179:117-33. [Crossref] [PubMed]
- Rea P. Chapter 2 - Essential Anatomy and Function of the Brain. In: Rea P. editor. Essential Clinical Anatomy of the Nervous System. San Diego: Academic Press; 2015: 51-76.
- Johns P. Chapter 3 - Functional neuroanatomy. In: Johns P, editor. Clinical Neuroscience. Churchill Livingstone; 2014: 27-47.
- Todorich B, Pasquini JM, Garcia CI, Paez PM, Connor JR. Oligodendrocytes and myelination: the role of iron. Glia 2009;57:467-78. [Crossref] [PubMed]
- Stüber C, Morawski M, Schäfer A, Labadie C, Wähnert M, Leuze C, Streicher M, Barapatre N, Reimann K, Geyer S, Spemann D, Turner R. Myelin and iron concentration in the human brain: A quantitative study of MRI contrast. NeuroImage 2014;93:95-106. [Crossref] [PubMed]
- Steiger TK, Weiskopf N, Bunzeck N. Iron Level and Myelin Content in the Ventral Striatum Predict Memory Performance in the Aging Brain. J Neurosci 2016;36:3552-8. [Crossref] [PubMed]
- Möller HE, Bossoni L, Connor JR, Crichton RR, Does MD, Ward RJ, Zecca L, Zucca FA, Ronen I. Iron, Myelin, and the Brain: Neuroimaging Meets Neurobiology. Trends Neurosci 2019;42:384-401. [Crossref] [PubMed]
- Zivadinov R, Bergsland N, Dwyer MG. Atrophied brain lesion volume, a magnetic resonance imaging biomarker for monitoring neurodegenerative changes in multiple sclerosis. Quant Imaging Med Surg 2018;8:979-83. [Crossref] [PubMed]
- Du YP, Chu R, Hwang D, Brown MS, Kleinschmidt-DeMasters BK, Singel D, Simon JH. Fast multislice mapping of the myelin water fraction using multicompartment analysis of T2* decay at 3T: a preliminary postmortem study. Magn Reson Med 2007;58:865-70. [Crossref] [PubMed]
- Lancaster JL, Andrews T, Hardies LJ, Dodd S, Fox PT. Three-pool model of white matter. J Magn Reson Imaging 2003;17:1-10. [Crossref] [PubMed]
- Wu Z, He H, Sun Y, Du Y, Zhong J. High resolution myelin water imaging incorporating local tissue susceptibility analysis. Magn Reson Imaging 2017;42:107-13. [Crossref] [PubMed]
- Lee H, Nam Y, Lee HJ, Hsu JJ, Henry RG, Kim DH. Improved three-dimensional multi-echo gradient echo based myelin water fraction mapping with phase related artifact correction. Neuroimage 2018;169:1-10. [Crossref] [PubMed]
- MacKay A, Whittall K, Adler J, Li D, Paty D, Graeb D. In vivo visualization of myelin water in brain by magnetic resonance. Magn Reson Med 1994;31:673-7. [Crossref] [PubMed]
- Wang Y, Liu T. Quantitative susceptibility mapping (QSM): Decoding MRI data for a tissue magnetic biomarker. Magn Reson Med 2015;73:82-101. [Crossref] [PubMed]
- Sun H, Walsh AJ, Lebel RM, Blevins G, Catz I, Lu JQ, Johnson ES, Emery DJ, Warren KG, Wilman AH. Validation of quantitative susceptibility mapping with Perls’ iron staining for subcortical gray matter. Neuroimage 2015;105:486-92. [Crossref] [PubMed]
- Langkammer C, Schweser F, Krebs N, Deistung A, Goessler W, Scheurer E, Sommer K, Reishofer G, Yen K, Fazekas F, Ropele S, Reichenbach JR. Quantitative susceptibility mapping (QSM) as a means to measure brain iron? A post mortem validation study. Neuroimage 2012;62:1593-9. [Crossref] [PubMed]
- Ke Y, Qian ZM. Iron misregulation in the brain: a primary cause of neurodegenerative disorders. Lancet Neurol 2003;2:246-53. [Crossref] [PubMed]
- Wang Z, Zeng YN, Yang P, Jin LQ, Xiong WC, Zhu MZ, Zhang JZ, He X, Zhu XH. Axonal iron transport in the brain modulates anxiety-related behaviors. Nat Chem Biol 2019;15:1214-22. [Crossref] [PubMed]
- Hadzhieva M, Kirches E, Mawrin C. Review: iron metabolism and the role of iron in neurodegenerative disorders. Neuropathol Appl Neurobiol 2014;40:240-57. [Crossref] [PubMed]
- Lv Q, Yang L, Li G, Wang Z, Shen Z, Yu W, Jiang Q, Hou B, Pu J, Hu H, Wang Z. Large-Scale Persistent Network Reconfiguration Induced by Ketamine in Anesthetized Monkeys: Relevance to Mood Disorders. Biol Psychiatry 2016;79:765-75. [Crossref] [PubMed]
- Wang Z, Chen LM, Negyessy L, Friedman RM, Mishra A, Gore JC, Roe AW. The relationship of anatomical and functional connectivity to resting-state connectivity in primate somatosensory cortex. Neuron 2013;78:1116-26. [Crossref] [PubMed]
- Zhang Z, Cai DC, Wang Z, Zeljic K, Wang Z, Wang Y. Isoflurane-Induced Burst Suppression Increases Intrinsic Functional Connectivity of the Monkey Brain. Front Neurosci 2019;13:296. [Crossref] [PubMed]
- Li W, Wu B, Liu C. Quantitative susceptibility mapping of human brain reflects spatial variation in tissue composition. Neuroimage 2011;55:1645-56. [Crossref] [PubMed]
- Wu B, Li W, Guidon A, Liu C. Whole brain susceptibility mapping using compressed sensing. Magn Reson Med 2012;67:137-47. [Crossref] [PubMed]
- Wei H, Dibb R, Zhou Y, Sun Y, Xu J, Wang N, Liu C. Streaking artifact reduction for quantitative susceptibility mapping of sources with large dynamic range. NMR Biomed 2015;28:1294-303. [Crossref] [PubMed]
- Smith SM. Fast robust automated brain extraction. Hum Brain Mapp 2002;17:143-55. [Crossref] [PubMed]
- Zhang Y, Brady M, Smith S. Segmentation of brain MR images through a hidden Markov random field model and the expectation-maximization algorithm. IEEE Trans Med Imaging 2001;20:45-57. [Crossref] [PubMed]
- Rohlfing T, Kroenke CD, Sullivan EV, Dubach MF, Bowden DM, Grant KA, Pfefferbaum A. The INIA19 Template and NeuroMaps Atlas for Primate Brain Image Parcellation and Spatial Normalization. Front Neuroinform 2012;6:27. [Crossref] [PubMed]
- Zarei M, Johansen-Berg H, Jenkinson M, Ciccarelli O, Thompson AJ, Matthews PM. Two-dimensional population map of cortical connections in the human internal capsule. J Magn Reson Imaging 2007;25:48-54. [Crossref] [PubMed]
- Pryluk R, Kfir Y, Gelbard-Sagiv H, Fried I, Paz R. A Tradeoff in the Neural Code across Regions and Species. Cell 2019;176:597-609.e18. [Crossref] [PubMed]
- Faizy TD, Kumar D, Broocks G, Thaler C, Flottmann F, Leischner H, Kutzner D, Hewera S, Dotzauer D, Stellmann JP, Reddy R, Fiehler J, Sedlacik J, Gellissen S. Age-Related Measurements of the Myelin Water Fraction derived from 3D multi-echo GRASE reflect Myelin Content of the Cerebral White Matter. Sci Rep 2018;8:14991. [Crossref] [PubMed]
- Peters A, Sethares C, Killiany RJ. Effects of age on the thickness of myelin sheaths in monkey primary visual cortex. J Comp Neurol 2001;435:241-8. [Crossref] [PubMed]
- Tigges J, Gordon TP, Mcclure HM, Hall EC, Peters A. Survival Rate and Life-Span of Rhesus-Monkeys at the Yerkes-Regional-Primate-Research-Center. Am J Primatol 1988;15:263-73. [Crossref] [PubMed]
- Chen X, Errangi B, Li L, Glasser MF, Westlye LT, Fjell AM, Walhovd KB, Hu X, Herndon JG, Preuss TM, Rilling JK. Brain aging in humans, chimpanzees (Pan troglodytes), and rhesus macaques (Macaca mulatta): magnetic resonance imaging studies of macro- and microstructural changes. Neurobiol Aging 2013;34:2248-60. [Crossref] [PubMed]
- Yan Y, Li L, Preuss TM, Hu X, Herndon JG, Zhang X. In vivo evaluation of optic nerve aging in adult rhesus monkey by diffusion tensor imaging. Quant Imaging Med Surg 2014;4:43-9. [PubMed]
- Meng Y, Zhang X. Transcallosal connectivity changes from infancy to late adulthood: an ex vivo diffusion spectrum imaging study of macaque brains. Brain Connect 2015;5:147-55. [Crossref] [PubMed]
- Heidari M, Johnstone DM, Bassett B, Graham RM, Chua AC, House MJ, Collingwood JF, Bettencourt C, Houlden H, Ryten M, Olynyk JK, Trinder D, Milward EA. Brain iron accumulation affects myelin-related molecular systems implicated in a rare neurogenetic disease family with neuropsychiatric features. Mol Psychiatry 2016;21:1599-607. [Crossref] [PubMed]
- Mash DC, Pablo J, Flynn DD, Efange SMN, Weiner WJ. Characterization and Distribution of Transferrin Receptors in the Rat Brain. J Neurochem 1990;55:1972-9. [Crossref] [PubMed]
- Taljan KAI. Investigations of Anatomical Connectivity in the Internal Capsule of Macaques with Diffusion Magnetic Resonance Imaging. Cleveland State University; 2011.
- Alonso-Ortiz E, Levesque IR, Pike GB. MRI-based myelin water imaging: A technical review. Magn Reson Med 2015;73:70-81. [Crossref] [PubMed]
- Birkl C, Birkl-Toeglhofer AM, Endmayr V, Hoftberger R, Kasprian G, Krebs C, Haybaeck J, Rauscher A. The influence of brain iron on myelin water imaging. Neuroimage 2019;199:545-52. [Crossref] [PubMed]
- Argyridis I, Li W, Johnson GA, Liu C. Quantitative magnetic susceptibility of the developing mouse brain reveals microstructural changes in the white matter. Neuroimage 2014;88:134-42. [Crossref] [PubMed]
- Langkammer C, Krebs N, Goessler W, Scheurer E, Yen K, Fazekas F, Ropele S. Susceptibility induced gray-white matter MRI contrast in the human brain. Neuroimage 2012;59:1413-9. [Crossref] [PubMed]