Chest lesion CT radiological features and quantitative analysis in RT-PCR turned negative and clinical symptoms resolved COVID-19 patients
Introduction
The pneumonia caused by a novel coronavirus (SARS-CoV-2) has been widely studied. It was first reported as an “unknown viral pneumonia” with a limited number in Wuhan, China, in December 2019 (1-3), but spread rapidly across China and the world (4-7). Experts from different fields and different countries are working together to improve the recognition, diagnosis and treatment of this disease. Chest computed tomography (CT) has been proven to be important for the diagnosis and the course monitoring of coronavirus disease 2019 (COVID-19) (8-10). Many studies (11-19) have reported typical CT features, including ground-glass opacity (GGO), consolidation, and/or interstitial changes with a peripheral or bronchovascular bundle distribution. Some studies (17-20) focused on changes in CT features during the active disease course. However, few studies summarized CT features of patients ready for discharge.
For better and more objective understanding of CT features in COVID-19 patients ready for discharge, in this study we describe the CT features of patients with two consecutive negative reverse transcription-polymerase chain reaction (RT-PCR) results and clinical symptoms resolved for more than 3 days, and perform quantitative lesion analysis with an artificial intelligence (AI) software.
We present the following article in accordance with the STROBE reporting checklist (available at http://dx.doi.org/10.21037/qims-20-531).
Methods
The study was approved by the ethics committee of the First Affiliated Hospital of China Medical University (No. AF-SOP-07-1.1-01). Informed consent was waived due to the retrospective nature of this study.
Study population
From February 10 to March 10, 2020, data of 125 COVID-19 patients ready for discharge (with two consecutive negative RT-PCR results and clinical symptoms resolved for more than 3 days) (21) at the Fangcang shelter hospital in Hongshan Gymnasium, Wuhan, Hubei Province, were reviewed retrospectively. All patients were mild cases from the onset and during the course of hospitalization, as defined with no hypoxemia or respiratory distress (respiratory rate ≥30 breaths/min, requirement for oxygen treatment or mechanical ventilation, or SpO2 ≤93% on room air) (21). Patient’s sex and age, dates of initial symptom onset and pre-discharge two consecutive negative RT-PCR samplings, dates of the pre-discharge CT and follow-up CT were recorded.
CT scan
One hundred and twenty-five patients underwent the pre-discharge CT 1–3 days after the second negative RT-PCR test, and 44 patients underwent the follow-up CT 2–13 days later (Figure 1). CT scans were performed with a mobile CT Unit (CT-NeuVz Prime, NEUSOFT, China) in inspiratory period. The scan parameters included the following: tube voltage of 120 kVp, tube current of 100–200 mA, detector collimation of 64 or 128×0.625 mm, field of view of 350 mm × 350 mm, and matrix size of 512×512. All images were reconstructed using a medium sharp reconstruction algorithm with a slice thickness of 5 mm and an interval of 1 mm.
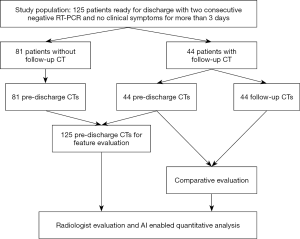
Chest CT image analysis
CT features
Two radiologists (Reader 1 and Reader 2 with 5 and 15 years of experience in chest CT diagnosis, respectively) reviewed all CT images together slice-by-slice. When inconsistency occurred, another radiologist (Reader 3) with 30 years of chest CT diagnosis experience participated, and the final reading was based on consensus. Specifically, the following features were evaluated: (I) GGO, (II) consolidation, (III) fibrosis, (IV) fibrous stripes, (V) lungs and lobes involved, (VI) distribution (categorized as peribronchovascular only, subplural only, peribronchovascular predominant, subplural predominant, random). Patients’ other lung manifestations included: (VII) reticulation, (VIII) air bronchogram, (IX) thickened bronchial wall, (X) thickened small blood vessels, and (XI) nodules. Extrapulmonary manifestations included: (XII) pleural effusion, (XIII) mediastinal lymph nodes (categorized as <10, 10–15, >15 mm in short-axis length).
GGO was defined as hazy increased lung attenuation with preservation of bronchial and vascular margins, whereas consolidation was defined as opacification with obscuration of the margins of vessels and airway walls (22). Fibrosis was defined as heterogeneous density with rigid or concave margins, with or without pleural and bronchial contraction (23).
Quantitative analysis
An AI-assisted screening and epidemic intelligence monitoring system for COVID-19 pneumonia, InferRead™ CT Pneumonia software (Beijing Infervision Technology Co., Ltd., Beijing, China), was used for quantitatively analyzing chest lesions on all CT scans (i.e., 125 pre-discharged scans and 44 follow-up scans). The system automatically segmented the lungs in chest CT images, identified lesions and classified lesions into seven categories according to their CT attenuation values (–570 to –470 HU, –470 to –370 HU, –370 to –270 HU, –270 to –170 HU, –170 to –70 HU, –70 to 30 HU, 30 to 60 HU), and calculated the involved lesions’ volumes and their percentages lung volume.
Statistical analysis
Statistical analysis was performed using IBM SPSS Statistics software (version 23.0; IBM, New York, USA). Categorical variables are presented as number and percentage. Quantitative variables are shown as the mean ± standard deviation (minimum, maximum). Comparisons between paired quantitative variables of pre-discharged and follow-up scans were carried out using the Wilcoxon signed-rank test. A P value <0.05 was considered statistically significant.
Results
The patient cohort included 63 men and 62 women (average age: 48±13 years, range: 16–67 years). The interval from the onset of initial symptoms to the pre-discharge CT was 22±10 days (Table 1).
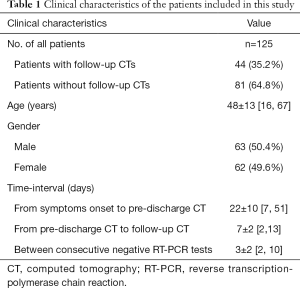
Full table
Among the 125 pre-discharge CTs, residual lesions were found in 105 cases (84.0%). 70 (66.7%) had were bilateral lungs involvement, while 19/16 (18.1%/15.2%) had right or left lung involvement only. The most common lesion distributions are subplural predominant (33/105, 31.4%) and subplural only (29/105, 27.6%); while the most common lesions appearances were GGO (99/125, 79.2%) and fibrosis (56/125, 44.8%) (Tables 2,3 and Figure 2). Other observable CT findings included fibrous stripe, reticulation, air bronchogram, consolidation, nodule, thickened bronchial wall, and thickened small blood vessel. Thirty-nine CTs (31.2%) displayed mediastinal lymph node of 10–15 mm, and 6 CTs (4.8%) had mediastinal lymph node short-axis diameter >15 mm. No pleural effusion was found in all 125 patients.
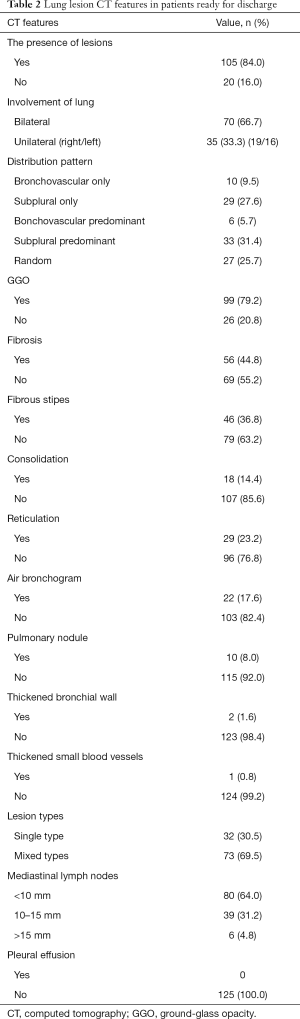
Full table
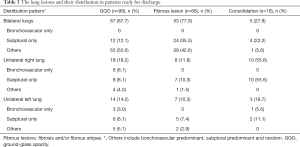
Full table
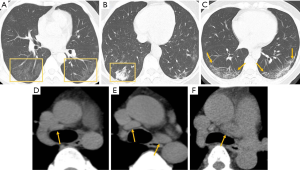
Among the 44 follow-up CTs, 41 cases (93.2%) showed further improvement in lung lesion involvement with GGO decrease in density, consolidation fading or shrinking in size, and development of fibrosis (Figures 3,4). The other three cases have unchanged lung CT findings compared with pre-discharge CTs. The enlarged mediastinal lymph nodes (short-axis diameter >10 mm) were found in 22 cases among the 44 follow-up CTs, and they did not change in appearance during the follow-up.
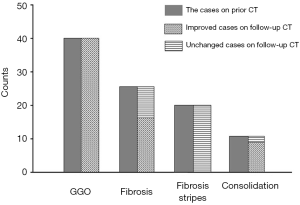
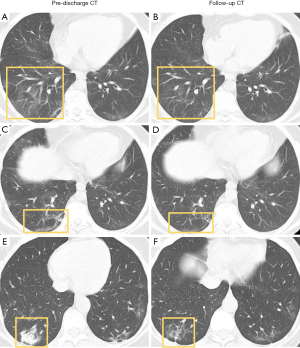
Among the 125 pre-discharge CTs, AI enabled quantitative analysis showed 59 cases (47.2%) had residual lesions. As shown in Table 4, the average volume was 26.20±62.97 cm3 for both lungs inclusive, accounting for 0.57%±1.38% of the whole lung. The right lower lobe had the significant largest involved volume (12.01±35.87 cm3). The highest proportion of the lesions were in the CT-value of −570 to −470 HU. Among 66 patients who AI software did not found lesions, 46 (69.7%) were recognized to have small and very low density GGOs by radiologists.
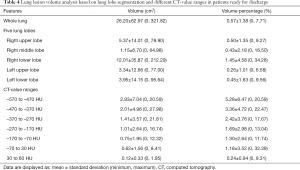
Full table
In 44 cases had follow-up, the involved volume decreased significantly reduced on follow-up CTs (Table 5). Figure 5 shows an example of AI enabled analysis results of GGO decrease in size and density on follow-up CT.
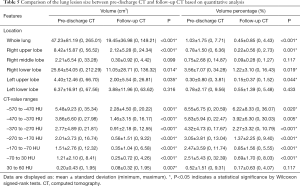
Full table
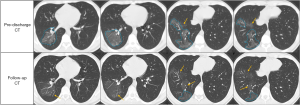
Discussion
In this study, we described the lung lesion CT features for COVID-19 patients when they were ready for discharge from the Fangfang shelter hospital after their clinical symptoms resolved for more than 3 days and RT-PCR results were negative for two consecutive times. Our results show GGO and fibrosis were the most common lesions and they further regressed during follow-up. Enlargement of the mediastinal lymph node was observed and unchanged during follow-up.
GGO was the main lesion manifestation for patients ready for discharge, with lower density and more homogenous without “crazy paving” pattern in contrast to the early stage of COVID-19 (17). Pan et al. (17) found that consolidation gradually disappear, with GGO and subpleural parenchymal bands remaining after 2 weeks. Our 44 follow-up CTs confirmed the changes: the original GGO further reduced or even disappeared; the fibrosis on pre-discharge CT changed to GGO during follow-up. The thinner and more homogenous GGO could be the remnant of GGO, or remnant of consolidation or fibrosis after partial absorption (24,25).
Fibrosis was the second common lesion manifestation, characterized with heterogeneous density and contraction (23). We observed in our 44 follow-up cases that the fibrosis was changed from consolidation, and could further change to GGO (26). Fibrinous exudation in the alveolar lumen has been reported in recent COVID-19 pathological studies (27), which might be the pathological basis for fibrosis on imaging. As a marked feature, reticulation is widely observed in fibrosis (Figure 2). Different from the “crazy paving” in GGO at the early stage of the disease, the reticulation in the fibrosis appeared to be more rigid and thicker. The reticulated structures may also continue to be absorbed until they disappear, as we observed in the 44 follow-up CTs.
Consolidation was not common for patients ready for discharge. Pan et al. (17) reported changes in chest CT findings in COVID-19 patients during the time course, noting that consolidation reached its peak at approximately 10 days after the onset of initial symptoms and was gradually absorbed after 14 days. In this study, CT exams were performed at 22 days after the onset of the initial symptoms. Our patients mostly show absence or small consolidation.
Mediastinal lymphadenopathy has not commonly been observed in the early stage of COVID-19 (12-15,17-19). Xiong et al. (28) found increased number of enlarged lymph nodes during follow-up in the course of the disease. In our study, enlargement of the mediastinal lymph node was observed in 45 patients (36%), especially with a diameter between 1.0 and 1.5 cm, with no changes on follow-up CT. Thus, lymph node involvement might occur later than lung involvement and change slowly in COVID-19 patients.
The data obtained from AI enabled quantitative analysis were broadly consistent with the radiological interpretation during follow-up period. At present, subjective segmental analysis is commonly applied to evaluate disease severity, which, as a semi-quantitative method, is not sufficiently precise. AI enabled analysis provides the quantitative volume and percentage for both of the whole lung and each lobe and of different CT-values representing different types of lesions. Previous studies (17,28,29) found that different types of lesions appeared in different stages of the disease. Such quantification is valuable for follow-up studies observing COVID-19 chest lesion resolving. However, this study shows the AI tool used in this study was not sensitive to detect GGO with very low density (Figure 5). In 46 (69.7%) patients with AI results of 0, small and thin GGOs on the image were observed by radiologists. This might be related to the density interval of the observation setting of the software. At present, the histogram provided by AI is distributed between –570 and 60 HU, meaning a GGO with density less than –570 HU cannot be detected.
There are some limitations in this study. First, it was a single center study including only one Fangcang shelter hospital, and the number of cases who had follow-up was limited. Second, only initially mild patients were included. Third, with a scanning thickness of 5 mm, some details may not be as good as those obtained with thin slices. However, the scanning protocol was in accordance with the workflow of the Fangcang shelter hospital, with multiple CT examinations for each patient and relatively limited medical staff. Actually, the analysis in this study may suggest thin-cut CT is not required for COVID-19 chest lesion evaluation. As multiple CT scans were involved, we advocate minimize radiation exposure to patients (30). In fact, low radiation dose mode and techniques to minimise the radiation dosage could have been applied to the patients, particularly to the younger patients (31,32).
In conclusion, the main CT features were GGO and fibrosis for COVID-19 patients ready for discharge, and further regressed after follow-up.
Acknowledgments
Funding: This work was supported by the National Financial Appropriation Research Project (2017YFC1309100) and National Scientific Foundation of China (81971695).
Footnote
Reporting Checklist: The authors have completed the STROBE reporting checklist. Available at http://dx.doi.org/10.21037/qims-20-531
Data Sharing Statement: Available at http://dx.doi.org/10.21037/qims-20-531
Conflicts of Interest: All authors have completed the ICMJE uniform disclosure form (available at http://dx.doi.org/10.21037/qims-20-531). YXJW serves as an unpaid Editor-In-Chief of Quantitative Imaging in Medicine and Surgery. The other authors have no conflicts of interest to declare.
Ethical Statement: The study complied with the Declaration of Helsinki and approved by the ethics committee of the First Affiliated Hospital of China Medical University (No. AF-SOP-07-1.1-01). Informed consent was waived due to the retrospective nature of this study.
Open Access Statement: This is an Open Access article distributed in accordance with the Creative Commons Attribution-NonCommercial-NoDerivs 4.0 International License (CC BY-NC-ND 4.0), which permits the non-commercial replication and distribution of the article with the strict proviso that no changes or edits are made and the original work is properly cited (including links to both the formal publication through the relevant DOI and the license). See: https://creativecommons.org/licenses/by-nc-nd/4.0/.
References
- Zhu N, Zhang D, Wang W, Li X, Yang B, Song J, Zhao X, Huang B, Shi W, Lu R, Niu P, Zhan F, Ma X, Wang D, Xu W, Wu G, Gao GF, Tan W. China Novel Coronavirus Investigating and Research Team. A Novel Coronavirus from Patients with Pneumonia in China, 2019. N Engl J Med 2020;382:727-33. [Crossref] [PubMed]
- Li Q, Guan X, Wu P, Wang X, Zhou L, Tong Y, Ren R, Leung KSM, Lau EHY, Wong JY, Xing X, Xiang N, Wu Y, Li C, Chen Q, Li D, Liu T, Zhao J, Liu M, Tu W, Chen C, Jin L, Yang R, Wang Q, Zhou S, Wang R, Liu H, Luo Y, Liu Y, Shao G, Li H, Tao Z, Yang Y, Deng Z, Liu B, Ma Z, Zhang Y, Shi G, Lam TTY, Wu JT, Gao GF, Cowling BJ, Yang B, Leung GM, Feng Z. Early transmission dynamics in Wuhan, China, of novel coronavirus-infected pneumonia. N Engl J Med 2020;382:1199-207. [Crossref] [PubMed]
- Huang C, Wang Y, Li X, Ren L, Zhao J, Hu Y, Zhang L, Fan G, Xu J, Gu X, Cheng Z, Yu T, Xia J, Wei Y, Wu W, Xie X, Yin W, Li H, Liu M, Xiao Y, Gao H, Guo L, Xie J, Wang G, Jiang R, Gao Z, Jin Q, Wang J, Cao B. Clinical features of patients infected with 2019 novel coronavirus in Wuhan, China. Lancet 2020;395:497-506. [Crossref] [PubMed]
- Phan LT, Nguyen TV, Luong QC, Nguyen TV, Nguyen HT, Le HQ, Nguyen TT, Cao TM, Pham QD. Importation and human-to-human transmission of a novel coronavirus in Vietnam. N Engl J Med 2020;382:872-4. [Crossref] [PubMed]
- Holshue ML, DeBolt C, Lindquist S, Lofy KH, Wiesman J, Bruce H, Spitters C, Ericson K, Wilkerson S, Tural A, Diaz G, Cohn A, Fox L, Patel A, Gerber SI, Kim L, Tong S, Lu X, Lindstrom S, Pallansch MA, Weldon WC, Biggs HM, Uyeki TM, Pillai SK. Washington State 2019-nCoV Case Investigation Team. First case of 2019 novel coronavirus in the United States. N Engl J Med 2020;382:929-36. [Crossref] [PubMed]
- Giovanetti M, Benvenuto D, Angeletti S, Ciccozzi M. The first two cases of 2019-nCoV in Italy: Where they come from? J Med Virol 2020;92:518-21. [Crossref] [PubMed]
- World Health Organization. Statement on the second meeting of the International Health Regulations (2005) Emergency Committee regarding the outbreak of novel coronavirus (2019-nCoV). 2020. Available online: . Accessed April 4, 2020.https://www.who.int/news-room/detail/30-01-2020-statement-on-the-second-meeting-of-the-international-health-regulations-(2005)-emergency-committee-regarding-the-outbreak-of-novel-coronavirus-(2019-ncov)
- Xie X, Zhong Z, Zhao W, Zheng C, Wang F, Liu J. Chest CT for typical 2019-nCoV pneumonia: relationship to negative RT-PCR testing. Radiology 2020.200343. [Crossref] [PubMed]
- Fang Y, Zhang H, Xie J, Lin M, Ying L, Pang P, Ji W. Sensitivity of chest CT for COVID-19: comparison to RT-PCR. Radiology 2020.200432. [Crossref] [PubMed]
- Ai T, Yang Z, Hou H, Zhan C, Chen C, Lv W, Tao Q, Sun Z, Xia L. Correlation of chest CT and RT-PCR testing in coronavirus disease 2019 (COVID-19) in China: a report of 1014 cases. Radiology 2020.200642. [Crossref] [PubMed]
- Hu X, Chen J, Jiang X, Tao S, Zhen Z, Zhou C, Wang J. CT imaging of two cases of one family cluster 2019 novel coronavirus (2019-nCoV) pneumonia: inconsistency between clinical symptoms amelioration and imaging sign progression. Quant Imaging Med Surg 2020;10:508-10. [Crossref] [PubMed]
- Kanne JP, Chest CT. Findings in 2019 Novel Coronavirus (2019-nCoV) Infections from Wuhan, China: Key Points for the Radiologist. Radiology 2020;295:16-7. [Crossref] [PubMed]
- Pan Y, Guan H. Imaging changes in patients with 2019-nCov. Eur Radiol 2020. Epub ahead of print. [Crossref] [PubMed]
- Ng MY, Lee EYP, Yang J, Yang F, Li X, Wang H, MM, Lo CSY, Leung B, Khong PL, Hui CKM, Yuen K, Kuo MD. Imaging profile of the COVID-19 infection: radiologic findings and literature review. Radiology: Cardiothoracic Imaging. doi: . [Crossref]
- Pan Y, Guan H, Zhou S, Wang Y, Li Q, Zhu T, Hu Q, Xia L. Initial CT findings and temporal changes in patients with the novel coronavirus pneumonia (2019-nCoV): a study of 63 patients in Wuhan, China. Eur Radiol 2020. Epub ahead of print. [Crossref] [PubMed]
- Sun Z, Zhang N, Li Y, Xu X. A systematic review of chest imaging findings in COVID-19. Quant Imaging Med Surg 2020;10:1058-79. [Crossref]
- Pan F, Ye T, Sun P, Gui S. Liang B1, Li L, Zheng D, Wang J, Hesketh RL, Yang L, Zheng C. Time course of lung changes on chest CT during recovery from 2019 novel coronavirus (COVID-19) pneumonia. Radiology 2020;295:715-21. [Crossref] [PubMed]
- Chung M, Bernheim A, Mei X, Zhang N, Huang M, Zeng X, Cui J, Xu W, Yang Y, Fayad ZA, Jacobi A, Li K, Li S, Shan H. CT imaging features of 2019 novel coronavirus (2019-nCoV). Radiology 2020;295:202-7. [Crossref] [PubMed]
- Song F, Shi N, Shan F, Zhang Z, Shen J, Lu H, Ling Y, Jiang Y, Shi Y. Emerging 2019 novel coronavirus (2019-nCoV) pneumonia. Radiology 2020;295:210-7. [Crossref] [PubMed]
- Shi H, Han X, Zheng C. Evolution of CT manifestations in a patient recovered from 2019 novel coronavirus (2019-nCoV) pneumonia in Wuhan, China. Radiology 2020;295:20. [Crossref] [PubMed]
- China National Health Commission. Diagnosis and treatment of pneumonitis caused by new coronavirus (trial version 7). Available online: . Accessed April 4, 2020.http://www.gov.cn/zhengce/zhengceku/2020-03/04/content_5486705.htm
- Hansell DM, Bankier AA, MacMahon H, McLoud TC, Müller NL, Remy J. Fleischner Society: glossary of terms for thoracic imaging. Radiology 2008;246:697-722. [Crossref] [PubMed]
- Salvatore M, Smith ML. Cross sectional imaging of pulmonary fibrosis translating pathology into radiology. Clin Imaging 2018;51:332-6. [Crossref] [PubMed]
- Wong KT, Antonio GE, Hui DS, Ho C, Chan PN, Ng WH, Shing KK, Wu A, Lee N, Yap F, Joynt GM, Sung JJ, Ahuja AT. Severe acute respiratory syndrome: thin-section computed tomography features, temporal changes, and clinicoradiologic correlation during the convalescent period. J Comput Assist Tomogr 2004;28:790-5. [Crossref] [PubMed]
- Lu PX, Wang YX, Zhou BP, Ge Y, Zhu WK, Chen XC, Ran XG. Radiological features of lung changes caused by avian influenza subtype A H5N1 virus: report of two severe adult cases with regular follow-up. Chin Med J (Engl) 2010;123:100-4. [PubMed]
- Radiology Association of Chinese Medical Association. The COVID-19’s radiological diagnosis: expert recommendation of Radiology Branch of Chinese Medical Association (first edition). Available online: . Accessed March 25, 2020.http://rs.yiigle.com/yufabiao/1180115.htm
- Xu Z, Shi L, Wang Y, Zhang J, Huang L, Zhang C, Liu S, Zhao P, Liu H, Zhu L, Tai Y, Bai C, Gao T, Song J, Xia P, Dong J, Zhao J, Wang FS. Pathological findings of COVID-19 associated with acute respiratory distress syndrome. Lancet Respir Med 2020;8:420-2. [Crossref] [PubMed]
- Xiong Y, Sun D, Liu Y, Fan Y, Zhao L, Li X, Zhu W. Clinical and high-resolution CT features of the COVID-19 infection: comparison of the initial and follow-up changes. Invest Radiol 2020;55:332-9. [Crossref] [PubMed]
- Shi H, Han X, Jiang N, Cao Y, Alwalid O, Gu J, Fan Y, Zheng C. Radiological findings from 81 patients with COVID-19 pneumonia in Wuhan, China: a descriptive study. Lancet Infect Dis 2020;20:425-34. [Crossref] [PubMed]
- Wang YXJ, Liu WH, Yang M, Chen W. The role of CT for Covid-19 patient's management remains poorly defined. Ann Transl Med 2020;8:145. [Crossref] [PubMed]
- Kalra MK, Maher MM, Rizzo S, Kanarek D, Shepard JA. Radiation exposure from chest CT: issues and strategies. J Korean Med Sci 2004;19:159-66. [Crossref] [PubMed]
- Jin S, Zhang B, Zhang L, Li S, Li S, Li P. Lung nodules assessment in ultra-low-dose CT with iterative reconstruction compared to conventional dose CT. Quant Imaging Med Surg 2018;8:480-90. [Crossref] [PubMed]