Evaluation of multi-shell diffusion MRI acquisition strategy on quantitative analysis using multi-compartment models
Introduction
Multi-shell diffusion MRI (dMRI) allows for characterizing the water diffusion signal behavior with low, moderate, and high diffusion-weighting and analyzing the dMRI data using multi-compartment diffusion models such as the Composite Hindered and Restricted Model of Diffusion (CHARMED) (1) and Neurite Orientation Dispersion and Density Imaging (NODDI) models (2,3). Data analysis with multi-compartment models can provide more specific characterization of brain tissue microstructures (like axonal diameter, neurite density and dispersion, or myelin g-ratio) than conventional single-shell diffusion tensor imaging (DTI) (1,2,4-7). The NODDI models the brain tissue with three compartments, and has been increasingly used recently in neuroscience and biomedical research as it can provide specific information about neurite orientation dispersion and density in the brain (4,8,9). The NODDI raw data can be collected using two-shell high angular resolution diffusion imaging (HARDI) protocol, and data acquisition with more HARDI shells could result in smaller bias and variance (2).
As the scanning duration for multi-shell dMRI data acquisition is proportional to the total number of gradient directions, it can be increased substantially when additional averages are needed to improve image signal-to-noise ratio (SNR) (10). As a result, the data collection becomes very susceptible to motion, and the dMRI data can be compromised with motion artifacts particularly in uncooperative patients like young children without sedation. Also, fast dMRI scan is highly demanded for acute stroke patients in clinical practice. The application of the multi-compartment model analysis may be hindered in clinical studies without using a fast multi-shell MRI protocol for data collection. In order to reduce the scanning duration in each session, several hybrid diffusion imaging (HYDI) schemes (11), in which the inner shell has lest gradient-encoding directions (~30 directions) while the outer shell has the most (~60 directions) for 2–5 shells with b-values of 700–3,000 s/mm2, have been used in adult and children studies (2,4,8,12,13). However, the scanning duration with ~90 gradient encoding directions may be still too long or clinically impractical for some uncooperative patients (like fetuses, infants) or stroke patients.
A novel method to generate sampling schemes for multi-shell dMRI was introduced previously (14). The unique design of such sampling schemes allows for acquisitions on multiple spheres in q-space with good angular distribution on each shell. We hypothesized the multi-shell sampling schemes generated with the novel sampling method would offer an effective and fast MRI protocol for quantitative data analysis with the NODDI diffusion model.
In addition, previous rodent studies demonstrated that the diffusion basis spectrum imaging (DBSI) model can be used to exploit the multi-shell dMRI data and quantitatively assess axonal injury, demyelination and concomitant inflammation as well (15-17). As inflammation is coexisting in many diseases like stroke and multiple sclerosis, further data analysis of the same dMRI data set with DBSI model can provide complementary information beneficial to diagnosis and therapeutic development.
In the present study, different gradient-encoding schemes were generated with the multi-shell sampling method reported by Caruyer et al. [14]. The effects of the different gradient-encoding schemes on quantitative analysis of multi-compartment models (NODDI and DBSI) were evaluated on a clinical 3T setting by using non-human primates.
Methods
Adult macaque monkeys (n=4, 9–12 kg) were scanned under 1–1.5% isoflurane anaesthesia. The monkey breathed spontaneously and its physiological readings (heart beat rate, breathing rate, O2-saturation, blood pressure) were continuously monitored and maintained in normal ranges during each scanning session (18). The monkey head was immobilized using custom-built head holder and placed on the patient table in the supine position. The DWI data of the five gradient encoding schemes were collected from the same adult macaque monkey in one scan session. T1-weigthed images were collected using a 3D MP-Rage sequence for identifying the interested regions during data analysis. The B0 field inhomogeneity was further improved with the approach for scanning primate brains on a clinical 3T after the standard shimming procedure was applied (19).
Multi-shell diffusion weighted images (DWI) were acquired with a Siemens 3T TIM Trio scanner using 8-channel phased-array volume coil (Siemens Medical Solutions, Erlangen, Germany) and an echo planar imaging (EPI) sequence with the voxel size = 1.3 mm × 1.3 mm × 1.3 mm, FOV = 91 mm × 67.6 mm, TE/TR =99/4,800 ms, 34 slices to cover the whole brain, and 4 averages for each gradient-encoding scheme. Generalized auto-calibrating partially parallel acquisition (GRAPPA) factor = 3 was applied. Phase-reversal data acquisition was applied for susceptibility artifact correction of EPI images (20).
The gradient encoding schemes for multi-shell dMRI data collection were generated with the multi-shell sampling method reported by Caruyer et al. previously (http://www.emmanuelcaruyer.com/q-space-sampling.php) (14). These schemes are consisted of 4 different protocols with 4–6 shells and moderate b-values (bmax =1,500 or 2,000 s/mm2) suitable for infant dMRI scans: (I) D32_b1500 (32 directions, bmax =1,500 s/mm2, time of acquisition (TA) = 3 minutes for single average); (II) D32_b2000 (32 directions, bmax =2,000 s/mm2, TA = 3 minutes); (III) D56_b1500 (56 directions, TA =5 minutes); and (IV) D80_b2000 (80 directions, TA =8 minutes). Also, the 2-shell HADRI protocol with 30 directions in each shell (b =1,000, 2,000 s/mm2, TA =5 minutes) (D30_b2000) was acquired for comparison purpose. Each scheme was repeated 4 times for average. The gradient encoding schemes are listed in Table 1. Images were pre-processed with FSL software package (www.fmrib.ox.ac.uk) for motion, eddy-current and distortion correction, and average. NODDI parameters and DBSI and DTI indices were obtained using the previously reported NODDI Matlab toolbox (http://mig.cs.ucl.ac.uk) (2) and DBSI data processing Matlab codes (https://osf.io/rmcjz/) (17) respectively.
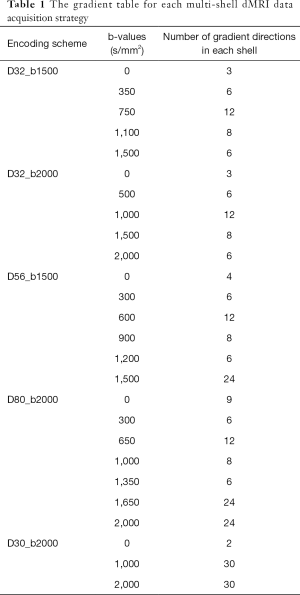
Full table
In order to examine the effects of different encoding schemes on quantification of the NODDI and DBSI indices, the grey matter and white matter structures including prefrontal cortex (PFC), anterior cingulate cortex (ACC), caudate, internal capsule, and corpus callosum were selected as regions of interest (ROIs) for statistical analysis. The anatomical structure of each ROI was identified on FA maps by referring to the corresponding anatomical T1-weighted images (Figure 1). The ROI analysis was conducted using the ImageJ software (imagej.nih.gov). Statistical process was performed using IBM SPSS software.
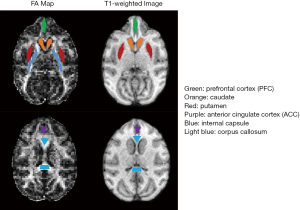
All procedures followed the protocols approved by the Institutional Animal Care and Use Committee (IACUC) of Emory University and were conformed to the NIH Guide for the care and use of Laboratory Animals.
Results
The maps of NODDI indices of one monkey brain, including neurite orientation dispersion index (ODI), intra-cellular volume fraction (ICVF), and volume fraction of Gaussian isotropic diffusion (FISO) from each sampling scheme are demonstrated. As seen in Figure 2, the ODI and FISO maps resemble with each other even though the gradient encoding scheme was changed from 32 directions (bmax =1,500 s/mm2) to 80 directions (bmax =2,000 s/mm2). In contrast, the ICVF maps show stronger dependence on the gradient schemes. The number of detected pixels in white matter is reduced when smaller number of gradient directions in 4–6 shell schemes were applied, while the ICVF results of 2-shell scheme (D30_b2000) were overestimated.
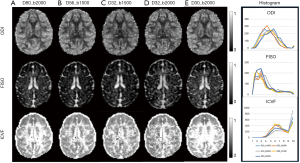
With DBSI model, the hindered diffusion ratios, restricted diffusion ratios, and CSF ratio, were estimated with the same dMRI data set of each subject accordingly. As seen in Figure 3, the maps of hindered diffusion ratio look comparable with each other across 4–6 shells of gradient-encoding schemes (Figure 3A,B,C,D, top row). However, evident difference is seen in the map of the 2-shell scheme (D30_b2000) in comparison with that of the D80_b2000 scheme. As seen in the restricted diffusion ratio maps, the number of detected pixels from lower b-value (b=1,500 s/mm2) schemes are much less than that from higher b-value (b=2,000 s/mm2) schemes. As seen in the maps of fractional anisotropy (FA), mean diffusivity (MD), NODDI indices (ODI, FICVF, FISO), and DBSI indices (hindered, restricted, and CSF ratio) from one slice of data set (D80_b2000) (Figure 4), the grey matter, white matter, and CSF are delineated differently with traditional DTI model, NODDI and DBSI models. In addition, the DTI indices (MD, FA, AD, and RD) are very consistent across these different encoding schemes (data not shown).
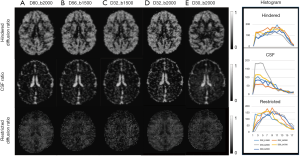
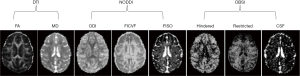
In order to examine the dependence of the DBSI and NODDI models on the gradient schemes, the mean value and pixel count of each index from each scheme is illustrated in Figure 5. Obviously, the CSF and hindered diffusion ratios were underestimated when the 2-shell scheme was applied compared to the 4–6 shell schemes (CSF: 0.25±0.01 vs. 0.32±0.01, Hindered: 0.41±0.01 vs. 0.45±0.02) (Figure 5A). In particular, the pixel numbers in restricted ratio maps of low b-value (bmax =1,500 s/mm2) scheme are dramatically reduced compared to that of high b-value (bmax =2,000 s/mm2) scheme (1,180±100 vs. 1,520±54) (Figure 5B). However, the mean restricted ratios (0.23±0.02 vs. 0.22±0.01) and pixel counts (1,540±70 vs. 1,510±38, one slice) between the schemes of 80 and 32 directions with b=2,000 s/mm2 are comparable.
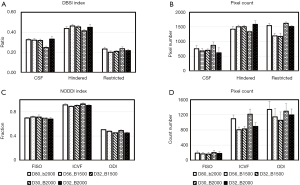
The mean value of each NODDI index in grey matter, white matter, and CSF is illustrated in Figure 5C and is comparable across these schemes. However, the ICVF in white matter was slightly underestimated when the 4–6 shell schemes with low b-value (bmax =1,500 s/mm2) were applied. Also, abnormal ICVF values were seen around and in the ventricles and cortical areas adjacent to the cranium, suggesting the fitting algorithm failed in these regions and further optimization is needed. In addition, the ICVF maps showed reduced pixel counts in white matter when fewer gradient directions were applied [1,100±98 (D80_b2000) vs. 806±70 (D32_b2000), one slice], indicating its strong dependence on the gradient encoding scheme.
In order to investigate the effects of different gradient-encoding schemes on NODDI and DBSI indices, the grey matter and white matter regions of monkey brains were examined for further analysis. The dependence of NODDI indices (ICVF and ODI) on the gradient-encoding schemes in the white matter [internal capsule, corpus callosum (CC)] and grey matter [prefrontal cortex (PFC), caudate] is illustrated in Figure 6.
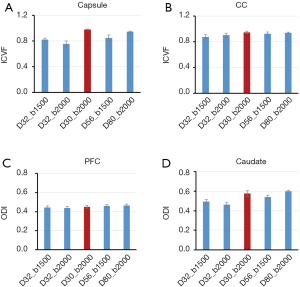
ROI analysis in grey matter and white matter exhibits the differences of ICVF and ODI maps across different sampling schemes (Figure 6). The ICVF values in capsule were underestimated when fewer shells/gradient directions and smaller b-value were applied compared to those with the two-shell (D32_b2000) and 6-shell (D80_b2000) schemes, as similar as seen in ODI maps of caudate. Also, the ICVF in CC and ODI in PFC look consistent across all sampling schemes.
The effects of gradient-encoding schemes on ROI analysis of DBSI indices are evaluated and demonstrated in Figure 7. The maps of hindered diffusion ratios across all sampling schemes look comparable in grey matter (PFC and caudate). The restricted diffusion ratios derived from the 4–6 shell schemes show lower values in white matter when bmax =1,500 s/mm2 was applied, compared to that with bmax =2,000 s/mm2. Also, the restricted diffusion ratios derived from the 2-shell scheme show comparable value with those derived from the gradient encoding schemes with b =2,000 s/mm2.
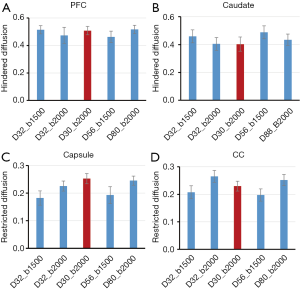
Discussion
Several multi-compartment models have been developed previously to delineate the diffusion behaviour of water in tissue for characterizing the microstructural integrity of the brain with specifications (1,2,5,6,15), showing advantage than conventional single-compartment DTI model in data interpretation. As unique information like axon diameter, neurite density and dispersion, and inflammation can be derived from these multi-compartment models, data analysis using multiple models on the same subject may provide complementary information for extensive examination of microstructural integrity in the brain. In the present report, both NODDI and DBSI models were applied to dMRI data of each multi-shell sampling scheme. The findings suggest that a multi-shell dMRI dataset could be collected with a fast and optimal protocol on a clinical setting, and the same dataset can be applied to the quantitative analysis using multiple diffusion models. In particular, the dMRI data sampling and processing strategy may be particularly useful for whole brain quantitative analysis of microstructural alteration and inflammation in infant or stroke patients.
Traditional DTI assumes a Gaussian diffusion process of water and delineates the diffusion property of tissue with simple scalar indices like FA, MD, axial diffusivity (AD), and radial diffusivities (AD). The DTI model has been used widely and successfully in biomedical research but limited with the lack of specification (21-26). In contrast, multi-compartment models could describe the biophysical process of water diffusion in the brain tissue with more specific information including axonal diameter, myelin g-ratio, neurite density and dispersion (1,2,6,16,27,28).
In the NODDI model, the water diffusion behavior is delineated using a three-compartment model which includes intra-cellular compartment (restricted diffusion), extra-cellular compartment (hindered diffusion), and CSF compartment (isotropic Gaussian diffusion), allowing for quantitative estimation of neurite orientation dispersion index (ODI, index of the degree of dispersion of fiber orientation), intra-cellular volume fraction (ICVF, marker of neurite density), and isotropic volume fraction (FISO, free water or CSF) (2). Previous study demonstrated the dependence of quantification using NODDI indices on the gradient-encoding schemes and suggested NODDI data should be collected with minimal two-shell dMRI protocol while more HARDI shells and gradient-encoding directions would result in smaller bias and variance (2). In previous studies, brain maturation and development of infants or young children were investigated with NODDI using two (8,29) or three (30) HARDI shells with maximal b-values of 1,500, 2,000, or 2,500 s/mm2 and ~90 gradient directions. As the scanning duration of multiple HARDI shells can be too long in clinical practice for uncooperative patients like young children, sedation is usually used to minimize the motion artifacts in NODDI scans of infants (8). Due to the potential risk of anesthesia on brain maturation, sedation can not be generally applied in infant (or fetus) MRI scans in regular clinical practice. A fast dMRI protocol is needed to overcome the limitation of multiple HARDI shell data collection for data analysis using multi-compartment models.
Caruyer et al. reported a novel multi-shell sampling method in which the angular distribution in each shell is optimal while the uniform coverage in global angular distribution is maintained as well (14). In the present report, we used Caruyer’s design to generate 4,6-shell gradient-encoding schemes with the acquisition time of a single scan lasted for 3 to 8 minutes. As demonstrated in the present study, similar ODI and FISO maps are exhibited in all the gradient-encoding schemes (Figure 2, top row), suggesting these indices may be derived from an MRI scan with as less as 3 minutes. In contrast, the ICVF maps are different across the schemes, showing its strong dependence on the numbers of shells and gradient-encoding directions and strength.
DBSI describes the diffusion behavior with three components including restricted diffusion (cellularity), hindered diffusion (vasogenic edema), and free diffusion (CSF), and these parameters can be derived from 9- shell dMRI data (15,31). In the present study, the same multi-shell dMRI data sets were further analyzed using the DBSI model. The maps of hindered diffusion ratios and CSF ratios look very comparable across these 4–6 shell schemes (Figure 3A,B,C,D, top row) while the result from the 2-shell scheme show more bias compared to the scheme D80_b2000 (Figure 3E, top row). In contrast, the pixel numbers of restricted diffusion ratio in white matter increases accordingly when higher b-value was applied (Figure 3A,B,C,D, bottom row). The values of restricted diffusion ratios vary in different ROIs of white matter bundles and sampling schemes (Figure 7), indicating regional dependence and sensitivity to the gradient encoding strategy. The ROI analysis of NODDI and DBSI indices in macaque brains demonstrated the reproducibility and dependence of these indices on the gradient-encoding schemes and the regional difference in grey matter and white matter.
In the previous DBSI study of mice, 99 gradient-encoding directions and 9 shells with bmax =1,000 s/mm2 were applied (31). Our results suggest that the DBSI indices could be derived reasonably with as less as 4-shell and 32 directions (bmax =2,000 s/mm2) for fast data collection on a clinical 3T. Also, the maps of DTI, NODDI, DBSI indices from a single slice (Figure 4), demonstrate that each diffusion model provides specific and unique information to delineate the grey matter and white matter microstructures. The combination use of these models (and other biophysical models) may provide complementary information for extensively examining the abnormality of the brain.
The current results suggest that dMRI data with the b-value of 2,000 s/mm2 can be acquired for both NODDI and DBSI analysis. The 4-shell scheme (D32_b2000) substantially reduced scanning duration, suggesting this data acquisition strategy may be useful for clinical scanning of infants (or even fetal brains) in which fast imaging is required. This data analysis approach may be particularly useful in pediatric research as the grey matter and white matter maturation and developmental abnormalities in infant brains can be examined with different biophysical modelling from one quick dMRI scan, providing complementary information about grey matter, white matter, and inflammation in the brain. As brain injury in acute stroke is always associated with inflammation and edema, the combined use of NODDI and DBSI models may be particularly useful to examine the brain infarct evolution in clinical and preclinical studies.
Although the present work of image acquisition and data analysis approach was preliminarily demonstrated for reproducibility and robustness using healthy non-human primate brains on a 3T scanner, the approach is translational to neuroimaging examination of human subjects in which fast imaging is required for clinical diagnosis and examination as the monkeys show similar brain anatomy and diffusivity properties with human. However, how the derived parameters from multiple diffusion models are correlated with human brain neuropathology will require future studies with specific disease models.
Conclusions
The present study demonstrates that a novel multi-shell gradient-encoding scheme can be used for fast dMRI data acquisition and quantitative analysis using both NODDI and DBSI models (in addition to the conventional DTI and diffusion kurtosis imaging (DKI) models) in preclinical and clinic studies. Such dMRI data acquisition and processing strategy allows for deriving multiple specific parameters from a single dMRI data set and may be used to examine the abnormality of brain tissue such as demyelination, axonal degeneration and inflammation which are coexisting in many diseases. In particular, our work introduces a fast dMRI scanning protocol which could be beneficial for characterizing the brain abnormalities of pediatric patients (infants) and acute stroke patients.
Acknowledgments
The authors thank Ruth Connelly and Doty Kempf (DVM) for animal care and handling.
Funding: The study is supported the Office of Research Infrastructure Programs/OD P51OD011132.
Footnote
Conflicts of Interest: All authors have completed the ICMJE uniform disclosure form (available at http://dx.doi.org/10.21037/qims.2020.03.11). XZ serves as an unpaid editorial board member of Quantitative Imaging in Medicine and Surgery. The other authors have no conflicts of interest to declare.
Ethical Statement: All procedures followed the protocols approved by the Institutional Animal Care and Use Committee (IACUC) of Emory University and were conformed to the NIH Guide for the care and use of Laboratory Animals.
Open Access Statement: This is an Open Access article distributed in accordance with the Creative Commons Attribution-NonCommercial-NoDerivs 4.0 International License (CC BY-NC-ND 4.0), which permits the non-commercial replication and distribution of the article with the strict proviso that no changes or edits are made and the original work is properly cited (including links to both the formal publication through the relevant DOI and the license). See: https://creativecommons.org/licenses/by-nc-nd/4.0/.
References
- Assaf Y, Basser PJ. Composite hindered and restricted model of diffusion (CHARMED) MR imaging of the human brain. Neuroimage 2005;27:48-58. [Crossref] [PubMed]
- Zhang H, Schneider T, Wheeler-Kingshott CA, Alexander DC. NODDI: practical in vivo neurite orientation dispersion and density imaging of the human brain. Neuroimage 2012;61:1000-16. [Crossref] [PubMed]
- Winston GP. The potential role of novel diffusion imaging techniques in the understanding and treatment of epilepsy. Quant Imaging Med Surg 2015;5:279-87. [PubMed]
- Kunz N, Zhang H, Vasung L, O'Brien KR, Assaf Y, Lazeyras F, Alexander DC, Huppi PS. Assessing white matter microstructure of the newborn with multi-shell diffusion MRI and biophysical compartment models. Neuroimage 2014;96:288-99. [Crossref] [PubMed]
- Assaf Y, Blumenfeld-Katzir T, Yovel Y, Basser PJ. AxCaliber: a method for measuring axon diameter distribution from diffusion MRI. Magn Reson Med 2008;59:1347-54. [Crossref] [PubMed]
- Alexander DC, Hubbard PL, Hall MG, Moore EA, Ptito M, Parker GJ, Dyrby TB. Orientationally invariant indices of axon diameter and density from diffusion MRI. Neuroimage 2010;52:1374-89. [Crossref] [PubMed]
- West KL, Kelm ND, Carson RP, Alexander DC, Gochberg DF, Does MD. Experimental studies of g-ratio MRI in ex vivo mouse brain. Neuroimage 2018;167:366-71. [Crossref] [PubMed]
- Batalle D, O'Muircheartaigh J, Makropoulos A, Kelly CJ, Dimitrova R, Hughes EJ, Hajnal JV, Zhang H, Alexander DC, Edwards AD, Counsell SJ. Different patterns of cortical maturation before and after 38 weeks gestational age demonstrated by diffusion MRI in vivo. Neuroimage 2019;185:764-75. [Crossref] [PubMed]
- By S, Xu J, Box BA, Bagnato FR, Smith SA. Application and evaluation of NODDI in the cervical spinal cord of multiple sclerosis patients. Neuroimage Clin 2017;15:333-42. [Crossref] [PubMed]
- Meng Y, Zhang X. In vivo diffusion spectrum imaging of non-human primate brain: initial experience in transcallosal fiber examination. Quant Imaging Med Surg 2014;4:129-35. [PubMed]
- Wu YC, Alexander AL. Hybrid diffusion imaging. Neuroimage 2007;36:617-29. [Crossref] [PubMed]
- Billiet T, Madler B, D'Arco F, Peeters R, Deprez S, Plasschaert E, Leemans A, Zhang H, den Bergh BV, Vandenbulcke M, Legius E, Sunaert S, Emsell L. Characterizing the microstructural basis of "unidentified bright objects" in neurofibromatosis type 1: A combined in vivo multicomponent T2 relaxation and multi-shell diffusion MRI analysis. Neuroimage Clin 2014;4:649-58. [Crossref] [PubMed]
- Timmers I, Roebroeck A, Bastiani M, Jansma B, Rubio-Gozalbo E, Zhang H. Assessing Microstructural Substrates of White Matter Abnormalities: A Comparative Study Using DTI and NODDI. PLoS One 2016;11:e0167884. [Crossref] [PubMed]
- Caruyer E, Lenglet C, Sapiro G, Deriche R. Design of multishell sampling schemes with uniform coverage in diffusion MRI. Magn Reson Med 2013;69:1534-40. [Crossref] [PubMed]
- Wang X, Cusick MF, Wang Y, Sun P, Libbey JE, Trinkaus K, Fujinami RS, Song SK. Diffusion basis spectrum imaging detects and distinguishes coexisting subclinical inflammation, demyelination and axonal injury in experimental autoimmune encephalomyelitis mice. NMR Biomed 2014;27:843-52. [Crossref] [PubMed]
- Assaf Y, Freidlin RZ, Rohde GK, Basser PJ. New modeling and experimental framework to characterize hindered and restricted water diffusion in brain white matter. Magn Reson Med 2004;52:965-78. [Crossref] [PubMed]
- Spees WM, Lin TH, Sun P, Song C, George A, Gary SE, Yang HC, Song SK. MRI-based assessment of function and dysfunction in myelinated axons. Proc Natl Acad Sci U S A 2018;115:E10225-34. [Crossref] [PubMed]
- Li CX, Patel S, Auerbach EJ, Zhang X. Dose-dependent effect of isoflurane on regional cerebral blood flow in anesthetized macaque monkeys. Neurosci Lett 2013;541:58-62. [Crossref] [PubMed]
- Zhang X, Kirsch JE, Zhong X. Artifact correction in diffusion MRI of non-human primate brains on a clinical 3T scanner. J Med Primatol 2016;45:21-7. [Crossref] [PubMed]
- Andersson JLR, Skare S, Ashburner J. How to correct susceptibility distortions in spin-echo echo-planar images: application to diffusion tensor imaging. Neuroimage 2003;20:870-88. [Crossref] [PubMed]
- Zhang X, Yan Y, Tong F, Li C, Jones B, Wang S, Meng Y, Muly E, Kempf D, Howell L. Progressive Assessment of ischemic injury to white matter using diffusion tensor imaging: A preliminary study of a macaque model of stroke. Open Neuroimag J 2018;12:30-41. [Crossref] [PubMed]
- Meng Y, Jiang J, Bachevalier J, Zhang X, Chan AW. Developmental Whole Brain White Matter Alterations in Transgenic Huntington's Disease Monkey. Sci Rep 2017;7:379. [Crossref] [PubMed]
- Mori S, Zhang J. Principles of diffusion tensor imaging and its applications to basic neuroscience research. Neuron 2006;51:527-39. [Crossref] [PubMed]
- Li L, Preuss TM, Rilling JK, Hopkins WD, Glasser MF, Kumar B, Nana R, Zhang X, Hu X. Chimpanzee (Pan troglodytes) precentral corticospinal system asymmetry and handedness: a diffusion magnetic resonance imaging study. PLoS One 2010;5:e12886. [Crossref] [PubMed]
- Li C, Zhang X, Komery A, Li Y, Novembre FJ, Herndon JG. Longitudinal diffusion tensor imaging and perfusion MRI investigation in a macaque model of neuro-AIDS: a preliminary study. Neuroimage 2011;58:286-92. [Crossref] [PubMed]
- Yan Y, Li L, Preuss TM, Hu X, Herndon JG, Zhang X. In vivo evaluation of optic nerve aging in adult rhesus monkey by diffusion tensor imaging. Quant Imaging Med Surg 2014;4:43-9. [PubMed]
- Barazany D, Basser PJ, Assaf Y. In vivo measurement of axon diameter distribution in the corpus callosum of rat brain. Brain 2009;132:1210-20. [Crossref] [PubMed]
- Scherrer B, Warfield SK. Parametric representation of multiple white matter fascicles from cube and sphere diffusion MRI. PLoS One 2012;7:e48232. [Crossref] [PubMed]
- Mah A, Geeraert B, Lebel C. Detailing neuroanatomical development in late childhood and early adolescence using NODDI. PLoS One 2017;12:e0182340. [Crossref] [PubMed]
- Dean DC 3rd, Planalp EM, Wooten W, Adluru N, Kecskemeti SR, Frye C, Schmidt CK, Schmidt NL, Styner MA, Goldsmith HH, Davidson RJ, Alexander AL. Mapping White Matter Microstructure in the One Month Human Brain. Sci Rep 2017;7:9759. [Crossref] [PubMed]
- Wang Y, Wang Q, Haldar JP, Yeh FC, Xie M, Sun P, Tu TW, Trinkaus K, Klein RS, Cross AH, Song SK. Quantification of increased cellularity during inflammatory demyelination. Brain 2011;134:3590-601. [Crossref] [PubMed]