Spatial density and diversity of architectural histology in prostate cancer: influence on diffusion weighted magnetic resonance imaging
Introduction
Prostate cancer is known to frequently manifest as a multifocal disease with high inter- and intra-tumoral heterogeneity in histologic grade (1). Clinically, prostate cancer pathology is interpreted using the modern Gleason grading system, which characterizes histologic morphology ranging from discrete well-formed glands to lack of glandular formation or single cell patterns (2). Risk stratification in prostate cancer has a large component based on histologic presence of cancer with “Gleason pattern 4”, which encompasses specific features such as cribriform, fused, and poorly formed glands (2). However, these patterns are not individually reported despite the evidence that they carry differential prognostic implications (3). Previously, McKenney et al. have characterized a set of distinct architectural growth patterns that comprehensively detail histologic presentation of prostate cancer and validated their independent association to cancer recurrence after surgery in a large patient cohort (Canary architectural analysis) (4).
Beyond the need for more optimized pathologic grading, undersampling of disease at biopsy leads to underestimation of clinical risk prior to surgical intervention. Multiparametric MRI (mpMRI) for disease localization prior to biopsy demonstrates higher detection of clinically significant disease (Gleason 3+4 or higher) and detects fewer clinically insignificant cancers (5,6). The relationship between MRI imaging features and prostate histological characteristics has been well-studied (7). Numerous groups demonstrated a moderate inverse relationship between MR imaging [T2, apparent diffusion coefficient (ADC)] and Gleason score, secondary to the association of each with nuclear density (7,8). Other work demonstrates mpMRI detection varies across broad patterns of poorly formed, cribriform and fused architectures, and lesions containing cribriform architecture are poorly identified (9). However, the influence of all individual prostate cancer architectures on imaging signal, particularly those described by McKenney et al., have yet to be described. Therefore, the objective of this study is to assess the spatial distribution of prostate cancer architecture and their influence on imaging characteristics in a cohort of prostate cancer patients undergoing mpMRI prior to radical prostatectomy.
Methods
Patient population
This retrospective pilot study included 15 prostate cancer patients who underwent mpMRI prior to radical prostatectomy between 2009 and 2014. This population represents a subset of patients included in prior studies evaluating correlation of tissue characteristics on digital pathology and mpMRI imaging (10). Patients included in this study underwent further pathologic assessment for detailed characterization of disease morphology. This study is compliant with local IRB and HIPAA guidelines.
mpMRI acquisition and interpretation
MR images were acquired at 3-Tesla (Achieva 3.0T-TX, Phillips Healthcare, Best, Netherlands) using a combination of the anterior half of a 32-channel cardiac SENSE coil (InVivo, Gainesville, FL, USA) and an endorectal coil (BPX-30, Medrad, Pittsburgh, PA, USA) filled with 45 mL perfluorocarbon-based fluid (Fluorinert, 3M, Maplewood, MN, USA) or a 32-channel cardiac SENSE coil (InVivo, Gainesville, FL, USA). Briefly, the imaging protocol includes T2W (axial, coronal, sagittal), diffusion-weighted imaging (DWI) for derivation of ADC using a mono-exponential decay model, and dynamic contrast-enhanced (DCE) MRI. Full acquisition parameters for scans obtained with coil and without coil are provided in Table S1. Following clinical evaluation, whole prostate and transition zone regions were annotated by a single expert genitourinary radiologist with >10 years’ experience.
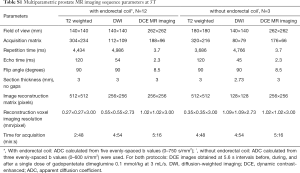
Full table
Pathology specimen processing and digitization
Highly standardized imaging and imaging-pathology correlation was made possible with a 3D-printed mold derived from radiologist annotations for precise sectioning of prostate specimen based on mpMRI imaging of each patient, ensuring that the tissue blocks correspond to the MR imaging sections (11). Following tissue fixation after surgical resection of the prostate, the tissue specimen was serially sectioned within the mold from apex to base at 6-mm intervals. Tissue blocks then underwent paraffin embedding, cutting and mounting on a glass slide for clinical assessment. Hematoxylin and eosin (H&E) slides of whole-mount specimens were digitized using a Zeiss AxioScan slide scanner at 20× magnification (pixel resolution 0.4972 µm).
Pathologic morphology interpretation and annotation
Morphological assessment of prostate cancer architecture was performed by a single expert genitourinary pathologist with >15 years’ extensive experience in prostate cancer morphology, blinded to clinical imaging and information. Twenty-one distinct morphological patterns, based on the Canary architectural analysis, and their predictive value to risk of biochemical recurrence following radical prostatectomy previously defined in (4), were listed in Table 1. Briefly, these morphological patterns represent the spectrum of architectural patterns observed within prostate cancer histology, ranging from well-formed glands of varying sizes and crowding to complex cribriforming and sheets of individual cells without gland formation. Patterns were further sub-grouped by prognosis, as determined by correlation to outcome in original Canary data, into high (worse prognosis), intermediate (indeterminate/non-independent prognosis), and low (favorable prognosis). All pattern definitions and prognosis groups are listed in Table 1.
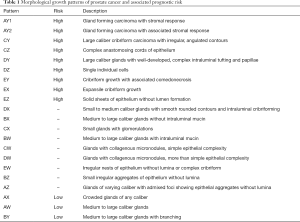
Full table
Local annotations were mapped using a stylus touchscreen computer to encompass the architectural subtype and area of regions containing specific morphological features. Local regions of interest (ROIs) were automatically derived from the manual annotations by fitting a region encompassing the annotation area and assigned to single morphological class using the bio-formats package for MATLAB (12,13). For each local ROI, the extent (mask region), center-of-mass, morphological class, and foci assignment were recorded at a 32:1 ratio from 20× resolution digital image. In addition to morphological annotations, Gleason grading was performed within each focus visualized on individual slices to assess the association of Gleason scoring with local morphology diversity and distribution (Figure 1). ROIs mapping the extent of tumor foci, i.e., mapping tumor boundaries, were created using a semi-automated tool at the 4× resolution level (14).
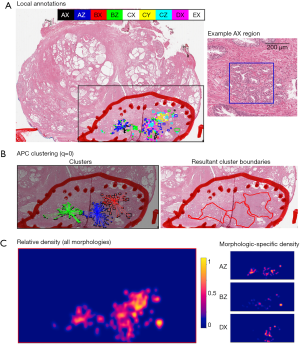
Spatial analysis of morphological growth patterns
Several spatial analyses were performed to assess local and regional diversity of morphological features, including density mapping, spatial clustering, and spatial diversity representation.
A relative density map of morphological pattern distribution was derived from extent of all local ROIs for each foci on whole-mount slides using spatstat package in R (version 1.56-1). These density maps represent the points per unit area of observed spatial patterns. All points (pixels) contained within local ROIs were considered for density mapping. Density maps were calculated using an isotropic Gaussian smoothing filter with σ=17 to reflect differences in spatial sampling between MR (0.273 mm) and digital annotations at high resolution (15.94 µm). Density maps were created for two separate purposes (I) all patterns present within individual foci and (II) morphological classes within individual foci.
Affinity propagation clustering (APC) was used to identify geospatial clusters of local morphology ROIs within individual foci using the apcluster library in R (version 1.4.7). For this, center-of-mass locations of each local ROI were used to ensure even representation of individual annotations. Sensitivity of cluster assignment was tested by varying the q parameter (q=0, 0.1, and 0.5), where smaller values of q tend to produce fewer number of clusters. Cluster assignment for each local ROI was recorded. Following APC assignment, cluster ROI boundaries were determined by fitting a boundary to encompass all member morphological ROIs.
Diversity metrics were assessing presence and abundance of morphological class representation across different regions (patient, foci, and APC clusters) using vegan library in R. This includes the Richness (number of unique classes present in region), Shannon’s diversity index (probability of predicting morphology class given randomly selected annotation from region), Evenness (relative abundance of morphological classes within a region), and Simpson’s diversity index (probability that two randomly selected annotations are of the same morphological class). Full derivation and equations of diversity metrics are available in Supplemental file. Beta diversity was calculated to characterize variation in morphological class diversity in communities across a region. This was tested in two community-region pairs, Gleason grade vs. foci and foci vs. APC clusters, to evaluate inter- and intra-foci diversity, respectively.
Digital pathology-mpMRI registration and analysis
The use of an endorectal coil during mpMRI acquisition and multiple steps within tissue preparation, including fixation and slicing, requires the use of slice-based registration. Prior to registration, whole prostate annotations on MR were used to crop T2W imaging to the boundary extent of the prostate gland (Figure 2). Digital slide images of 4× resolution were resampled to the resolution and orientation of the prostate gland on T2W imaging. A control point co-registration pattern was implemented in MATLAB utilizing similar techniques to previously published prostate radio-pathomic work (15,16). In this work, 50–100 control points were selected for each prostate slide and corresponding MR T2W slice. Control points corresponding to external ink mapping, peripheral-transition zone boundaries, and urethra. When available, additional points corresponding to large intraprostatic cysts, benign hyperplastic nodules, or ejaculatory ducts were used. All control points were evaluated jointly by an imaging scientist and pathology fellow with >2 years experience in prostate imaging and specimen evaluation. Registration transformations were estimated using local weighted mean transformation from fitgeotrans function (MATLAB version 2018b), using weighting from 20–35 neighboring points depending on the total number of control points per slice. Following registration, all resampling and registration transformations were applied to ROIs of individual morphological annotations and relative density maps.
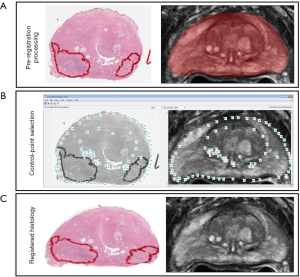
Quantitative diffusion characteristics were compared to morphological density and diversity across multiple levels. Two region-based analyses were evaluated considering individual foci (tumor) regions and individual cluster (APC q=0) regions. For all levels of ROI and cluster-based analysis, minimum ADC (ADCmin) was reported. Finally, diversity characteristics specific to each region-based assessment were correlated to ADC metrics. Voxel-based analysis was completed within all voxels contained within tumor foci to evaluate the relationship between ADC and tumor morphology. For each voxel, the presence and density of any morphology annotation (binary) was considered, as well as the presence and density of all individual morphologies.
Statistical analysis
Prevalence of all architectural morphologies present in patient samples and diversity metrics (Shannon, Simpson, Evenness, Richness) across corresponding foci-level Gleason Grades were summarized using descriptive statistics. Inter- and intra-foci diversity were reported using descriptive statistics. Foci- and cluster-level correlation between ADC metrics and diversity metrics were evaluated using Spearman Correlation Coefficient. All 95% confidence intervals (CIs) were determined based on 2,000 bootstrap samples at the patient level to account for intra-patient, inter-foci and inter-cluster correlation. Presence of high-risk morphologies vs. ADC metrics were evaluated across all foci using Wilcoxon Rank Sum test for clustered data using Rosner-Lynne-Glee method. Association of morphology pattern and density with ADC within all voxels of tumor foci was evaluated using a linear mixed effects model with morphology pattern and density as fixed effects and nested random effects to account for intra-patient, intra-level, intra-ROI correlation.
Results
Population summary
In total, 52 block slides from 15 patients, median PSA 7.33 (2.96–13.02) ng/mL and median age 64 [52–70] years, were included this study. Spatial analysis of morphology distribution included 4,550 annotations from 111 distinct slide-level foci. Each slide-level foci received an individual Gleason score, ranging from 3+3 (N=63), 3+4 (N=37), 4+3 (N=9), and 4+4 (N=2). The prevalence of morphological patterns observed in patients, slides, and tumor foci are summarized in Table 2. As expected, the proportion of high-risk morphological patterns contained within individual foci increased with increasing Gleason grade while the proportion of low-risk patterns decreased (Figure S1). For statistical considerations, Gleason 4+3 and 4+4 tumors were grouped for subsequent analysis.
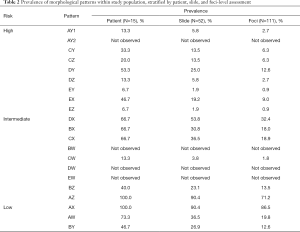
Full table
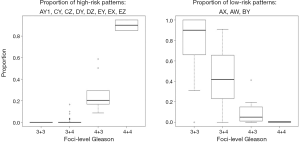
Morphological diversity
Qualitatively, richness, number of unique morphological patterns present within an individual foci, increased with increasing Gleason grade (Table 3). Similarly, Simpson’s diversity index, the probability of two randomly selected annotations from a single foci having the same morphology, decreased according to Gleason grading. Within these foci, an imbalanced representation of morphological classes were noted, with Shannon’s diversity index increasing with higher Gleason grades. Evenness did not demonstrate differences across different Gleason grades.

Full table
From the 111 foci, APC clustering revealed 201, 260, and 445 unique clusters for q=0, q=0.1, and q=0.5, respectively. Following prior works, q=0 was selected for further analysis as it tends towards the fewest number of sub-clusters. For the final analysis using q=0, the median number of clusters per foci were 2 (range, 1–12) with median community size (i.e., number of morphological ROIs per cluster) of 13 (range, 1–120). Summary statistics for q=0.1 and q=0.5 clusters are provided in Table S2.
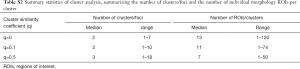
Full table
When evaluating inter-foci diversity, beta diversity estimates were modestly higher in Gleason 3+3 tumors as compared to Gleason 3+4 and 4+3/4+4 foci (Table 4), indicating that the diversity was disproportionate across foci-level ROIs of 3+3 grade. Conversely, alpha diversity was significantly higher in Gleason 4+3/4+4 foci, indicating that the local diversity dominates across foci-level ROIs for this grade. However, evaluating intra-foci diversity demonstrates that beta diversity is higher within APC clusters from higher-grade tumors, indicating regional existence of the morphological heterogeneity within foci of higher Gleason grades. This is reflected in the tendency for high-risk patterns tended to cluster together, with higher representation when present within cluster compared to representation within foci (Table S3). The same was observed for low-risk patterns.
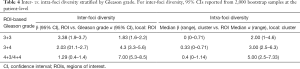
Full table

Full table
Correlation to mpMRI
Of 52 total block slides, 46 were successfully registered to mpMRI with remaining 6 slides removed due to tissue processing artifacts that resulted in poor landmark identification for control point mapping. A representative example of foci-level and cluster-level regions mapped to mpMRI for quantification is shown in Figure 3. ADC metrics within each tumor foci demonstrated an inverse relationship to Gleason grade for each focus and was similarly decreased in foci containing high-risk morphological patterns (Figure 4), though not significant (P=0.2). ADC was further sub-stratified when considering intra-foci clusters containing high-risk patterns (median ADCmin 829 when high-risk present vs. median ADCmin 1,217.5 when high-risk absent, P=0.0013). Correlation of foci-level diversity with ADC metrics were consistent between foci-level and cluster-level metrics, with Shannon index most notably showing a moderate inverse correlation with diffusion characteristics (Table 5).
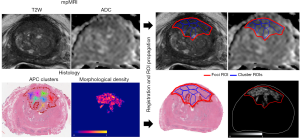
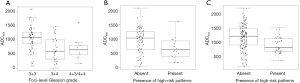
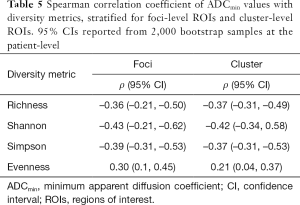
Full table
To further understand the influence of morphological patterns and diffusion characteristics, mixed effects model of voxel-based ADC values across morphology presence and density was assessed. Voxels within tumor foci that contained annotations demonstrated significantly decreased ADC, which further significantly decreased with increasing density of any morphological pattern (Table S4). Furthermore, this negative relationship was significantly dependent on the presence and density of specific morphological patterns within each voxel (ANOVA, chi-squared coefficient 2,332.6, DF =32, P<2.2×10–16). The influence of density and presence of individual morphological patterns on ADC within tumor foci is summarized in Table 6. In total, the presence of an individual morphology pattern was associated with significantly lower ADC for 8/17 patterns observed in this patient population (AW, AZ, BZ, CX, DX, AY1, CY, DZ). Furthermore, increasing density of individual morphologies demonstrated an additional negative relationship (decrease) in ADC for 6/17 patterns (AX, AZ, BZ, AY1, CY, DY) with modest, yet insignificant, negative association in Dx. Half of high-risk patterns (AY1, CY, DY, DZ) incurred a negative relationship with ADC either by presence or density within all voxels of tumor foci, reflecting prior ROI- and foci-level analysis. Specifically within the presence of high-risk cribriforming patterns, only presence of large caliber cribriform growth with irregularly infiltrative patterns (CY) show an enhanced negative relationship with ADC with increasing density. This was similar to observed behavior of glands forming carcinoma with stromal response (AY1), which demonstrated an increasingly negative relationship with ADC.
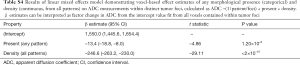
Full table
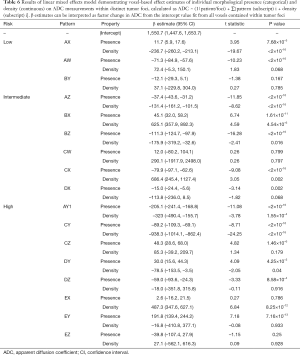
Full table
Discussion
Prostate cancer is observed as a spectrum of histological features at histopathological assessment. Prior work by McKenney et al. was able to fully characterize the spectrum of observed histology architectures within prostate cancer and validated their prognostic relevance with regard to time to recurrence following radical prostatectomy (Canary architectural analysis) (4). In this study, we aimed to further study the spatial relationship and diversity of architectural patterns with respect to quantitative characteristics on clinical imaging at tumor foci, intra-foci, and voxel-level analyses. Within this patient cohort, we find regional and local diversity of morphology influences diffusion characteristics of prostate cancer, with both density and morphologic appearance affecting signal intensity.
By assessing the diversity across the entire spectrum of observed architectural patterns within a whole-mount specimen, we have quantitatively characterized the degree to which morphologic diversity is present within foci of similar pathologic grades. In a quantitative study by Iczkowski et al., the presence of cribriform formation within tumor volumes was associated with worse outcome, which effect was greatly enhanced in 17 patients who demonstrated cribriform architecture accounting for at least one third of the tumor volume (3). Regionally the number of represented morphologies (Richness) observed in our study increased in higher-grade tumors, but this was observed with the tendency for a dominant pattern (Shannon diversity) to be present, in agreement with Iczkowski et al.’s localized findings. Furthermore, we additionally demonstrate the spatial diversity of these growth patterns varies more regionally within a tumor than it does focally, as determined by cluster-based analysis.
In this study we have observed morphological heterogeneity occurring at varying scales within and across tumors. Correlation of tumor grade and morphology with medical imaging can be summarized as either regional (at the level of the entire tumor volume) vs. quantitative characteristics at local scales. The level of correlation also differs by the level of detail defined on pathology including overall grade, individual presence of morphology, or microenvironmental features. Voxel-based spatial correspondence between digital pathology and mpMRI for quantitative intra-lesion assessment of disease heterogeneity requires accurate registration. Within the relatively sparse literature, previously published methods lack critical involvement of a pathologist in detailing intra-prostatic points of spatial co-localization (16,17). We attempted to control for variation due to registration inconsistencies by highly detailed control point selection with a pathologist and use of previously published methodology (16).
For region-based correlation of MRI to pathology, moderate relationship between prostate cancer grade and MRI characteristics has been well-established in the literature (7,18). Beyond overall grade, prior studies have further indicated that specific morphological growth architectures can influence MRI characteristics. Work by Truong et al. suggested that lesions with cribriform architecture are more likely to be missed on prospective mpMRI assessment (9,19). McGarry et al. similarly reported a complex relationship between morphology and ADC characteristics, illustrating Gleason 4 cribriform areas have a moderate, unstable inverse relationship with diffusion restriction. While our analysis was limited to voxels within tumor foci, the methodology of our study allows us to additionally bridge qualitative and quantitative works by assessing the influence of granular architectures on local imaging features. Our study is concordant with prior works in that cribriform patterns demonstrate a complex modulation of ADC, with some establishing a robust inverse relationship (Cy, Dx) when present that can further enhanced with increasing density. However, others (Ex, Ey) demonstrate a positive relationship to ADC, potentially in support of Truong et al. findings.
Kwak et al. (10,17) and Hectors et al. (20) have demonstrated the cellular components of histology strongly correlate to diffusion characteristics. Several large studies have demonstrated correlation of ADC values with Gleason grade. Langer et al. have demonstrated differential diffusion characteristics in “sparse” compared to “dense” tumors, based on semi-quantitative estimation by expert pathologists assessing the dominant composition of tumor regions by cancer cells vs. intermixed normal prostate tissue (21). Dense tissues demonstrated significantly restricted diffusion characteristics compared to sparse and normal tissues, the latter two of which did not show significant differences between each other. These studies all indicate that tissue composition and density within tumor regions may influence perceivable imaging characteristics. We have investigated this relationship with higher specificity to prognostic significance by assessing the influence of different structural patterns, where we observed both the presence and density of each architecture modulates the correlation with imaging signal. This is particularly noted in cribriform patterns. While we only assessed diffusion characteristics in this small study, ADC is a known correlate of tissue microenvironment related to glandular structure (8). Further assessment of the differential influence on presence and density of morphology in larger patient cohorts is warranted to understand the biophysical relationship between architectural morphology and its influence on imaging.
The heterogeneity of prostate cancer patterns (and thus Gleason grade) across tumors is well known, particularly in tumors with higher-grade (1). This is inherently due to the nature of Gleason scoring, which reports the two highest grades observed within a single foci and where each grade encompasses a variety of morphologic patterns (2). The prevalence of all morphologies evaluated in this study were previously reported within a larger patient population (4). This study demonstrates higher frequency of pattern presence, indicating a higher-grade heterogeneity within patients, likely due to the inclusion of the entire tumor field in review. This reflects the subsampling of disease heterogeneity contained within tissue microarrays, which is more concordant with the foci-level representation observed in this study. This work is additionally concordant with prior works by Aihara et al. and Ruijter et al., which demonstrate substantial grade heterogeneity in radical proctectomy specimens that is highly correlated to tumor volume (1,22).
Our study has several limitations. Control-points for each patient varied for each pathologic slide depending on the available paired 2D structural information between MRI slices and pathology. Methodology that uses 3D structural information may improve our technique. We attempted to control for gross registration errors by detailed analysis and pathologist review. Additionally, the density-based correlation analysis was performed using an isotropic Gaussian smoothing filter to reflect differences in spatial sampling between MR and pathology. While grading of pathologic lesions was carried out on individual foci contained within each slide (N=111), only 15 patients were used in this study. The subjectivity in pathologic interpretation and segmentation limits the generalization of these methods to larger cohorts, as all individual morphologic patterns characterized in this study are not routinely identified in clinical grading. However, the results of this study do indicate that specific patterns, particularly differential cribriform architecture should be evaluated in larger patient cohorts due to their influence on patient prognosis, intra-tumoral diversity, and imaging characteristics.
In conclusion, architectural features within prostate cancer foci influence ADC characteristics of MRI, with more complex tumors having lower ADC values regulated by presence and density of specific morphologies. Further exploration of these architectural histopathology features’ correlation with in vivo imaging in larger scaled studies can enable better understanding of tumor biology.
Supplementary
Spatial diversity metrics
| [1] |
Where S Eq. [1] is the set containing N total observations (o) of R patterns observed in region.
R = number of unique classes observed in region.
| [2] |
Where pjEq. [2] represents the proportion of observations belonging to a specified class pattern (j) of all observed classes (R).
| [3] |
Shannon’s diversity index (H, Eq. [3]): probably of predicting morphology class given randomly selected observation from region.
| [4] |
Simpson’s diversity index (λ, Eq. [4]): probably that two randomly selected annotations are of the same morphological class.
| [5] |
Evenness (EH, Eq. [5]): relative abundance of morphological classes within a region.
| [6] |
Alpha diversity (α, Eq. [6]): local mean species diversity within sites.
Where N = total number of distinct classes in region, S = total number of distinct classes in population, p = proportional abundance of each class weighted by proportion of data, and q taken to be 0 in this manuscript.
| [7] |
Gamma diversity: total species diversity.
| [8] |
Beta diversity: differential diversity across local and regional sites.
Acknowledgments
Funding: This project has been funded in whole or in part with federal funds from the National Cancer Institute, National Institutes of Health, under Contract No. HHSN261200800001E. The content of this publication does not necessarily reflect the views or policies of the Department of Health and Human Services, nor does mention of trade names, commercial products, or organizations imply endorsement by the U.S. Government. This project is supported by the Prostate Cancer Foundation (Young Investigator Award).
Footnote
Conflicts of Interest: The authors have no conflicts of interest to declare.
Ethical Statement: This study is compliant with local IRB and HIPAA guidelines.
Contributions: (I) Conception and design: SA Harmon, GT Brown, T Sanford, JK McKenney, B Turkbey; (II) Administrative support: SA Harmon, B Turkbey; (III) Provision of study materials or patients: MJ Merino, PL Choyke, PA Pinto, BJ Wood, B Turkbey; (IV) Collection and assembly of data: SA Harmon, GT Brown, T Sanford, S Xu, JK McKenney, B Turkbey; (V) Data analysis and interpretation: SA Harmon, GT Brown, T Sanford, S Mehralivand, JH Shih, JK McKenney, B Turkbey; (VI) Manuscript writing: All authors; (VII) Final approval of manuscript: All authors.
References
- Aihara M, Wheeler TM, Ohori M, Scardino PT. Heterogeneity of prostate cancer in radical prostatectomy specimens. Urology 1994;43:60-6; discussion 66-7. [Crossref] [PubMed]
- Epstein JI, Egevad L, Amin MB, Delahunt B, Srigley JR, Humphrey PA. Grading Committee. The 2014 International Society of Urological Pathology (ISUP) Consensus Conference on Gleason Grading of Prostatic Carcinoma: definition of grading patterns and proposal for a new grading system. Am J Surg Pathol 2016;40:244-52. [PubMed]
- Iczkowski KA, Torkko KC, Kotnis GR, Wilson RS, Huang W, Wheeler TM, Abeyta AM, La Rosa FG, Cook S, Werahera PN, Lucia MS. Digital quantification of five high-grade prostate cancer patterns, including the cribriform pattern, and their association with adverse outcome. Am J Clin Pathol 2011;136:98-107. [Crossref] [PubMed]
- McKenney JK, Wei W, Hawley S, Auman H, Newcomb LF, Boyer HD, Fazli L, Simko J, Hurtado-Coll A, Troyer DA, Tretiakova MS, Vakar-Lopez F, Carroll PR, Cooperberg MR, Gleave ME, Lance RS, Lin DW, Nelson PS, Thompson IM, True LD, Feng Z, Brooks JD. Histologic grading of prostatic adenocarcinoma can be further optimized: analysis of the relative prognostic strength of individual architectural patterns in 1275 patients from the canary retrospective cohort. Am J Surg Pathol 2016;40:1439-56. [Crossref] [PubMed]
- Siddiqui MM, Rais-Bahrami S, Turkbey B, George AK, Rothwax J, Shakir N, Okoro C, Raskolnikov D, Parnes HL, Linehan WM, Merino MJ, Simon RM, Choyke PL, Wood BJ, Pinto PA. Comparison of MR/ultrasound fusion-guided biopsy with ultrasound-guided biopsy for the diagnosis of prostate cancer. JAMA 2015;313:390-7. [Crossref] [PubMed]
- Kasivisvanathan V, Rannikko AS, Borghi M, Panebianco V, Mynderse LA, Vaarala MH, Briganti A, Budäus L, Hellawell G, Hindley RG, Roobol MJ, Eggener S, Ghei M, Villers A, Bladou F, Villeirs GM, Virdi J, Boxler S, Robert G, Singh PB, Venderink W, Hadaschik BA, Ruffion A, Hu JC, Margolis D, Crouzet S, Klotz L, Taneja SS, Pinto P, Gill I, Allen C, Giganti F, Freeman A, Morris S, Punwani S, Williams NR, Brew-Graves C, Deeks J, Takwoingi Y, Emberton M, Moore CM. PRECISION Study Group Collaborators. MRI-targeted or standard biopsy for prostate-cancer diagnosis. N Engl J Med 2018;378:1767-77. [Crossref] [PubMed]
- Turkbey B, Shah VP, Pang Y, Bernardo M, Xu S, Kruecker J, Locklin J, Baccala AA Jr, Rastinehad AR, Merino MJ, Shih JH, Wood BJ, Pinto PA, Choyke PL. Is apparent diffusion coefficient associated with clinical risk scores for prostate cancers that are visible on 3-T MR images? Radiology 2011;258:488-95. [Crossref] [PubMed]
- Chatterjee A, Watson G, Myint E, Sved P, McEntee M, Bourne R. Changes in epithelium, stroma, and lumen space correlate more strongly with Gleason pattern and are stronger predictors of prostate ADC changes than cellularity metrics. Radiology 2015;277:751-62. [Crossref] [PubMed]
- Truong M, Feng C, Hollenberg G, Weinberg E, Messing EM, Miyamoto H, Frye TP. A comprehensive analysis of cribriform morphology on magnetic resonance imaging/ultrasound fusion biopsy correlated with radical prostatectomy specimens. J Urol 2018;199:106-13. [Crossref] [PubMed]
- Kwak JT, Sankineni S, Xu S, Turkbey B, Choyke PL, Pinto PA, Moreno V, Merino M, Wood BJ. Prostate cancer: a correlative study of multiparametric MR imaging and digital histopathology. Radiology 2017;285:147-56. [Crossref] [PubMed]
- Shah V, Pohida T, Turkbey B, Mani H, Merino M, Pinto PA, Choyke P, Bernardo M. A method for correlating in vivo prostate magnetic resonance imaging and histopathology using individualized magnetic resonance-based molds. Rev Sci Instrum 2009;80:104301. [Crossref] [PubMed]
- Linkert M, Rueden CT, Allan C, Burel JM, Moore W, Patterson A, Loranger B, Moore J, Neves C, Macdonald D, Tarkowska A, Sticco C, Hill E, Rossner M, Eliceiri KW, Swedlow JR. Metadata matters: access to image data in the real world. J Cell Biol 2010;189:777-82. [Crossref] [PubMed]
- Goldberg IG, Allan C, Burel JM, Creager D, Falconi A, Hochheiser H, Johnston J, Mellen J, Sorger PK, Swedlow JR. The open microscopy environment (OME) data model and XML file: open tools for informatics and quantitative analysis in biological imaging. Genome Biol 2005;6:R47. [Crossref] [PubMed]
- Harmon S. Pathology_DetectInkedBoundaries. GitHub. Available online: https://github.com/NIH-MIP/Pathology_DetectInkedBoundaries
- Hurrell SL, McGarry SD, Kaczmarowski A, Iczkowski KA, Jacobsohn K, Hohenwalter MD, Hall WA, See WA, Banerjee A, Charles DK, Nevalainen MT, Mackinnon AC, LaViolette PS. Optimized b-value selection for the discrimination of prostate cancer grades, including the cribriform pattern, using diffusion weighted imaging. J Med Imaging (Bellingham) 2018;5:011004. [PubMed]
- McGarry SD, Hurrell SL, Iczkowski KA, Hall W, Kaczmarowski AL, Banerjee A, Keuter T, Jacobsohn K, Bukowy JD, Nevalainen MT, Hohenwalter MD, See WA, LaViolette PS. Radio-pathomic maps of epithelium and lumen density predict the location of high-grade prostate cancer. Int J Radiat Oncol Biol Phys 2018;101:1179-87. [Crossref] [PubMed]
- Kwak JT, Sankineni S, Xu S, Turkbey B, Choyke PL, Pinto PA, Merino M, Wood BJ. Correlation of magnetic resonance imaging with digital histopathology in prostate. Int J Comput Assist Radiol Surg 2016;11:657-66. [Crossref] [PubMed]
- Gibbs P, Liney GP, Pickles MD, Zelhof B, Rodrigues G, Turnbull LW. Correlation of ADC and T2 measurements with cell density in prostate cancer at 3.0 Tesla. Invest Radiol 2009;44:572-6. [Crossref] [PubMed]
- Truong M, Hollenberg G, Weinberg E, Messing EM, Miyamoto H, Frye TP. Impact of Gleason subtype on prostate cancer detection using multiparametric magnetic resonance imaging: correlation with final histopathology. J Urol 2017;198:316-21. [Crossref] [PubMed]
- Hectors SJ, Semaan S, Song C, Lewis S, Haines GK, Tewari A, Rastinehad AR, Taouli B. Advanced diffusion-weighted imaging modeling for prostate cancer characterization: correlation with quantitative histopathologic tumor tissue composition-a hypothesis-generating study. Radiology 2018;286:918-28. [Crossref] [PubMed]
- Langer DL, van der Kwast TH, Evans AJ, Sun L, Yaffe MJ, Trachtenberg J, Haider MA. Intermixed normal tissue within prostate cancer: effect on MR imaging measurements of apparent diffusion coefficient and T2--sparse versus dense cancers. Radiology 2008;249:900-8. [Crossref] [PubMed]
- Ruijter ET, van de Kaa CA, Schalken JA, Debruyne FM, Ruiter DJ. Histological grade heterogeneity in multifocal prostate cancer. Biological and clinical implications. J Pathol 1996;180:295-9. [Crossref] [PubMed]