Implant placement accuracy in total knee arthroplasty: validation of a CT-based measurement technique
Introduction
The primary goal of computer-assisted surgical systems such as robotic systems and patient specific instruments for total knee arthroplasty (TKA), is to accurately place an implant according to a preoperative plan. The accuracy of implant placement in most clinical studies is usually evaluated by determining whether neutral limb alignment has been achieved on postoperative coronal long-leg radiographs (1-4). However, this technique has limitations (5-8) which include low repeatability and reproducibility, the assessment of implant placement is limited to the coronal plane, and the applicability is limited to surgical techniques where neutral limb alignment is the goal.
To overcome the limitations of long-leg radiographs, a different measurement technique based on preoperative and postoperative computed tomography (CT)/magnetic resonance imaging (MRI) scans has been adopted in some studies (9-12) to determine implant placement accuracy in all six degrees-of-freedom (DoF) and for any planned limb alignment (i.e., not only mechanical alignment). This measurement technique requires, in addition to a preoperative CT or MRI scan needed for the preoperative plan, a postoperative CT or MRI scan to generate 3D models of the bones and implants after surgery. Then, a 3D-to-3D surface registration between the uncut bone regions of the preoperative and postoperative 3D bone models is required to establish a common reference plane between the preoperative and postoperative CT/MRI scans (13). Similarly, once the 3D bone models are registered to each other, a 3D-to-3D surface registration between the planned and postoperative 3D implant models is required to compute the 6-DoF differences between planned and postoperative implant placement. However, both 3D-to-3D surface registration of the 3D bone models and implants are susceptible to errors if the morphology of the preoperative and postoperative 3D models do not match closely. The difference in morphologies between preoperative and postoperative 3D bone and implant models can originate from inaccuracies in image segmentation, from image artifacts due to the presence of metal in the postoperative CT or MRI scan, and from the process of identifying common anatomical regions between the preoperative and postoperative 3D model.
Any error in the 3D-to-3D surface registration between the 3D bone models and between the 3D implant models may affect the accuracy of the 6-DoF implant placement error determined with such a methodology. Thus, it is important to validate this measurement method to understand the contribution of the measurement technique alone to the implant placement errors that are identified. To the best of the author’s knowledge, only one study validated a similar method based on preoperative and postoperative CT scans (14). However, this study did not specify which anatomical regions were used for the 3D-to-3D surface registration of the bone and only used sawbones for validation. Given the lack of surrounding soft tissues, sawbones can be segmented much more accurately than human bones on a CT/MRI image, which may have resulted in an underestimation of the errors of the measurement technique.
Hence, the purpose of this paper is to validate a CT-based measurement technique for the evaluation of the 6-DoF differences between postoperative and planned implant placement in patients that undergo a TKA requiring a preoperative plan. Specifically this study will determine (I) the anatomical regions that result in the lowest 6-DoF errors for 3D-to-3D surface registration of preoperative and postoperative bone models, (II) the 6-DoF errors for 3D-to-3D surface registration of the planned and postoperative implant models, and (III) the 6-DoF errors of the complete measurement technique for component placement error in TKA.
Methods
Eighteen unpaired fresh-frozen human cadaveric lower limb specimens (average age: 74 years, age range, 51–94 years, 8 females and 10 males) with full femur and tibia were included in the study. Each limb was free of radiographic signs of degenerative joint disease at the knee and the hip. Fiducial markers were fabricated using a 3D printer (Object Connex 260V, Stratasys, USA) as semi-hollow spheres of 10 mm in radius. The fiducial markers were designed in two parts: a cap and a body. The cap and body were assembled with Epoxy applied to the flat rim around the body (Figure 1A). The assembled cap and body formed a hollow sphere which could be seen in CT images with high contrast (Figure 1B). Using nylon threaded studs and methyl methacrylate nine to ten fiducial markers were implanted along the shaft of the femur and tibia to not damage the surfaces of interest (Figure 1C). The design, number, and location of the fiducial markers used in this study was previously validated in a study by Hsieh et al. where it was found that the 6 DoF registration error between two 3D fiducial models across 1,000 simulations was within 0.0±0.1 mm/deg using different numbers (7, 8, and 9) of fiducial markers (15).
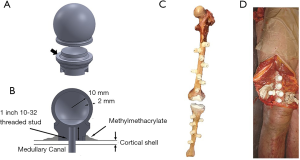
After implantation of the fiducial markers, the incision was closed (Figure 1D) and each specimen was CT scanned using a clinically adopted imaging protocol with a slice thickness of 0.625 mm, pixels size of 0.37 mm × 0.37 mm, matrix size 512×512, and 120 kV. The CT images were segmented to create contours of the whole femur and of the fiducial markers with a commercially-available segmentation software (Mimics®, Materialise - Belgium) by using thresholding and manual editing. The femur and tibia were segmented with an initial standard threshold range of 320–2,535 Hounsfield units, then local thresholding was applied in different regions of the knee and ranges varied within specimen and location. The fiducial markers were segmented with a threshold range of 0–300 Hounsfield units. Three-dimensional models of the preoperative femur and fiducial markers were generated using a variation of the standard marching cubes algorithm as implemented in Mimics®.
Errors in the 3D-to-3D bone models registration
Twelve specimens were used to validate the 3D-to-3D bone model registration. After the preoperative CT scans were performed, the specimens were subjected to a second CT scan as it would occur after a TKA procedure when using this measurement technique, hereafter referred as “postoperative CT scan”. For each specimen, the preoperative and postoperative CT scans were segmented to generate the 3D model of the femur and of the fiducial markers.
The 3D-to-3D surface registration of the preoperative and postoperative 3D femur models was performed using the iterative closest point algorithm (ICP), which is a common shape-matching algorithm widely available. For each specimen, the ICP registration was performed four times, each one using a different femoral region segmented from CT to evaluate which one would result in a more accurate surface registration (Figure 2). This resulted in four transformation matrices (TICP) from the different femoral regions, being (I) the full 3D femur model (FF), (II) the 3D femur model excluding the distal femur (FED), (III) the 3D femur model excluding both the proximal and distal femur (FEPD), (IV) the 3D femur model excluding the femoral shaft and the distal femur (FESD). The four femoral regions were chosen to account for different CT scan protocols where only a limited region of the femur may be included in the CT scan reconstruction. Also, it needs to be noted that the 3D model of the distal femur cannot be used for the 3D-to-3D femur registration as the surface has been replaced with the femoral component in the postoperative 3D femur model, therefore the scenario including the FF for registration was used as the ideal case, but will not be usable for TKA applications.
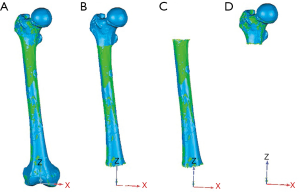
A gold standard registration was obtained by registering the preoperative and postoperative 3D models of fiducial markers using a closed-form algorithm (16) resulting in a gold standard transformation matrix (TGS). For each specimen, the placement error in the 3D-to-3D surface registration was quantified for each femoral region as follows:
| [1] |
| [2] |
| [3] |
| [4] |
from which the 6-DoF errors were expressed in standard anatomical parameters (Figure 3). For each DoF, the bias in the registration was computed as the average of the placement errors across all the 12 femurs, precision was computed as the standard deviation of the placement errors across all the 12 femurs, and the overall accuracy was computed as the root mean square (RMS) of the placement errors across all 12 femurs.
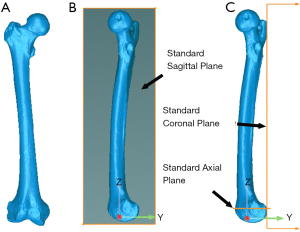
Errors in the 3D-to-3D implant model registration
Six specimens were used to validate the 3D-to-3D implant model registration errors. The specimens were prepared for TKA using an active robotic system (TSolution One, Think Surgical, Inc.) and the femoral and tibial implants were subsequently implanted into the bone using bone cement. The six specimens were then dissected from soft tissues to facilitate laser scanning (Figure 1C). Three-dimensional models of the implants and fiducial markers were generated using a high-accuracy laser scanner system (Metrascan 3D, Creaform, Canada) (17). Next, the specimens were CT-scanned and 3D models of the implants and fiducial markers were generated after segmenting the CT images. To reduce metal artifacts, the CT images were filtered with a median filter and initially segmented with a threshold between 2,500–3,100 Hounsfield units and finalized manually.
The accuracy in the 3D-to-3D surface registration between the postoperative 3D implant model segmented from CT and the planned 3D implant model (i.e., nominal implant in CAD—Computer Aided Design format) was computed by comparing the resulting transformation matrix (TICP Implant)) to the gold standard transformation matrix obtained after registration of the same surfaces using fiducial markers (TGS implant) as outlined in Figures 4,5. Specifically, the 3D-to-3D registration error was computed as
| [4] |
from which the 6-DoF errors were expressed in the same standard anatomical parameters as for the bone registration error. For each DoF, the bias, precision, and overall accuracy were computed as described for the 3D-to-3D bone registration error.
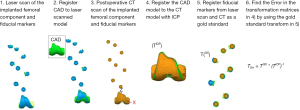
Overall error
The total 3D-to-3D registration errors (i.e., bias, precision, and accuracy) were computed for each specimen as the sum of each 3D-to-3D registration error for the bone and implant. Since this study did not compute a 3D-to-3D bone registration error for the tibia, the total error for the tibia including tibial implant and tibia 3D-to-3D registration errors were computed using the femur 3D-to-3D bone registration errors. It was assumed that the 3D-to-3D bone registration errors for the tibia are the same as the 3D-to-3D bone registration errors for the femur which may be justified by the high amount of points used in the ICP.
Statistical analysis
The analysis plan was designed to compare the bone placement error when using four different anatomical regions for the 3D-to-3D bone registration. The study was conducted with three observers and five randomly selected specimens from an available twelve. A Bayesian hierarchical model for each method was fit separately. Each model contained as a fixed effect the DOF (medial-lateral, anterior-posterior, proximal-distal, flexion-extension, varus-valgus, internal-external), and the observer, specimen, and observer by specimen interaction included as random effects. The metric used to compare among bone registration methods was the Bayesian posterior distribution of observing a value within the range of “clinical insignificance” (within ±0.2 mm/° for at least 1, at least 2, 3, 4, 5, and 6 DOF). Non-informative gaussian prior distributions N (0, 2.5) were assumed for parameters associated with the above effects and modeled through rstanarm (18). The results were used to determine the likelihood that a specimen will have ≥k (k=1 to 6) DOF within the desired range.
Results
The accuracy of the 3D-to-3D bone registration varied depending on the regions of the femur used for the analysis (Table 1). When the FF (i.e., ideal case) was used to register the preoperative and the postoperative 3D model (Figure 2A), the average bias, precision, and accuracy were within 0.2 mm for translations and 0.1° for rotation (Table 1). When only the FESD was used for registration (Figure 2D), the accuracy was particularly low for the medial-lateral and anterior-posterior translations, being on average 1.7 and 1.1 mm respectively (Table 1). However, when the FED was used for registration (Figure 2B), the accuracy was within 0.2 mm for translations and 0.2° for rotations (Table 1). When the FEPD was used for registration (Figure 2C), the accuracy was particularly low for the medial-lateral translation, being 2.3 mm (Table 1). The Bayesian posterior probabilities of observing clinical significance in all 6-DoF errors were relatively low when using the FF (0.827) or the FED (0.679), but very high when using only the FESD (0.000). The accuracy of the 3D-to-3D femoral and tibial implant registration was within 0.7 mm for translations and 0.6°–0.4° for rotations, respectively (Tables 2,3).
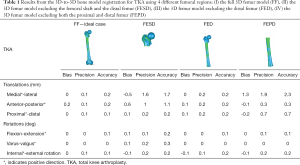
Full table
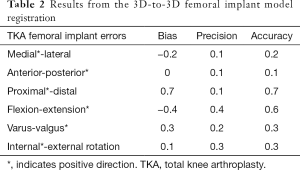
Full table
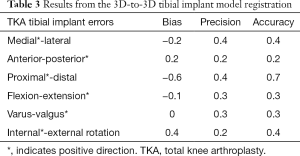
Full table
For the femur, the total error including 3D-to-3D bone and implant registration errors was the lowest when the FF or FED were used with an accuracy of 0.8–0.9 mm and 0.6°, respectively (Table 4). Similarly, for the tibia, the total error was the lowest when the 3D tibia model included the complete surface or, at a minimum included the tibial shaft and the distal tibia, with an accuracy of 0.8–0.9 mm and 0.6°, respectively (Table 5).
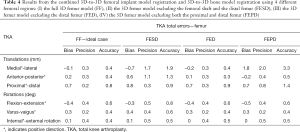
Full table
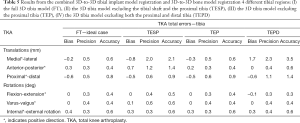
Full table
Discussion
The present study validates a CT-based measurement technique to determine implant placement accuracy for TKA. The key finding of this study is that implant placement accuracy can be determined reproducibly with this CT-based measurement technique with errors that are within 0.9 mm and 0.6°. Thus, this technique can be used for any orthopedic applications aiming at determining implant placement accuracies that are higher or as high as these values. The second finding of this study is that using the FEPD or the FESD to perform a 3D-to-3D bone registration is not adequate to determine implant placement errors that are within 1 mm and 1° and larger surface area that are more feature-rich are preferable. The third finding of this study is that the main source of error in determining implant placement comes from the 3D-to-3D registration of 3D implants. Inaccurate registration of 3D implants is mainly due to an inaccurate implant segmentation caused by metal artifacts (i.e., beam hardening) in the postoperative CT scan. Specifically, these artifacts resulted in a larger implant (Figure 6) which created a bias mostly in the proximal-distal direction for which the femoral implant tends to be on average 0.7 mm more proximal and the tibial implant 0.6 mm more distal.
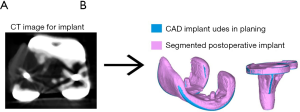
Jonkergouw et al. (14) validated a similar CT-based 3D measurement technique and found that the average absolute error in 3D-to-3D surface registration was within 0.3 mm and 0.1° for the femur registration and within 1–0.5 mm and 0.3–0.4° for the registration of the femoral and tibial component, respectively. In agreement to our study, Jonkergouw et al. found that the main source of error of this measurement technique is introduced by the 3D-to-3D implant registration error with the error in the proximal-distal direction being the highest.
There are some limitations to the present study. One limitation is that the total registration error for the tibia was computed under the assumption that the errors in the 3D-to-3D registration of the tibia 3D bone models are comparable to the 3D-to-3D registration of the femur 3D bone models. Although it is possible that the 3D-to-3D registration of the tibia 3D bone models could have higher errors than the registration of the femur since there are less anatomical features in the tibia than the femur, the assumption of comparable errors seems to be reasonable based on the high amount of points used in the ICP. Another limitation is that a further reduction of metal artifacts in the postoperative CT scan could have contributed to a more accurate implant segmentation leading to a more accurate 3D implant registration and an overall reduction of the error with this measurement technique (19).
In conclusion, this CT-based measurement technique can be used in patients to evaluate implant placement accuracy when measurement errors of up to 0.9 mm in translation and 0.6° in rotation are acceptable. Based on these results, it is recommended to use the entire uncut surface of the femur and tibia after TKA to minimize the error in the 3D-to-3D bone registration.
Acknowledgments
The authors would like to thank individuals who donate their bodies for the advancement of education and research.
Footnote
Conflicts of Interest: David Maislin has received personal fees from MCRA. MCRA has received fees from Think Surgical to support statistical analyses. The other authors have no conflicts of interest to declare.
References
- Choong PF, Dowsey MM, Stoney JD. Does Accurate Anatomical Alignment Result in Better Function and Quality of Life? Comparing Conventional and Computer-Assisted Total Knee Arthroplasty. J Arthroplasty 2009;24:560-9. [Crossref] [PubMed]
- Cho KJ, Seon JK, Jang WY, Park CG, Song EK. Robotic versus conventional primary total knee arthroplasty: clinical and radiological long-term results with a minimum follow-up of ten years. Int Orthop 2019;43:1345-54. [Crossref] [PubMed]
- Chin PL, Yang KY, Yeo SJ, Lo NN. Randomized control trial comparing radiographic total knee arthroplasty implant placement using computer navigation versus conventional technique. J Arthroplasty 2005;20:618-26. [Crossref] [PubMed]
- Daniilidis K, Tibesku CO. Frontal plane alignment after total knee arthroplasty using patient-specific instruments. Int Orthop 2013;37:45-50. [Crossref] [PubMed]
- Brouwer RW, Jakma TS, Brouwer KH, Verhaar JA. Pitfalls in determining knee alignment: a radiographic cadaver study. J Knee Surg 2007;20:210-5. [Crossref] [PubMed]
- Yaffe MA, Koo SS, Stulberg SD. Radiographic and navigation measurements of TKA limb alignment do not correlate. Clin Orthop Relat Res 2008;466:2736-44. [Crossref] [PubMed]
- Holme TJ, Henckel J, Cobb J, Hart AJ. Quantification of the difference between 3D CT and plain radiograph for measurement of the position of medial unicompartmental knee replacements. Knee 2011;18:300-5. [Crossref] [PubMed]
- Hirschmann MT, Konala P, Amsler F, Iranpour F, Friederich NF, Cobb JP. The position and orientation of total knee replacement components: a comparison of conventional radiographs, transverse 2D-CT slices and 3D-CT reconstruction. J Bone Joint Surg Br 2011;93:629-33. [Crossref] [PubMed]
- Kerens B, Leenders AM, Schotanus MGM, Boonen B, Tuinebreijer WE, Emans PJ, Jong B, Kort NP. Patient-specific instrumentation in Oxford unicompartmental knee arthroplasty is reliable and accurate except for the tibial rotation. Knee Surg Sports Traumatol Arthrosc 2018;26:1823-30. [Crossref] [PubMed]
- De Vloo R, Pellikaan P, Dhollander A, Vander Sloten J. Three-dimensional analysis of accuracy of component positioning in total knee arthroplasty with patient specific and conventional instruments: A randomized controlled trial. Knee 2017;24:1469-77. [Crossref] [PubMed]
- Citak M, Suero EM, Citak M, Dunbar NJ, Branch SH, Conditt MA, Banks SA, Pearle AD. Unicompartmental knee arthroplasty: Is robotic technology more accurate than conventional technique? Knee 2013;20:268-71. [Crossref] [PubMed]
- Dunbar NJ, Roche MW, Park BH, Branch SH, Conditt MA, Banks SA. Accuracy of Dynamic Tactile-Guided Unicompartmental Knee Arthroplasty. J Arthroplasty 2012;27:803-8.e1. [Crossref] [PubMed]
- Navruzov T, Rivière C, Van Der Straeten C, Harris S, Cobb J, Auvinet E, Aframian A, Iranpour F. Registration of pre- and post-operative CT data using ICP for patients undergoing total knee arthroplasty (TKA). Orthopaedic Proceedings. 2018. Available online: https://online.boneandjoint.org.uk/doi/abs/10.1302/1358-992X.99BSUPP_1.EORS2016-100
- Jonkergouw F, Allé F, Chellaoui K, Vander Sloten J, Vangeneugden D. Three-dimensional measurement technique to assess implant position and orientation after total knee arthroplasty. Med Eng Phys 2016;38:1513-7. [Crossref] [PubMed]
- Hsieh CM, Howell S, Hull M. What are the six degree-of-freedom errors of a robotically-machined femoral cavity in total hip arthroplasty and are they clinically important? An in-vitro study. Med Eng Phys 2017;48:120-30. [Crossref] [PubMed]
- Arun KS, Huang TS, Blostein SD. Least-squares fitting of two 3-D point sets. IEEE Trans Pattern Anal Mach Intell 1987;9:698-700. [Crossref] [PubMed]
- Campanelli V, Howell SM, Hull ML. Accuracy evaluation of a lower-cost and four higher-cost laser scanners. J Biomech 2016;49:127-31. [Crossref] [PubMed]
- Goodrich B, Gabry J, Ali I, Brilleman S. rstanarm: Bayesian applied regression modeling via Stan. R package version 2.17.4. 2018. Available online: http://mc-stan.org/
- Morsbach F, Bickelhaupt S, Wanner GA, Krauss A, Schmidt B, Alkadhi H. Reduction of Metal Artifacts from Hip Prostheses on CT Images of the Pelvis: Value of Iterative Reconstructions. Radiology 2013;268:237-44. [Crossref] [PubMed]