Characterizing optical coherence tomography speckle fluctuation spectra of mammary organoids during suppression of intracellular motility
Introduction
There is increasing need for label-free, longitudinal and quantitative methods to assess three-dimensional (3D) tissue models of carcinogenesis (e.g., under toxicant exposure), progression, and treatment (e.g., drug screening and development). Compared to conventional two-dimensional (2D) cell cultures that are often inadequate to model complex tissues structurally and functionally, 3D tissue models can establish cell-cell and cell-extracellular matrix (ECM) interactions that more closely recapitulate in vivo tissue architecture and exhibit more physiologically relevant tissue properties and drug responses (1-3). The study of cell motility, an important underlying mechanism of cell function, is critical for understanding the migration/invasion and metastasis of breast cancer and developing associated treatments (4,5). However, current methods are either limited to 2D models or require cell fixation and staining, precluding efficient longitudinal analysis (6).
Emerging technologies based on coherence imaging such as optical coherence tomography (OCT), known as a method of “optical histology”, address these limitations by providing depth-resolved imaging using near-infrared light scattering, analogous to ultrasound imaging (7). The micrometer-scale resolution and millimeter-scale depth penetration of OCT makes it particularly well suited for quantifying morphology in 3D organoid models where existing assays are cumbersome (8,9). The non-invasive nature of OCT enables longitudinal measurements of 3D tissue cultures. We have previously employed OCT to monitor growth of mammary epithelial organoids (including size, lumen size, and asphericity) over weeks, and quantified morphological changes under culture conditions that modified stromal-epithelial interactions (9).
In addition to enabling morphological measurements, the high frame rate of OCT has been exploited to quantify subcellular dynamics in 3D tissue cultures (10-13). OCT image speckles are sensitive to “intracellular motility”, i.e., high-speed, in-place motions of subcellular light scattering components occurring over short (seconds to minutes) time scales, such as organelle transport and membrane undulations. Intracellular motility is a useful metric that contains abundant information about cell state; it can also be employed as an OCT contrast method to distinguish live cells from nonliving cells and background material. Differences in intracellular motility of live and fixed tissues were first detected by holographic optical coherence imaging (OCI) in 2004 (10). Since then, OCT speckle fluctuation statistics specific to intracellular motility have been used to differentiate live mammary epithelial cell (MEC) organoids from a surrounding ECM that contained highly scattering nanoparticles undergoing diffusive motion (11). Recently, full-field OCT of fresh ex vivo tissues (brain, liver) was shown to provide rich subcellular metabolic contrast in the speckle statistics (14). In the present study, to quantify the intracellular motility signal of MEC organoids, we employ two previously reported metrics that are independent of light attenuation and position within OCT images: the inverse-power-law exponent of the speckle fluctuation spectrum (α), and an autocorrelation-based motility amplitude (M) (12). Importantly, these motility-based metrics exhibited significant differences in 3D co-cultures as a function of modifications to stromal-epithelial cell interactions (12). In another effort, α and M were employed in a high-throughput manner to assess effects of toxicants on 3D MEC organoid models, with findings validated by a standard MTT assay (13).
The underlying biological processes that give rise to coherence imaging-based intracellular motility signals are a topic of ongoing study (15-21). Time- and dose-dependent responses of rat osteogenic sarcoma spheroids to different cytoskeleton-targeting drugs, in terms of the speckle fluctuation amplitude, were first characterized by digital holographic OCI in 2007 (15). Since then, speckle fluctuation spectroscopy (SFS) of the same tumor spheroids has been performed under a number of different environmental and pharmacological perturbations (16-19). Spectral responses to different perturbations were measured from 0.005 to 5 Hz, where the fluctuations approximately fell into three frequency bands: low-frequency (0.005 to 0.05 Hz), mid-frequency (0.05 to 0.5 Hz), and high-frequency (0.5 to 5 Hz), corresponding to intracellular motions of membranes, mitochondria and organelles, and vesicles and the cytoplasm, respectively (17). These signatures were used to construct multi-dimensional feature vectors for phenotypic profiling of drug effects in 3D cultures, applicable more broadly for compound screening (18,19). However, we are still in the early stages of understanding the speckle fluctuation spectra of coherence imaging-based intracellular motility signals, as they are highly dependent on cell lines and culture models (12,13,20,21).
In this study, we focus on elucidating some of the processes that give rise to the intracellular motility signals obtained from 3D MEC organoids via OCT-SFS. Specifically, suppression of two highly dynamic cellular components, microtubules and myosin II, was induced in 3D MEC organoid models by two inhibitors: a microtubule stabilizer (Paclitaxel, Taxol), and a myosin II inhibitor (Blebbistatin). Microtubules are highly dynamic cytoskeletal polymers that constantly grow and shrink at a rapid rate. They serve as molecular tracks for intracellular transport during interphase, and are responsible for chromosome separation during mitosis with a 20–100 fold increase in dynamics (22,23). Taxol is a well-studied chemotherapeutic agent that inhibits mitotic spindle function by stabilizing microtubule polymers and preventing their disassembly (24,25). Myosin II contributes to intracellular motility by generating mechanical forces required for processes associated with cell division and migration (e.g., cellular reshaping and adhesion) (26,27). Blebbistatin is a small molecule compound that specifically inhibits myosin II (28). Here, two cell lines representing different breast cancer subtypes were investigated for possible characteristic differences: a basal-like pre-malignant breast cell line, MCF10DCIS.com, and an invasive luminal adenocarcinoma cell line, MCF7. Using the α and M OCT-SFS metrics, we characterized the responses of each cell line to both disruption mechanisms, and the effects of generalized cell stress and cytotoxicity in 3D mammary cultures longitudinally. We expected to observe changes in speckle fluctuation spectra for both cell lines in response to both inhibitors, while possible characteristic differences might be expected between the two cell lines. This paper provides a foundation for future OCT-SFS studies of longitudinal responses of the mammary gland in toxicology and drug research.
Methods
Cell lines and reagents
Premalignant, basal-like MCF10DCIS.com cells were purchased from Karmanos Cancer Institute (Detroit, MI, USA) and were maintained in Dulbecco’s modified Eagle’s medium (DMEM)/F12 nutrient mixture supplemented with 5% horse serum, 10 µg/mL insulin (GIBCO, Life Technologies, Carlsbad, CA, USA), 20 ng/mL Epidermal Growth Factor (Invitrogen, Life Technologies), 0.5 µg/mL hydrocortisone (Sigma-Aldrich, St. Louis, MO, USA), 0.1 µg/mL cholera toxin (EMD Millipore, Darmstadt, Germany) and 1% penicillin-streptomycin. MCF7 luminal invasive adenocarcinoma cells were purchased from the American Type Culture Collection (Manassas, VA) and were maintained in RPMI 1640 medium (GIBCO) supplemented with 10% fetal bovine serum (Gemini Bio-Products, West Sacramento, CA) and 1% penicillin-streptomycin. All cultures were maintained in a humidified incubator at 37 °C with 5% CO2. Both inhibitors (Taxol and Blebbistatin) used for organoid culture exposures in this study were purchased from Sigma-Aldrich.
3D culture and inhibitor exposures
The 3D cultures used in this study were constructed as previously described (9,12,13). Briefly, after cells in 2D cultures reached 70% confluency, cells were seeded at a constant density of 30,000 cells/cm3 into 275 µL of a biologically-derived ECM consisting of 1:1 (v/v) mixture of collagen I: Matrigel (BD Biosciences, Franklin Lakes, NJ) (29), with collagen I at a final concentration of 1 mg/mL. The cell-laden gels were then plated into individual wells of 48-well cell culture plates (diameter: 10 mm) that were pre-coated with 85 µL of the collagen I- Matrigel mixture. After gelation, 250 µL of the relevant culture medium was dispensed to the surface of each culture, and was refreshed every 2–3 days. Prior to inhibitor exposure, MCF10DCIS.com and MCF7 cells were cultured in 3D for 10 and 14 days after seeding, respectively, to enable organoid formation. Then the cultures were exposed daily for a total of 6 days to Taxol (0, 10, or 20 µM) or Blebbistatin (0, 25, or 50 µM). OCT-SFS of inhibitor-exposed cultures was first performed prior to exposure (“0 hour”), and then at 1 hour, 24 hours, 48 hours, and 6 days after the initial exposure. Most of the excess liquid culture media lying on top of the culture matrix was removed before imaging to reduce dispersion-induced image degradation, with a small amount of excess media retained to protect the culture matrix from dehydration. Fresh media with corresponding inhibitor concentration was applied immediately after imaging. Three independent cultures per condition (inhibitor type, inhibitor concentration, and cell line) were prepared and tracked longitudinally with OCT-SFS. An average of 6 organoids per culture was imaged by OCT-SFS, resulting in a total of n=12–24 organoids analyzed per condition.
OCT-SFS methods and analysis metrics
Imaging of the 3D cultures was performed using a custom built, spectral-domain optical coherence tomography (SD-OCT) system as shown in Figure 1A, which has been described in detail previously (30). Briefly, the system consists of three parts: the light source, a Michelson interferometer and a custom spectrometer. The light source consisted of a Ti:Sapphire laser with a central wavelength of 800 nm and bandwidth of 120 nm. Light from the source was linearly polarized (horizontal to the surface of the optical table; H polarized) and directed into the Michelson interferometer. The H polarized light was split into reference and sample arms, where the sample arm light (~6 mW) was focused by a 30 mm focal length lens upon the sample. The co-polarized backscattered light (H) interfered with the reference at the beam splitter. The interfered light (HH) was then directed to the custom spectrometer where spectral interferograms were collected into the first 2,048 pixels of a 4,096-pixel Dalsa Piranha line scan CCD camera, operated at an A-line rate of 2 kHz for this study. The resolution of the OCT system was ~10 µm × 3.0 µm (in aqueous medium) in x × z, and the signal-to-noise ratio (SNR) was ~108 dB. B-mode (cross-sectional) image frames were collected into 1,000×1,024 pixels over 1.5 mm × 1.5 mm (in aqueous medium) in x × z, respectively. 300 frames per time series were collected at a frame rate of 0.876±0.004 Hz in order to capture cellular dynamics (12,13), and then divided into three groups of n=100 consecutive images for independent analysis with cross-validation.
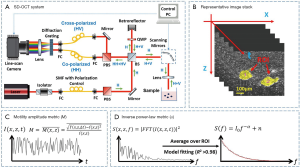
All image analysis was performed as described in our previous studies (9,12,13). Briefly, depth-resolved OCT images were computed from raw spectral images after reference subtraction and digital dispersion compensation (31), where the intensity at each image pixel, I(x, z), was computed from the absolute value of the complex analytic signal obtained from Fourier transform of the spectral domain OCT data. A time series of OCT images of MEC organoids, as shown in Figure 1B, were used to generate movies for the SFS analysis. Each organoid, containing hundreds to thousands of cells, was identified and segmented as one region of interest (ROI) by custom semi-automated MATLAB scripts; two representative ROIs are color-coded in yellow in Figure 1B. The temporal fluctuation of speckle intensity at each pixel, I(x, z, t), was extracted from the image stack, as shown in Figure 1C, which was attributed to the motions of the intracellular particles that backscatter OCT light. These speckle fluctuations were characterized by two parameters: the so-called “motility amplitude”, M, that characterizes the modulation amplitude of the speckle fluctuations in time, and the power-law exponent of the decay of the power spectral density in frequency, α. M is an autocorrelation-based modified standard deviation that is normalized by average pixel intensity (12), and provides two complementary benefits for data analysis: the autocorrelation at each image pixel obtained at a given sampling time Δt, Γ(x, z, Δt), naturally omits shot noise that decorrelates instantaneously, while normalization by average pixel intensity eliminates the depth-dependent SNR roll-off, making M intensity- and depth-invariant. M at each pixel was then spatially averaged over each ROI to represent the M of an organoid. To characterize the frequency (f) dependence of the motility signals, the power spectral density S(x, z, f) was computed by a discrete Fourier transform of the time signal I(x, z, t) at each pixel. The spectra were then spatially averaged over each ROI, then fitted to an inverse power-law model with the power exponent α (12), as illustrated in Figure 1D. A goodness-of-fit test was performed for model fitting of each ROI spectrum, where only data exhibiting R2>0.98 compared to the model were included in the subsequent analysis. Figure 2A shows a cross-sectional OCT image of a representative data set. The motility metrics derived from this data set are visualized in Figure 2B,C,D, with more details of the visualization method described in (12). Together, the two metrics α and M characterized the intracellular motility of an organoid (one ROI), with ~12–24 values of α and M each computed over n=3 cultures at each culture condition (inhibitor type, inhibitor concentration, and cell line). To assess the time-evolution of intracellular motility, multiple comparison t-tests with Bonferroni correction were then performed to compare α and M for each culture condition against the pre-exposure α and M for that same condition. P values were calculated to indicate the statistically significant differences, where the critical P value was set to 0.01 for all comparisons.
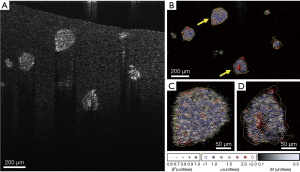
As previously discovered (12,13), values of α and M are cell type-dependent, which is attributed to differences in morphology, metabolism, invasiveness, and ECM interactions. Therefore, in order to define a typical dynamic range for each metric, cell line-specific baselines were established based on the α and M values of live and fixed cells measured previously (13). A linear mapping was used from the raw α and M values to normalized values where 0% and 100% represent the fixed and live values, respectively. Note that raw α exhibits an increase under cell fixation, indicating that the speckle fluctuation spectra more rapidly decay in frequency (suppressing high frequency motions), while the linear scaling releases the users from constantly recalling this fact when assessing longitudinal data. It should be noted that the fixed values represent “death by fixation”, which is mechanistically different from inhibitor-induced cell death evaluated in this paper. In addition, the live values may be subject to biologic variations over time such as the phase of cell growth, which may cause untreated cells to exhibit initial values that are above or below 100%. However, this does not affect the observations and conclusions from longitudinal data, as effects of inhibitors are only reflected by the relative changes between the pre-exposure and post-exposure data.
Results and discussion
Effect of Taxol on MEC organoids
The time- and concentration-dependent response of MCF10DCIS.com organoids to Taxol exposure were measured longitudinally by OCT-SFS, as shown in Figure 3. Note that the scaled “live” values corresponding to 100% were defined from the control (untreated) organoids from a previous study (13); thus, the live values (>100%) observed in Figure 3 may arise from biologic variability in cell growth or incubation time. Here the relative changes between the pre-exposure and the post-exposure data to track the effects of the inhibitors applied. In MCF10DCIS.com organoids, both α and M decreased (though non-monotonically) over exposure time for both 10 and 20 µM concentrations of Taxol, with statistically significant differences (P<0.001) between pre-exposure and exposed cultures at 24 hours and later (relative to baseline) for both α and M.
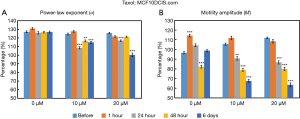
Figure 4 displays the time- and concentration-dependent responses of MCF7 organoids to Taxol exposure as measured by OCT-SFS. In this experiment, α decreased over exposure time for both 10 and 20 µM concentrations of Taxol, where statistically significant reductions in α (P<0.001) between pre-exposure and exposed cultures were observed at 6 days for organoids treated with 10 µM of Taxol, and beginning at 48 hours of exposure for those treated with 20 µM. No significant reductions in the M metric were observed for any exposure time or inhibitor concentration in the MCF7 organoids.
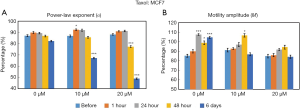
These OCT-based findings are attributed to Taxol-induced cell death, wherein microtubule stabilization suppresses intracellular motility. Both α and M are sensitive to Taxol in MCF10DCIS.com organoids, while only α is sensitive to Taxol in MCF7 organoids under the tested exposure times and concentrations. It is necessary to point out that the speckle fluctuation spectra of both cell line controls (0 µM Taxol) exhibit baseline variability over the 6-day time course; this represents several population doubling times (6 days) for both cell lines. It is interesting to note that the timing of observed reductions in α correlate with the division rates of each cell line (i.e., MCF10DCIS.com cells divide faster than MCF7 cells in both 2D and 3D cultures), suggesting that the biological function(s) captured by the α metric may be related to microtubule-related and/or mitotic processes. In fact, the time scales of the significant responses in α (i.e., ≥24 hours for MCF10DCIS.com and ≥48 hours for MCF7 cells, respectively) in this study closely match the 2D doubling times of each cell line (i.e., 18.5 hours for MCF10DCIS.com cells and 36.7 hours for MCF7 cells). Future studies with a larger number of cell lines with varying doubling times would be useful for verifying the connection between α and doubling time.
The OCT-SFS data suggest that α and M are differentially responsive to Taxol treatment in a cell-type specific manner; M in Taxol-treated MCF7 cells was the only metric that failed to significantly change in this context. Interestingly, the same patterns of α and M responsivity were observed in our previous study of MCF10DCIS.com and MCF7 cells exposed to another chemotherapy drug, Doxorubicin (13), which inhibits cell division by causing DNA double-strand breaks (32). In our previous study (13), α and M of MCF10DCIS.com organoids significantly changed in response to Doxorubicin treatment; however, only α was sensitive to Doxorubicin treatment in MCF7 organoids under the same range of exposure times and in response to concentrations of 1 and 10 µM. This lack of M responsivity unique to MCF7 organoids may be caused by the relatively slow growth rate of this cell line; as both chemotherapy drugs inhibit cell division, higher concentrations and/or longer exposure times will be employed in future studies to investigate whether M in MCF7 organoids may respond significantly to antineoplastic drug exposure.
Effect of Blebbistatin on MEC organoids
The time- and concentration-dependent responses to Blebbistatin exposure for both MCF10DCIS.com and MCF7 organoids were measured longitudinally by OCT-SFS, as shown in Figures 5 and 6, respectively. In MCF10DCIS.com organoids, statistically significant differences in M between pre-exposure and exposed cultures were found at the longest exposure time (6 days, P<0.001) for the 25 µM concentration, and at an earlier exposure time (24 hours, P<0.01) for the 50 µM concentration. In contrast, changes in α were more modest and did not exhibit monotonic decreases over time. In MCF7 organoids, statistically significant differences in both α and M between pre-exposure and exposed cultures were found following the longest exposure time (6 days, P<0.001) for both Blebbistatin concentrations.
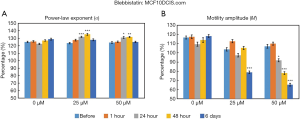
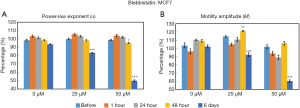
The above OCT-based findings were attributed Blebbistatin-induced myosin II inhibition and subsequent suppression of intracellular motility. Here OCT-SFS data suggested different responsivities to Blebbistatin between the two cell lines under the given exposure times and concentrations: in MCF7 cells, both α and M were significantly reduced in response to Blebbistatin, whereas similar reductions in MCF10DCIS.com cells were only apparent for the M metric. The lack of significant reductions in α in MCF10DCIS.com-treated organoids may reflect relatively low baseline levels of myosin II expression. Therefore, in future work, higher concentrations and/or longer exposure times may be required to elicit significant reductions in MCF10DCIS.com organoid α in this context.
As expected, OCT-SFS revealed time- and concentration-dependent responses of mammary epithelial organoids to compounds known to affect cell function, similarly to our previous work (13). In particular, the OCT-SFS data suggest that differences in cell line doubling times may result in different time scales of susceptibility to the motility suppression mechanisms of Taxol. Furthermore, it is interesting that α and M exhibit cell line specificity with respect to each inhibitor. This finding is consistent with observations from our previous study (13): in response to Doxorubicin exposure, α and M were strongly reduced in MCF10DCIS.com, but not MCF7, organoids, whereas in MCF7 organoids, α and M were uniquely responsive to treatment with tamoxifen and estrogen, respectively. Future studies are required to further delineate the manner in which the characteristics of mammary epithelial organoid OCT speckle fluctuation spectra respond to different mechanisms of intracellular motility suppression.
Conclusions
In this work, we characterized the response patterns of 3D mammary epithelial organoid models to inhibitors offering distinct intracellular motility suppression mechanisms, using non-invasive, longitudinal and high-throughput OCT-SFS to quantify intracellular motility. Two complementary OCT motility metrics extracted from OCT-SFS were applied in the analysis: the inverse power law exponent, α, of the speckle fluctuation spectrum, and the fractional modulation amplitude, M, of the speckle intensity. We quantified the responses of both pre-malignant basal-like and luminal adenocarcinoma breast cancer cell lines to two different suppressors of intracellular motility, Taxol and Blebbistatin. The results revealed time- and concentration-dependent responses to the inhibitors; statistically significant differences between pre-exposed and exposed cultures were attributed to either cell death caused by Taxol-induced microtubule stabilization or Blebbistatin-induced myosin II inhibition. The results also demonstrated the ability of OCT-SFS to reveal cell line-specific differences in intracellular motility in response to different mechanisms of motility suppression. Ultimately, this data may improve our understanding of how the mammary gland responds to toxicant exposures, and highlights the potential of OCT for future use in development of effective treatments for breast cancer.
Acknowledgments
Funding: National Cancer Institute at the National Institutes of Health (R21 CA172904 to AL Oldenburg); National Institute of Environmental Health Sciences at the National Institutes of Health (P30 ES010126); National Science Foundation (CBET 1351473 and CBET 1803830 to AL Oldenburg, CBET 1803785).
Footnote
Conflicts of Interest: The authors have no conflicts of interest to declare.
References
- Baker BM, Chen CS. Deconstructing the third dimension – how 3D culture microenvironments alter cellular cues. J Cell Sci 2012;125:3015-24. [Crossref] [PubMed]
- Duval K, Grover H, Han LH, Mou Y, Pegoraro AF, Fredberg J, Chen Z. Modeling Physiological Events in 2D vs. 3D Cell Culture. Physiology (Bethesda) 2017;32:266-77. [Crossref] [PubMed]
- Imamura Y, Mukohara T, Shimono Y, Funakoshi Y, Chayahara N, Toyoda M, Kiyota N, Takao S, Kono S, Nakatsura T, Minami H. Comparison of 2D- and 3D-culture models as drug-testing platforms in breast cancer. Oncology Reports 2015;33:1837-43. [Crossref] [PubMed]
- Paul CD, Mistriotis P, Konstantopoulos K. Cancer cell motility: lessons from migration in confined spaces. Nat Rev Cancer 2017;17:131-40. [Crossref] [PubMed]
- Greenshields AL, Doucette CD, Hoskin DW. Piperine inhibits the growth and motility of triple-negative breast cancer cells. Cancer Lett 2015;357:129-40. [Crossref] [PubMed]
- Kramer N, Walzla A, Ungera C, Rosnera M, Krupitzab G, Hengstschlägera M, Dolznig H. In vitro cell migration and invasion assays. Mutat Res 2013;752:10-24. [Crossref] [PubMed]
- Huang D, Swanson EA, Lin CP, Schuman JS, Stinson WG, Chang W, Hee MR, Flotte T, Gregory K, Puliafito CA, Fujimoto JG. Optical coherence tomography. Science 1991;254:1178-81. [Crossref] [PubMed]
- Yu P, Mustata M, Turek JJ, French PMW, Melloch MR, Nolte DD. Holographic optical coherence imaging of tumor spheroids. Appl Phys Lett 2003;83:575-7. [Crossref]
- Chhetri RK, Phillips ZF, Troester MA, Oldenburg AL. Longitudinal study of mammary epithelial and fibroblast co-cultures using optical coherence tomography reveals morphological hallmarks of pre-malignancy. PLoS One 2012;7:e49148. [Crossref] [PubMed]
- Yu P, Peng L, Mustata M, Turek JJ, Melloch MR, Nolte DD. Time-dependent speckle in holographic optical coherence imaging and the health of tumor tissue. Optics Letters 2004;29:68-70. [Crossref] [PubMed]
- Oldenburg AL, Chhetri RK, Cooper JM, Wu WC, Troester MA, Tracy JB. Motility-, autocorrelation-, and polarization-sensitive optical coherence tomography disciminates cells and gold nanorods within 3D tissue cultures. Optics Letters 2013;38:2923-6. [Crossref] [PubMed]
- Oldenburg AL, Yu X, Gilliss T, Alabi O, Taylor R, Troester M. Inverse power-law behavior of cellular motility reveals stromal-epithelial interactions in 3D co-culture by OCT fluctuation spectroscopy. Optica 2015;2:877-85. [Crossref] [PubMed]
- Yu X, Fuller AM, Blackmon R, Troester M, Oldenburg AL. Quantification of the Effect of Toxicants on the Intracellular Kinetic Energy and Cross-Sectional Area of Mammary Epithelial Organoids by OCT Fluctuation Spectroscopy. Toxicol Sci 2018;162:234-40. [Crossref] [PubMed]
- Apelian C, Harms F, Thouvenin O, Boccara AC. Dynamic full field optical coherence tomography: subcellular metabolic contrast revealed in tissues by interferometric signals temporal analysis. Biomed. Opt. Express 2016;7:1511-24. [Crossref] [PubMed]
- Jeong K, Turek JJ, Nolte DD. Volumetric motility-contrast imaging of tissue response to cytoskeletal anti-cancer drugs. Opt. Express 2007;15:14057-64. [Crossref] [PubMed]
- Jeong K, Turek JJ, Nolte DD. Speckle fluctuation spectroscopy of intracellular motion in living tissue using coherence-domain digital holography. J Biomed Opt 2010;15:030514. [Crossref] [PubMed]
- Nolte DD, An R, Turek J, Jeong K. Holographic tissue dynamics spectroscopy. J Biomed Opt 2011;16:087004. [Crossref] [PubMed]
- Nolte DD, An R, Turek J, Jeong K. Tissue Dynamics Spectroscopy for Three-Dimensional Tissue-Based Drug Screening. J Lab Autom 2011;16:431-42. [Crossref] [PubMed]
- Nolte DD, An R, Turek J, Jeong K. Tissue dynamics spectroscopy for phenotypic profiling of drug effects in three-dimensional culture. Biomed Opt Express 2012;3:2825-41. [Crossref] [PubMed]
- An R, Turek J, Matei DE, Nolte DD. Live tissue viability and chemosensitivity assays using digital holographic motility contrast imaging. Appl Opt 2013;52:A300-9. [Crossref] [PubMed]
- An R, Merrill D, Avramova L, Sturgis J, Tsiper M, Paul Robinson J, Nolte DD. Phenotypic Profiling of Raf Inhibitors and Mitochondrial Toxicity in 3D Tissue Using Biodynamic Imaging. Journal of Biomolecular Screening 2014;19:526-37. [Crossref] [PubMed]
- Bates D, Eastman A. Microtubule destabilising agents: far more than just antimitotic anticancer drugs. Br J Clin Pharmacol 2017;83:255-68. [Crossref] [PubMed]
- Jordan MA, Wilson L. Microtubules as a target for anticancer drugs. Nature Reviews 2004;4:253-65. [PubMed]
- Yvon AM, Wadsworth P, Jordan MA. Taxol suppresses dynamics of individual microtubules in living human tumor cells. Mol Biol Cell 1999;10:947-59. [Crossref] [PubMed]
- Liebmann JE, Cook JA, Lipschultz C, Teague D, Fisher J, Mitchell JB. Cytotoxic studies of paclitaxel (Taxol) in human tumour cell lines. Br J Cancer 1993;68:1104-9. [Crossref] [PubMed]
- Vicente-Manzanares M, Ma X, Adelstein RS, Horwitz AR. Non-muscle myosin II takes centre stage in cell adhesion and migration. Nat Rev Mol Cell Biol 2009;10:778-90. [Crossref] [PubMed]
- Lesman A, Notbohm J, Tirrell DA, Ravichandran G. Contractile forces regulate cell division in three-dimensional environments. J Cell Biol 2014;205:155-62. [Crossref] [PubMed]
- Kovács M, Tóth J, Hetényi C, Málnási-Csizmadia A, Sellers JR. Mechanism of Blebbistatin Inhibition of Myosin II. J Biol Chem 2004;279:35557-63. [Crossref] [PubMed]
- Johnson KR, Leight JL, Weaver VM. Demystifying the effects of a three-dimensional microenvironment in tissue morphogenesis. Methods Cell Biol 2007;83:547-83. [Crossref] [PubMed]
- Chhetri RK, Kozek KA, Johnston-Peck AC, Tracy JB, Oldenburg AL. Imaging three-dimensional rotational diffusion of plasmon-resonant gold nanorods using polarization-sensitive optical coherence tomography. Phys Rev E Stat Nonlin Soft Matter Phys 2011;83:040903. [Crossref] [PubMed]
- Marks DL, Oldenburg AL, Reynolds JJ, Boppart SA. Digital algorithm for dispersion correction in optical coherence tomography for homogeneous and stratified media. Appl Opt 2003;42:204-17. [Crossref] [PubMed]
- Yang F, Kemp CJ, Henikoff S. Anthracyclines induce double-strand DNA breaks at active gene promoters. Mutat Res 2015;773:9-15. [Crossref] [PubMed]