Neuroanatomical substrates underlying contrast sensitivity
Introduction
Contrast sensitivity (CS), a measurement of the ability to discriminate an object from its background, is an essential domain of visual functions (1,2). CS decline or impairment indicates one’s disability to discern an object in low contrast, which has been related to poor performance in driving (3,4), face recognition (5), real-world mobility tasks (6,7), postural stability (8), reading processes (9,10), and other functions of everyday life (11). Aging or lesions in the eye (including the retina) as well as in thalamic or cortical locations are usually responsible for this decline or impairment (12,13). Previous studies in animals have suggested that lesions in medial posterior thalamus and striate cortex significantly depress CS (14,15). However, whether neuroanatomical substrates are underlying CS in humans is largely unknown. Furthermore, there is evidence that CS deficits are associated with several neurological and psychiatric disorders, such as Alzheimer’s disease (16,17), Parkinson’s disease (18) and depression (19). Thus, a better understanding of the neural mechanism of CS may have some clinical significance in understanding these brain diseases.
In this study, we aimed to explore the relationship between CS and whole-brain morphology in a cohort of healthy young participants from the Human Connectome Project (HCP) dataset (20,21). CS was assessed using the Mars Contrast Sensitivity Test, a simple portable set of contrast-calibrated charts for testing peak CS recommended by the Committee on Vision of the U.S. National Academy of Sciences and National Research Council (22-25). The scoring procedure was designed in a unified form for accuracy, simplicity, and comparability between different clinics or labs. Thus, the test has been widely and commonly used by researchers and clinicians (25). Whole-brain morphology was measured by using voxel-based morphometry (VBM) analysis (26) based on the high-resolution structural magnetic resonance imaging (MRI) data. A prior functional MRI study has demonstrated that the CS is linked to neural activity in V3A located in the hierarchy of cortical visual areas that is intermediate between lower and higher tier areas (27). Therefore, we hypothesized that variability in morphology in this area would be related to inter-individual differences in CS.
Methods
Participants
One hundred subjects (46 males) were selected from the HCP “100 Unrelated Subjects” dataset (http://www.humanconnectome.org) (21). These participants are healthy young adults without documented history of major psychiatric, neurological or physical disorders and within a restricted age range of 22–36 years, which corresponds to a period after the completion of significant neurodevelopment and before the onset of neurodegenerative changes. The full set of inclusion and exclusion criteria is detailed in prior publications (20,21). All methods were performed in accordance with the relevant guidelines and regulations by the Institutional Review Board of Washington University in St. Louis, MO, USA. Written informed consent was obtained from each participant.
CS scores
CS was assessed using the Mars Contrast Sensitivity Test (20). This test is a brief, valid and reliable measure that improves upon the traditional Pelli-Robson measure (23). The Mars test presents 48 letters of the same size, but each letter decreases in contrast by 0.04 log unit across and down the chart. The test stops when the participant makes two consecutive errors. The final score (Mars_Final) is the log CS of the last correct letter, minus 0.04 for any mistakes that precede the two consecutive errors (22). The current norms recommend by Mars Letter Contrast Sensitivity Test USER MANUAL (http://www.marsperceptrix.com) are as follows: 0.04–0.48 represents a profound loss; 0.52–1.00 a severe loss; 1.04–1.48 a moderate loss; l.52–1.76 normal (age >60 years); 1.72–1.92 normal middle/young adult (age between 18 and 60 years).
MRI data acquisition and processing
High-resolution structural images were acquired using an HCP-customized Siemens 3.0 T “Connectome Skyra” scanner with a 32-channel head coil. The imaging parameters of the structural MRI were as follows: 3D MPRAGE T1-weighted sequence, repetition time =2,400 ms, echo time =2.14 ms, inversion time =1,000 ms, flip angle =8°, field of view =224 mm × 224 mm, matrix =320 × 320, 0.7 mm isotropic voxels, and 256 sagittal slices. The total acquisition time for the structural MRI was 7 min and 40 s. Detailed descriptions of the HCP imaging procedures can be found in previous literature (18,19).
VBM analysis was performed using the CAT12 toolbox (http://www.neuro.uni-jena.de/cat) implemented in the Statistical Parametric Mapping software (SPM12, http://www.fil.ion.ucl.ac.uk/spm). First, all the structural T1-weighted images were corrected for bias-field inhomogeneities. Second, these images were segmented into gray matter (GM), white matter (WM), cerebrospinal fluid (CSF) density maps using the “new-segment” approach, an extension of the old unified segmentation algorithm (28). In contrast to the old unified segmentation, the new-segment approach has the following advantages: (I) a slightly different treatment of the mixing proportions; (II) the use of an improved registration model; (III) the ability to use multi-spectral data; (IV) an extended set of tissue probability maps, which allows a different treatment of voxels outside the brain. Third, the Diffeomorphic Anatomical Registration using Exponentiated Lie algebra (DARTEL) technique was used to generate custom, study-specific template (29). Fourth, each participant’s GM density image was warped to the customized template; then the resultant images were affine-registered to the Montreal Neurological Institute (MNI) space and resampled to a voxel size of 1 mm × 1 mm × 1mm. Fifth, the modulation was applied by multiplying the transformed GM density (per unit volume in native space) (26) maps with the non-linear components of Jacobian determinants, which resulted in the normalized GM volume (GMV) maps representing the local native-space GMV after correcting the confounding effect of variance induced by individual whole-brain size. Actually, an analysis of modulated data tests for regional differences in the absolute amount (volume) of gray matter, whereas analysis of unmodulated data tests for regional differences in density of gray matter (30). Finally, to make a balance between compensating for registration errors and reserving anatomical details, the GMV images were smoothed with a moderate full-width at half-maximum (FWHM) Gaussian kernel of 6 mm.
Statistical analysis
We used a standard, univariate approach to investigate the relationship between the CS and the GMV in a voxel-wise manner within the whole gray matter. A multiple regression model in the SPM12 software was used to identify any voxels in the GMV maps that showed a significant association with the Mars_Final scores. The total intracranial volume was considered as a nuisance variable. Correction for multiple comparisons was performed using the non-stationary cluster-level family-wise error (FWE) method (31). Initially, the group-level statistical map was set to a threshold of voxel-level P<0.001 (cluster defining threshold). Then, all reported brain regions were corrected P<0.05 at the cluster-level using the random field theory and the FWE correction, following the current standard (32).
Results
The range of Mars_Final scores for the 100 participants was from 1.08 to 1.88. Among them, one score was 1.08 (a moderate loss), 10 scores were within l.52–1.72 (normal >age 60 years), and the remaining 89 scores were within 1.72–1.92 (normal middle/young adult). In the voxel-wise whole gray matter analysis, we found significant positive correlations (cluster-level P<0.05, FWE corrected; a minimum cluster size of 1,013 voxels) between the Mars_Final scores and the GMV in the bilateral visual cortex [left: cluster size =1,109, peak MNI coordinates x/y/z = −20.5/−88.5/38.5, peak T =4.3, partial correlation coefficient (pr) =0.429, P<0.001; right: cluster size =1,699, peak MNI coordinates x/y/z =24.5/−88.5/32.5, peak T =5.0, pr =0.467, P<0.001] (Figure 1). To rule out the potential confounds due to covariates such as age, gender and visual acuity, we repeated the partial correlation analyses controlling for these additional covariates and found that the positive correlations between the Mars_Final scores and the GMV in the bilateral visual cortex remained significant (left: pr =0.434, P<0.001; right: pr =0.450, P<0.001). However, we observed an outlier in the lower left corner in the scatter plots of Figure 1. After removal of the subject corresponding to this outlier, the positive correlations were still significant (left: pr =0.294, P=0.003; right: pr =0.310, P=0.002) (Figure 2). Furthermore, Figure 3 illustrates that the significant bilateral clusters are mainly located in bilateral V3A of the visual cortex according to the Human PALS-12 atlas (33), with the superior parts extending to the bilateral posterior parietal cortex.
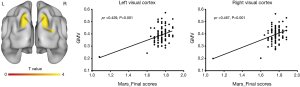
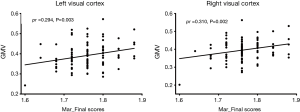
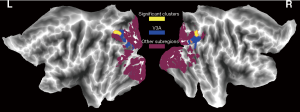
Discussion
By using high-resolution structural MRI and VBM analysis, we found that an increase in CS scores was associated with increased GMV in the bilateral V3A and its superior parts extending to the bilateral posterior parietal cortex. Despite different neuroimaging techniques (structural vs. functional MRI) (34,35) and analytic methods (morphology vs. activation), our results were highly consistent with previous studies reporting an intrinsic relation between activity in area V3A and CS (27). These findings jointly underscore the central role of V3A in CS processing. In addition, the visual cortical system can be segregated into two parallel hierarchical processing pathways anatomically and functionally, that is, ventral visual pathway from V1, V2, V4 to the inferior temporal cortex representing object shape and identity (“what”) and dorsal visual pathway from V1, V3A, MT/V5 to parietal cortex representing objects location or spatial relationships (“where”) and the more updated visuomotor control (“how”) (36,37). Our results support that CS processing mainly depends on the dorsal stream compromising V3A and posterior parietal cortex.
However, there is evidence that other areas of the visual cortex are also involved in CS. For examples, Tootell et al. reported that middle temporal visual area (MT) and V3 had a much higher CS using functional MRI in human (38). Moreover, Sclar et al. found that neurons in the area MT were more sensitive to CS than any cell in other regions along the magnocellular pathway in macaque monkeys by using electrophysiological methods (39). Some possible reasons may account for these additional findings. First, the study of Tootell et al. used moving contrast stimuli while our research used stationary contrast stimuli. One may speculate that MT and V3 are more activated by moving contrast stimuli rather than stationary contrast stimuli. Second, the purpose of the present study was to identify neuroanatomical substrates responsible for inter-individual differences in CS, while the prior studies aimed to identify neural correlates engaged in CS processing. Therefore, although these relevant regions (MT and V3) may be implicated in CS processing, their morphology does not relate to individual CS levels. Our findings suggest that when focusing on stimulus-unspecific brain morphology that is considered an inherent trait and independent of states, it is more the structure of V3A, rather than other relevant visual areas, which is related to inter-individual CS differences.
In some clinical studies, CS has been linked to a variety of neurological and psychiatric disorders. For example, previous studies have demonstrated that CS is impaired in Alzheimer’s disease (16,17) and CS measure can accurately classify mild cognitive impairment versus healthy controls (40), suggesting that CS may have promise as a novel Alzheimer’s disease biomarker. In another study, Stenc Bradvica et al. found that CS dysfunction was present as the earliest symptoms of Parkinson’s disease, and could facilitate differential diagnosis between Parkinson’s disease and essential tremor (41). Besides, Fam et al. found that visual CS was significantly lower in depressed patients and poorer visual CS was related to greater severity of depressive symptoms (42). Based on these findings, we assume that the affected posterior parietal region may partially explain the relationship between CS and disease-related cognitive, motorial and psychological impairment because the posterior parietal cortex has a functional and anatomical connection to the prefrontal cortex (43) and involves various cognitive domains (44-46). Therefore, clinicians should pay more attention to CS in patients with these brain disorders and CS test may assist clinicians in the early diagnosis and effective treatment.
There are several limitations to this study. First, the HCP sample included only healthy young adults with an age range from 22 to 36 years may lead to the failure to cover a good range of the CS variable, which may limit the sensitivity of the study and generalizability of the findings. To further improve our understanding of individual variability in CS, future research is encouraged by enrolling a cohort of subjects with different degrees of CS damage and broader age range. Second, while the Mars Contrast Sensitivity Test is the most frequently used test for the assessment of CS, it also depends on other visual functions such as visual acuity, which could have influenced our interpretations to some extent. Third, the correlational nature of analyses does not resolve causality. Cortical morphological variability may contribute to individual differences in CS performance. However, we cannot rule out the possibility that different CS experience might lead to changes in cortical morphology.
In conclusion, we found an association between CS and morphology in the V3A and adjacent posterior parietal cortex, suggesting the critical role of the dorsal visual stream in CS processing. These findings may provide insights into the neuroanatomical mechanism of CS and its relation to some brain disorders.
Acknowledgements
Data were provided by the Human Connectome Project, WU-Minn Consortium (principal investigators: David Van Essen and Kamil Ugurbil; 1U54MH091657) funded by the 16 NIH Institutes and Centers that support the NIH Blueprint for Neuroscience Research; and by the McDonnell Center for Systems Neuroscience at Washington University.
Funding: The work was supported by the National Natural Science Foundation of China (grant numbers: 81801679 and 81571308).
Footnote
Conflicts of Interest: The authors have no conflicts of interest to declare.
Ethical Statement: The study was approved by the Institutional Review Board of Washington University in St. Louis, MO, USA.
References
- Kelly DH. Visual contrast sensitivity. Opt Acta (Lond) 2010;24:107-29. [Crossref]
- Owsley C. Contrast sensitivity. Ophthalmol Clin North Am 2003;16:171-7. [Crossref] [PubMed]
- Wood JM, Troutbeck R. Elderly drivers and simulated visual impairment. Optom Vis Sci 1995;72:115-24. [Crossref] [PubMed]
- Owsley C, Stalvey BT, Wells J, Sloane ME, McGwin G Jr. Visual risk factors for crash involvement in older drivers with cataract. Arch Ophthalmol 2001;119:881-7. [Crossref] [PubMed]
- Owsley C, Sloane ME. Contrast sensitivity, acuity, and the perception of 'real-world' targets. Br J Ophthalmol 1987;71:791-6. [Crossref] [PubMed]
- Kuyk T, Elliott JL, Fuhr PS. Visual correlates of mobility in real world settings in older adults with low vision. Optom Vis Sci 1998;75:538-47. [Crossref] [PubMed]
- Turano KA, Geruschat DR, Stahl JW, Massof RW. Perceived visual ability for independent mobility in persons with retinitis pigmentosa. Invest Ophthalmol Vis Sci 1999;40:865-77. [PubMed]
- Lord SR, Menz HB. Visual contributions to postural stability in older adults. Gerontology 2000;46:306-10. [Crossref] [PubMed]
- Whittaker SG, Lovie-Kitchin J. Visual requirements for reading. Optom Vis Sci 1993;70:54-65. [Crossref] [PubMed]
- Williams MJ, Stuart GW, Castles A, McAnally KI. Contrast sensitivity in subgroups of developmental dyslexia. Vision Res 2003;43:467-77. [Crossref] [PubMed]
- West SK, Rubin GS, Broman AT, Munoz B, Bandeen-Roche K, Turano K. How does visual impairment affect performance on tasks of everyday life? The SEE Project. Salisbury Eye Evaluation. Arch Ophthalmol 2002;120:774-80. [Crossref] [PubMed]
- Faye EE. Contrast sensitivity tests in predicting visual function. Int Congr Ser 2005;1282:521-4. [Crossref]
- Owsley C. Aging and vision. Vision Res 2011;51:1610-22. [Crossref] [PubMed]
- Legg CR. Spatial contrast and flicker sensitivity following medial thalamic or visual cortex lesions in hooded rats. Behav Brain Res 1986;19:41-7. [Crossref] [PubMed]
- Lehmkuhle S, Sherman SM, Kratz KE. Spatial contrast sensitivity of dark-reared cats with striate cortex lesions. J Neurosci 1984;4:2419-24. [Crossref] [PubMed]
- Hutton JT, Morris JL, Elias JW, Poston JN. Contrast sensitivity dysfunction in Alzheimer's disease. Neurology 1993;43:2328-30. [Crossref] [PubMed]
- Gilmore GC, Whitehouse PJ. Contrast sensitivity in Alzheimer's disease: a 1-year longitudinal analysis. Optom Vis Sci 1995;72:83-91. [Crossref] [PubMed]
- Pieri V, Diederich NJ, Raman R, Goetz CG. Decreased color discrimination and contrast sensitivity in Parkinson's disease. J Neurol Sci 2000;172:7-11. [Crossref] [PubMed]
- Bubl E, Tebartz Van Elst L, Gondan M, Ebert D, Greenlee MW. Vision in depressive disorder. World J Biol Psychiatry 2009;10:377-84. [Crossref] [PubMed]
- Van Essen DC, Ugurbil K, Auerbach E, Barch D, Behrens TE, Bucholz R, Chang A, Chen L, Corbetta M, Curtiss SW, Della Penna S, Feinberg D, Glasser MF, Harel N, Heath AC, Larson-Prior L, Marcus D, Michalareas G, Moeller S, Oostenveld R, Petersen SE, Prior F, Schlaggar BL, Smith SM, Snyder AZ, Xu J, Yacoub E. The Human Connectome Project: a data acquisition perspective. Neuroimage 2012;62:2222-31. [Crossref] [PubMed]
- Van Essen DC, Smith SM, Barch DM, Behrens TE, Yacoub E, Ugurbil K. The WU-Minn Human Connectome Project: an overview. Neuroimage 2013;80:62-79. [Crossref] [PubMed]
- Arditi A. Improving the design of the letter contrast sensitivity test. Invest Ophthalmol Vis Sci 2005;46:2225-9. [Crossref] [PubMed]
- Dougherty BE, Flom RE, Bullimore MA. An evaluation of the Mars Letter Contrast Sensitivity Test. Optom Vis Sci 2005;82:970-5. [Crossref] [PubMed]
- Haymes SA, Roberts KF, Cruess AF, Nicolela MT, LeBlanc RP, Ramsey MS, Chauhan BC, Artes PH. The letter contrast sensitivity test: clinical evaluation of a new design. Invest Ophthalmol Vis Sci 2006;47:2739-45. [Crossref] [PubMed]
- Thayaparan K, Crossland MD, Rubin GS. Clinical assessment of two new contrast sensitivity charts. Br J Ophthalmol 2007;91:749-52. [Crossref] [PubMed]
- Ashburner J, Friston KJ. Voxel-based morphometry--the methods. Neuroimage 2000;11:805-21. [Crossref] [PubMed]
- Tootell RB, Mendola JD, Hadjikhani NK, Ledden PJ, Liu AK, Reppas JB, Sereno MI, Dale AM. Functional analysis of V3A and related areas in human visual cortex. J Neurosci 1997;17:7060-78. [Crossref] [PubMed]
- Ashburner J, Friston KJ. Unified segmentation. Neuroimage 2005;26:839-51. [Crossref] [PubMed]
- Ashburner J. A fast diffeomorphic image registration algorithm. Neuroimage 2007;38:95-113. [Crossref] [PubMed]
- Good CD, Johnsrude IS, Ashburner J, Henson RN, Friston KJ, Frackowiak RS. A voxel-based morphometric study of ageing in 465 normal adult human brains. Neuroimage 2001;14:21-36. [Crossref] [PubMed]
- Hayasaka S, Phan KL, Liberzon I, Worsley KJ, Nichols TE. Nonstationary cluster-size inference with random field and permutation methods. Neuroimage 2004;22:676-87. [Crossref] [PubMed]
- Eklund A, Nichols TE, Knutsson H. Cluster failure: Why fMRI inferences for spatial extent have inflated false-positive rates. Proc Natl Acad Sci U S A 2016;113:7900-5. [Crossref] [PubMed]
- Van Essen DC. A. Population-Average, Landmark- and Surface-based (PALS) atlas of human cerebral cortex. Neuroimage 2005;28:635-62. [Crossref] [PubMed]
- Shi L, Du FL, Sun ZW, Zhang L, Chen YY, Xie TM, Li PJ, Huang S, Dong BQ, Zhang MM. Radiation-induced gray matter atrophy in patients with nasopharyngeal carcinoma after intensity modulated radiotherapy: a MRI magnetic resonance imaging voxel-based morphometry study. Quant Imaging Med Surg 2018;8:902-9. [Crossref] [PubMed]
- Su H, Zuo C, Zhang H, Jiao F, Zhang B, Tang W, Geng D, Guan Y, Shi S. Regional cerebral metabolism alterations affect resting-state functional connectivity in major depressive disorder. Quant Imaging Med Surg 2018;8:910-24. [Crossref] [PubMed]
- Creem SH, Proffitt DR. Defining the cortical visual systems: "what", "where", and "how". Acta Psychol (Amst) 2001;107:43-68. [Crossref] [PubMed]
- Freud E, Plaut DC, Behrmann M. 'What' Is Happening in the Dorsal Visual Pathway. Trends Cogn Sci 2016;20:773-84. [Crossref] [PubMed]
- Tootell RB, Reppas JB, Kwong KK, Malach R, Born RT, Brady TJ, Rosen BR, Belliveau JW. Functional analysis of human MT and related visual cortical areas using magnetic resonance imaging. J Neurosci 1995;15:3215-30. [Crossref] [PubMed]
- Sclar G, Maunsell JH, Lennie P. Coding of image contrast in central visual pathways of the macaque monkey. Vision Res 1990;30:1-10. [Crossref] [PubMed]
- Risacher SL, Wudunn D, Pepin SM. MaGee TR, McDonald BC, Flashman LA, Wishart HA, Pixley HS, Rabin LA, Pare N, Englert JJ, Schwartz E, Curtain JR, West JD, O'Neill DP, Santulli RB, Newman RW, Saykin AJ. Visual contrast sensitivity in Alzheimer's disease, mild cognitive impairment, and older adults with cognitive complaints. Neurobiol Aging 2013;34:1133-44. [Crossref] [PubMed]
- Stenc Bradvica I, Bradvica M, Matic S, Reisz-Majic P. Visual dysfunction in patients with Parkinson's disease and essential tremor. Neurol Sci 2015;36:257-62. [Crossref] [PubMed]
- Fam J, Rush AJ, Haaland B, Barbier S, Luu C. Visual contrast sensitivity in major depressive disorder. J Psychosom Res 2013;75:83-6. [Crossref] [PubMed]
- Zanto TP, Rubens MT, Thangavel A, Gazzaley A. Causal role of the prefrontal cortex in top-down modulation of visual processing and working memory. Nat Neurosci 2011;14:656-61. [Crossref] [PubMed]
- Rawley JB, Constantinidis C. Neural correlates of learning and working memory in the primate posterior parietal cortex. Neurobiol Learn Mem 2009;91:129-38. [Crossref] [PubMed]
- Rishel CA, Huang G, Freedman DJ. Independent category and spatial encoding in parietal cortex. Neuron 2013;77:969-79. [Crossref] [PubMed]
- Rutishauser U, Aflalo T, Rosario ER, Pouratian N, Andersen RA. Single-Neuron Representation of Memory Strength and Recognition Confidence in Left Human Posterior Parietal Cortex. Neuron 2018;97:209-20. [Crossref] [PubMed]