Quantitative radiomic model for predicting malignancy of small solid pulmonary nodules detected by low-dose CT screening
Introduction
Lung cancer is the leading cause of cancer-related death worldwide (1). It has the highest rate of incidence and mortality in China (2). Low-dose computed tomography (LDCT) is the most widely used modality for early lung cancer detection and mortality reduction (3,4). Research has shown that among LDCT screening participants, the prevalence of small solid pulmonary nodules (SSPNs) was higher than that of non-solid nodules (NSNs) and part-solid nodules (PSNs), while other studies have demonstrated that malignancy is most frequently detected in SSPNs larger than 6 mm (5,6). As reported by the International Early Lung Cancer Action Program (I-ELCAP) (6), only 0.3% of the nodules less than 6 mm at the baseline screening round were found to be malignant. However, with increasing diameter, the prevalence of malignancy with an SSPN ≥6 mm on the baseline round of screening was sufficiently high that it prompted additional work-up in most screening guidelines.
Moreover, the growth rate of malignant SSPNs are more rapid, and their volume doubling time is faster than that of the sub-solid nodules (GGNs and PSNs) (7-9), which increases the urgency of making an early diagnosis. However, the imaging characteristics, such as pleural tag, spiculation and lobulation, are less specific for SSPNs of 6 to 15 mm (5,10) compared to larger ones, and there is greater overlap in features between benign and malignant nodules. Most of the indeterminate SSPNs detected by screening CT scans need annual repeat screening or follow-up scan with relevant guidelines, such as the ACR Lung RADS.
Radiomics, via high-throughput extraction of large numbers of image features from radiographic images (11), has been used to build descriptive and predictive models which relate image features to tumor characteristics, and can thereby provide valuable diagnostic and prognostic information. Radiomics has been shown to have significantly higher accuracy in predicting malignancy (12,13), and has four categories of quantitative descriptor features: morphological, statistical, regional, and model-based (14). These provide a greater differential diagnosis of lung nodules than radiology can offer. Despite the available knowledge, there are no studies that are specifically focused on the usefulness of radiomics for predicting malignancy in 6–15 mm SSPNs in LDCT screening for lung cancer. The present study aimed to develop a radiomic predictive model on the differential diagnosis of SSPNs in this size category and to compare its performance with radiology using the ACR Lung-RADS.
Methods
Patients
Ethical approval was obtained for this study, and the necessity to obtain informed consent was waived as the data were analyzed retrospectively and anonymously.
The inclusion criteria were as follows: (I) LDCT scan with 1 mm slice thickness; (II) detection of solid pulmonary nodule (6–15 mm in diameter) without calcification typical for benign lesion; (III) final pathological confirmation or clinical diagnosis based on long-term follow-up available for each nodule. The exclusion criteria were as follows: (I) respiratory artifacts that potentially affected the lesion characterization; (II) nodules with obscure border, which limited the ability to perform robust segmentation.
The nodule evaluation process was performed by two radiologists (3 and 15 years of experience in chest imaging). A total of 294 cases (199 men, 95 women; average age, 52.1±9.6 years; age range 40–79 years) with 294 solid lung nodules detected from September 2011 to December 2017 in one institution were enrolled in this study. Sixty-one of the 294 nodules were malignant, and included adenocarcinoma (n=39), squamous cell carcinoma (n=16), small cell carcinoma (n=5), and large cell carcinoma (n=1). The remaining 233 nodules were confirmed as benign based either on stability during long term follow-up (n=209) or pathological diagnosis, including tuberculoma (n=9), inflammatory granulomas (n=6), pulmonary lymph node (n=4), sclerosing alveolar cytoma (n=4) and pleural fibrous tumor (n=1). Based on the number of cases and using a conventional protocol for modeling, the 294 nodules were divided into a training data set (156 benign, 40 malignant) and a validation data set (77 benign, 21 malignant). Computer-generated random numbers were used to assign cases.
Image acquisition
The non-contrast enhanced CT scan was performed using a 16-row detector scanner (Somatom Sensation, Siemens Healthcare, Germany). The acquisition parameters were as follows: tube voltage of 120 kV, tube current of 20–60 mAs, pitch of 0.75, B50 kernel, 512×512 matrix size, and 1 mm section thickness. All of the CT images were retrieved from the picture archiving and communication system (Neusoft, Shenyang, China) for post processing.
Segmentation and imaging texture analysis
CT images were displayed with a window level of −600 Hounsfield units (HU) and a window width of 1,500 HU. All target nodules were successfully segmented in 3D with a manual single-click ensemble segmentation approach, running on the ITK-SNAP platform (an open-source software on the internet). The extraction of radiomic features based on the volume of interest (VOI) was completed by using in-house texture analysis algorithms implemented in Analysis-Kinetic (Version 1.0.3, GE Healthcare, Guangzhou, China). The inter-observer reproducibility was initially analyzed with 20 randomly chosen images for VOI based morphological features extraction by two experienced radiologists (Liting Mao and Mingzhu Liang with 3 and 15 years of experience in chest CT imaging respectively) in a blinded form. The same two radiologists who were blinded to the final diagnosis also classified the nodules of the validation sets into four categories according to the ACR Lung-RADS (15). The inter-observer and intra-observer consistency of lung nodule classification were measured, and the intra-observer consistency test was based on repeated observations after a 6-month interval.
All images were resampled into 8-bit gray level scale (256 different gray levels) images for the extractions of second-order features. In total, 385 radiomic features were extracted from the CT images of the nodules, and of these, 329 quantitative features including morphological features and statistical features were finally selected as some of the features could not be obtained in portions of the nodules. The statistical features were further classified into histogram statistical (first order) features and texture (higher-order) features.
Statistical analysis
The statistical analysis was performed with R software, version 3.0.1 (http://www.R-project.org). The reported statistical significance levels were all two-sided, with the statistical significance level set at P<0.05.
The differences in age, sex and mean follow-up time between the training and validation data sets were assessed by using an independent samples t-test, χ2, or Mann-Whitney U test, where appropriate. Comparisons of morphological related features and histogram measurements between benign and malignant nodules were made using t-tests. The inter-observer and intra-observer agreement of lung nodule classification were analyzed by McNemar method, and the statistic κ was calculated.
Intra-class correlation coefficient (ICC) was determined to assess the inter-observer agreement for nodule segmentations. Inter-observer agreement was considered as slight (ICC =0.11–0.40), fair (ICC =0.41–0.60), moderate (ICC =0.61–0.80), and good (ICC ≥0.81–1.00).
Feature selection and radiomic score-based model construction
We used the principle of the least redundant and maximum correlation to select out the non-redundant and optimized quantitative image features on the training data set. Since the quantitative radiomic features did not have a normal distribution, Kruskal-Wallis Test was employed to select the features that were statistically different between the benign and malignant groups, and Spearman correlation analysis was used to exclude the highly interrelated features based on a correlation coefficient of r≥0.9. The least absolute shrinkage and selection operator (LASSO) method, which is appropriate for the reduction of high-dimensional data (16), was applied to select the most important predictive features from the training data set. The selected features were then combined into a linear regression equation, and a radiomic score (Rad-score) was computed for each case.
Comparison between radiomic model and ACR Lung-RADS in predicting lung cancer
According to the ACR Lung-RADS, nodules of category 4 are considered to be likely malignancy, and categories 1–3 are considered to be benign or likely benign lesions. Receiver operating characteristic (ROC) was performed, and the area under the curve (AUC) was calculated both in the training and validation data sets. The flowchart of this study is summarized in Figure 1.
Results
Clinical characteristics
The baseline clinical-pathologic characteristics, including age, sex, mean follow-up time of benign nodules and histologic subtype, of the patients in the training and validation dataset, are listed in Table 1. There was no difference between the training dataset and the validation dataset in regards to clinical pathologic characteristics (P=0.13–0.70).
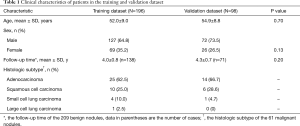
Full table
The inter-observer reproducibility of drawing VOI was high (ICC >0.92, Table 2). The intra- and inter-observer reproducibility of lung nodule classification was fairly good, with the κ values of inter-observer and intra-observer being 0.86 (P<0.0001) and 0.93 (P<0.0001) respectively. Therefore, the segmentation of nodules were determined by one radiologist (Liting Mao) while the classification was carried out by two radiologists (Liting Mao and Mingzhu Liang). If the two radiologists had different opinions, the issue was resolved through consensus; if consensus could not be reached, the issue was resolved by a third radiologist (Xueguo Liu with 30 years’ experience).

Full table
Comparison between the benign and malignant nodules
At baseline, the malignant nodules were larger in size than the benign ones (9.0±2.9 vs. 6.1±1.5 mm, P<0.001). Moreover, Maximum 3D Diameter and Spherical Disproportion of the malignant nodules were larger than those of the benign ones (P<0.001 and P=0.001). The benign nodules had greater skewness (P<0.001) and less kurtosis (P=0.021) compared to the malignant nodules. As shown in Figure 2, it was difficult to distinguish the benign (upper row) from cancerous nodules (lower row) on the baseline scan by routine morphological features, while the radiomic features including histogram features and mean attenuation were significantly differentiable between the two nodules.
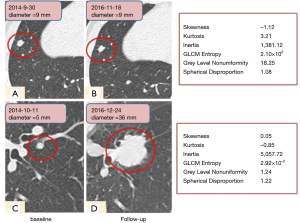
Construction of the radiomic score-based predictive model
Eleven non-redundant predictors were extracted from the 385 features based on the training set of the 196 cases (Figure 3), and those features with nonzero coefficients were used in the LASSO logistic regression model. Their coefficients are shown in Table 3. The AUC value of the training dataset was 0.953 (95% CI, 0.905–0.987).
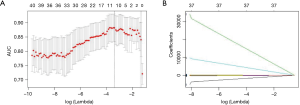
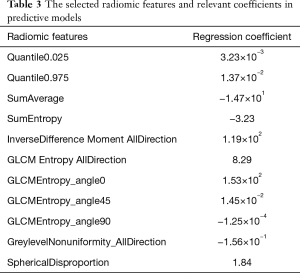
Full table
Validation of radiomic predictive model
In this study, the outcome variable was either a benign or malignant nodule, a dichotomous outcome. In the radiomic model, the P value was the standard to classify the nodules, while the optimum threshold came from ROC. As shown in Table 4 and Figure 4, the accuracy of the radiomic model is higher than that of ACR Lung-RADS (89.8% vs. 76.5%, P<0.01), with the AUC values of 0.97 and 0.77 respectively. The sensitivity and specificity was 81.0% and 92.2% respectively, using the radiomic predictive model, and 47.6% and 84.4% respectively, using the ACR lung RADS approach. Six cases were misdiagnosed in both approaches, but ACR lung RADS misdiagnosed an additional 17 cases. Illustrations of the predictive results are shown in Figure 5.
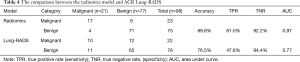
Full table
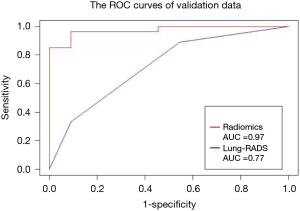
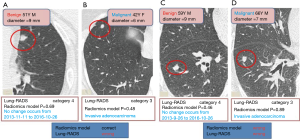
Discussion
This study aimed to develop a radiomic predictive model that could facilitate distinction between benign and malignant SSPNs. Nodule morphologic features including size, consistency, shape and volume have been reported to be correlated with invasiveness and prognosis of lung cancers (17-19), and textural features have also exhibited substantial promise as prognostic indicators in thoracic oncology (20-23). Besides the description of conventional characteristics (shape, volume etc.), invisible information including histogram, higher order features etc. can be extracted using radiomic analysis. A histogram displays the range and frequency of pixel values within the defined lesion ROI, which reflects the planar characteristics (24), while the higher order features correspond to the spatial information among pixels, thus reflecting more internal characteristics of tumor texture and heterogeneity. We selected 11 non-redundant radiomic features with statistical difference out of 385 features. The numerical value of Maximum3D Diameter and spherical disproportion in lung cancers was higher than that in benign nodules. In the histogram analysis, a significant difference was found in the kurtosis values between benign and malignant nodules, which was consistent with the results of Kamiya (12). The greater kurtosis and reduced skewness in malignant nodules may be related to the greater heterogeneity than benign nodules, although they appear to be uniformly solid nodules on CT images. These differences in internal density homogeneity are reflected by the differences in the kurtosis measurements but are not detected by conventional visual assessments.
We used the non-redundant radiomic features to build a predictive model, which were helpful for qualitative diagnosis of SSPNs with an overall accuracy of 89.8%. As far as we know, a radiomic analysis focusing on small solid lung nodules (6–15 mm) has not been thoroughly investigated. Given that a small nodule has much fewer specific image features, and solid lung cancers usually progress faster than sub-solid ones, it follows that a differential diagnosis of small solid nodules using a robust approach including the newly emerging radiomic methods may help improve the treatment of lung cancer. We compared our model with the ACR Lung-RADS system which has been widely used in lung cancer screening (15). In the data set used in the current study, the radiomic model outperformed the ACR lung RADS approach, which was consistent with another report (13). However, our predictive radiomic model had higher accuracy and sensitivity due to the fact that it included not only shape and density features, but also volumetric high-order texture information undetectable to the human eye. The discrepancy between the two studies may also be related to differences in sample size and radiomic algorithms. Furthermore, the focus of our study on small solid nodules may also account for these differences.
The segmentation of the lesion is the most challenging aspect of radiomic evaluation. Considering the complementary nature of the manual and automatic approaches, segmentation can be improved with computer-aided edge detection followed by manual rectification (25). Despite this advantage, we segmented all nodules manually because our software cannot segment nodules semi-automatically. As the report showed, high ICC implied that the features are not very sensitive to the underlying segmentation (26). We chose three morphological features, Maxi3DDiameter, surface area, and volume, in evaluating the inter-observer variation, in order to ensure that the method of our segmentation and calculation was stable. The high inter-observer agreement confirmed that the manual segmentation was robust, and consistent with those found in other reports (27-29). Balagurunathan et al. reported that the texture features in the manual segmentation were repeatable (30). Pre-procession, such as resampling, is usually used in radiomics analysis to minimize the variability in feature values due to differing voxel sizes. However, we did not use this approach considering that applying pre-processing methods like the resampling approach may add bias to the information of original images, such as in cases where the smaller grey level bound leads to better shape information but worse texture information.
There were several limitations in this study including the relatively small sample size. Firstly, it is generally understood that within the field of radiomics the accuracy of results are highly dependent on the amount of data and the consistency of the parameters used to produce the images. However, the majority of radiomics studies were based on retrospective analysis, and image parameters vary across different research institutions. In order to build practical radiomic models, multiple larger data bases and multi-center prospective studies are required. Secondly, the majority of lung nodules in the current study were less than 10 mm which might have caused error in the extraction of high-throughput quantitative features on small targets. Nevertheless, we found reliable data showing that extracting more features on small targets is durable, a result similar to that of a previous study on small pulmonary nodules which had satisfactory results (13). Because the techniques of image segmentation and feature extraction have matured in recent years, only GLCM and RLM features are affected by small ROI size as step size needs to be considered in relation to pixel number in the target. In our study, we did not find any target size smaller than the limit of the matrix step. Thirdly, we only used radiomics to do the image analysis on a small sample size. With machine learning being used successfully in recent studies (31,32), we hope to compare the two analysis approaches in the near future when larger scale screening data are available.
In conclusion, with this radiomic model, it is possible to predict malignant solid nodules 6–15 mm in diameter at baseline LDCT screening for lung cancer.
Acknowledgements
We appreciate Dr. Hongjun Jin for kindly improving the organization of this paper.
Funding: This research was funded by the Science and Technology Planning Project of Zhuhai City (grant number: 20161027E030069) and Medical Scientific Research Foundation of Guangdong Province of China (grant number: A2018254).
Footnote
Conflicts of Interest: The authors have no conflicts of interest to declare.
Ethical Statement: Ethical approval was obtained for this study, and the necessity to obtain informed consent was waived as the data were analyzed retrospectively and anonymously.
References
- Brawley OW. Avoidable cancer deaths globally. CA Cancer J Clin 2011;61:67-68. [Crossref] [PubMed]
- Available online: http://mt.sohu.com/20170318/n483773035.shtml
- Aberle DR, Adams AM, Berg CD, Black WC, Clapp JD, Fagerstrom RM, Gareen IF, Gatsonis C, Marcus PM, Sicks JD. Reduced lung-cancer mortality with low-dose computed tomographic screening. N Engl J Med 2011;365:395-409. [Crossref] [PubMed]
- Barton H, Shatti D, Jones CA, Sakthithasan M, Loughborough WW. Review of radiological screening programmes for breast, lung and pancreatic malignancy. Quant Imaging Med Surg 2018;8:525-34. [Crossref] [PubMed]
- McWilliams A, Tammemagi MC, Mayo JR, Roberts H, Liu G, Soghrati K, Yasufuku K, Martel S, Laberge F, Gingras M, Atkar-Khattra S, Berg CD, Evans K, Finley R, Yee J, English J, Nasute P, Goffin J, Puksa S, Stewart L, Tsai S, Johnston MR, Manos D, Nicholas G, Goss GD, Seely JM, Amjadi K, Tremblay A, Burrowes P, MacEachern P, Bhatia R, Tsao MS, Lam S. Probability of cancer in pulmonary nodules detected on first screening CT. N Engl J Med 2013;369:910-9. [Crossref] [PubMed]
- Yip R, Henschke CI, Yankelevitz DF, Smith JP. CT screening for lung cancer: alternative definitions of positive test result based on the national lung screening trial and international early lung cancer action program databases. Radiology 2014;273:591-6. [Crossref] [PubMed]
- Liu X, Liang M, Wang Y, Chen K, Chen X, Qin P, He J, Yi X. The outcome differences of CT screening for lung cancer pre and post following an algorithm in Zhuhai, China. Lung Cancer 2011;73:230-6. [Crossref] [PubMed]
- Mikita K, Saito H, Sakuma Y, Kondo T, Honda T, Murakami S, Oshita F, Ito H, Tsuboi M, Nakayama H, Yokose T, Kameda Y, Noda K, Yamada K. Growth rate of lung cancer recognized as small solid nodule on initial CT findings. Eur J Radiol 2012;81:e548-53. [Crossref] [PubMed]
- Song YS, Park CM, Park SJ, Lee SM, Jeon YK, Goo JM. Volume and mass doubling times of persistent pulmonary subsolid nodules detected in patients without known malignancy. Radiology 2014;273:276-84. [Crossref] [PubMed]
- Ost D, Fein A. Evaluation and management of the solitary pulmonary nodule. Am J Respir Crit Care Med 2000;162:782-7. [Crossref] [PubMed]
- Lambin P, Rios-Velazquez E, Leijenaar R, Carvalho S, van Stiphout RG, Granton P, Zegers CM, Gillies R, Boellard R, Dekker A, Aerts HJ. Radiomics: extracting more information from medical images using advanced feature analysis. Eur J Cancer 2012;48:441-6. [Crossref] [PubMed]
- Kamiya A, Murayama S, Kamiya H, Yamashiro T, Oshiro Y, Tanaka N. Kurtosis and skewness assessments of solid lung nodule density histograms: differentiating malignant from benign nodules on CT. Jpn J Radiol 2014;32:14-21. [Crossref] [PubMed]
- Hawkins S, Wang H, Liu Y, Garcia A, Stringfield O, Krewer H, Li Q, Cherezov D, Gatenby RA, Balagurunathan Y, Goldgof D, Schabath MB, Hall L, Gillies RJ. Predicting Malignant Nodules from Screening CT Scans. J Thorac Oncol 2016;11:2120-8. [Crossref] [PubMed]
- Lee G, Lee HY, Park H, Schiebler ML, van Beek EJ, Ohno Y, Seo JB, Leung A. Radiomics and its emerging role in lung cancer research, imaging biomarkers and clinical management: State of the art. Eur J Radiol 2017;86:297-307. [Crossref] [PubMed]
- Available online: https://www.acr.org/Quality-Safety/Resources/LungRADS
- Sauerbrei W, Royston P, Binder H. Selection of important variables and determination of functional form for continuous predictors in multivariable model building. Stat Med 2007;26:5512-28. [Crossref] [PubMed]
- Einenkel J, Braumann UD, Horn LC, Pannicke N, Kuska JP, Schutz A, Hentschel B, Hockel M. Evaluation of the invasion front pattern of squamous cell cervical carcinoma by measuring classical and discrete compactness. Comput Med Imaging Graph 2007;31:428-35. [Crossref] [PubMed]
- Detterbeck FC, Gibson CJ. Turning gray: the natural history of lung cancer over time. J Thorac Oncol 2008;3:781-92. [Crossref] [PubMed]
- Tan BB. KFKE. The solitary pulmonary nodule. Chest 2003;123:89S-96S. [Crossref] [PubMed]
- Pyka T, Bundschuh RA, Andratschke N, Mayer B, Specht HM, Papp L, Zsótér N, Essler M. Textural features in pre-treatment [F18]-FDG-PET/CT are correlated with risk of local recurrence and disease-specific survival in early stage NSCLC patients receiving primary stereotactic radiation therapy. Radiat Oncol 2015;10:100. [Crossref] [PubMed]
- Fried DV, Tucker SL, Zhou S, Liao Z, Mawlawi O, Ibbott G, Court LE. Prognostic value and reproducibility of pretreatment CT texture features in stage III non-small cell lung cancer. Int J Radiat Oncol Biol Phys 2014;90:834-42. [Crossref] [PubMed]
- Ganeshan B, Panayiotou E, Burnand K, Dizdarevic S, Miles K. Tumour heterogeneity in non-small cell lung carcinoma assessed by CT texture analysis: a potential marker of survival. Eur Radiol 2012;22:796-802. [Crossref] [PubMed]
- Dennie C, Thornhill R, Souza CA, Odonkor C, Pantarotto JR, MacRae R, Cook G. Quantive texture analynis on pre-treatment computed tomography predicts local recurrence in stage-I non-small cell lung cancer following stereotactic radiation therapy. Quant Imag Med Surg 2017;6:614-22. [Crossref]
- Bae KT, Fuangtharnthip P, Prasad SR, Joe BN, Heiken JP. Adrenal masses: CT characterization with histogram analysis method. Radiology 2003;228:735-42. [Crossref] [PubMed]
- Gillies RJ, Kinahan PE, Hricak H. Radiomics: Images Are More than Pictures, They Are Data. Radiology 2016;278:563-77. [Crossref] [PubMed]
- Kalpathy-Cramer J, Mamomov A, Zhao B, Lu L, Cherezov D, Napel S, Echegaray S, Rubin D, McNitt-Gray M, Lo P, Sieren JC, Uthoff J, Dilger SK, Driscoll B, Yeung I, Hadjiiski L, Cha K, Balagurunathan Y, Gillies R, Goldgof D. Radiomics of Lung Nodules: A Multi-Institutional Study of Robustness and Agreement of Quantitative Imaging Features. Tomography 2016;2:430-7. [Crossref] [PubMed]
- Rios Velazquez E, Aerts HJ, Gu Y, Goldgof DB, De Ruysscher D, Dekker A, Korn R, Gillies RJ, Lambin P. A semiautomatic CT-based ensemble segmentation of lung tumors: comparison with oncologists' delineations and with the surgical specimen. Radiother Oncol 2012;105:167-73. [Crossref] [PubMed]
- van Dam IE, van Sörnsen de Koste JR, Hanna GG, Muirhead R, Slotman BJ, Senan S. Improving target delineation on 4-dimensional CT scans in stage I NSCLC using a deformable registration tool. Radiother Oncol 2010;96:67-72. [Crossref] [PubMed]
- Parmar C, Rios VE, Leijenaar R, Jermoumi M, Carvalho S, Mak RH, Mitra S, Shankar BU, Kikinis R, Haibe-Kains B, Lambin P, Aerts HJ. Robust Radiomics feature quantification using semiautomatic volumetric segmentation. Plos One 2014;9:e102107. [Crossref] [PubMed]
- Balagurunathan Y, Gu Y, Wang H, Kumar V, Grove O, Hawkins S, Kim J, Goldgof DB, Hall LO, Gatenby RA, Gillies RJ. Reproducibility and Prognosis of Quantitative Features Extracted from CT Images. Transl Oncol 2014;7:72-87. [Crossref] [PubMed]
- Sun R, Limkin EJ, Vakalopoulou M, Dercle L, Champiat S, Han SR, Verlingue L, Brandao D, Lancia A, Ammari S, Hollebecque A, Scoazec JY, Marabelle A, Massard C, Soria JC, Robert C, Paragios N, Deutsch E, Ferte C. A radiomics approach to assess tumour-infiltrating CD8 cells and response to anti-PD-1 or anti-PD-L1 immunotherapy: an imaging biomarker, retrospective multicohort study. Lancet Oncol 2018;19:1180-91. [Crossref] [PubMed]
- Wang K, Lu X, Zhou H, Gao Y, Zheng J, Tong M, Wu C, Liu C, Huang L, Jiang T, Meng F, Lu Y, Ai H, Xie XY, Yin LP, Liang P, Tian J, Zheng R. Deep learning Radiomics of shear wave elastography significantly improved diagnostic performance for assessing liver fibrosis in chronic hepatitis B: a prospective multicentre study. Gut 2018. Epub ahead of print. [Crossref] [PubMed]