Quantitative features can predict further growth of persistent pure ground-glass nodule
Introduction
Pure ground-glass opacity (GGO) nodule has been detected with an increasing rate as the comprehensive introduction of low-dose CT in the course of lung cancer screening, can be managed by long-term follow-up or elective surgery. Persistent GGN is a group of focal lesions of increased attenuation without a solid internal component that either remains stable or experiences growth during follow-up (1). Nodule growth was defined as an increase of at least 2 mm in size or the development of a solid internal component, which would be an indication for surgical resection. These nodules have a great heterogeneity, which has been shown in previous studies to correspond pathologically to invasive pulmonary adenocarcinoma or pre-invasive lesions in resected lesions with nodule growth (2-6). Therefore, it is of utmost importance to differentiate between stable PGGN and PGGN with high potential in further growth accurately in clinical practice.
The assessment of nodule growth was performed by an experienced radiologist who visually examined and manually measured the interested nodule. Thus, there may be some nodules experiencing three-dimensional development which would be omitted by optical measurement. With the broad application of quantitative imaging analysis, it has revealed that quantitative features potentially allowed excellent nodule characterization and differentiation (7-13). The accuracy comparison of nodule growth detection between radiologist assessment and quantitative image analysis has not been reported. Herein, our study was aimed to investigate if the quantitative features assessment can evaluate the actual growth rate of PGGNs and predict further nodule growth more accurately.
Methods
Study population
The Hospital Institutional Review Board approved this retrospective study with a waiver for informed patient consent. CT images, whose radiological reports included descriptive words referring to PGGNs, were retrieved from the radiology database of our hospital. CT images reviewed by an experienced radiologist were eligible for the study according to several criteria: (I) PGGNs with long-axis diameter ≥5 mm but ≤3 cm in the initial CT; (II) confirmation of persistent PGGNs in the follow-up CT scans 3−6 months after first CT scan; (III) no history of previous or concurrent malignancy; (IV) follow-up period >3 years for stable nodules; and (V) all initial and follow-up CT scans were performed with section thickness <1 mm. In our hospital, patients who had PGGN larger than 10−15 mm or displayed strong anxiety because of the persistent lung nodules and desired surgery underwent surgical intervention. From January 2011 to December 2012, 59 patients with 101 PGGNs were included in this study.
Image acquisition and analysis
All unenhanced CT images were obtained by two different manufacturers: Siemens and Philips. For both manufacturers, CT scans were acquired at full inspiration without contrast medium at 120 KVp tube energy and 200 mAs effective dose. In the Siemens group, a Somatom Definition AS scanner (64×0.625 mm detector, 1.0 pitch) was used. All images were reconstructed at 1.0 mm slice thickness, with an increment of 0.7 mm and a standard soft kernel (Siemens B31 filter, Siemens Medical Solutions, Forchheim, Germany). In the Philips group, scans were conducted using a Brilliance 40 scanner (40×0.625 mm detector configuration, 0.4 pitch). The image was reconstructed at 1.0 mm slice thickness, with an increment of 0.7 mm and a sharp reconstruction kernel (C filter, Philips, Cleveland, OH). Nodule segmentation was performed by a radiologist and confirmed by another experienced radiologist using 3D slicer (14) in a semi-automatic GrowCut approach (www.slicer.org). All segmentations were performed under lung window settings at the width of 1,400 Hounsfield Unit (HU) and a level of −450 HU. After that, the quantitative features (volumetric and texture) for the selected nodule were automatically calculated in the Slicer Radiomics package (15).
Definition of quantitative features and nodule growth assessment
The 3D maximum diameter (MD) was measured as the most substantial pairwise Euclidean distance between voxels on the surface of the nodule volume. The volume was determined by counting the number of pixels in the nodule region and multiplying the value with the voxel size. The following equation attained the mass: Mass = Volume × [(Mean + 1000) × 0.001], in which the value of Mean is the mean attenuation of HU. Algorithms for the calculation of other features are described in the Table S1. To test the stability of quantitative feature extraction, nodule segmentation was performed by another radiologist on the last follow-up CT scan, and the interobserver ICC (intraclass coefficient) was calculated (Figures S1,S2). In the quantitative analysis, PGGN growth was defined based on two parameters: (I) an increase of at least 2 mm in MD and (II) an increase of at least 30% in volume or mass (Figures S3,S4). In the radiological assessment, the growth of PGGNs was defined based on two parameters: (I) an increase of at least 2 mm in long-axis diameter and (II) the emergence of a solid component.
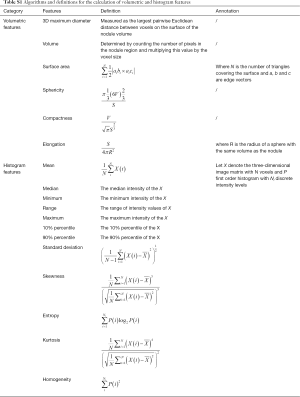
Full table
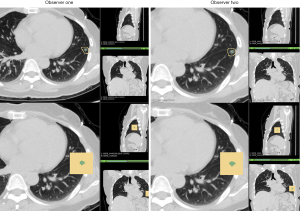
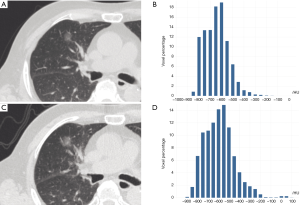
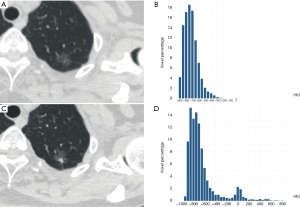
Statistical analysis
Continuous variables were compared using the independent sample t-test or Mann-Whitney U-test, and categorical data were analyzed by chi-square analysis. Variables with P<0.05 were introduced as potential predictors into the logistic regression analysis. To connect the information of time to nodule growth, variables were also analyzed using a Cox proportional hazard model. In multivariate analysis, a backward stepwise selection model was applied with the iterative entry of variables based on test results (P<0.05), and the removal of variables was based on likelihood ratio statistics with a probability of 0.10. A receiver operating characteristic curve (ROC) analysis was performed to determine the cutoff value of selected variables.
Results
Baseline characteristics of the study participants
The demographic and clinical characteristics of the 59 included participants are summarized in Table 1. There were 19 females and 40 males with a median age of 61 years (range, 40−85 years). A single nodule was detected in 32 patients, bi-synchronous nodules in 16 patients, and tri- and Quadri-synchronous nodules in 7 and 4, respectively. 101 PGGNs were identified in this study entirely. The median follow-up period was 52 months with a range of 32 to 69 months. During the follow-up, nodule growth was detected in 10 patients via radiological assessment and in 16 patients via quantitative analysis. The frequency of nodule growth was 27.6% (16/58) and 15.8% (16/101) by per-person and per-nodule analysis, respectively.
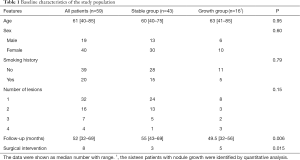
Full table
According to the presence or absence of nodule growth, participants were divided into two groups. Except patients with nodule growth who most likely received the surgical intervention (7% versus 32%, P=0.015), there were no significant differences in age, sex, or smoking history.
Nodule growth detected by radiologists or quantitative analysis
Based on long-axis diameter increase and the emergence of solid components that were evaluated by radiologists (Figure 1), 10 out of 101 nodules were identified in 10 patients. Among the 10 PGGN with further growth, seven had long-axis diameters that had increased by >2 mm, and three had solid component emergence. As the ICCs were larger than 0.8 for most quantitative features (see Figure S2), the quantitative analysis showed a high level of reproducibility. For all of the included patients, three parameters including 3D MD, volume, and mass were also calculated to detect nodule growth by quantitative analysis. Twelve nodules with further growth in 3D MD were detected, 12 in nodule mass, and 10 in nodule volume according to the prespecified criteria. Through the combined use of these three parameters, 16 nodules were identified with further growth during the same follow-up period. Thus, compared to radiological assessment, another six nodules were also detected with occult growth on the quantitative analysis.
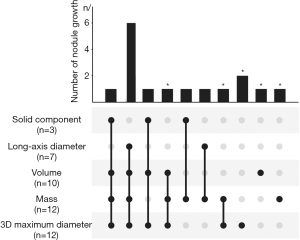
Prediction of nodule growth using quantitative CT features on initial CT scan
As listed in Table 2, the quantitative analysis of the initial CT scan was performed on volumetric and texture features. In the comparison of nodules with and without growth, significant differences were found in most of the volumetric features, including 3D MD, volume, mass, and surface area (P<0.05). Nodules with further growth were significantly larger than stable nodules on the initial CT scan in terms of 3D MD (14.3±3.6 versus 8.9±2.6 mm; P=0.040), volume (933.0±600.2 versus 290.7±238.4 mm3; P<0.001), mass (244.3±247.9 versus 58.9±71.7 mg; P<0.001), and surface area (525.5±238.1 versus 227.1±124.1 mm2; P<0.001). However, most of the texture features showed no significant differences between the stable and growth nodules, except for the 90% percentile and standard deviation (P<0.001).
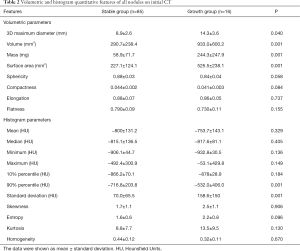
Full table
3D MD, volume, mass, surface area, 90% percentile, and standard deviation were introduced as input variables for logistic regression and Cox proportional hazard analysis. The univariate and multivariate Cox proportional hazard analyses also confirmed that the 3D MD (HR, 3.75; 95% CI: 2.14−6.55; P<0.001) and standard deviation (HR, 2.06; 95% CI: 1.35−3.14; P<0.001) predicted further nodule growth (Table 3). Logistic regression analysis revealed that larger 3D MD (OR, 6.35; 95% CI: 2.59−15.54; P<0.001) and higher standard deviation (OR, 2.05; 95% CI: 1.06−3.97; P=0.033) were independent predictors of nodule growth (Table S2).
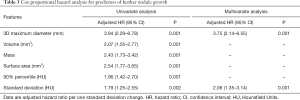
Full table

Full table
ROC analysis and risk stratification of nodule growth
Regarding the predictive performance, the area under the curve (AUC) was 0.896 (95% CI: 0.820−0.948) for the 3D MD, and the maximum Youden index (0.68: sensitivity, 93.75%; specificity, 74.12%) was obtained at a cut-off value of 10.2 mm. As for the standard deviation, AUC was 0.813 (95% CI: 0.723−0.883), and the maximum Youden index (0.59: sensitivity, 100.00%; specificity, 58.82%) was obtained at a cut-off value of 50.0 HU (Figure 2). Based on these two risk factors, PGGOs were stratified into three groups showing an increasing incidence of nodule growth (P=0.001): (I) the high-risk group consisted of nodules with 3D MD ≥10.2 mm and standard deviation of HU ≥50 HU; (II) the intermediate risk group consisted of nodules with 3D MD <10.2 mm and standard deviation of HU ≥50 HU; and (III) the lower risk group consisted of nodules with a standard deviation of <50 HU. Also, the growth rate of PGGN was 55.6% (15/27) in the high-risk group (Figure 3).
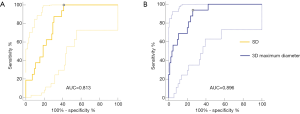
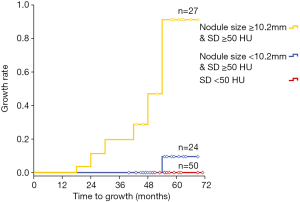
Discussion
To differentiate the nodule growth during the follow-up interval of persistent GGN is essential in clinical practice. Our study results demonstrated that 3D MD and standard deviation (SD) features were independent predictors for nodule growth. Furthermore, the ROC analysis of MD and SD attained an AUC value of 0.896 and 0.813, respectively, indicating that MD and SD could be a valuable predictor of GGN growth.
From Revel’s study that the 95% CI for the variability of two-dimension (2D) measurements among different readers was from −1.73 to 1.73 mm, the growth of nodule was defined as an increase of >2 mm (16). Similarly, previous studies determined an increase of >2 mm in long-axis diameter as an essential criterion for PGGN growth (2,5,17-19). However, 2D measurements of PGGN are unreliable because of the lower contrast of PGGN with the surrounding pulmonary parenchyma compared with that of solid nodules. Consequently, different decisions have been made among observers with relation to the location of PGGN boundary, causing great inter- and intra-observer variabilities (20). Meanwhile, recent studies showed that a semi-quantitative or quantitative assessment could improve the interobserver agreement for sub-solid nodule (21,22). Similar to previous studies (23,24), in which was indicated that mass measurement variability ranged from −19.0% to 20.6% and volume measurement variability ranged from −27.3% to 29.5%, we also defined nodule growth as an increase of at least 30% in nodule volume or mass.
Additionally, de Hoop et al. demonstrated that mass measurements could facilitate earlier nodule growth detection and were subject to less variability than volume or size measurements (7). We evaluated the nodule growth of all patients by volume, mass, and 3D MD, and another six patients were found. While 2/3 of new detection is associated with volume and mass, 2/3 is related to 3D MD. In our study, the results showed that quantitative analysis could more accurately detect the growth rate compared with conventional criteria. Therefore, not only should we focus on changes in diameter, but also mass or volume, for evaluating PGGNs with further growth more precisely in the early stage.
In this context, quantitative analysis can maximize the information obtained from the diagnostic images, thus provide precise lesion characterization. With the open source software of 3D slicer, we could reproducibly and efficiently extract robust quantitative features for evaluating 3D volumetric characteristics and texture of CT value (14). Preliminary studies of quantitative analysis in sub-solid nodules already have demonstrated that quantitative traits such as entropy, kurtosis, homogeneity, and mass were significant differentiators for invasive adenocarcinomas (8,10,11,25). We found most of the volumetric features on initial CT scans were significantly different between the stable PGGNs and PGGNs with further growth. Thus, we believe that these features provide more detailed and reliable measurements than visual morphological analysis in the assessment of PGGN.
Generally, nodule size has been regarded as an indicator of malignancy of PGGNs. It was reported that large nodule size and a history of lung cancer were risk factors for nodule growth and 10 mm was proposed as a cut-off value for PGGN size on the first CT scan (17). Recently, a prospective study identified that initial nodule size was associated with further growth of PGGNs (6). Consistently, nodule MD on the initial CT scan was determined to be a predictive factor for new growth in our study, and a cut-off value of 10.2 mm was obtained with a specificity of 93.75%. Similarly, Lee et al. reported that the optimal cut-off value for discriminating between pre-invasive lesions and invasive adenocarcinomas appearing as PGGNs was 10 mm (18). Furthermore, Lim et al. demonstrated that the size remains a determinant of invasive adenocarcinomas even in persistent PGGNs of >10 mm in diameter (26). Therefore, we believe that large PGGNs, especially those >10 mm in diameter, have a higher probability of further growth during the follow-up period.
On the other hand, tumor heterogeneity is an established feature of malignancy and is radiologically reflected by the standard deviation (SD) and entropy value of the texture features (8,10,25). In the present study, PGGNs with further growth showed higher entropy and SD value than solid nodules, but the difference of entropy feature did not reach a statistical significance. The SD value of the texture features provided the first quantifier and represented the degree of variation of pixel values within the PGGNs (8), which could suggest that nodules with further growth were shown to be more different with their internal characteristics. However, our results showed that the entropy might not be the same as SD, which could independently predict new PGGN changes. Interestingly, there was no nodule experiencing new growth when SD value was less than the cut-off value of 50 HU for nodule sizes ranging from 5.0 to 16.7 mm. One patient with SD >50 HU was found with nodule growth even MD <10.2 mm. The sensitivity of this cut-off was 100%; thus, it may be better to consider conservative observation for lesions with a standard deviation of <50 HU even if they have a larger nodule size. After combining the two independent predictors, the incidence of growth was 55.6% when MD >10.2 mm and SD >50 HU. Therefore, we suggest close follow-up or surgical intervention for these lesions, which requires further prospective validation if these results are to be applied in clinical practice.
It was reported that the 97.5% percentile value could be helpful in predicting further nodule changes (27). In the current study, the 90% percentile value of texture features on the initial CT scan was significantly higher in nodules with further growth. However, the factor was not an independent predictor in multivariate analysis. Two previous studies have proven that the mean CT attenuation of nodules could be useful in predicting PGGO growth, and nodules with further growth had a higher value of >–670 HU (28,29). Regarding our study, the majority of PGGN have a mean CT value of <–670, and it may be reasonable that the mean CT attenuation of PGGNs with growth has a no significant higher tendency compared with that of stable nodules.
The present study has several limitations in addition to those described above. First, this is a retrospective study based on a small sample in a single institution. To validate the findings, a retrospective external validation study or a prospective observational study should be conducted in the future. Second, we defined an increase of at least 30% in volume or mass as the growth of PGGN based on previous studies, but we didn’t evaluate the inter- and intra-observer variabilities. Finally, we excluded patients with a history of lung cancer because of the different protocols for follow-up, which was a risk factor for PGGN growth according to previous studies. Future prospective studies are warranted to confirm our results and to investigate the proper management of these additionally detected lung nodules in quantitative analysis.
Conclusions
The quantitative analysis was useful in identifying nodule change and predicting further growth based on the initial CT scan. PGGOs with MD >10.2 mm and SD >50.0 HU on the initial CT scan may require close follow-up or surgical intervention because of the high incidence of nodule growth.
Acknowledgements
Funding: This study received funding support from National Key R&D Program of China (grant number 2016YFC0905403 & 2016YFC0905402), Shanghai Pujiang Program (15PJD034), Science and Technology Commission of Shanghai Municipality (16411965800), and Shanghai Hospital Development Center (16CR3116B).
Footnote
Conflicts of Interest: The authors have no conflicts of interest to declare.
Ethical Statement: The Hospital Institutional Review Board approved this retrospective study with a waiver for informed patient consent.
References
- MacMahon H, Naidich DP, Goo JM, Lee KS, Leung AN, Mayo JR, Mehta AC, Ohno Y, Powell CA, Prokop M, Rubin GD, Schaefer-Prokop CM, Travis WD, Van Schil PE, Bankier AA. Guidelines for Management of Incidental Pulmonary Nodules Detected on CT Images: From the Fleischner Society 2017. Radiology 2017;284:228-43. [Crossref] [PubMed]
- Chang B, Hwang JH, Choi YH, Chung MP, Kim H, Kwon OJ, Lee HY, Lee KS, Shim YM, Han J, Um SW. Natural history of pure ground-glass opacity lung nodules detected by low-dose CT scan. Chest 2013;143:172-8. [Crossref] [PubMed]
- Scholten ET, de Jong PA, de Hoop B, van Klaveren R, van Amelsvoort-van de Vorst S, Oudkerk M, Vliegenthart R, de Koning HJ, van der Aalst CM, Vernhout RM, Groen HJ, Lammers JW, van Ginneken B, Jacobs C, Mali WP, Horeweg N, Weenink C, Thunnissen E, Prokop M, Gietema HA. Towards a close computed tomography monitoring approach for screen detected subsolid pulmonary nodules? Eur Respir J 2015;45:765-73. [Crossref] [PubMed]
- She Y, Zhao L, Dai C, Ren Y, Zha J, Xie H, Jiang S, Shi J, Shi S, Shi W, Yu B, Jiang G, Fei K, Chen Y, Chen C. Preoperative nomogram for identifying invasive pulmonary adenocarcinoma in patients with pure ground-glass nodule: A multi-institutional study. Oncotarget 2017;8:17229-38. [Crossref] [PubMed]
- Matsuguma H, Mori K, Nakahara R, Suzuki H, Kasai T, Kamiyama Y, Igarashi S, Kodama T, Yokoi K. Characteristics of subsolid pulmonary nodules showing growth during follow-up with CT scanning. Chest 2013;143:436-43. [Crossref] [PubMed]
- Kakinuma R, Noguchi M, Ashizawa K, Kuriyama K, Maeshima AM, Koizumi N, Kondo T, Matsuguma H, Nitta N, Ohmatsu H, Okami J, Suehisa H, Yamaji T, Kodama K, Mori K, Yamada K, Matsuno Y, Murayama S, Murata K. Natural History of Pulmonary Subsolid Nodules: A Prospective Multicenter Study. J Thorac Oncol 2016;11:1012-28. [Crossref] [PubMed]
- de Hoop B, Gietema H, van de Vorst S, Murphy K, van Klaveren RJ, Prokop M. Pulmonary ground-glass nodules: increase in mass as an early indicator of growth. Radiology 2010;255:199-206. [Crossref] [PubMed]
- Hwang IP, Park CM, Park SJ, Lee SM, McAdams HP, Jeon YK, Goo JM. Persistent Pure Ground-Glass Nodules Larger Than 5 mm: Differentiation of Invasive Pulmonary Adenocarcinomas From Preinvasive Lesions or Minimally Invasive Adenocarcinomas Using Texture Analysis. Invest Radiol 2015;50:798-804. [Crossref] [PubMed]
- Rios Velazquez E, Parmar C, Liu Y, Coroller TP, Cruz G, Stringfield O, Ye Z, Makrigiorgos M, Fennessy F, Mak RH, Gillies R, Quackenbush J, Aerts H. Somatic Mutations Drive Distinct Imaging Phenotypes in Lung Cancer. Cancer Res 2017;77:3922-30. [Crossref] [PubMed]
- Son JY, Lee HY, Lee KS, Kim JH, Han J, Jeong JY, Kwon OJ, Shim YM. Quantitative CT analysis of pulmonary ground-glass opacity nodules for the distinction of invasive adenocarcinoma from pre-invasive or minimally invasive adenocarcinoma. PLoS One 2014;9:e104066. [Crossref] [PubMed]
- Chae HD, Park CM, Park SJ, Lee SM, Kim KG, Goo JM. Computerized texture analysis of persistent part-solid ground-glass nodules: differentiation of preinvasive lesions from invasive pulmonary adenocarcinomas. Radiology 2014;273:285-93. [Crossref] [PubMed]
- Dennie C, Thornhill R, Souza CA, Odonkor C, Pantarotto JR, MacRae R, Cook G. Quantitative texture analysis on pre-treatment computed tomography predicts local recurrence in stage I non-small cell lung cancer following stereotactic radiation therapy. Quant Imaging Med Surg 2017;7:614-22. [Crossref] [PubMed]
- Gavrielides MA, Berman BP, Supanich M, Schultz K, Li Q, Petrick N, Zeng R, Siegelman J. Quantitative assessment of nonsolid pulmonary nodule volume with computed tomography in a phantom study. Quant Imaging Med Surg 2017;7:623-35. [Crossref] [PubMed]
- Fedorov A, Beichel R, Kalpathy-Cramer J, Finet J, Fillion-Robin JC, Pujol S, Bauer C, Jennings D, Fennessy F, Sonka M, Buatti J, Aylward S, Miller JV, Pieper S, Kikinis R. 3D Slicer as an image computing platform for the Quantitative Imaging Network. Magn Reson Imaging 2012;30:1323-41. [Crossref] [PubMed]
- van Griethuysen JJM, Fedorov A, Parmar C, Hosny A, Aucoin N, Narayan V, Beets-Tan RGH, Fillion-Robin JC, Pieper S, Aerts H. Computational Radiomics System to Decode the Radiographic Phenotype. Cancer Res 2017;77:e104-e107. [Crossref] [PubMed]
- Revel MP, Bissery A, Bienvenu M, Aycard L, Lefort C, Frija G. Are two-dimensional CT measurements of small noncalcified pulmonary nodules reliable? Radiology 2004;231:453-8. [Crossref] [PubMed]
- Hiramatsu M, Inagaki T, Inagaki T, Matsui Y, Satoh Y, Okumura S, Ishikawa Y, Miyaoka E, Nakagawa K. Pulmonary ground-glass opacity (GGO) lesions-large size and a history of lung cancer are risk factors for growth. J Thorac Oncol 2008;3:1245-50. [Crossref] [PubMed]
- Kim HS, Lee HJ, Jeon JH, Seong YW, Park IK, Kang CH, Kim KB, Goo JM, Kim YT. Natural history of ground-glass nodules detected on the chest computed tomography scan after major lung resection. Ann Thorac Surg 2013;96:1952-7. [Crossref] [PubMed]
- Kobayashi Y, Fukui T, Ito S, Usami N, Hatooka S, Yatabe Y, Mitsudomi T. How long should small lung lesions of ground-glass opacity be followed? J Thorac Oncol 2013;8:309-14. [Crossref] [PubMed]
- Revel MP, Mannes I, Benzakoun J, Guinet C, Leger T, Grenier P, Lupo A, Fournel L, Chassagnon G, Bommart S. Subsolid Lung Nodule Classification: A CT Criterion for Improving Interobserver Agreement. Radiology 2018;286:316-25. [Crossref] [PubMed]
- Kim H, Park CM, Hwang EJ, Ahn SY, Goo JM. Pulmonary subsolid nodules: value of semi-automatic measurement in diagnostic accuracy, diagnostic reproducibility and nodule classification agreement. Eur Radiol 2018;28:2124-33. [Crossref] [PubMed]
- Chen PA, Huang EP, Shih LY, Tang EK, Chien CC, Wu MT, Wu FZ, Qualitative CT. Criterion for Subsolid Nodule Subclassification: Improving Interobserver Agreement and Pathologic Correlation in the Adenocarcinoma Spectrum. Acad Radiol 2018;25:1439-45. [Crossref] [PubMed]
- Kim H, Park CM, Woo S, Lee SM, Lee HJ, Yoo CG, Goo JM. Pure and part-solid pulmonary ground-glass nodules: measurement variability of volume and mass in nodules with a solid portion less than or equal to 5 mm. Radiology 2013;269:585-93. [Crossref] [PubMed]
- Song YS, Park CM, Park SJ, Lee SM, Jeon YK, Goo JM. Volume and mass doubling times of persistent pulmonary subsolid nodules detected in patients without known malignancy. Radiology 2014;273:276-84. [Crossref] [PubMed]
- Ganeshan B, Goh V, Mandeville HC, Ng QS, Hoskin PJ, Miles KA. Non-small cell lung cancer: histopathologic correlates for texture parameters at CT. Radiology 2013;266:326-36. [Crossref] [PubMed]
- Lim HJ, Ahn S, Lee KS, Han J, Shim YM, Woo S, Kim JH, Yie M, Lee HY, Yi CA. Persistent pure ground-glass opacity lung nodules >/= 10 mm in diameter at CT scan: histopathologic comparisons and prognostic implications. Chest 2013;144:1291-9. [Crossref] [PubMed]
- Bak SH, Lee HY, Kim JH, Um SW, Kwon OJ, Han J, Kim HK, Kim J, Lee KS, Quantitative CT. Scanning Analysis of Pure Ground-Glass Opacity Nodules Predicts Further CT Scanning Change. Chest 2016;149:180-91. [Crossref] [PubMed]
- Tamura M, Shimizu Y, Yamamoto T, Yoshikawa J, Hashizume Y. Predictive value of one-dimensional mean computed tomography value of ground-glass opacity on high-resolution images for the possibility of future change. J Thorac Oncol 2014;9:469-72. [Crossref] [PubMed]
- Eguchi T, Kondo R, Kawakami S, Matsushita M, Yoshizawa A, Hara D, Matsuoka S, Takeda T, Miura K, Agatsuma H, Sakaizawa T, Tominaga Y, Saito G, Toishi M, Hamanaka K, Hashizume M, Shiina T, Amano J, Koizumi T, Yoshida K. Computed tomography attenuation predicts the growth of pure ground-glass nodules. Lung Cancer 2014;84:242-7. [Crossref] [PubMed]