Regional cerebral metabolism alterations affect resting-state functional connectivity in major depressive disorder
Introduction
18F-FDG positron emission tomography (PET) brain scan reflects complex brain glucose consumption. In a resting state (RS), neural activity triggers neuronal oxidative metabolism followed by astrocytic glycolysis. Astrocytes, which account for 20–50% of brain volume, utilize glucose to regulate dynamic neurovascular blood flow (1-3). Neurovascular coupling and the hemodynamic response are the basic principles of functional magnetic resonance imaging (fMRI), thereby fMRI mainly images the neurovascular signaling as a consequence of synaptic activity (4,5). Based on blood oxygen level-dependent (BOLD) signals, RS-functional connectivity (RS-FC) is a frequency-specific relationship between neuronal with a synchronized oscillation across brain spatio-temporal system (6-8), RS-FC mainly manifests as vascular activities and partly represents the neural metabolic process (9). In 1984, Horwitz proposed that in the RS pairs of brain regions whose regional glucose metabolic rates were significantly correlated were functionally associated (10). At present, the founding is encouraging that human brain local neuronal metabolic activity correlated with the RS-FC in default mode network (DMN) and dorsal attention networks has been confirmed by integrated FDG-PET/fMRI research evidence (11,12). And higher glucose metabolism are proportionally associated with a higher degree of connectivity, this synchronicity indicates a neuronal metabolic and functional interaction among the energy-efficient regions (13). Undoubtedly, the energy-efficient regions (densely connected nodes) dysfunction is linked to neuropsychiatric diseases as literature reported (14,15).
Major depressive disorder (MDD) is a brain dysfunction disorder. The hypothesis that MDD etiology comes from an imbalance of neurotransmitters is supported by the effectiveness of antidepressant medications (16-18). 18F-FDG PET is a reliable technique to differentiate regional neural glucose metabolism even with the heterogeneous features of MDD. Much research indicates that cortico-striato-thalamo-cortical circuit have metabolism dysfunction in MDD, and reduced metabolic regions such as the insular, basal ganglia and temporal cortex, can be reversed with treatment (19-21). Converging fMRI evidence also reveals a distributed pattern of abnormal FC in MDD. The orbital frontal cortex (OFC), the precuneus, the superior temporal gyrus (STG) and the visual cortex are important brain network hubs in MDD (22). A RS-fMRI and magnetic resonance spectroscopy (MRS) integrated study proposed that local glutamatergic-creatine metabolism ratio abnormality was an underlying candidate damaging anterior insular FC towards the whole brain and had relevance with HAMD score in MDD (21). A similar result was found in the posterior cingulate cortex (23). A better understanding of the relationship between neuronal metabolic activity and FC patterns may help to reveal the entire brain neural pathway in MDD, and could be a guide for future pharmacological, psychological and brain stimulation therapies (24).
In this study, we designated alternative glucose metabolism regions as the region of interest (ROI) to compare intrinsic RS-FC patterns towards the whole brain between an MDD and healthy control group, proposing a hypothesis that aberrant cerebral metabolism will share its neural activity traits with FC communication in MDD. Then we located nodes of the abnormal RS-FC to network parcellation by independent component analysis (ICA) (25), and analyzed the correlation between depression severity and RS-FC intensity.
Methods
Participants
According to the declaration of Helsinki, this clinical prospective study was approved by the Committee for Medical and Health Research Ethics, Huashan Hospital, Fudan University, Shanghai, China. All participants provided written informed consent before participating in this study. All procedures were conducted in accordance with the institutional regulations and ethical guidelines.
All subjects met the following inclusion criteria: (I) aged from 18 to 50 years; (II) free medication for at least 40 days; (III) have completed at least 6 years of primary education; (IV) able to comply with study procedures. Exclusion criteria were the following: (I) history of head trauma; (II) serious/unstable medical conditions; (III) alcoholism or substance abuse; (IV) neurological disorders such as stroke, seizure and dementia; (V) contraindication to 18F-FDG PET or fMRI scanning; (VI) pregnancy or breast-feeding.
The patients were recruited from the Outpatient Clinic of Psychiatry in Huashan Hospital of Fudan University from 2014 to 2015. At baseline, patients needed to meet the diagnostic criteria for MDD based on DSM-IV-TR and be screened by the Structured Clinical Interview for Axis I DSM-lV-TR Disorders-Patient Edition (SCID-I/P) (26), Chinese Version (27). Patients had a major depressive episode lasting at least 2 weeks and a Hamilton depression rating scale-17 items (HDRS-17) total score of 17 or higher (28). Healthy subjects were recruited from the local community.
PET imaging procedure and data preprocessing
Prior to the injection of 18F-FDG, the blood glucose level of all subjects was in the normal range (4.7–5.5 mmol/L). A 222 to 296 MBq injection of 18F-FDG was administered intravenously under standardized conditions (in a quiet, dimly lit room, with the patient’s eyes open). A 10-min 3-dimensional brain emission scan was acquired at 45-min post injection with a PET scanner (Siemens Biograph 64 HD PET/CT, Siemens, Germany). During the scanning procedure, subjects’ heads were immobilized using a head holder. Attenuation correction was performed using a low-dose CT (150 mAs, 120 kV, Acq. 64×0.6 mm) before the emission scan. Following corrections for scatter, dead time, and random coincidences, PET images were reconstructed by 3-dimensional filtered back projection and a Gaussian filter (full width at half maximum, FWHM 3.5 mm), providing 64 contiguous transaxial slices of 5-mm-thick spacing (29).
Preprocessing of imaging data was performed by SPM5 software (Welcome Department of Imaging Neuroscience, Institute of Neurology, London, UK) implemented in Matlab 7.4.0 (Mathworks Inc., Sherborn, MA, USA). Montreal Neurological Institute brain space with linear and nonlinear 3-D transformations was applied to the spatial normalization of each subject scan. The normalized PET images were then smoothened by a Gaussian filter of 10 mm full width at half maximum over a 3-D space to rise up the signal-to-noise ratio for statistical analysis.
To identify the areas displaying different glucose metabolism, we compared the MDD patients’ PET images with those of the HC subjects with SPM software. We used the “two-sample t-test” routine, which performed simple fixed-effects t test for each voxel. The effect of overall differences in blood flow was removed by using proportional scaling, with the global mean set at 50 and threshold masking set at 0.8. Clusters of at least 100 contiguous voxels, with threshold 2-tailed uncorrected P=0.001, were considered to be significantly different in hypermetabolism or hypometabolism, following a criterion used in several previous studies.
fMRI imaging and data preprocessing
All brain imaging data were obtained by using a 3.0 Tesla MRI scanner (GE Signa GE Healthcare, Waukesha, WI, USA) at Huashan Hospital, Fudan. Participants underwent an imaging protocol including structural imaging and rs-fMRI. The structural T1 weighted images were obtained using three-dimensional (3D) fast spoiled gradient recalled sequence (TR =8.2 ms; TE =3.1 ms; flip angle =8°, matrix =256×256; number of axial slices =176; thickness =1 mm and voxel size = 3×3×3 mm3). RS images were collected by echo planar imaging (EPI) sequence with the following parameters: (TR =2,000 ms; TE =30 ms, flip angle =90°; FOV =220×220 mm2; matrix =64×64; slice thickness =3.2 mm, gap =1 mm, voxel size =1×1×4 mm3, 43 slices per volume). The resting-state fMRI scanning lasted for 400 s and resulted in 200 volumes for each participant.
The fMRI data were preprocessed by a Data Processing Assistant for Resting-State fMRI (DPARSF) tool, including removal of the first 10 time points, slice timing and head motion correction, spatial normalization to a template at the Montreal Neurological Institute space, spatial smoothing with a 6 mm Gaussian kernel, linear detrend removal, nuisance signal regression, and temporal band pass filtering (0.01–0.08 Hz) for the time series of each voxel to reduce the effect of low-frequency drifts and high-frequency noise (30-32). During nuisance signal regression, variables included 24 head-motion parameters, tissue-based average signals from CSF, white matter and ventricles, and multiple linear regression analysis.
ROI
The glucose-based ROI seed was outlined and masking these brain structures with an automated anatomical labeling atlas (AAL) (33) by the MRIcron Soft (http://www.mccauslandcenter.sc.edu/CRNL/).
FC
The time series of all voxels in the ROI were averaged to acquire the seed reference time series. The correlation analysis was carried out between the seed reference and the whole brain in a voxel-wise manner. Individual FC maps were generated for each participant, and the correlation coefficients were transformed to Z values by Fisher Z transformation as a measure of FC (31).
The individual Z value was input to a random effect one-sample t-test in a voxelwise cluster to show significant resting-state connectivity pattern with each seed within each group. Then a random effect two-sample t-test identified the differences in connectivity with seed between two groups. The positive Z value means that the spontaneous signal fluctuations in brain networks were in phase with the fluctuations observed in the corresponding seed region (MDD >HC); whereas negative Z value means that the spontaneous signal fluctuations are antiphase related with the fluctuations observed in the corresponding seed region (MDD<hC). The statistic threshold was set at P<0.005, cluster size >40.
Statistics
Demographic and clinical data were analyzed using SPSS, version 19.0 (SPSS, Inc., Chicago, USA). Significant statistic threshold was set at P<0.05. Person correlation analysis was made between RS-FC Z value and HAMD factors scores in MDD, and the threshold for statistical significance was set at P<0.05.
Results
Demographics and clinical characteristics
A total of 25 right-handed participants enrolled in this study, 11 cases were diagnosed as MDD melancholic subtype, and 14 cases were health subjects (HC). The MDD sample consisted of 8 females (72%) and 3 males (28%), outpatients aged 18–50 years (mean 37.00±6.11 years). The HC sample consisted of 8 females (57%), 6 males (43%), aged 25–48 years (mean 38.38±6.37 years). There were no significant differences in terms of age, gender, and years of education between groups. The patients had an average illness duration of 22.75±15.57 weeks. The baseline HAMD-17 total score (HDRS-T) measure ranged from 17–40, and the average total score was 20.73±4.38. The detailed demographic and clinical profiles are summarized in Table 1.

Full table
Based on factorial analysis found in Bertelli and the Istituto Superiore di Sanità (Italy 1977), and in the HAMD-17, factors included: (I) anxiety/somatization (HDRS-A); (II) body weight (HDRS-B); (III) cognitive disturbances (HDRS-C); (IV) retardation (HDRS-R); (V) sleep disturbances (HDRS-S). In the HDRS-17, anxiety/somatization factor measurement included anxiety (psychic), anxiety (somatic), somatic symptoms (gastrointestinal), hypochondriasis and insight. Retardation factor measurement included depressed mood, work and interests, retardation and genital symptoms. The cognitive disturbances factors included feelings of guilt, suicide and agitation. Pearson correlation was used for clinical HDRS factors, and the MDD patients had a significant correlation coefficient between HDRS-T and HDRS-R (r=0.68, P<0.05), and between HDRS-T and HDRS-C (r=0.71, P<0.05). The correlation coefficient between the HDRS-T and HDRS-A was 0.40, with no statistically significant difference (see Table 2).
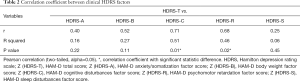
Full table
Brain regions with significant metabolic decrease in MDD patients compared with HC subjects
According to the quantitative result of cerebral clusters with contiguous voxels, the MDD patients had a widespread hypometabolism performance in the right putamen (x =33, y =0, z =0), left claustrum (x =−27, y =−3, z =3), left insular (x =−36, y =−9, z =3), right inferior gyrus (par opercular) (x =51, y =12, z =15) as well as right supramarginal (x =66, y =−27, z =30), compared with the HC group (k >20, uncorrected test, P<0.001) (see Table 3, Figure 1). The relative hypermetabolism had not been observed as the threshold K-value setting larger than 20.
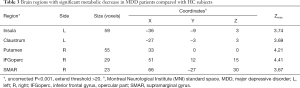
Full table
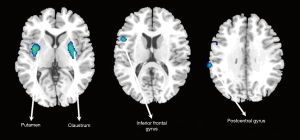
Brain decreased glucose-metabolism seeded RS-FC in MDD patients compared with HC subjects
Taking the five glucose metabolism seeds as ROIs, we made a connectivity map toward the whole brain in each group. Surface based clusterwise correction for multiple comparisons was performed at the threshold setting P value <0.005 and the cluster size threshold of K-value >40. The results are shown in Table 4. MDD patients demonstrated significantly decreased RS-FC between the left claustrum and left calcarine, between the right putamen and right inferior frontal gyrus (pars triangularis), between the left insular and right middle frontal gyrus (MFG), the right inferior frontal gyrus (pars triangularis), and right angular gyrus. The right supramarginal gyrus also had decreased FC to the right MFG, right inferior frontal gyrus (pars triangularis) and left angular gyrus (P<0.005, k>40). No increased connectivity was found.
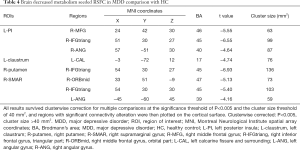
Full table
Derived from a previously reported cortical parcellation method (https://surfer.nmr.mgh.harvard.edu/fswiki/CorticalParcellation_Yeo2011) (25), the linked nodes with reduced connectivity were positioned in functional networks as shown in Table 5 and Figures 2-5. The left claustrum had reduced connectivity with the left calcarine in the primary visual cortex. The right putamen had reduced connectivity with the Inferior frontal gyrus (par triangularis) (x =54, y =30, z =27) in the frontoparietal control network (FPN). The left insular had reduced connectivity with the inferior frontal gyrus (par triangularis) (x =51, y =30, z =27) also in the FPN, with the right MFG (x =24, y =42, z =30) in the insular/dACC network, with the right angular gyrus (x =57, y =−51, z =30) in the language network. The right supramarginal gyrus had reduced connectivity with the inferior frontal gyrus (par triangularis) (x =54, y =30, z =30) and with the left angular (x =−45, y =−60, z =45), both belonging to the frontoparietal control (FPN). Additionally there was reduced connectivity with the right MFG (x =33, y =51, z =−9) in the prefrontal lobe.
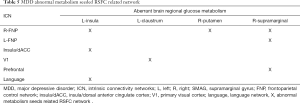
Full table
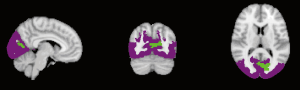
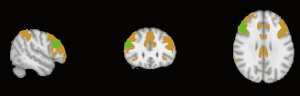
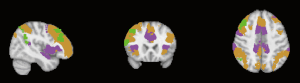
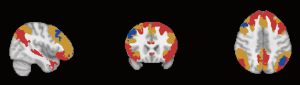
Correlation analysis between metabolism-seeded RS-FC Z value and clinical variables in MDD
We converted the individual clinical variables of MDD patients to Z scores to find out the relationship between the strength of the RS-FC based on metabolism-seeded and symptom severity. The results had no statistical significance to MDD.
Discussion
In the current study, we used a multimode function imaging technique to study the static brain metabolism and RS-FC changes in MDD patients compared with healthy controls. The results revealed that the glucose hypometabolism regions were accompanied with abnormal FC toward the entire brain. The connected nodes with the altered FC were mainly located in the functional networks, such as the primary visual cortex, frontoparietal control network, and language network in MDD patients.
As Parker noted in an earlier study, the HAMD core score defined melancholic features, such as retardation, agitation and non-interactivity (34). In this study, the severity of depressive symptoms was associated with cognitive disturbances and retardation which was identified as special lesion of melancholic depression (35,36). Since the comparison was based on a gender, age matched group (37), the melancholic feature of this MDD sample should be a preferential reference in the following brain metabolic and functional manifestations.
In this study, right-handed patients with MDD had significantly reduced glucose metabolism with the prefrontal-limbic-basal ganglia-parietal circuit, including right Inferior frontal gyrus (pars opercular), left insular, right putamen, left claustrum, and right supramarginal gyrus. The results were mostly consistent with a previous report that found the severity of depression and psychomotor anhedonia were negatively correlated with lower rCMRglu in contiguous regions of insular, claustrum, caudate and putamen, with the exception of the temporal gyrus (38). Our results partially agreed with a meta-analysis showing no finding of brain regions with increased activity, and the disparity may involve MDD subtype classification (19).
In the current study, we found that the abnormal metabolism ROIs had corresponding reduced rs-FC towards the whole brain. Earlier studies reported that in the static aerobic glycolysis, significantly elevated glycolysis were found bilaterally in the prefrontal cortex, lateral parietal cortex, posterior cingulate/precuneus, lateral temporal gyrus, gyrus rectus, caudate nucleus, the DMN. These highly connected hubs, especially in the cortex, had higher rates of cerebral blood flow, aerobic glycolysis and oxidative glucose metabolism, resulting in a stable cortical network architecture (39-41). The higher the effectiveness of metabolism for neural exchange, the greater susceptibility to oxidative stress. Therefore, in this study, the hypometabolism regions with destroyed connectivity indicated possible pathological metabolism hubs in MDD.
In the current study, according to the literature, the hypometabolism region (x =−36, y =−9, z =3) belonged to the left posterior insular (PI) (42). Previous research found that PI-seeded connectivity had correlations with the entire insular and adjacent frontal, temporal, and parietal opercula, same as the supplementary motor area (SMA) and pre-SMA (43), which was consistent with our findings that the left PI has abnormal function connected with the right inferior frontal gyrus (IFG, pars triangular part) within the right DLPFC/parietal lobe (BA 45). PI deactivated to a greater degree in the incongruent stroop condition (44), and the rFIG is likely to switch between distinct brain networks across task paradigms and stimulus modalities (45). Combining the above evidence, left PI having decreased FC with rIFG might weaken MDD patients’ emotional capacity or motivation to carry out attention tasks. Left PI also had reduced function connection within the right MFG, the latter belonged to the insular/dACC network, and Kandilarova et al. found that reduced effective connectivity was from the AI to MFG in the depressive group (46). SN-related dysfunction may promote ruminative thinking and attention biases toward negative events in MDD (47). And melancholia depression was characterized by a pervasive disconnectivity involving AI and SN (48). Our finding adds evidence to the theory that the abnormal neural metabolism in the left PI might weaken attention function in patients with melancholic depression as AI did.
In this study, hypometabolism appeared in the bilateral basal ganglia parts, right putamen and left claustrum. Although the ventral striatum participated in rewards and motivations, the central and dorsal striatum—including the caudate nucleus and the putamen—played more a holistic role in cognition and executive function (49). Retardation was associated with the reduction of dopamine in the caudate and putamen (50-52), which slows down all behaviors of the individual, including exercise, mental activity, and speech. The MDD psychomotor and cognitive retardation may reflect dopamine dysfunction with fronto-striatal-collicular neuronal circuit, in contrast to that the serotonergic system playing a greater role in non-melancholic depression (53). In our study, the right putamen had reduced FC with the right inferior frontal cortex (pars triangularis) (BA 45), the latter belonged to the central control network (CCN), and the subcortical regions, including the putamen, caudate, and claustrum mainly participated in the affective network. Thus our results suggested a subcortical connectivity lesion in MDD allowing the depressive mood to weaken cognitive regulation as was previously reported (54).
The hypometabolism left claustrum had abnormal connectivity to the calcarine sulcus. Calcarine sulcus was a concentration of the primary visual cortex (V1), presumably accounted for by a greater perceptual advantage of emotional stimuli. Coincidently, claustrum played a strong role in controlling attention specifically in cortical regions. Therefore, claustrum neurological abnormality may affect the visual processing, deregulate visual attention and further impair cognitive control in MDD. It had been verified that MDD abnormal effective connectivity across visuo-attentional networks likely impaired attention filtering information (55).
The functional clusters aggregated in the inferior parietal lobule (IPL) exceeded anatomical and cytoarchitectural boundaries which updated the definition of the intraparietal sulcus (lbIPS), angular gyrus (AG), and supramarginal gyrus (SMG). Numerous studies showed that each of these three subdivisions plays an important role in cognitive processes. According to reference MNI coordinates (56), in this study, SMG clusters (x =66, y =−27, z =30) were in the right lateral posteriormiddle IPL. The cytoarchitechtonics and tractography studies revealed that the middle IPL had positive connectivity with the MFG and the inferior frontal gyrus (pars triangular), and may play a specific role each in luminance-based and attention-based motion processing (57,58). The right IPL allocated spatial attention (59,60) and IPL with the parietal cortex and mirror neurons seemed to play a fundamental role in action prediction, planning, observation and execution (61,62). In the current study, the right SMG with two abnormal RS-FC nodes, IFG (pars triangular) and left angular gyrus, both showed a possible substrate weakening of the brain spatial attention allocation and cognition execution in MDD. The posteriormiddle IPL also had a wider connectivity with the orbital part of the MFG (63,64). The medial network in the orbital and medial prefrontal cortex (OMPFC) resembled DMN, which displayed a higher connectivity degree primarily in MDD (65). In fact, IPL is also the real hub junction of DMN, ventromedial prefrontal cortex (vMPFC) and posterior cingulate cortex/Retrosplenial cortex (PCC/Rsp) (63). Fox et al. proposed “anticorrelation” that distinguishes brain function systems showing strong negative correlations with one another (64,66). Therefore our finding that the right SMG had reduced with the medial prefrontal cortex (pars orbital, BA 47) meaning that the external attention system might attenuate in MDD, which was consistent with the evidence mentioned above: when DMN was overactive, the external attention system is attenuated and vice versa (63).
Brain network dysfunction may be caused by aberrant regions (nodes) or connections (edges) that linked them (67), and focal node damage can induce further downstream functional deficits (68). Interestingly, in our study it was the pars triangularis (BA 45) that a central hub node receiving three reduction connection from hypometabolism regions, left PI, right putmamen, right supramarginal. The pars triangularis is located in dorsolateral prefrontal cortex (DLPFC)/parietal lobe network (69), while the hypometabolism region, right inferior frontal gyrus, pars opercular (rIFG, BA 44) belonged to VLPFC. Literature review showed that the DLPFC was closely related to the brain attention and cognition function regions, while the ventral prefrontal cortex was interconnected with brain emotion regions (70,71). As mentioned in the preceding context, rIFG was supposed as a brake to suppress a response or partially pause in different modes to salient signals externally or internally (72-75). Significant self-inhibitory effective connectivity was found from the IFG to anterior cingulate cortex (ACC) and to hippocampus (HPC) (46), meaning the rIFG can be turned on endogenously for self-control rather than externally signaled control. Another study reported the rIFG was important for attentional detection rather than for inhibition (76). The above information gave us a clue as the brain cognition mechanism in compulsive disorders and depressive rumination (77-79). Ruminators had attenuated cortical activity in the right IFG (80). At the same time converged evidence verified that this kind of self-control was not equal to self-referential processing in DMN dominance (81,82). While the lateral parts of cortical midline structures mostly corresponded to the CCN and attention network (83). It was rIFG and DLPFC that had synchronous cognitive control and inhibition together, giving a clear explanation for our results, the IFG subregion, pars triangularis, may be a critical and fragile hub in MDD. There was supporting evidence demonstrating that the rIFG dysfunction mechanism underpining the MDD remitted treatment and mindfulness practices (62). In detail, the aberrant neural activity regions, left PI, right putmamen, right supramarginal, were all dependent on abnormal FC with rIFC (pars triangularis) in the CCN, thus having a significant contribution to deficits of salience attribution, introspective thought, and executive control in melancholic MDD. This hub node metabolism and function profile need to be the focus of future treatments.
In this study, the bilateral angular (inferior parietal cortex) were also critical hub nodes. The hypometabolism right supramarginal had reduced RS-FC with left angular in CCN, while left insular had decreased RS-FC with right angular in the language network. Depending on the literature reported, the right angular was assumed to be compatible with language and attention processing to modulate the perception of affection content for sentences in speech (84), different from that the left angular plays an important cognition role in the integration of linguistic material and relevant aspects in context, crucial for appropriate meanings and speech comprehension ability (85). In our study, we found that the bilateral angular gyrus dysfunction might weaken performance in language, attention processing and cognitive function separately in MDD. And other reports also supposed that the left angular gyrus correlated with part of the CCN probably more prone to cognitive function, is not responsible for somatosensory modality alterations as the right one is (86).
Finally, some studies observed RS-FC disturbances were correlated with depression severity, diagnostic categories, specific depressive symptoms, and treatment response (87,88). However, due to small sample size and other possibilities, this correlation had not been confirmed in this study. We agreed with the comment, Drysdale et al. proposed that not all MDD patients differing clinical-symptom profiles could meet any biotypes (88). Therefore, the correlation of clinical symptoms and functional performance as a pathological reference for MDD should be given with some reservation.
Limitations of this study
First, the sample size was relatively small, which may affect the statistical power. Although the use of a glucose metabolism based seed to avoid ROIs arbitral selection bias, the random error was still inevitably inside. Secondly, we used the RS BOLD signal RS-FC as valuation tool to observe the abnormal neural activity influence on the functional network, the pitiful point is the significant results could not explain the causal relationship among multi-connectivity, deficited a potentially explanation for MDD pathological mechanism. Future functional effectivity causal analysis should be considered. Third, we want to apply an integrated PET/fMRI scanning to verify current results and offset the multimode scanning asynchronization confounding factors.
Conclusions
This study implemented a multimode method to distinguish the FC patterns based on alteration of neural metabolism. The results verified the hypothesis that RS brain regional neural metabolism aberrant almost weakened FC with critical hubs, such as the pars triangularis of inferior frontal gyrus, bilateral angular gyrus, calcarine, MFG, belonging to the CCN (DLPFC/parietal lobe), salience network (SN), primary visual cortex (V1), and language network. We put forward a possibility that focal neural activity alternation was vulnerable to hubs of critical FC which might induce functional network dysynchronization in MDD. The neural spontaneous fluctuation correlation performance had no clinical significance. In addition, the pathology of inferior frontal gyrus (pars triangularis) metabolism and function profiles should be emphasized in future MDD studies.
Acknowledgements
None.
Footnote
Conflicts of Interest: The authors have no conflicts of interest to declare.
Ethical Statement: The study was approved by the Committee for Medical and Health Research Ethics, Huashan Hospital, Fudan University, Shanghai, China and written informed consent was obtained from all patients.
References
- Kasischke KA, Vishwasrao HD, Fisher PJ, Zipfel WR, Webb WW. Neural activity triggers neuronal oxidative metabolism followed by astrocytic glycolysis. Science 2004;305:99-103. [Crossref] [PubMed]
- Sestini S, Castagnoli A, Mansi L. The new FDG brain revolution: the neurovascular unit and the default network. Eur J Nucl Med Mol Imaging 2010;37:913-6. [Crossref] [PubMed]
- Koehler RC, Roman RJ, Harder DR. Astrocytes and the regulation of cerebral blood flow. Trends Neurosci 2009;32:160-9. [Crossref] [PubMed]
- Attwell D, Iadecola C. The neural basis of functional brain imaging signals. Trends Neurosci 2002;25:621-5. [Crossref] [PubMed]
- Wu CW, Gu H, Lu H, Stein EA, Chen JH, Yang Y. Mapping functional connectivity based on synchronized CMRO2 fluctuations during the resting state. Neuroimage 2009;45:694-701. [Crossref] [PubMed]
- Mantini D, Perrucci MG, Del GC, Romani GL, Corbetta M. Electrophysiological signatures of resting state networks in the human brain. Proc Natl Acad Sci U S A 2007;104:13170-5. [Crossref] [PubMed]
- Brookes MJ, Woolrich M, Luckhoo H, Price D, Hale JR, Stephenson MC, Barnes GR, Smith SM, Morris PG. Investigating the electrophysiological basis of resting state networks using magnetoencephalography. Proc Natl Acad Sci U S A 2011;108:16783-8. [Crossref] [PubMed]
- Hipp JF, Hawellek DJ, Corbetta M, Siegel M, Engel AK. Large-scale cortical correlation structure of spontaneous oscillatory activity. Nat Neurosci 2012;15:884-90. [Crossref] [PubMed]
- Fukunaga M, Horovitz SG, de Zwart JA, van Gelderen P, Balkin TJ, Braun AR, Duyn JH. Metabolic origin of BOLD signal fluctuations in the absence of stimuli. J Cereb Blood Flow Metab 2008;28:1377-87. [Crossref] [PubMed]
- Horwitz B, Duara R, Rapoport SI. Intercorrelations of glucose metabolic rates between brain regions: application to healthy males in a state of reduced sensory input. J Cereb Blood Flow Metab 1984;4:484-99. [Crossref] [PubMed]
- Riedl V, Bienkowska K, Strobel C, Tahmasian M, Grimmer T, Forster S, Friston KJ, Sorg C, Drzezga A. Local activity determines functional connectivity in the resting human brain: a simultaneous FDG-PET/fMRI study. J Neurosci 2014;34:6260-6. [Crossref] [PubMed]
- Passow S, Specht K, Adamsen TC, Biermann M, Brekke N, Craven AR, Ersland L, Gruner R, Kleven-Madsen N, Kvernenes OH, Schwarzlmuller T, Olesen RA, Hugdahl K. Default-mode network functional connectivity is closely related to metabolic activity. Hum Brain Mapp 2015;36:2027-38. [Crossref] [PubMed]
- Fox MD, Raichle ME. Spontaneous fluctuations in brain activity observed with functional magnetic resonance imaging. Nat Rev Neurosci 2007;8:700-11. [Crossref] [PubMed]
- Buckner RL, Sepulcre J, Talukdar T, Krienen FM, Liu H, Hedden T, Andrews-Hanna JR, Sperling RA, Johnson KA. Cortical hubs revealed by intrinsic functional connectivity: mapping, assessment of stability, and relation to Alzheimer's disease. J Neurosci 2009;29:1860-73. [Crossref] [PubMed]
- Lan CC, Tsai SJ, Huang CC, Wang YH, Chen TR, Yeh HL, Liu ME, Lin CP, Yang AC. Functional Connectivity Density Mapping of Depressive Symptoms and Loneliness in Non-Demented Elderly Male. Front Aging Neurosci 2016;7:251. [Crossref] [PubMed]
- Kupfer DJ, Frank E, Phillips ML. Major depressive disorder: new clinical, neurobiological, and treatment perspectives. Lancet 2012;379:1045-55. [Crossref] [PubMed]
- Walter M, Henning A, Grimm S, Schulte RF, Beck J, Dydak U, Schnepf B, Boeker H, Boesiger P, Northoff G. The relationship between aberrant neuronal activation in the pregenual anterior cingulate, altered glutamatergic metabolism, and anhedonia in major depression. Arch Gen Psychiatry 2009;66:478-86. [Crossref] [PubMed]
- Yuen EY, Qin L, Wei J, Liu W, Liu A, Yan Z. Synergistic regulation of glutamatergic transmission by serotonin and norepinephrine reuptake inhibitors in prefrontal cortical neurons. J Biol Chem 2014;289:25177-85. [Crossref] [PubMed]
- Su L, Cai Y, Xu Y, Dutt A, Shi S, Bramon E. Cerebral metabolism in major depressive disorder: a voxel-based meta-analysis of positron emission tomography studies. BMC Psychiatry 2014;14:321. [Crossref] [PubMed]
- Sundermann B, Olde LBM, Pfleiderer B. Toward literature-based feature selection for diagnostic classification: a meta-analysis of resting-state fMRI in depression. Front Hum Neurosci 2014;8:692. [Crossref] [PubMed]
- Demenescu LR, Colic L, Li M, Safron A, Biswal B, Metzger CD, Li S, Walter M. A spectroscopic approach toward depression diagnosis: local metabolism meets functional connectivity. Eur Arch Psychiatry Clin Neurosci 2017;267:95-105. [Crossref] [PubMed]
- Zou K, Qing G, Zhiliang L, Fei X, Xiao S, Huafu C, Xueli S. Abnormal functional connectivity density in first-episode, drug-naive adult patients with major depressive disorder. J Affect Disord 2016;194:153-8. [Crossref] [PubMed]
- Lord AR, Li M, Demenescu LR, van den Meer J, Borchardt V, Krause AL, Heinze H-J, Breakspear M, Walter M. Richness in Functional Connectivity Depends on the Neuronal Integrity within the Posterior Cingulate Cortex. Front Neurosci 2017;11:184. [Crossref] [PubMed]
- Mayberg HS. Targeted electrode-based modulation of neural circuits for depression. J Clin Invest 2009;119:717-25. [Crossref] [PubMed]
- Yeo BT, Krienen FM, Sepulcre J, Sabuncu MR, Lashkari D, Hollinshead M, Roffman JL, Smoller JW, Zollei L, Polimeni JR, Fischl B, Liu H, Buckner RL. The organization of the human cerebral cortex estimated by intrinsic functional connectivity. J Neurophysiol 2011;106:1125-65. [Crossref] [PubMed]
- First M, Spitzer R, Gibbon M, Williams J. Structured Clinical Interview for DSM-IV-TR Axis I Disorders, Research Version, Patient Edition (SCID-I/P). New York: Biometrics Research Department, New York State Psychiatric Institute, 2002.
- Phillips MR, Shen Q, Liu X, Pritzker S, Streiner D, Conner K, Yang G. Assessing depressive symptoms in persons who die of suicide in mainland China. J Affect Disord 2007;98:73-82. [Crossref] [PubMed]
- Hamilton M. Development of a rating scale for primary depressive illness. Br J Soc Clin Psychol 1967;6:278-96. [Crossref] [PubMed]
- Wu P, Yu H, Peng S, Dauvilliers Y, Wang J, Ge J, Zhang H, Eidelberg D, Ma Y, Zuo C. Consistent abnormalities in metabolic network activity in idiopathic rapid eye movement sleep behaviour disorder. Brain 2014;137:3122-8. [Crossref] [PubMed]
- Greicius MD, Krasnow B, Reiss AL, Menon V. Functional connectivity in the resting brain: a network analysis of the default mode hypothesis. Proc Natl Acad Sci U S A 2003;100:253-8. [Crossref] [PubMed]
- Lowe MJ, Mock BJ, Sorenson JA. Functional connectivity in single and multislice echoplanar imaging using resting-state fluctuations. Neuroimage 1998;7:119-32. [Crossref] [PubMed]
- Biswal B, Yetkin FZ, Haughton VM, Hyde JS. Functional connectivity in the motor cortex of resting human brain using echo-planar MRI. Magn Reson Med 1995;34:537-41. [Crossref] [PubMed]
- Tzourio-Mazoyer N, Landeau B, Papathanassiou D, Crivello F, Etard O, Delcroix N, Mazoyer B, Joliot M. Automated anatomical labeling of activations in SPM using a macroscopic anatomical parcellation of the MNI MRI single-subject brain. Neuroimage 2002;15:273-89. [Crossref] [PubMed]
- Parker G. Defining melancholia: the primacy of psychomotor disturbance. Acta Psychiatr Scand Suppl 2007.21-30. [Crossref] [PubMed]
- Quinn C, Harris A, Kemp A. The interdependence of subtype and severity: contributions of clinical and neuropsychological features to melancholia and non-melancholia in an outpatient sample. J Int Neuropsychol Soc 2012;18:361-9. [Crossref] [PubMed]
- Rush AJ. The varied clinical presentations of major depressive disorder. J Clin Psychiatry 2007;68 Suppl 8:4-10. [PubMed]
- Monzón S, Gili M, Vives M, Serrano MJ, Bauza N, Molina R, Garcia-Toro M, Salva J, Llobera J, Roca M. Melancholic versus non-melancholic depression: differences on cognitive function. A longitudinal study protocol. BMC Psychiatry 2010;10:48. [Crossref] [PubMed]
- Bench CJ, Friston KJ, Brown RG, Frackowiak RS, Dolan RJ. Regional cerebral blood flow in depression measured by positron emission tomography: the relationship with clinical dimensions. Psychol Med 1993;23:579-90. [Crossref] [PubMed]
- Vaishnavi SN, Vlassenko AG, Rundle MM, Snyder AZ, Mintun MA, Raichle ME. Regional aerobic glycolysis in the human brain. Proc Natl Acad Sci U S A 2010;107:17757-62. [Crossref] [PubMed]
- Tomasi D, Wang GJ, Volkow ND. Energetic cost of brain functional connectivity. Proc Natl Acad Sci U S A 2013;110:13642-7. [Crossref] [PubMed]
- Liang X, Zou Q, He Y, Yang Y. Coupling of functional connectivity and regional cerebral blood flow reveals a physiological basis for network hubs of the human brain. Proc Natl Acad Sci U S A 2013;110:1929-34. [Crossref] [PubMed]
- Peng X, Lin P, Wu X, Gong R, Yang R, Wang J. Insular subdivisions functional connectivity dysfunction within major depressive disorder. J Affect Disord 2018;227:280-8. [Crossref] [PubMed]
- Deen B, Pitskel NB, Pelphrey KA. Three systems of insular functional connectivity identified with cluster analysis. Cereb Cortex 2011;21:1498-506. [Crossref] [PubMed]
- Harrison BJ, Shaw M, Yucel M, Purcell R, Brewer WJ, Strother SC, Egan GF, Olver JS, Nathan PJ, Pantelis C. Functional connectivity during Stroop task performance. Neuroimage 2005;24:181-91. [Crossref] [PubMed]
- Sridharan D, Levitin DJ, Menon V. A critical role for the right fronto-insular cortex in switching between central-executive and default-mode networks. Proc Natl Acad Sci U S A 2008;105:12569-74. [Crossref] [PubMed]
- Kandilarova S, Stoyanov D, Kostianev S, Specht K. Altered Resting State Effective Connectivity of Anterior Insula in Depression. Front Psychiatry 2018;9:83. [Crossref] [PubMed]
- Wagner G, de la Cruz F, Kohler S, Bar KJ. Treatment Associated Changes of Functional Connectivity of Midbrain/Brainstem Nuclei in Major Depressive Disorder. Sci Rep 2017;7:8675. [Crossref] [PubMed]
- Hyett MP, Breakspear MJ, Friston KJ, Guo CC, Parker GB. Disrupted effective connectivity of cortical systems supporting attention and interoception in melancholia. JAMA Psychiatry 2015;72:350-8. [Crossref] [PubMed]
- Peters SK, Dunlop K, Downar J. Cortico-Striatal-Thalamic Loop Circuits of the Salience Network: A Central Pathway in Psychiatric Disease and Treatment. Front Syst Neurosci 2016;10:104. [Crossref] [PubMed]
- Shah PJ, Ogilvie AD, Goodwin GM, Ebmeier KP. Clinical and psychometric correlates of dopamine D2 binding in depression. Psychol Med 1997;27:1247-56. [Crossref] [PubMed]
- Austin MP, Mitchell P, Hadzi-Pavlovic D, Hickie I, Parker G, Chan J, Eyers K. Effect of apomorphine on motor and cognitive function in melancholic patients: a preliminary report. Psychiatry Res 2000;97:207-15. [Crossref] [PubMed]
- Meyer JH, McNeely HE, Sagrati S, Boovariwala A, Martin K, Verhoeff NP, Wilson AA, Houle S. Elevated putamen D(2) receptor binding potential in major depression with motor retardation: an [11C]raclopride positron emission tomography study. Am J Psychiatry 2006;163:1594-602. [Crossref] [PubMed]
- Winograd-Gurvich C, Georgiou-Karistianis N, Fitzgerald PB, Millist L, White OB. Ocular motor differences between melancholic and non-melancholic depression. J Affect Disord 2006;93:193-203. [Crossref] [PubMed]
- Amedi A, Malach R, Pascual-Leone A. Negative BOLD differentiates visual imagery and perception. Neuron 2005;48:859-72. [Crossref] [PubMed]
- Desseilles M, Schwartz S, Dang-Vu TT, Sterpenich V, Ansseau M, Maquet P, Phillips C. Depression alters "top-down" visual attention: a dynamic causal modeling comparison between depressed and healthy subjects. Neuroimage 2011;54:1662-8. [Crossref] [PubMed]
- Dehaene S, Molko N, Cohen L, Wilson AJ. Arithmetic and the brain. Curr Opin Neurobiol 2004;14:218-24. [Crossref] [PubMed]
- Claeys KG, Lindsey DT, De Schutter E, Orban GA. A higher order motion region in human inferior parietal lobule: evidence from fMRI. Neuron 2003;40:631-42. [Crossref] [PubMed]
- Zhang S, Li CS. Functional clustering of the human inferior parietal lobule by whole-brain connectivity mapping of resting-state functional magnetic resonance imaging signals. Brain Connect 2014;4:53-69. [PubMed]
- Ciçek M, Gitelman D, Hurley RS, Nobre A, Mesulam M. Anatomical physiology of spatial extinction. Cereb Cortex 2007;17:2892-8. [Crossref] [PubMed]
- Singh-Curry V, Husain M. The functional role of the inferior parietal lobe in the dorsal and ventral stream dichotomy. Neuropsychologia 2009;47:1434-48. [Crossref] [PubMed]
- Stevens MC, Calhoun VD, Kiehl KA. Hemispheric differences in hemodynamics elicited by auditory oddball stimuli. Neuroimage 2005;26:782-92. [Crossref] [PubMed]
- Peters AT, Burkhouse K, Feldhaus CC, Langenecker SA, Jacobs RH. Aberrant resting-state functional connectivity in limbic and cognitive control networks relates to depressive rumination and mindfulness: A pilot study among adolescents with a history of depression. J Affect Disord 2016;200:178-81. [Crossref] [PubMed]
- Buckner RL, Andrews-Hanna JR, Schacter DL. The brain's default network: anatomy, function, and relevance to disease. Ann N Y Acad Sci 2008;1124:1-38. [Crossref] [PubMed]
- Fox MD, Snyder AZ, Vincent JL, Corbetta M, Van Essen DC, Raichle ME. The human brain is intrinsically organized into dynamic, anticorrelated functional networks. Proc Natl Acad Sci U S A 2005;102:9673-8. [Crossref] [PubMed]
- Raichle ME, MacLeod AM, Snyder AZ, Powers WJ, Gusnard DA, Shulman GL. A default mode of brain function. Proc Natl Acad Sci U S A 2001;98:676-82. [Crossref] [PubMed]
- Gong Q, He Y. Depression, neuroimaging and connectomics: a selective overview. Biol Psychiatry 2015;77:223-35. [Crossref] [PubMed]
- Sporns O. Networks of the Brain. MIT Press, 2011.
- Crofts JJ, Higham DJ, Bosnell R, Jbabdi S, Matthews PM, Behrens TE, Johansen-Berg H. Network analysis detects changes in the contralesional hemisphere following stroke. Neuroimage 2011;54:161-9. [Crossref] [PubMed]
- Seeley WW, Menon V, Schatzberg AF, Keller J, Glover GH, Kenna H, Reiss AL, Greicius MD. Dissociable intrinsic connectivity networks for salience processing and executive control. J Neurosci 2007;27:2349-56. [Crossref] [PubMed]
- Price JL. Prefrontal cortical networks related to visceral function and mood. Ann N Y Acad Sci 1999;877:383-96. [Crossref] [PubMed]
- Smith JM, Alloy LB. A roadmap to rumination: a review of the definition, assessment, and conceptualization of this multifaceted construct. Clin Psychol Rev 2009;29:116-28. [Crossref] [PubMed]
- Garavan H, Ross TJ, Stein EA. Right hemispheric dominance of inhibitory control: an event-related functional MRI study. Proc Natl Acad Sci U S A 1999;96:8301-6. [Crossref] [PubMed]
- Konishi S, Nakajima K, Uchida I, Sekihara K, Miyashita Y. No-go dominant brain activity in human inferior prefrontal cortex revealed by functional magnetic resonance imaging. Eur J Neurosci 1998;10:1209-13. [Crossref] [PubMed]
- Aron AR, Robbins TW, Poldrack RA. Inhibition and the right inferior frontal cortex. Trends Cogn Sci 2004;8:170-7. [Crossref] [PubMed]
- Rubia K, Smith AB, Brammer MJ, Taylor E. Right inferior prefrontal cortex mediates response inhibition while mesial prefrontal cortex is responsible for error detection. Neuroimage 2003;20:351-8. [Crossref] [PubMed]
- Sharp DJ, Bonnelle V, De Boissezon X, Beckmann CF, James SG, Patel MC, Mehta MA. Distinct frontal systems for response inhibition, attentional capture, and error processing. Proc Natl Acad Sci U S A 2010;107:6106-11. [Crossref] [PubMed]
- Brass M, Haggard P. To do or not to do: the neural signature of self-control. J Neurosci 2007;27:9141-5. [Crossref] [PubMed]
- Berman MG, Nee DE, Casement M, Kim HS, Deldin P, Kross E, Gonzalez R, Demiralp E, Gotlib IH, Hamilton P, Joormann J, Waugh C, Jonides J. Neural and behavioral effects of interference resolution in depression and rumination. Cogn Affect Behav Neurosci 2011;11:85-96. [Crossref] [PubMed]
- Kühn S, Vanderhasselt MA, De Raedt R, Gallinat J. Why ruminators won't stop: the structural and resting state correlates of rumination and its relation to depression. J Affect Disord 2012;141:352-60. [Crossref] [PubMed]
- Rosenbaum D, Thomas M, Hilsendegen P, Metzger FG, Haeussinger FB, Nuerk HC, Fallgatter AJ, Nieratschker V, Ehlis AC. Stress-related dysfunction of the right inferior frontal cortex in high ruminators: An fNIRS study. Neuroimage Clin 2018;18:510-7. [Crossref] [PubMed]
- Conway MA, Fthenaki A. Disruption of inhibitory control of memory following lesions to the frontal and temporal lobes. Cortex 2003;39:667-86. [Crossref] [PubMed]
- Zhong X, Pu W, Yao S. Functional alterations of fronto-limbic circuit and default mode network systems in first-episode, drug-naive patients with major depressive disorder: A meta-analysis of resting-state fMRI data. J Affect Disord 2016;206:280-6. [Crossref] [PubMed]
- Nejad AB, Fossati P, Lemogne C. Self-referential processing, rumination, and cortical midline structures in major depression. Front Hum Neurosci 2013;7:666. [Crossref] [PubMed]
- Hervé P-Y, Razafimandimby A, Vigneau M, Mazoyer B, Tzourio-Mazoyer N. Disentangling the brain networks supporting affective speech comprehension. Neuroimage 2012;61:1255-67. [Crossref] [PubMed]
- Bambini V, Gentili C, Ricciardi E, Bertinetto PM, Pietrini P. Decomposing metaphor processing at the cognitive and neural level through functional magnetic resonance imaging. Brain Res Bull 2011;86:203-16. [Crossref] [PubMed]
- Lai CH, Wu YT, Hou YM. Functional network-based statistics in depression: Theory of mind subnetwork and importance of parietal region. J Affect Disord 2017;217:132-7. [Crossref] [PubMed]
- Brakowski J, Spinelli S, Dorig N, Bosch OG, Manoliu A, Holtforth MG, Seifritz E. Resting state brain network function in major depression - Depression symptomatology, antidepressant treatment effects, future research. J Psychiatr Res 2017;92:147-59. [Crossref] [PubMed]
- Drysdale AT, Grosenick L, Downar J, Dunlop K, Mansouri F, Meng Y, Fetcho RN, Zebley B, Oathes DJ, Etkin A, Schatzberg AF, Sudheimer K, Keller J, Mayberg HS, Gunning FM, Alexopoulos GS, Fox MD, Pascual-Leone A, Voss HU, Casey BJ, Dubin MJ, Liston C. Resting-state connectivity biomarkers define neurophysiological subtypes of depression. Nat Med 2017;23:28-38. [Crossref] [PubMed]