Quantitative parameters of intravoxel incoherent motion diffusion weighted imaging (IVIM-DWI): potential application in predicting pathological grades of pancreatic ductal adenocarcinoma
Introduction
Pancreatic ductal adenocarcinoma (PDAC) is one of the leading death causes of cancer worldwide (1). Surgical eradication is the only curative therapy for PDAC. However, most PDAC patients have no chance to receive surgery due to metastasis or local invasion at the diagnosis (2). Therefore, a non-invasive detection and diagnosis method at the early stage of PDAC is needed to improve efficacy and prognosis and to fill in the blank in this area (3,4).
A meta-analysis about imaging tests for staging of PDAC showed that endoscopic ultrasound with fine-needle aspiration (EUS-FNA) was more accurate than CT in T staging and similar in accuracy to CT in assessing resectability (5). However, another meta-analysis showed EUS-FNA had the most frequent rate of adverse reaction such as pancreatitis, a procedural pain, bleeding and perforation compared with other imaging modalities (6). A study has shown that perfusion CT can predict tumor grade of PDAC (7). However, excess exposure to radiation limits its application in evaluating PDAC.
Apparent diffusion coefficient (ADC) derived from diffusion weighted imaging (DWI) can be used to measure the magnitude of hindered water diffusion in tissues. It can be applied to assess tissue microstructure at the early stage of diseases. DWI has been increasingly applied in differentiating benign lesions from malignant ones in the abdomen as MR technology advances. Recently, DWI has been extensively used to detect and characterize pancreatic lesions (2,8-21). However, conflicting results have been reported for ADC values in characterizing pancreatic cancer (PC). Yoshikawa et al. (22) reported that PC had a higher ADC value than normal pancreas while some studies showed no significant difference in ADC values between PC and normal pancreatic parenchyma (8,17-19). However, more studies have shown that ADC values of PC are much lower than those of normal pancreas (13-16,20).
The ADC values can reflect Brownian motion of water protons not only in tissue extracellular and intercellular space but also in tissue microcirculation (perfusion effects) (23-25). Perfusion effects may affect the reliability of ADC in characterizing tissue microstructure (26). Based on the intravoxel incoherent motion (IVIM) model, DWI with sufficient b values by using the biexponential analysis enables to separately reflect pure tissue diffusivity and information about microcapillary perfusion (10,23-27). Intravoxel incoherent motion diffusion weighted imaging (IVIM-DWI) can simultaneously provide information about tumor perfusion and diffusion characteristics without using contrast medium, which is meaningful to patients with renal insufficiency (28). Although quantitative parameters derived from IVIM-DWI have been increasingly used to diagnose and differentiate pancreatic lesions recently (10,11,14,18), there has been no report applying them in classifying PDAC into different pathological grades.
Therefore, our study aimed to compare IVIM-DWI derived parameters such as standard ADC (ADCstandard), pure diffusion coefficient (Dslow), pseudodiffusion coefficient (Dfast) and perfusion fraction (ƒ) of PDAC with different pathological grades.
Methods
Patients
The study protocol was granted by the Institutional Ethical Review Board of our hospital with a waiver of informed consent. From May 2014 to August 2017, 108 patients with a suspect focal solid pancreatic mass seen in CT examination were referred for inclusion in this study. Eligibility inclusion criteria included: histologically confirmed diagnosis of PDAC by surgery or aspiration biopsy; PDAC with clear pathological grade; without chemotherapy or radiotherapy before MRI examination; and no contraindications to MRI examination. Finally, among 108 patients, 70 were excluded due to various reasons. The case accrual process was summarized in Figure 1. Finally, 38 patients (25 men and 13 women, age range: 20–73 years, mean age: 53.2±14.2 years) with histopathologically confirmed PDAC were enrolled in this study. Of these, 25 patients were proven to be well/moderately differentiated PDAC, and 13 to be poorly differentiated PDAC. All patients in the final cohort underwent routine pancreatic MR imaging and multiple b values DW imaging within 1 week before surgery.
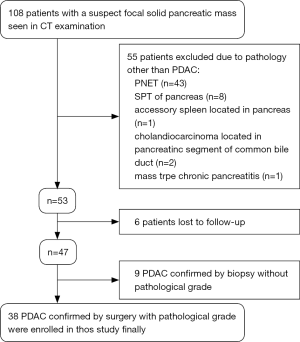
MRI protocols
All MR examinations were performed at a whole-body 3.0T MR scanner (Discovery MR750, GE Medical Systems, Milwaukee, Wis., USA) with a 32-channel body array coil. Routine pancreatic MR imaging protocols included axial respiratory triggering fat-suppressed FSE T2WI and 3D Liver Acquisition with Volume Acceleration Flex (LAVA Flex) sequence with breath-hold. Pancreatic axial respiratory triggering DW imaging was employed with 15 b values of 0, 10, 20, 40, 60, 80, 100, 150, 200, 400, 800, 1,000, 1,200, 1,500, and 2,000 s/mm2. The detailed parameters for each sequence were summarized in Table 1. No adverse events occurred during MR examinations.
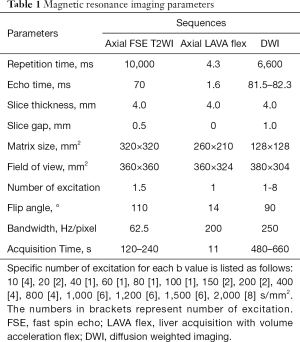
Full table
Data analysis
All DWI data were sent to an AW 4.6 workstation provided by the manufacturer (GE Healthcare, Milwaukee, WI) after the examination. All functional parameters maps were post-processed using the MADC programs on AW 4.6 workstation.
Standard ADC value was calculated by mono-exponential model using the total available b values according to the following equation:
S(b)/S0=exp(-b·ADC) [1]
The IVIM parameters were calculated by bi-exponential fitting according to the following equation, suggested by Le Bihan et al. (24):
S(b)/S0=ƒ·exp(-b·Dfast)+(1-ƒ)·exp(-b·Dslow) [2]
Where S(b) corresponds to mean signal intensity on DWI with a certain b value. S0 is the mean signal intensity on DWI with b value=0. Dslow represents pure molecular diffusivity where a physiological perfusion effect is excluded. Dfast represents the average blood velocity and mean capillary segment length. ƒ represents the ratio of water movement within capillaries compared with the total volume of water in a voxel. Because Dfast is roughly one order of magnitude greater than Dslow (29), −b·Dfast would be less than −3 at a high b value (>200 s/mm2), and the term ƒ·exp(-b·Dfast) would be less than 0.05ƒ and can therefore be neglected. In this case, Eq. [2] can be simplified as follows:
S(b)/S0=(1-ƒ)·exp(-b·Dslow) [3]
Hence, for high b values (>200 s/mm2) S(b) was first fitted to Eq. [3] using a linear model, and then pure diffusion coefficient Dslow was calculated. Although we had previously calculated the ƒ value using Eq. [3], its accuracy was not acceptable. Therefore, ƒ was recalculated using Eq. [2]. Then, we fitted S(b) for all b values using Eq. [2] with a fixed Dslow value using the nonlinear Levenberg-Marquardt method. When fitting Eq. [2], the initial estimated values for ƒ and Dfast were set as the previously calculated ƒ value from Eq. [3] and 10×10−3 mm2/s, respectively. Subsequently, the ƒ and Dfast were obtained.
All measurements were performed by experienced radiologists with 11 years of experience in abdominal MRI, who were unaware of the histopathologic results. On DW images with b value which showed tumor most clearly among all DW images (Figure 2), the largest possible irregular regions of interest (ROIs) for each PDAC were manually drawn along the margin of tumor on three of the largest consecutive lesion slices. More attention was paid to exclude vascular, pancreatic duct, necrosis area within the lesion in process of measurement. Well-matched copies of the ROI were automatically and synchronously generated and appeared on each functional map of ADCstandard and IVIM-DWI parameters on corresponding locations by using built-in software (MADC programs on AW 4.6 workstation). The functional parameter maps and ROI setting were shown in Figure 3A,B,C,D,E. The average value of the results of three measurements was used as the final result. ROI areas of PDAC ranged from 136 mm2 (long diameter × short diameter: 20.5 mm × 14.7 mm) to 895 mm2 (long diameter × short diameter: 46.7 mm × 34.2 mm) with mean areas of 444.75 mm2 (long diameter × short diameter: 33.9 mm × 25.3 mm).
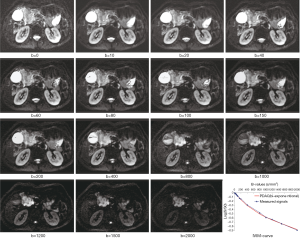
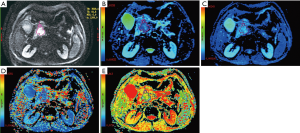
Statistical analysis
All statistical analyses were performed using SPSS Statistics (version 17.0, SPSS Inc., Chicago, IL) and MedCalc (version 12.3, MedCalc Software, Mariakerke, Belgium). Parameter values were recorded as means ± standard deviations. Cohen’s Kappa statistics (κ) was used to evaluate the inter-rater agreement between the two observers for ADC and IVIM-DWI parameters’ measurements. The Independent Sample t Test was used for the comparison of ADCstandard, Dslow, Dfast and ƒ values between well/moderately differentiated PDAC and poorly differentiated PDAC. The diagnostic performances of IVIM-DWI derived parameters were evaluated using receiver operating characteristics (ROC) analysis. The areas under curve (AUC) values were compared, and the cut-off values with the largest Youden index (the sum of sensitivity and specificity) were calculated from the ROC-curves. The P values less than 0.05 were considered statistically significant.
Results
Interobserver agreement in imaging analysis
The measurements of IVIM-DWI parameters and ADC values had the excellent interobserver reproducibility. The interobserver agreement showed the κ value of 0.904 [95% confidence interval (CI), 0.862–0.947] in ADC values. In addition, the agreement between two observers was obtained in Dslow, Dfast, and ƒ values’ measurements with κ values of 0.917 (95% CI, 0.885–0.949), 0.818 (95% CI, 0.706–0.930), and 0.889 (95% CI, 0.840–0.939), respectively.
Quantitative analysis of IVIM-DWI parameters
Mean Dslow value of well/moderately differentiated PDAC was significantly lower than that of poorly differentiated PDAC (0.540×10−3vs. 0.676×10−3 mm2/s, t=5.635, P<0.001). Mean ƒ value of well/moderately differentiated PDAC was significantly higher than that of poorly differentiated PDAC (60.3% vs. 38.4%, t=5.371, P<0.001). There were no significant differences for ADCstandard and Dfast between well/moderately differentiated and poorly differentiated PDAC (0.853×10−3vs. 0.826×10−3 mm2/s, t=0.670, P=0.507; and 3.448×10−3vs. 4.225×10−3 mm2/s, t=0.917, P=0.365; respectively). Detailed results were shown in Table 2. The box and whisker plots (Figure 4) compared ADCstandard and IVIM-DWI parameters between well/moderately differentiated and poorly differentiated PDAC.
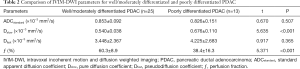
Full table
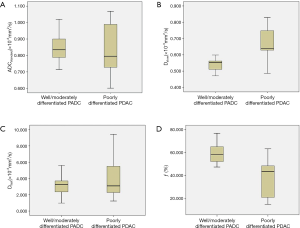
ROC analysis of IVIM-DWI parameters
AUC value of ƒ was slightly higher than that of Dslow (0.894 vs. 0.865). When Dslow value was less than or equal to 0.599×10−3 mm2/s, the sensitivity and specificity were 100% and 84.6% respectively for differentiating well/moderately differentiated PDAC from poorly differentiated PDAC. When ƒ value was greater than 49.6%, the sensitivity and specificity were 92.0% and 84.6% respectively for differentiating well/moderately differentiated and poorly differentiated PDAC. ROC curve map was shown in Figure 5. The detailed results were listed in Table 3.
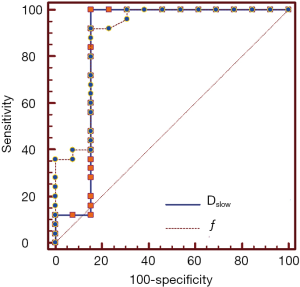
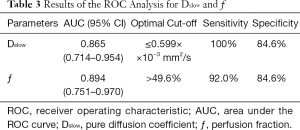
Full table
Discussion
This study showed significant statistical difference in Dslow and no significant difference in ADCstandard between well/moderately differentiated and poorly differentiated PDAC. The calculation of ADC value usually uses the mono-exponential diffusion model which obeys the Gaussian distribution based on water molecular Brownian motion in vivo. However, water molecular diffusion in vivo biologic tissues is much more sophisticated and always deviates from Gaussian law (26). ADC value can be influenced not only by water molecular diffusion in tissue microstructure but also in tissue microcirculation (24,30). Therefore, ADC value is no longer the true diffusion coefficient and cannot reflect sophisticated true diffusion features of water molecular in vivo tissues (26). Since the ADCstandard value in our study was calculated based on conventional mono-exponential diffusion model, it might not reflect true diffusion features of water molecular in vivo.
Quantitative parameters derived from IVIM-DWI can separately reflect tissue diffusivity and tissue microcapillary perfusion (10,23-27). Pure diffusion coefficient (Dslow) derived from IVIM-DWI with more and greater b values can better eliminate the perfusion-related diffusion (31). It can better reflect the obstacle of free diffusion of intracellular and extracellular water molecules due to barriers such as membranes, macromolecules, and fibers (23,24,32). Quantitative parameters derived from IVIM-DWI with the higher b values can better reflect the characteristics of the non-Gauss diffusion of water molecules in the tissues (33). An increased number of b values can decrease the CoV (coefficient of variation) of Dslow and increase measurement accuracy (34). DWI in our study was performed with 7 high b values (≥200 s/mm2) and highest b value up to 2,000 s/mm2. Dslow in our study might be closest to the true diffusion characteristics of water molecules in biological tissues. Glandular formation is the crucial morphological characteristic for grading differentiation of PDAC (35). Well/moderately differentiated PDAC shows abundant fibrosis and glandular formation characterized by neoplastic tubular and duct-like structures (35-37). These tubular and duct-like structures contain massive mucus rich in macromolecular protein, which may account for restrained diffusion of water molecules in well/moderately differentiated PDAC. Abundant fibrosis within well/moderately differentiated PDAC can account for more restricted diffusivity of water molecules in vivo (8). In contrast, poorly differentiated PDAC characteristically shows limited to no glandular formation and mucus (35). The variability of fibrosis content in poorly differentiated PDAC may have no significant influence on ADC values compared with well/moderately differentiated PDAC (8). This may explain the significant difference in Dslow value between poorly and well/moderately differentiated PDAC observed in our study.
IVIM-DWI with more b values in segment of low b values can get more accurate perfusion-related diffusion (31). DWI in our study was performed with 8 low b values (<200 s/mm2). The perfusion fraction ƒ in our study might better demonstrate perfusion characteristics of PDAC. IVIM-DWI can better demonstrate tumor perfusion characteristics without contrast enhancement (10,23-27). It may be a recommendable substitute for perfusion MR imaging for its risk-free of nephrogenic systemic fibrosis caused by contrast agents and thus is especially suited for patients with contraindications to contrast agents or patients with renal dysfunction (26,28). The perfusion fraction ƒ derived from IVIM-DWI describes the volume fraction of intravoxel incoherent signals which comes from the vascular component (23-27,38). The ƒ derived from IVIM-DWI showed significant positive correlation with MVD (28). One study demonstrated that well-differentiated PDAC contained higher MVD than poorly differentiated PDAC (39). This could explain that ƒ value of well/moderately differentiated PDAC was significantly higher than poorly differentiated PDAC in our study. Due to the high variance and standard deviation (S.D.) in the quantitative analysis (31), no significant difference in Dfast was observed between well/moderately differentiated and poorly differentiated PDAC in our study.
The IVIM-DWI derived ƒ value showed higher diagnostic performance and closely followed by Dslow in differentiating well/moderately differentiated and poorly differentiated PDAC. Wang et al. (40) reported that among the three parameters, ƒ derived from IVIM-DWI offered the best diagnostic value, followed by Dslow, in evaluating liver fibrosis. Both studies showed IVIM technique to be used in the evaluation of abdominal organs. When Dslow value was less than or equal to 0.599×10−3 mm2/s and ƒ value was greater than 49.6%, both sensitivities were greater than 90% and specificities were 84.6% in differentiating well/moderately differentiated and poorly differentiated PDAC.
Tumor grade of PDAC is a significant prognostic indicator after resection (41,42). High tumor grade has a larger impact on survival than tumor size and lymph node metastases (43). Therefore, a non-invasive imaging method for predicting pathological grade of PDAC before surgery would be helpful to identify the aggressive prognosis of poorly differentiated tumors. This may allow clinicians to optimize therapeutic strategy and further improve prognosis. Our study has shown that Dslow and ƒ can distinguish well/moderately differentiated and poorly differentiated PDAC. IVIM-DWI can be an important tool for predicting pathological grade of PDAC before surgery.
There were several limitations in our study. First, our study population included only a few poorly differentiated PDAC. However, all patients underwent surgery and acquired histopathological grade in our study. Second, only a respiratory-triggered technique was used, but no pulse or cardiac triggering was performed. This might lead to heterogeneous perfusion parameters maps due to pulsating motion influences caused by large vessels and the high S.D. in the quantitative analysis, which may limit the measurement reproducibility. Third, the influences of fibrosis on IVIM-DWI parameters were not taken into account, which have to be evaluated in further studies. Finally, the b-factor applied in this study may not be optimal. Signal-to-noise ratio (SNR) would decrease with higher b values than 1,000 s/mm2. Low SNR of image with higher b-value would affect the accuracy of measurement. DWI with more and higher b values would increase sampling time. This needs to be further optimized in order to balance parameter estimation reliability with minimum sampling time.
In conclusion, both IVIM-DWI derived parameters Dslow and ƒ have high diagnostic performance in distinguishing well/moderately differentiated PDAC from poorly differentiated PDAC. IVIM-DWI may be a promising non-invasive tool for predicting pathological grade of PDAC. Perfusion related parameter ƒ derived from IVIM-DWI can be used as an early biomarker in evaluating tumor vascularity of PDAC without use of contrast agents.
Acknowledgements
We thank Xiaocheng Wei (senior engineer, MR Research China, GE healthcare Greater China, Beijing, P. R. China) for designing the MRI scanning protocols and specifying DWI data fitting method used in our study.
Funding: The authors express sincerest gratitude to National Natural Science Foundation of China (Grants NSFC 81220108011, and NSFC 81370039) for grant support.
Footnote
Conflicts of Interest: The authors have no conflicts of interest to declare.
Ethical Statement: Institutional Review Board of our hospital approved this study protocol with a waiver of informed consent.
References
- Jemal A, Siegel R, Ward E, Hao Y, Xu J, Thun MJ. Cancer statistics, 2009. CA Cancer J Clin 2009;59:225-49. [Crossref] [PubMed]
- De Robertis R, Tinazzi Martini P, Demozzi E, Dal Corso F, Bassi C, Pederzoli P, D'Onofrio M. Diffusion-weighted imaging of pancreatic cancer. World J Radiol 2015;7:319-28. [Crossref] [PubMed]
- Gemmel C, Eickhoff A, Helmstadter L, Riemann JF. Pancreatic cancer screening: state of the art. Expert Rev Gastroenterol Hepatol 2009;3:89-96. [Crossref] [PubMed]
- Klapman J, Malafa MP. Early detection of pancreatic cancer: why, who, and how to screen. Cancer Control 2008;15:280-7. [Crossref] [PubMed]
- Treadwell JR, Zafar HM, Mitchell MD, Tipton K, Teitelbaum U, Jue J. Imaging Tests for the Diagnosis and Staging of Pancreatic Adenocarcinoma: A Meta-Analysis. Pancreas 2016;45:789-95. [Crossref] [PubMed]
- Toft J, Hadden WJ, Laurence JM, Lam V, Yuen L, Janssen A, Pleass H. Imaging modalities in the diagnosis of pancreatic adenocarcinoma: A systematic review and meta-analysis of sensitivity, specificity and diagnostic accuracy. Eur J Radiol 2017;92:17-23. [Crossref] [PubMed]
- D'Onofrio M, Gallotti A, Mantovani W, Crosara S, Manfrin E, Falconi M, Ventriglia A, Zamboni GA, Manfredi R, Pozzi Mucelli R. Perfusion CT can predict tumoral grading of pancreatic adenocarcinoma. Eur J Radiol 2013;82:227-33. [Crossref] [PubMed]
- Wang Y, Chen ZE, Nikolaidis P, McCarthy RJ, Merrick L, Sternick LA, Horowitz JM, Yaghmai V, Miller FH. Diffusion-weighted magnetic resonance imaging of pancreatic adenocarcinomas: association with histopathology and tumor grade. J Magn Reson Imaging 2011;33:136-42. [Crossref] [PubMed]
- Jang KM, Kim SH, Kim YK, Park MJ, Lee MH, Hwang J, Rhim H. Imaging features of small (≤3cm) pancreatic solid tumors on gadoxetic-acid-enhanced MR imaging and diffusion-weighted imaging: an initial experience. Magn Reson Imaging 2012;30:916-25. [Crossref] [PubMed]
- Klauss M, Lemke A, Grünberg K, Simon D, Re TJ, Wente MN, Laun FB, Kauczor HU, Delorme S, Grenacher L, Stieltjes B. Intravoxel incoherent motion MRI for the differentiation between mass forming chronic pancreatitis and pancreatic carcinoma. Invest Radiol 2011;46:57-63. [Crossref] [PubMed]
- Klauss M, Gaida M, Lemke A, Grünberg K, Simon D, Wente MN, Delorme S, Kauczor HU, Grenacher L, Stieltjes B. Fibrosis and pancreatic lesions: counterintuitive behavior of the diffusion imaging-derived structural diffusion coefficient D. Invest Radiol 2013;48:129-33. [Crossref] [PubMed]
- Wiggermann P, Grützmann R, Weissenböck A, Kamusella P, Dittert DD, Stroszczynski C. Apparent diffusion coefficient measurements of the pancreas, pancreas carcinoma, and mass-forming focal pancreatitis. Acta Radiol 2012;53:135-9. [Crossref] [PubMed]
- Hao JG, Wang JP, Gu YL, Lu ML. Importance of b value in diffusion weighted imaging for the diagnosis of pancreatic cancer. World J Gastroenterol 2013;19:6651-5. [Crossref] [PubMed]
- Kim H, Arnoletti PJ, Christein J, Heslin MJ, Posey JA 3rd, Pednekar A, Mark Beasley T, Morgan DE. Pancreatic adenocarcinoma: a pilot study of quantitative perfusion and diffusion-weighted breath-hold magnetic resonance imaging. Abdom Imaging 2014;39:744-52. [Crossref] [PubMed]
- Yao XZ, Yun H, Zeng MS, Wang H, Sun F, Rao SX, Ji Y. Evaluation of ADC measurements among solid pancreatic masses by respiratory-triggered diffusion-weighted MR imaging with inversion-recovery fat-suppression technique at 3.0T. Magn Reson Imaging 2013;31:524-8. [Crossref] [PubMed]
- Fukukura Y, Takumi K, Kamimura K, Shindo T, Kumagae Y, Tateyama A, Nakajo M. Pancreatic Adenocarcinoma: Variability of Diffusion-weighted MR Imaging Findings. Radiology 2012;263:732-40. [Crossref] [PubMed]
- Barral M, Sebbag-Sfez D, Hoeffel C, Chaput U, Dohan A, Eveno C, Boudiaf M, Soyer P. Characterization of focal pancreatic lesions using normalized apparent diffusion coefficient at 1.5-Tesla: preliminary experience. Diagn Interv Imaging 2013;94:619-27. [Crossref] [PubMed]
- Kang KM, Lee JM, Yoon JH, Kiefer B, Han JK, Choi BI. Intravoxel incoherent motion diffusion-weighted MR imaging for characterization of focal pancreatic lesions. Radiology 2014;270:444-53. [Crossref] [PubMed]
- Lee SS, Byun JH, Park BJ, Park SH, Kim N, Park B, Kim JK, Lee MG. Quantitative analysis of diffusion-weighted magnetic resonance imaging of the pancreas: usefulness in characterizing solid pancreatic masses. J Magn Reson Imaging 2008;28:928-36. [Crossref] [PubMed]
- Muraoka N, Uematsu H, Kimura H, Imamura Y, Fujiwara Y, Murakami M, Yamaguchi A, Itoh H. Apparent diffusion coefficient in pancreatic cancer: characterization and histopathological correlations. J Magn Reson Imaging 2008;27:1302-8. [Crossref] [PubMed]
- Ichikawa T, Erturk SM, Motosugi U, Sou H, Iino H, Araki T, Fujii H. High-b value diffusion-weighted MRI for detecting pancreatic adenocarcinoma: preliminary results. AJR Am J Roentgenol 2007;188:409-14. [Crossref] [PubMed]
- Yoshikawa T, Kawamitsu H, Mitchell DG, Ohno Y, Ku Y, Seo Y, Fujii M, Sugimura K. ADC measurement of abdominal organs and lesions using parallel imaging technique. AJR Am J Roentgenol 2006;187:1521-30. [Crossref] [PubMed]
- Le Bihan D, Breton E, Lallemand D, Grenier P, Cabanis E, Laval-Jeantet M. MR imaging of intravoxel incoherent motions: application to diffusion and perfusion in neurologic disorders. Radiology 1986;161:401-7. [Crossref] [PubMed]
- Le Bihan D, Breton E, Lallemand D, Aubin ML, Vignaud J, Laval-Jeantet M. Separation of diffusion and perfusion in intravoxel incoherent motion MR imaging. Radiology 1988;168:497-505. [Crossref] [PubMed]
- Le Bihan D, Turner R, Moonen CT, Pekar J. Imaging of diffusion and microcirculation with gradient sensitization: design, strategy, and significance. J Magn Reson Imaging 1991;1:7-28. [Crossref] [PubMed]
- Iima M, Le Bihan D. Clinical Intravoxel Incoherent Motion and Diffusion MR Imaging: Past, Present, and Future. Radiology 2016;278:13-32. [Crossref] [PubMed]
- Koh DM, Collins DJ, Orton MR. Intravoxel incoherent motion in body diffusion-weighted MRI: reality and challenges. AJR Am J Roentgenol 2011;196:1351-61. [Crossref] [PubMed]
- Lee HJ, Rha SY, Chung YE, Shim HS, Kim YJ, Hur J, Hong YJ, Choi BW. Tumor perfusion-related parameter of diffusion-weighted magnetic resonance imaging: correlation with histological microvessel density. Magn Reson Med 2014;71:1554-8. [Crossref] [PubMed]
- Le Bihan D. Intravoxel incoherent motion perfusion MR imaging: a wake-up call. Radiology 2008;249:748-52. [Crossref] [PubMed]
- Chandarana H, Lee VS, Hecht E, Taouli B, Sigmund EE. Comparison of biexponential and monoexponential model of diffusion weighted imaging in evaluation of renal lesions: preliminary experience. Invest Radiol 2011;46:285-91. [PubMed]
- Lemke A, Stieltjes B, Schad LR, Laun FB. Toward an optimal distribution of b values for intravoxel incoherent motion imaging. Magn Reson Imaging 2011;29:766-76. [Crossref] [PubMed]
- Muhi A, Ichikawa T, Motosugi U, Sano K, Matsuda M, Kitamura T, Nakazawa T, Araki T. High-b-value diffusion-weighted MR imaging of hepatocellular lesions: estimation of grade of malignancy of hepatocellular carcinoma. J Magn Reson Imaging 2009;30:1005-11. [Crossref] [PubMed]
- Anderson SW, Barry B, Soto JA, Ozonoff A, O'Brien M, Jara H. Quantifying hepatic fibrosis using a biexponential model of diffusion weighted imaging in ex vivo liver specimens. Magn Reson Imaging 2012;30:1475-82. [Crossref] [PubMed]
- Li YT, Cercueil JP, Yuan J, Chen W, Loffroy R, Wang YX. Liver intravoxel incoherent motion (IVIM) magnetic resonance imaging: a comprehensive review of published data on normal values and applications for fibrosis and tumor evaluation. Quant Imaging Med Surg 2017;7:59-78. [Crossref] [PubMed]
- Klöppel G, Lingenthal G, von Bülow M, Kern HF. Histological and fine structural features of pancreatic ductal adenocarcinomas in relation to growth and prognosis: studies in xenografted tumours and clinico-histopathological correlation in a series of 75 cases. Histopathology 1985;9:841-56. [Crossref] [PubMed]
- Jaster R, Emmrich J. Crucial role of fibrogenesis in pancreatic diseases. Best Pract Res Clin Gastroenterol 2008;22:17-29. [Crossref] [PubMed]
- Erkan M, Hausmann S, Michalski CW, Schlitter AM, Fingerle AA, Dobritz M, Friess H, Kleeff J. How fibrosis influences imaging and surgical decisions in pancreatic cancer. Front Physiol 2012;3:389. [Crossref] [PubMed]
- Hwang EJ, Lee JM, Yoon JH, Kim JH, Han JK, Choi BI, Lee KB, Jang JY, Kim SW, Nickel MD, Kiefer B. Intravoxel incoherent motion diffusion-weighted imaging of pancreatic neuroendocrine tumors: prediction of the histologic grade using pure diffusion coefficient and tumor size. Invest Radiol 2014;49:396-402. [Crossref] [PubMed]
- Barău A, Ruiz-Sauri A, Valencia G, Gómez-Mateo Mdel C, Sabater L, Ferrandez A, Llombart-Bosch A. High microvessel density in pancreatic ductal adenocarcinoma is associated with high grade. Virchows Arch 2013;462:541-6. [Crossref] [PubMed]
- Wáng YXJ, Deng M, Li YT, Huang H, Leung JCS, Chen W, Lu PX. A Combined Use of Intravoxel Incoherent Motion MRI Parameters Can Differentiate Early-Stage Hepatitis-b Fibrotic Livers from Healthy Livers. SLAS Technol 2017. Epub ahead of print. [Crossref] [PubMed]
- Lim JE, Chien MW, Earle CC. Prognostic factors following curative resection for pancreatic adenocarcinoma: a population based, linked database analysis of 396 patients. Ann Surg 2003;237:74-85. [Crossref] [PubMed]
- Kuhlmann KF, de Castro SM, Wesseling JG, ten Kate FJ, Offerhaus GJ, Busch OR, van Gulik TM, Obertop H, Gouma DJ. Surgical treatment of pancreatic adenocarcinoma; actual survival and prognostic factors in 343 patients. Eur J Cancer 2004;40:549-58. [Crossref] [PubMed]
- Wasif N, Ko CY, Farrell J, Wainberg Z, Hines OJ, Reber H, Tomlinson JS. Impact of tumor grade on prognosis in pancreatic cancer: should we include grade in AJCC staging? Ann Surg Oncol 2010;17:2312-20. [Crossref] [PubMed]