Quantitative texture analysis on pre-treatment computed tomography predicts local recurrence in stage I non-small cell lung cancer following stereotactic radiation therapy
Introduction
Stereotactic body radiotherapy (SBRT) has brought promising results for the treatment of medically inoperable patients with early stage non-small cell lung cancer (NSCLC) with high rates of local control (LC) and relatively little toxicity (1,2). Computed tomography (CT) of the chest is routinely used at baseline and in follow-up to monitor response to SBRT and detect local recurrence (LR). The complex beam arrangement used for SBRT often leads to the development of unusual patterns of radiation-induced changes compared to those typically seen following conventional radiotherapy (3) thus creating a greater challenge for radiologists to detect LR based on visual methods alone (4). Recent research efforts have focused on identifying imaging features at the time of diagnosis that correlate with tumor behavior and prognosis. Results demonstrate that certain morphological CT features may predict histologic subtypes of lung cancer as well as patient outcome and these may be as relevant than conventional tumor staging alone (5,6).
Several studies have also shown the utility of fluorine 18 (18F) fluorodeoxyglucose (FDG) positron emission tomography (PET)/CT as a predictor of survival with high baseline maximum standard uptake value (SUVmax) correlating with poor survival (7). Recently, texture analysis, or the quantification of gray-level patterns, pixel interrelationships, and spectral properties of an image has shown the potential to predict treatment response and survival from baseline 18F-FDG PET/CT (8-11) and CT (12,13). Most studies have identified tumor heterogeneity as a prognostic indicator independent of tumor stage and patient characteristics (14). The primary objective of this study was to determine whether texture analysis on pre-treatment CT could predict LR of stage I NSCLC. The secondary objective was to assess whether combining quantitative imaging features with SUVmax on 18F FDG PET-CT would provide added benefit to the prediction of LR.
Methods
This was a single center retrospective case-control study approved by our Research Ethics Board Protocol # 20150381-01H. The need for informed consent was waived. Patients were identified by searching the Radiation Oncology database from a single academic tertiary care institution for patients who had biopsy-proven stage I NSCLC definitively treated with SBRT between September 2008 and December 2013. Included patients had a CT of the thorax and whole body 18F-FDG PET/CT performed at baseline and a CT of the thorax performed 3 months and at least approximately 12 months after SBRT.
Due to the retrospective nature of the study, CT studies were performed on a variety of scanners with a breath-held helical acquisition of the entire thorax, 120 kV, 100–200 mAs, pitch 0.75–1.0 and collimation of 0.5 or 0.625 mm. All imaging data were reconstructed with a medium-sharp reconstruction algorithm and a slice thickness of 2.0–2.5 mm, FOV 500 mm, matrix 512 mm × 512 mm. CT images were reviewed using a picture archiving and communication system (PACS) (Horizon Medical Imaging, McKesson Corporation, San Francisco, California, USA) on lung window settings (W: 1,500; L: −550).
18F-FDG-PET/CT studies were performed on one system (Gemini Dual EXP PET/CT system, Philips Medical Systems, Cleveland, Ohio, USA), using the same protocol: Z-axis image from the base of the skull to mid-thigh, 60 minutes after intravenous (IV) injection of 6 MBq/kg of FDG. Patients had blood glucose <8 mmol/L and were fasting for over 8 hours. Low mA, 6.0 mm thick CT images were acquired without contrast for attenuation correction and a Hermes Hybrid Viewer® workstation was used for image interpretation.
Image analysis
An axial CT image at the level of the largest diameter of the tumor was selected for texture analysis by consensus by a thoracic radiology fellow with 5 years of cross-sectional imaging experience and a thoracic radiologist (Carole Dennie) with 25 years of experience both blinded to patient outcome. Each image was then exported to an independent workstation and de-identified. A contour was manually prescribed by the thoracic radiology fellow along the outer margin of the tumor using a polygonal region of interest (ROI) tool [Image J® (National Institutes of Health, USA, http://rsbweb.nih.gov)] (Figure 1). Each contour was verified for accuracy by the thoracic radiologist. For each tumor, contours were drawn on the baseline CT (baseline), the 3-month post-SBRT CT (post) and the last CT (last). The last CT was defined as the last CT available or the CT which demonstrated recurrence.
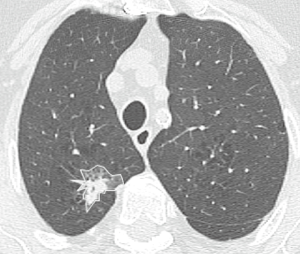
Prior to analysis, CT image intensities were normalized between µ ± 3σ where µ was the mean value of gray levels inside the ROI and µ the standard deviation (SD). Gray levels between (µ − 3σ) and (µ + 3σ) were then decimated to 64 gray levels. Previous research has shown that this normalization procedure minimizes inter-scanner effects in magnetic resonance imaging (MRI) texture analysis (15) and it is presumed to reduce inter-scanner effects in other modalities such as CT. Textural features were extracted from gray-level co-occurrence (GLCM), namely gray-level correlation (“f3”), sum of squares (“f4”), sum variance (“f7”), sum entropy (“f8”), and difference variance (“f10”) (16) were calculated using MaZda version 4.6 (17) by a physicist (Rebecca Thornhill) blinded to patient outcome. Feature definitions are provided in Table 1. The decision to assess these five features was based on a previous report showing that these showed potential for discriminating benign from malignant nodules on non-contrast CT (18). Note that each GLCM feature was initially computed for a distance of 1 pixel in each of the following directions: θ =0°, 45°, 90°, and 135°.
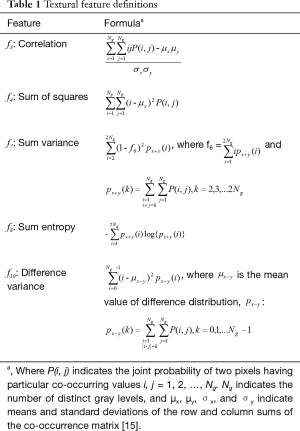
Full table
Study endpoints
The endpoint was local tumor recurrence. Patient response was assessed with Response Evaluation Criteria in Solid Tumors (RECIST). Recurrence was defined as local progression of the treated lesion on serial CT thorax ± 18 F-FDG PET/CT and multidisciplinary group consensus. Pathological proof was obtained when feasible.
Statistical analysis
Given the large number of features calculated and the relatively small number of patients, we summarized each GLCM textural feature as the mean of all four directions (θ =0°, 45°, 90°, and 135°). Longitudinal (baseline-post-last) changes in each textural feature were assessed using non-parametric repeated measures ANOVA (Friedman tests) with post-hoc Bonferroni correction for multiple comparisons. For baseline textural features, differences between patients who had LR and those without were calculated using Mann-Whitney U tests. Subsets of these features were used as predictors in logistic regression models, with LR as outcome (19). We then constructed receiver-operating characteristic (ROC) curves and calculated the area under the curve (AUC) (and standard error) for each model, both with and without 18F-FDG PET/CT SUVmax. In each case, sensitivity and specificity for predicting recurrence were assessed using the optimal criterion. Given the sample size, we combined only two textural features for any given model, to prevent over-fitting. Statistical analyses were performed using MedCalc software (version 12.14.0, Mariakerke/Belgium) and P values <0.05 were considered statistically significant.
Results
A total of 36 patients with 37 stage I peripheral NSCLC met the inclusion criteria. Patient and tumor characteristics are listed in Table 2. There were 18 LR tumors and 19 cases with LC. Thirty-six CT scans of the thorax were reviewed for the baseline pre-treatment analysis and 108 CT scans were reviewed for the longitudinal analysis. There were no statistically significant differences in the CT imaging parameters including kVp, slice thickness or pixel resolution. Tumor size, T stage and SUVmax at baseline did not differ significantly between the two groups. The median time to LR was 19.4 months (range 15–49 months). LR was proven by tissue biopsy in 5.6% (n=1), serial CT and 18F-FDG PET/CT as well as multidisciplinary consensus in 33.3% (n=6) and serial CT and multidisciplinary consensus in 61.1% (n=11). Distant metastases developed in 72.2% (n=13) of LR group and 5.6% (n=1) of the tumors that did not locally recur (P<0.001).
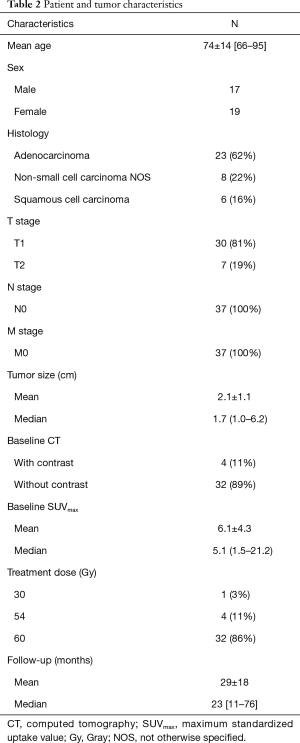
Full table
Tumor gray-level textural features were unchanged between baseline and either the CT obtained after determination of LR group, or the final CT scan evaluated (in the group with LC group; P>0.05 for each). The medians and interquartile ranges (IQR) for each feature, and for every time point are depicted in Figure 2.
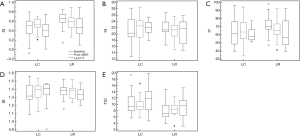
With respect to baseline CT textural features, both gray-level correlation (f3) and sum variance (f7) were greater in the group with LR, compared with the group with LC [f3 LC 0.50 (0.34–0.61) vs. f3 LR 0.66 (0.58–0.75), P=0.02; f7 with LC 61.9 (48.7–76.4) vs. f7 LR 70.5 (67.2–78.4), P=0.04]. Conversely, gray-level difference variance (f10) were significantly lower in the group with LR, compared with the LC group [f10 LC 9.33 (7.99–12.37) vs. f10 LR 7.16 (6.02–9.70), P=0.004]. There were no significant differences between groups for either f4 (sum of squares) or f8 (sum entropy) [f4 LC 20.3 (19.1–24.7) vs. f4 LR 21.3 (20.7–23.8), P=0.33; f8 LC 1.32 (1.28–1.45) vs. f8 LR 1.38 (1.33–1.42), P=0.45].
The areas under the ROC curve (AUCs) for 18F-FDG PET/CT SUVmax as well as for each of the individual CT textural features are provided in Table 3. Of the five CT features evaluated, f3, f7, and f10 were each significant predictors of LR; the AUCs associated with f3 [AUC (SE)] = [0.73 (0.08), P=0.007], f7 [0.69 (0.09), P=0.04], and f10 [0.78 (0.08), P=0.001] were significantly greater than 0.5. The baseline SUVmax alone did not perform as well as these radiomic features [AUC (SE)] = [0.62 (0.09), P=0.20].
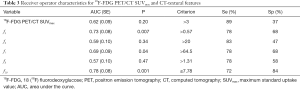
Full table
The AUCs for each combination of CT textural features, both with and without 18F-FDG PET/CT SUVmax are provided in Table 4. The logistic regression models generated using difference variance (f10) and each of f3, f4, f7, and f8 [resulted in AUC (SE) 0.75 (0.08), 0.76 (0.08), 0.77 (0.08), and 0.75 (0.08), respectively (P≤0.003 for each model)]. The addition of 18F-FDG PET/CT SUVmax to each model did not result in a significant improvement in the AUC (P≥0.75, for each comparison; Table 4).
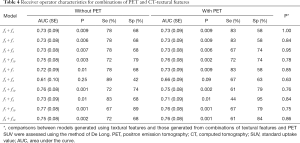
Full table
Discussion
Our study has shown that, while tumor GLCM features did not show significant changes on serial CT imaging studies, there were significant differences in baseline textural features between tumours that locally recurred and those that did not. Three of the five gray-level correlation matrix features that have previously been applied to discriminate benign from malignant nodules on CT (gray level correlation, sum variance, and difference variance) (18), also demonstrate potential for predicting LR of early stage NSCLC on baseline CT prior to SBRT.
Our results are aligned with those reported in the literature but it is challenging to compare their performance due to the choice of variable tumor stage for analysis, definition of outcome variable as well as the use of different scanning parameters, tumor segmentation methods, type of filters, quantization schemes and feature selection algorithms.
In a retrospective review of 54 consecutive patients with stage I-IV NSCLC, Ganeshan (20) investigated the relationship between tumor heterogeneity and survival. The CT portion of the 18F FDG PET/CT examination was used for texture analysis. First-order tumor uniformity was a significant independent predictor of survival with an AUC =0.596. None of the patients with uniformity <0.624 survived more than 2.5 years [odds ratio (OR), 56.4; 95% CI, 4.79–666; P=0.001]. Baseline SUVmax on 18F FDG PET-CT was not significantly associated with survival. Ravanelli (21) assessed the utility of first-order CT texture on baseline contrast-enhanced CT in 53 patients with advanced lung cancer. High texture uniformity was associated with a favorable response to first line chemotherapy in patients with adenocarcinoma. The product of mean gray-level and first-order uniformity was predictive of response (OR, 1.14; AUC under ROC curve =0.79). Weiss (22) assessed the use of first-order features on pre-treatment CT in 48 stage I–II NSCLC. Cox regression analysis indicated kurtosis with coarse-texture was a significant predictor of overall survival (HR, 2.547; 95% CI, 1.001–6.479; P=0.050). A lower kurtosis was associated with poor survival. Parmar (23) conducted the largest retrospective analysis of quantitative CT textural features in 647 patients with stage I-III NSCLC treated with radiation or chemo-radiation at two institutions in the Netherlands. The authors derived 11 clusters of radiomic features based on first-order features, second-order features (GLCM, RLNU), shape and wavelet transformation that were associated with patient survival and tumor stage. Depeursinge (24) derived predictive models from wavelet transform on baseline CT images in 91 patients with stage I adenocarcinoma treated with surgery, 17 of which recurred. Their model was predictive of recurrence with an AUC =0.8±0.1. Their definition of recurrence was variable, being either local or due to the development of distant metastatic disease. Grove (25) looked at tumor shape (convexity score) and first-order entropy of tumor periphery and tumor core on baseline CT in 108 patients with stage I–IV treated with surgery and/or radiation and/or chemo-radiation. Low convexity scores and high entropy ratios were associated with poor survival (P=0.008 and P=0.04 respectively).
Other investigators have assessed the utility of CT texture in stage 1 NSCLC treated with SBRT to predict LR but they performed their analysis on post-treatment scans rather than at baseline. Mattonen (13) analyzed 22 patients with 24 lesions and found that tumors that recurred had significantly increased variability of Hounsfield Units (HU) as measured by the SD of the HU histogram in the ground-glass opacity (GGO) surrounding the tumor as early as 9 months post-SBRT [210±14.5 HU for LR vs. 175.1±18.7 HU for no recurrence (P=0.0078)] compared to 15 months using tumor size assessment on CT alone. One of the reasons we chose the baseline CT for analysis was that we found contouring the tumor at baseline much easier and potentially more accurate than on the post-treatment scans where the edges of the lesion became obscured.
This study has several limitations. As with most reports involving quantitative CT texture analysis, our sample size is small and our study is retrospective in nature. The small sample size is particularly relevant when considering the number of textural features that were initially computed for each tumor. Given our sample size, we elected to limit our focus to the five GLCM textural features reported by Dennie et al. (18) for the identification of malignant nodules. Furthermore, we included only 10 combinations of two textural features (with or without SUVmax on 18F-FDG PET/CT) to reduce the likelihood of “overfitting” or introducing bias from random differences between tumor classes. Results were derived from a single institution and thus are hypothesis generating. Patient data were acquired on a variety of CT scanners and this may have resulted in variability in CT attenuation with a potential effect on the estimation of texture features. This should have been mitigated by the normalization procedure that was performed prior to texture analysis, however, future studies will need to address the potential influence of both scanner and noise on CT textural features. Tumor regions of interest for textural analysis were drawn manually by a single observer but manual segmentation is the current reference and previous texture analysis studies have reported a high degree of intra- and inter-observer reproducibility (21,26). We hope to investigate the use of automated segmentation in the future. Despite its limitations, our study is unique in that we specifically limited our analysis to patients with stage I NSCLC treated with SBRT rather than patients with a variety of stages undergoing a variety of treatment approaches and we assessed the utility of textural features to predict LR on pre-treatment CT. We implemented models that incorporate both quantitative imaging and SUVmax on 18F FDG PET-CT to assess the added benefit of quantitative imaging features to conventional prognostic factors as well as SUVmax. Models capable of stratifying patients with a single disease stage may be more clinically useful because different stages of disease dictate different therapeutic options.
In conclusion, our results suggest that quantitative CT texture analysis has the potential to predict LR in early stage NSCLC treated with SBRT. With optimization and a large, prospectively designed study, this approach shows the potential to provide predictive markers of recurrence thus allowing better prognostication as well as tailored treatment and post-therapeutic monitoring.
Acknowledgements
The authors would like to thank Ola Habash for her help with contour drawing and data collection.
Footnote
Conflicts of Interest: CA Souza has received consultant fees from Pfizer and honoraria from Pfizer, Boehringer-Ingelheim and Hoffmann-LaRoche unrelated to the topic of this publication; the other authors have no conflicts of interest to declare.
Ethical Statement: The study was a single center retrospective case-control study approved by our Research Ethics Board Protocol # 20150381-01H. The need for informed consent was waived.
References
- Guckenberger M, Allgäuer M, Appold S, Dieckmann K, Ernst I, Ganswindt U, Holy R, Nestle U, Nevinny-Stickel M, Semrau S, Sterzing F, Wittig A, Andratschke N. Safety and efficacy of stereotactic body radiotherapy for stage 1 non-small-cell lung cancer in routine clinical practice: a patterns-of-care and outcome analysis. J Thorac Oncol 2013;8:1050-8. [Crossref] [PubMed]
- Vansteenkiste J, De Ruysscher D, Eberhardt WE, Lim E, Senan S, Felip E, Peters S. ESMO Guidelines Working Group. Early and locally advanced non-small-cell lung cancer (NSCLC): ESMO Clinical Practice Guidelines for diagnosis, treatment and follow-up. Ann Oncol 2013;24 Suppl 6:vi89-98. [Crossref] [PubMed]
- Larici AR, del Ciello A, Maggi F, Santoro SI, Meduri B, Valentini V, Giordano A, Bonomo L. Lung abnormalities at multimodality imaging after radiation therapy for non-small cell lung cancer. Radiographics 2011;31:771-89. [Crossref] [PubMed]
- Halpenny D, Ridge CA, Hayes S, Zheng J, Moskowitz CS, Rimner A, Ginsberg MS. Computed tomographic features predictive of local recurrence in patients with early stage lung cancer treated with stereotactic body radiation therapy. Clin Imaging 2015;39:254-8. [Crossref] [PubMed]
- Gevaert O, Xu J, Hoang CD, Leung AN, Xu Y, Quon A, Rubin DL, Napel S, Plevritis SK. Non-small cell lung cancer: identifying prognostic imaging biomarkers by leveraging public gene expression microarray data--methods and preliminary results. Radiology 2012;264:387-96. [Crossref] [PubMed]
- Rami-Porta R, Crowley JJ, Goldstraw P. The revised TNM staging system for lung cancer. Ann Thorac Cardiovasc Surg 2009;15:4-9. [PubMed]
- Berghmans T, Dusart M, Paesmans M, Hossein-Foucher C, Buvat I, Castaigne C, Scherpereel A, Mascaux C, Moreau M, Roelandts M, Alard S, Meert AP, Patz EF Jr, Lafitte JJ, Sculier JP. European Lung Cancer Working Party for the IASLC Lung Cancer Staging Project. Primary tumor standardized uptake value (SUVmax) measured on fluorodeoxyglucose positron emission tomography (FDG-PET) is of prognostic value for survival in non-small cell lung cancer (NSCLC): a systematic review and meta-analysis (MA) by the European Lung Cancer Working Party for the IASLC Lung Cancer Staging Project. J Thorac Oncol 2008;3:6-12. [Crossref] [PubMed]
- Tixier F, Hatt M, Valla C, Fleury V, Lamour C, Ezzouhri S, Ingrand P, Perdrisot R, Visvikis D, Le Rest CC. Visual versus quantitative assessment of intratumor 18F-FDG PET uptake heterogeneity: prognostic value in non-small cell lung cancer. J Nucl Med 2014;55:1235-41. [Crossref] [PubMed]
- Lovinfosse P, Janvary ZL, Coucke P, Jodogne S, Bernard C, Hatt M, Visvikis D, Jansen N, Duysinx B, Hustinx R. FDG PET/CT texture analysis for predicting the outcome of lung cancer treated by stereotactic body radiation therapy. Eur J Nucl Med Mol Imaging 2016;43:1453-60. [Crossref] [PubMed]
- Pyka T, Bundschuh RA, Andratschke N, Mayer B, Specht HM, Papp L, Zsótér N, Essler M. Textural features in pre-treatment [F18]-FDG-PET/CT are correlated with risk of local recurrence and disease-specific survival in early stage NSCLC patients receiving primary stereotactic radiation therapy. Radiat Oncol 2015;10:100. [Crossref] [PubMed]
- Cook GJ, Yip C, Siddique M, Goh V, Chicklore S, Roy A, Marsden P, Ahmad S, Landau D. Are pretreatment 18F-FDG PET tumor textural features in non-small cell lung cancer associated with response and survival after chemoradiotherapy? J Nucl Med 2013;54:19-26. [Crossref] [PubMed]
- Mattonen SA, Palma DA, Haasbeek CJ, Senan S, Ward AD. Early prediction of tumor recurrence based on CT texture changes after stereotactic ablative radiotherapy (SABR) for lung cancer. Med Phys 2014;41:033502. [Crossref] [PubMed]
- Mattonen SA, Palma DA, Haasbeek CJ, Senan S, Ward AD. Distinguishing radiation fibrosis from tumour recurrence after stereotactic ablative radiotherapy (SABR) for lung cancer: a quantitative analysis of CT density changes. Acta Oncol 2013;52:910-8. [Crossref] [PubMed]
- Bashir U, Siddique MM, Mclean E, Goh V, Cook GJ. Imaging Heterogeneity in Lung Cancer: Techniques, Applications, and Challenges. AJR Am J Roentgenol 2016;207:534-43. [Crossref] [PubMed]
- Collewet G, Strzelecki M, Mariette F. Influence of MRI acquisition protocols and image intensity normalization methods on texture classification. Magn Reson Imaging 2004;22:81-91. [Crossref] [PubMed]
- Haralick RM, Shanmugam K, Dinstein I. Textural features for image classification. IEEE Trans Syst Man Cybern 1973;SMC-3:610-21. [Crossref]
- Szczypiński PM, Strzelecki M, Materka A, Klepaczko A. MaZda--a software package for image texture analysis. Comput Methods Programs Biomed 2009;94:66-76. [Crossref] [PubMed]
- Dennie C, Thornhill R, Sethi-Virmani V, Souza CA, Bayanati H, Gupta A, Maziak D. Role of quantitative computed tomography texture analysis in the differentiation of primary lung cancer and granulomatous nodules. Quant Imaging Med Surg 2016;6:6-15. [PubMed]
- Aguilera AM, Escabias M, Valderrama MJ. Using principal components for estimating logistic regression with high-dimensional multicollinear data. Comput Stat Data Anal 2006;50:1905-24. [Crossref]
- Ganeshan B, Panayiotou E, Burnand K, Dizdarevic S, Miles K. Tumour heterogeneity in non-small cell lung carcinoma assessed by CT texture analysis: a potential marker of survival. Eur Radiol 2012;22:796-802. [Crossref] [PubMed]
- Ravanelli M, Farina D, Morassi M, Roca E, Cavalleri G, Tassi G, Maroldi R. Texture analysis of advanced non-small cell lung cancer (NSCLC) on contrast-enhanced computed tomography: prediction of the response to the first-line chemotherapy. Eur Radiol 2013;23:3450-5. [Crossref] [PubMed]
- Weiss GJ, Ganeshan B, Miles KA, Campbell DH, Cheung PY, Frank S, Korn RL. Noninvasive image texture analysis differentiates K-ras mutation from pan-wildtype NSCLC and is prognostic. PLoS One 2014;9:e100244. [Crossref] [PubMed]
- Parmar C, Leijenaar RT, Grossmann P, Rios Velazquez E, Bussink J, Rietveld D, Rietbergen MM, Haibe-Kains B, Lambin P, Aerts HJ. Radiomic feature clusters and prognostic signatures specific for Lung and Head & Neck cancer. Sci Rep 2015;5:11044. [Crossref] [PubMed]
- Depeursinge A, Yanagawa M, Leung AN, Rubin DL. Predicting adenocarcinoma recurrence using computational texture models of nodule components in lung CT. Med Phys 2015;42:2054-63. [Crossref] [PubMed]
- Grove O, Berglund AE, Schabath MB, Aerts HJ, Dekker A, Wang H, Velazquez ER, Lambin P, Gu Y, Balagurunathan Y, Eikman E, Gatenby RA, Eschrich S, Gillies RJ. Quantitative computed tomographic descriptors associate tumor shape complexity and intratumor heterogeneity with prognosis in lung adenocarcinoma. PLoS One 2015;10:e0118261. [Crossref] [PubMed]
- Hodgdon T, McInnes MD, Schieda N, Flood TA, Lamb L, Thornhill RE. Can Quantitative CT Texture Analysis be Used to Differentiate Fat-poor Renal Angiomyolipoma from Renal Cell Carcinoma on Unenhanced CT Images? Radiology 2015;276:787-96. [Crossref] [PubMed]