Detecting pseudo versus true progression of glioblastoma via accurate quantitative DCE-MRI using point-of-care portable perfusion phantoms: a pilot study
Introduction
Glioblastoma remains the primary malignant brain tumor in adults, necessitating a treatment regimen involving surgical tumor resection along with chemoradiation therapy (1,2). Despite these measures, patient prognosis remains bleak, with a median survival period of only 15 months (3). A significant obstacle in managing glioblastoma is accurately distinguishing between pseudo and true progression, which complicates timely therapeutic interventions (4). Pseudoprogression arises from localized inflammation triggered by irradiation and worsened by concurrent chemotherapy, causing a transient increase in the blood-brain barrier permeability (5). It is challenging to distinguish pseudoprogression from true progression using contrast-enhanced brain magnetic resonance imaging (MRI) because both often exhibit increased contrast enhancement (6).
Currently, no definitive methods exist to differentiate between these appearances reliably. It is widely recognized that pseudoprogression is often associated with more favorable clinical outcomes (7). Therefore, misidentifying pseudoprogression as true progression can prematurely halt effective therapy (8), while mistaking true progression for pseudoprogression may lead to the inadvertent continuation of ineffective treatment and unnecessary adverse effects (9).
Dynamic contrast-enhanced magnetic resonance imaging (DCE-MRI) holds promise in distinguishing between pseudo and true progression in glioblastoma (10). True progression typically displays higher perfusion levels than pseudoprogression (11). The ability of DCE-MRI to assess blood perfusion with minimal susceptibility artifacts (12) helps ameliorate interpretative challenges associated with post-surgical changes and highlights its potential in refining glioblastoma diagnostics (13).
However, concerns persist regarding the intra/inter-scanner variability of quantitative DCE-MRI measurements due to the variations in hardware configurations, post-image-processing schemes, reconstruction algorithms, and pulse sequences (14). One possible approach entails utilizing an external phantom with a predetermined contrast-agent concentration to identify and compensate for variations. To tackle the challenges associated with quantitative DCE-MRI, an initial proposal suggested a static phantom consisting of multiple objects with different contrast-agent concentrations (15). However, a static phantom is suboptimal because it cannot mimic living tissues, where the in vivo movement of the contrast agent leads to additional MRI signal reduction (16). A portable perfusion phantom (P4) designed to mimic human tissue contrast concentration dynamics emerged as a superior reference for quantitative DCE-MRI (16-19). However, the original P4 design was unsuitable for brain scans due to its excessive size.
In this study, we developed a new P4 designed to fit inside a conventional brain coil during human subject scans. We hypothesized that employing quantitative DCE-MRI analysis with this phantom could reduce the measurement variability in quantitative DCE-MRI and subsequently improve the accuracy in differentiating between pseudo and true glioblastoma progression regardless of pharmacokinetic (PK) models. This manuscript presents the results of validating the hypothesis through a pilot clinical study conducted at a single center. We present this article in accordance with the CLEAR reporting checklist (available at https://qims.amegroups.com/article/view/10.21037/qims-2024-2566/rc).
Methods
This study received approval from the institutional review board of the University of Alabama at Birmingham (No. 300006446) and was conducted in accordance with the Declaration of Helsinki and its subsequent amendments, and the International Conference on Harmonization Good Clinical Practice guidelines. All participants provided informed consent to undergo imaging with the P4 during DCE-MRI.
Patients
Prior to enrollment, all patients provided informed consent and were thoroughly informed about the investigational nature of the study. Compliance with Health Insurance Portability and Accountability Act (HIPAA) regulations was strictly upheld. This study followed the WHO 2016 classification of glioblastoma, encompassing both isocitrate dehydrogenase (IDH) wild-type and mutant variants. From March 28, 2022 to July 12, 2023, we prospectively recruited twelve patients from the University of Alabama at Birmingham who had contrast enhancement in a follow-up MRI scan, suspicious as the recurrence of glioblastoma, after adjuvant chemoradiation therapy. All patients received radiation therapy (40–60 Gy) concurrently with chemotherapy [temozolomide or an investigational drug, BAL101553 (20)], followed by chemotherapy (temozolomide; n=7), immunotherapy [pembrolizumab (21); n=1], kinase inhibitor [trametinib (22); n=1], or anti-angiogenic agent [bevacizumab (23); n=1]. Two patients did not undergo follow-up treatment before our DCE-MRI exams due to toxicity. Patients were excluded from recruitment if they had safety contraindications for MRI, as identified through standard clinical screening, or if they were undergoing hemodialysis or had acute or chronic renal failure. The clinical characteristics of the 12 participants are summarized in Table 1. One patient was an IDH1 R132H mutant, while the others were IDH wild type. Ten subjects were white males, one subject was a Hispanic male, and one patient was a white female (median age 62 years; age range 41–76 years). The O6-methylguanine-DNA-methyltransferase (MGMT) status of each tumor was included (M: methylated, UM: unmethylated). The boundaries of pseudo or true progression on the fat-suppressed T1-weighted MRI after contrast agent (gadoteridol) injection were determined by a neuroradiologist with more than 10 years of professional experience. Two DCE-MRI scans were conducted within 1 week. The interquartile range for the time between tumor resection and the first DCE-MRI scan was 6 to 15 months, with a median of 10 months. Pseudo or true glioblastoma progression was determined according to the Response Assessment in Neuro-Oncology (RANO) criteria about 5 months after DCE-MRI exams.
Table 1
Response | Patients | Time from resection to DCE-MRI | Adjuvant chemoradiation | Follow-up therapy | IDH status | MGMT status | Age (years) | Sex | Race | BW (kg) | |
---|---|---|---|---|---|---|---|---|---|---|---|
Radiation | Chemotherapy | ||||||||||
TP | 1 | 6 months | 60 Gy | Temozolomide (75 mg/m2) | Temozolomide (150 mg/m2) | WT | M | 48 | Male | White | 85 |
2 | 10 months | 60 Gy | Phase I trial: BAL101553 | Pembrolizumab (200 mg) | WT | UM | 41 | Female | White | 68 | |
3 | 15 months | 60 Gy | Temozolomide (75 mg/m2) | None | WT | UM | 68 | Male | White | 64 | |
4 | 7 months | 40 Gy | Temozolomide (75 mg/m2) | Bevacizumab (10 mg/kg) | WT | UM | 76 | Male | White | 94 | |
5 | 24 months | 60 Gy | Temozolomide (75 mg/m2) | Trametinib (1.5 mg) | WT | UM | 64 | Male | White | 93 | |
PP | 6 | 15 months | 60 Gy | Temozolomide (75 mg/m2) | None | WT | UM | 61 | Male | White | 95 |
7 | 5 months | 60 Gy | Temozolomide (75 mg/m2) | Temozolomide (150 mg/m2) | WT | M | 58 | Male | White | 83 | |
8 | 15 months | 60 Gy | Temozolomide (75 mg/m2) | Temozolomide (200 mg/m2) | IDH1 R132H mutant | M | 42 | Male | White | 85 | |
9 | 18 months | 60 Gy | Temozolomide (75 mg/m2) | Temozolomide (200 mg/m2) | WT | M | 74 | Male | White | 86 | |
10 | 6 months | 60 Gy | Temozolomide (75 mg/m2) | Temozolomide (200 mg/m2) | WT | M | 69 | Male | White | 75 | |
11 | 6 months | 60 Gy | Temozolomide (75 mg/m2) | Temozolomide (200 mg/m2) | WT | M | 60 | Male | White | 98 | |
12 | 10 months | 60 Gy | Temozolomide (75 mg/m2) | Temozolomide (200 mg/m2) | WT | M | 63 | Male | Hispanic | 77 |
Clinical data of twelve participants with TP or PP when the therapy response was determined according to the RANO criteria about five months after DCE-MRI. BW, body weight; DCE-MRI, dynamic contrast-enhanced magnetic resonance imaging; IDH, isocitrate dehydrogenase; MGMT, O6-methylguanine-DNA-methyltransferase; M, methylated; UM, unmethylated; PP, pseudoprogression; RANO, Response Assessment in Neuro-Oncology; TP, true progression; WT, wild type.
Perfusion phantom
Figure 1A presents a photograph and an exploded computer-aided design (CAD) view of the point-of-care P4 specifically designed for brain DCE-MRI. The P4 was designed using CAD software, SolidWorks (Dassault Systèmes American Corp., Waltham, MA, USA), and 3D printed with VeroClear material on a Stratasys Objet30 Pro (Eden Prairie, MN, USA). It consists of top and bottom chambers separated by a semi-permeable membrane with a pore size of 12–14 kD (SpectrumLabs, Rancho Dominguez, CA, USA). Prior to use, both chambers were filled with degassed and deionized water. At 30 seconds after the start of DCE-MRI, a contrast agent (gadoteridol; 50 mM) was infused into the top chamber (1 mm × 10 mm × 75 mm in height, width, and length) at a constant rate of 0.16 mL/s (total volume: 1.3 mL) using a syringe pump (NE-1600, New Era Pump Systems, Inc., Farmingdale, NY, USA), displacing the water within the top chamber. The displaced water moves into the waste chamber, while the contrast agent gradually diffuses from the top chamber into the bottom chamber, which measures 7.5 mm × 10 mm × 75 mm in height, width, and length, respectively. Independent measurements conducted using liquid chromatography with tandem mass spectrometry (LC-MS) verified a linear increase in contrast concentration within the bottom chamber over 10 minutes at a rate of 0.11 mM per minute. Figure 1B showcases a photograph and an exploded CAD view of the phantom cassette capable of accommodating two P4s. Sorbothane material is employed to mitigate vibrations transmitted from the scanner. Figure 1C illustrates the cassette positioned beneath the brain coil using a hanger, with a bubble level ensuring parallel alignment with the ground. Each P4 is wrapped in water-resistant paper to prevent potential leakage. The inclusion of multiple phantom components improves the signal-to-noise ratio and aids in identifying any functional errors that may occur. Figure 1D illustrates the schematic placement of a P4 above a patient, and Figure 1E shows the T1-weighted image of a human subject with a P4.
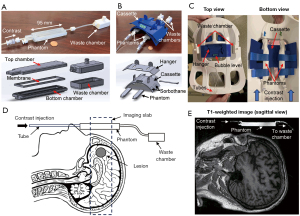
Clinical MRI protocol
This study utilized a single 3T MRI scanner (GE Signa) equipped with a dual-channel transmit RF coil. Prior to imaging, B0/B1 shimming was conducted, followed by B1 mapping using the manufacturer’s software (24). DCE-MRI was performed using a 3D fast spoiled gradient echo (SPGE) sequence. Preceding DCE-MRI, conventional MRI examinations, including 3D T1-weighted (T1W) imaging and T2-weighted (T2W) fluid-attenuated inversion recovery (FLAIR) imaging, were conducted. For T1 mapping, T1W images with varying flip angles (2°, 15°, and 30°) were acquired (25). The imaging parameters for DCE-MRI were as follows: field of view (FOV) =260 mm × 260 mm, phase/frequency encoding =154/192, number of slices =12, matrix size =256×256, slice thickness/gap =5/0 mm, flip angle =30°, repetition time (TR)/echo time (TE) =6.1/2.1 ms, number of excitations =1, SENSE factor =2, and temporal resolution =4.82 s. Gadoteridol (0.1 mmol/kg) was injected intravenously into each subject 30 seconds after the start of DCE-MRI, followed by a saline injection (20 mL) at a constant rate of 2 mL/s, using Medrad Spectris Solaris EP injector (Bayer USA, Whippany, NJ, USA). DCE-MRI acquisition lasted for 9 minutes.
Image processing
Image processing was conducted through a series of eight sequential procedures. Initially, DCE-MRI images were co-registered using the expiration-phase B-spline method (26). Subsequently, the assessment of local flip-angle (FA) variation was executed via B1 mapping (24). Following this, T1 maps were derived utilizing the various flip angle (VFA) technique (25). Contrast maps were then generated employing the method proposed by Bokacheva et al. (27). Throughout the processes of T1 and contrast mapping, adjustments were made for FA variation across the FOV using B1 maps. A lookup table was created to correlate the reference contrast enhancement curve (CEC) obtained through LC-MS with the curves measured via MRI. The population-based arterial input function (AIF) (28) was utilized to deduce the plasma input function [PIF = AIF/(1 − HCT)], with HCT representing the hematocrit measured prior to the DCE-MRI examination. Corrections were applied to the contrast maps using the aforementioned lookup table. A non-linear curve, m=α(1−exp(−βr)), was created to best fit the look-up table. In this equation, m represents the contrast agent concentration measured via MRI, r is the contrast concentration determined using the LC-MS method, and α and β are constants specific to each look-up table. These constants are determined during the fitting process. Using this curve, the contrast agent concentration corrected for MRI scanner-dependent errors was calculated using the equation, r=−(1/β)ln(1−(m/α)) [refer to Appendix B of a prior manuscript for detailed elucidation (16)]. Finally, a total of eleven PK maps were extracted using the Tofts model (TM) (Ktrans, kep, and ve) (29), extended Tofts model (ETM) (Ktrans, kep, ve, and vp) (30), and shutter speed model (SSM) (Ktrans, kep, ve, and τi) (31), where τi is mean intracellular water lifetime, vp is fractional plasma volume, ve is extravascular extracellular space, kep is flux rate constant, and Ktrans is volume transfer constant. Due to the extended time required for calculating SSM PK maps compared to TM and ETM, SSM PK maps were exclusively obtained near the region of interest (ROI). Within the ROI, the values of each PK parameter were averaged, and the kep was computed using the equation kep=Ktrans/ve. The image processing procedures were carried out using a custom software package developed in LabVIEW v17.0, with the sub-function for SSM-based PK mapping programmed in MATLAB v2020a (Mathworks, Natick, MA, USA). Validation of T1 mapping and TM/ETM-based PK mapping was performed using digital reference objects (DROs) created by Dr. Barboriak’s group (Duke University, Durham, NC, USA) and the Quantitative Imaging Biomarker Alliance. The SSM-based PK mapping was validated using the DROs developed by Dr. Yankeelov’s group (University of Texas, Austin, TX, USA).
Statistical analysis
The data consistency of the P4 CEC was assessed using the intra-class correlation coefficient (ICC). The within-subject coefficient of variation (wCV) was calculated to evaluate the consistency of the PK parameter measurements before and after P4-based error correction. Two measurements from each patient were treated as individual data points to demonstrate the classification inaccuracy caused by intra-scanner variability. The sensitivity, specificity, and accuracy of each PK parameter in distinguishing between pseudo and true progression were determined through receiver-operation characteristic curve analysis (32), with the optimal thresholds identified based on the Youden index (33). A one-way ANOVA was conducted to compare PK parameters in glioblastoma with pseudo and true progression. A P value less than 0.05 was regarded as statistically significant. All statistical analyses were conducted using SAS, version 9.4 (SAS Institute Inc., Cary, NC, USA).
Results
Figure 2A exhibits DCE-MRI images of a representative subject with recurrent glioblastoma alongside two P4s (P1 and P2) before (baseline) and at 3 and 8 minutes after contrast injection, presented in an arbitrary scale. Figure 2B displays the CECs in millimoles per liter (mM) of the two P4s depicted in Figure 2A, along with their mean. The ICC represents the concordance of the two CECs.
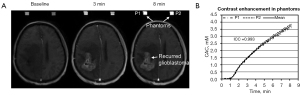
Employing P4-based error correction yielded enhancements in the repeatability of Ktrans measurements. Table 2 summarizes the wCV for each PK parameter before and after P4-based error correction. The wCV of Ktrans measurement displayed approximately 20% variability across PK models before error correction, which was markedly reduced by approximately threefold post-P4-based error correction. Notably, the wCV of kep measurement exhibited the lowest variability among all PK parameters pre-correction and experienced minimal alteration post-correction. Conversely, the wCV of vp measurement demonstrated the highest variability, attributed presumably to low signal-to-noise ratio, consistent with previous literature (17), and remained unaffected by P4-based error correction. The wCV of τi was modestly reduced following error correction.
Table 2
P4-based error correction | Repeatability | ETM | TM | SSM | ||||||||||
---|---|---|---|---|---|---|---|---|---|---|---|---|---|---|
Ktrans | ve | kep | vp | Ktrans | ve | kep | Ktrans | ve | kep | τi | ||||
After correction | wCV | 7% | 12% | 10% | 104% | 6% | 16% | 15% | 8% | 17% | 17% | 58% | ||
Before correction | wCV | 22% | 20% | 11% | 97% | 22% | 20% | 16% | 24% | 16% | 17% | 67% |
Repeatability is assessed by the wCV of each pharmacokinetic parameter, such as Ktrans, ve, kep, vp, and τi, when ETM, TM, and SSM were employed. kep, flux rate constant; Ktrans, volume transfer constant; ve, extravascular extracellular space; vp, fractional vascular space; τi, mean intracellular water lifetime. ETM, extended Tofts model; SSM, shutter speed model; TM, Tofts model; wCV, within-subject coefficient of variation.
P4-based error correction improved accuracy in distinguishing between pseudo and true glioblastoma progression. Among the participants, five exhibited true progression, while seven displayed pseudoprogression. Figure 3 presents conventional MR images alongside ETM-based Ktrans and ve maps, as well as SSM-based τi maps of two representative patients with pseudo and true progression, respectively, after P4-based error correction. All participants’ Ktrans and τi maps before and after P4-based error correction are in Figures S1-S8. Figure 4 presents box plots illustrating all eleven PK parameters of pseudo and true progression, with corresponding accuracy in differentiation for each PK parameter. Notably, TM or ETM-based Ktrans and SSM-based τi demonstrated 100% accuracy after P4-based error correction. Table 3 summarizes the sensitivity, specificity, and accuracy of each PK parameter in discerning pseudo and true progression, with optimal thresholds established based on the Youden index (33).
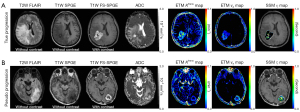
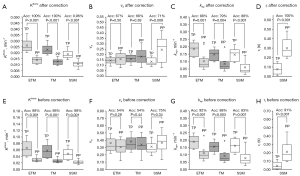
Table 3
P4-based error correction | Performance metrics | ETM | TM | SSM | ||||||||||
---|---|---|---|---|---|---|---|---|---|---|---|---|---|---|
Ktrans | ve | kep | vp | Ktrans | ve | kep | Ktrans | ve | kep | τi | ||||
After correction | Threshold | 0.018 | 0.168 | 0.115 | <0.0001 | 0.018 | 0.200 | 0.087 | 0.013 | 0.189 | 0.077 | 0.156 | ||
Sensitivity | 100% | 80% | 100% | 100% | 100% | 70% | 100% | 100% | 100% | 100% | 100% | |||
Specificity | 100% | 57% | 79% | 7% | 100% | 43% | 71% | 93% | 64% | 79% | 100% | |||
Accuracy | 100% | 67% | 88% | 46% | 100% | 66% | 79% | 96% | 71% | 88% | 100% | |||
Before correction | Threshold | 0.031 | 0.258 | 0.133 | <0.0001 | 0.028 | 0.315 | 0.115 | 0.025 | 0.289 | 0.098 | 0.081 | ||
Sensitivity | 100% | 80% | 100% | 100% | 100% | 60% | 90% | 100% | 70% | 100% | 80% | |||
Specificity | 79% | 36% | 86% | 29% | 79% | 50% | 86% | 79% | 79% | 79% | 100% | |||
Accuracy | 88% | 54% | 92% | 58% | 88% | 54% | 88% | 88% | 75% | 83% | 91% |
The sensitivity, specificity, and accuracy of each pharmacokinetic parameter in differentiating between pseudo and true progression before and after P4-based error correction. The pharmacokinetic parameters include Ktrans, ve, kep, vp, and τi when ETM, TM, and SSM were employed. The optimal thresholds identified based on the Youden index are included, where the unit for Ktrans and kep is min-1 and that for τi is second. The ve is unitless. kep, flux rate constant; Ktrans, volume transfer constant; ve, extravascular extracellular space; vp, fractional vascular space; τi, mean intracellular water lifetime. ETM, extended Tofts model; SSM, shutter speed model; TM, Tofts model.
Discussion
We demonstrated that the P4 can be effectively employed within a brain coil during human subject studies to minimize scanner-dependent errors in quantitative DCE-MRI measurements. This led to enhanced accuracy in distinguishing between pseudo and true glioblastoma progression and holds significant clinical benefits, as clinicians can make more informed decisions regarding treatment strategies. The earlier diagnosis of true progression would be helpful by allowing patients to be treated when the tumor burden is smaller and patients are healthier and better at tolerating experimental treatments. We enrolled patients, irrespective of the specific follow-up therapy employed, but further investigation would be required to evaluate the changes in PK parameters associated with each therapeutic mechanism in an expanded patient cohort. In addition, on average, about one-third of glioblastoma patients exhibit pseudoprogression, while two-thirds show true progression (34). However, the patients in this study displayed approximately the opposite pattern. Our recruitment criteria included patients who exhibited contrast enhancement in follow-up MRI scans and were suspected of glioblastoma recurrence. As no recruitment bias should have been introduced, we believe this discrepancy is likely due to the small sample size.
The accuracy of TM-based Ktrans was 88% in differentiating pseudo and true progression even before error correction, surpassing the findings of Yun et al. by 12% (11). Moreover, our study identified an optimal threshold value for TM-based Ktrans, approximately 0.03 min-1, which was substantially lower than the threshold reported by Yun et al. (11). This discrepancy could be attributed to differences in methodology, specifically in the AIF measurement. While Yun et al. relied on semi-automatic AIF measurements from intracranial tumor-supplying arteries proximal to the tumor (11), we opted for a population-based AIF (28). The utilization of intracranial arteries, which are approximately 1 mm in size for AIF measurement, may introduce partial volume effects, thereby potentially diminishing AIF magnitude and subsequently overestimating Ktrans. Besides, Shalom et al. presented the higher reproducibility of glioblastoma Ktrans quantified using population-based AIF compared to those quantified with measured AIF (35). This possibly explains the higher accuracy of Ktrans observed in our findings than that reported by Yun et al. (11).
The true progression showed lower τi than the pseudoprogression even before P4-based error correction, suggesting τicould be a useful imaging biomarker for differentiating pseudo and true glioblastoma progression. The τi is an SSM-exclusive parameter accounting for trans-cell membrane water exchange kinetics in PK modeling of DCE-MRI. Recent studies have demonstrated that τi is an imaging biomarker for cellular metabolic activity, exhibiting an inverse relationship with the Na+-K+-ATPase (NKA) pumping. This pumping is activated by a phosphate produced through the ATP cycle and plays a crucial role in maintaining the K+ and Na+ gradient in vivo (36-38). Our findings support the hypothesis that recurring glioblastoma (i.e., true progression) consumes more ATP than inflammation (i.e., pseudoprogression), increasing NKA pumping and reducing τi. Tudorica et al. showed in a DCE-MRI study that changes in tumor τi provided an accurate prediction of breast cancer response to neoadjuvant chemotherapy after only the first cycle of chemotherapy, with more significant increases of τi in responding patients (39). Chawla et al. also demonstrated that pre-treatment τi of head and neck cancer was an independent predictor of overall survival following chemoradiotherapy, with higher τi corresponding to more prolonged survival (40).
Before P4-based error correction, the wCV of kep measurement was lower than that of Ktrans, consistent with findings reported by Huang et al. (41,42). This is likely because kep is the contrast-agent intravasation rate constant, mainly determined by the wash-out phase where the range of contrast-agent concentration changes is relatively small. Conversely, Ktrans is the contrast-agent extravasation rate constant, predominantly characterized by the uptake phase where the range of contrast-agent concentration changes is more extensive, leading to a larger error in quantitating contrast-agent concentration, particularly in a high-field MRI scanner. The P4-based error correction mitigated this variability, enhancing the measurement repeatability of Ktrans.
In this study, the patient’s discomfort with the P4s remains a concern. While all twelve participants were successfully fitted with P4s within the brain coil, instances occurred where the P4s inadvertently made contact with the face of the participant. Consequently, the cushion positioned at the base of the coil had to be removed sometimes, leading to discomfort among the participants. The dimensions of the P4 bottom chamber were initially 10 mm × 7.5 mm (width × height), but this could be reduced to 4 mm × 4 mm without inducing a partial volume effect, given the spatial image resolution of 1 mm. Additionally, considerations were made to place two P4s on the left and right sides of the brain coil, allowing a human subject to see the outside of the MRI bore via a mirror and potentially alleviate claustrophobia if it occurs.
Conclusions
Quantitative DCE-MRI accurately differentiated pseudo and true progression, achieving 100% accuracy with P4-based error correction. However, multi-institutional validation is necessary to confirm its robustness across diverse clinical settings. Additionally, due to variations in brain coil designs among vendors, developing a universal P4 hanger adaptable to different coil shapes is essential for broader clinical implementation.
Acknowledgments
The authors thank Ms. Nicole Haynes and Mr. Nicholas Hatfield for their assistance with phantom evaluation and patient imaging. All experiments comply with current regulatory requirements (including ethics requirements) and laws of the United States of America.
Footnote
Reporting Checklist: The authors have completed the CLEAR reporting checklist. Available at https://qims.amegroups.com/article/view/10.21037/qims-2024-2566/rc
Funding: This study was supported by
Conflicts of Interest: All authors have completed the ICMJE uniform disclosure form (available at https://qims.amegroups.com/article/view/10.21037/qims-2024-2566/coif). H.K. reports that this study was funded by an NCI grant (R03CA245986). D.B. reports that he is the National Study Chair for the NCI funded ECOG-ACRIN trial EA223/GABLE. He serves as Imaging Co-Chair for ECOG-ACRIN’s Brain Tumor Working Group and as Chair of ECOG-ACRIN’s Imaging Diagnostics and Biomarker Committee. He has been a consultant for Servier about imaging in a different group of patients, those with low-grade glioma. Sevier reimbursed his travel and paid for meals for a trip to their headquarters in Boston, where he gave a presentation and participated in the discussion. The other authors have no conflicts of interest to declare.
Ethical Statement: The authors are accountable for all aspects of the work in ensuring that questions related to the accuracy or integrity of any part of the work are appropriately investigated and resolved. This study received approval from the institutional review board of the University of Alabama at Birmingham (No. 300006446) and was conducted in accordance with the Declaration of Helsinki and its subsequent amendments, and the International Conference on Harmonization Good Clinical Practice guidelines. All participants provided informed consent to undergo imaging with the P4 during DCE-MRI.
Open Access Statement: This is an Open Access article distributed in accordance with the Creative Commons Attribution-NonCommercial-NoDerivs 4.0 International License (CC BY-NC-ND 4.0), which permits the non-commercial replication and distribution of the article with the strict proviso that no changes or edits are made and the original work is properly cited (including links to both the formal publication through the relevant DOI and the license). See: https://creativecommons.org/licenses/by-nc-nd/4.0/.
References
- Wirsching HG, Galanis E, Weller M. Glioblastoma. Handb Clin Neurol 2016;134:381-97. [Crossref] [PubMed]
- Le Rhun E, Preusser M, Roth P, Reardon DA, van den Bent M, Wen P, Reifenberger G, Weller M. Molecular targeted therapy of glioblastoma. Cancer Treat Rev 2019;80:101896. [Crossref] [PubMed]
- Campos B, Olsen LR, Urup T, Poulsen HS. A comprehensive profile of recurrent glioblastoma. Oncogene 2016;35:5819-25. [Crossref] [PubMed]
- Young JS, Al-Adli N, Scotford K, Cha S, Berger MS. Pseudoprogression versus true progression in glioblastoma: what neurosurgeons need to know. J Neurosurg 2023;139:748-59. [Crossref] [PubMed]
- Le Fèvre C, Constans JM, Chambrelant I, Antoni D, Bund C, Leroy-Freschini B, Schott R, Cebula H, Noël G. Pseudoprogression versus true progression in glioblastoma patients: A multiapproach literature review. Part 2 - Radiological features and metric markers. Crit Rev Oncol Hematol 2021;159:103230. [Crossref] [PubMed]
- Strauss SB, Meng A, Ebani EJ, Chiang GC. Imaging Glioblastoma Posttreatment: Progression, Pseudoprogression, Pseudoresponse, Radiation Necrosis. Neuroimaging Clin N Am 2021;31:103-20. [Crossref] [PubMed]
- Gahramanov S, Varallyay C, Tyson RM, Lacy C, Fu R, Netto JP, Nasseri M, White T, Woltjer RL, Gultekin SH, Neuwelt EA. Diagnosis of pseudoprogression using MRI perfusion in patients with glioblastoma multiforme may predict improved survival. CNS Oncol 2014;3:389-400. [Crossref] [PubMed]
- Nabavizadeh A, Bagley SJ, Doot RK, Ware JB, Young AJ, Ghodasara S, Zhao C, Anderson H, Schubert E, Carpenter EL, Till J, Henderson F Jr, Pantel AR, Chen HI, Lee JYK, Amankulor NM, O'Rourke DM, Desai A, Nasrallah MP, Brem S. Distinguishing Progression from Pseudoprogression in Glioblastoma Using (18)F-Fluciclovine PET. J Nucl Med 2023;64:852-8. [Crossref] [PubMed]
- Young RJ, Gupta A, Shah AD, Graber JJ, Chan TA, Zhang Z, Shi W, Beal K, Omuro AM. MRI perfusion in determining pseudoprogression in patients with glioblastoma. Clin Imaging 2013;37:41-9. [Crossref] [PubMed]
- Thomas AA, Arevalo-Perez J, Kaley T, Lyo J, Peck KK, Shi W, Zhang Z, Young RJ. Dynamic contrast enhanced T1 MRI perfusion differentiates pseudoprogression from recurrent glioblastoma. J Neurooncol 2015;125:183-90. [Crossref] [PubMed]
- Yun TJ, Park CK, Kim TM, Lee SH, Kim JH, Sohn CH, Park SH, Kim IH, Choi SH. Glioblastoma treated with concurrent radiation therapy and temozolomide chemotherapy: differentiation of true progression from pseudoprogression with quantitative dynamic contrast-enhanced MR imaging. Radiology 2015;274:830-40. [Crossref] [PubMed]
- Maiter A, Butteriss D, English P, Lewis J, Hassani A, Bhatnagar P. Assessing the diagnostic accuracy and interobserver agreement of MRI perfusion in differentiating disease progression and pseudoprogression following treatment for glioblastoma in a tertiary UK centre. Clin Radiol 2022;77:e568-75. [Crossref] [PubMed]
- Suh CH, Kim HS, Jung SC, Choi CG, Kim SJ. Multiparametric MRI as a potential surrogate endpoint for decision-making in early treatment response following concurrent chemoradiotherapy in patients with newly diagnosed glioblastoma: a systematic review and meta-analysis. Eur Radiol 2018;28:2628-38. [Crossref] [PubMed]
- Kim H. Variability in Quantitative DCE-MRI: Sources and Solutions. J Nat Sci 2018;4:e484.
- Jackson EF, Gupta SN, A. RM, et al. QIBA DCE-MRI technical committee update: phantom studies and first DCEMRI profile. In: Proceedings of the 96th Scientific Assembly and Annual Meeting of the Radiological Society of North America, Chicago, Ill, USA, December 2010. 2010.
- Kim H, Mousa M, Schexnailder P, Hergenrother R, Bolding M, Ntsikoussalabongui B, Thomas V, Morgan DE. Portable perfusion phantom for quantitative DCE-MRI of the abdomen. Med Phys 2017;44:5198-209. [Crossref] [PubMed]
- Holland MD, Morales A, Simmons S, Smith B, Misko SR, Jiang X, Hormuth DA, Christenson C, Koomullil RP, Morgan DE, Li Y, Xu J, Yankeelov TE, Kim H. Disposable point-of-care portable perfusion phantom for quantitative DCE-MRI. Med Phys 2022;49:271-81. [Crossref] [PubMed]
- Kim H, Morgan DE, Schexnailder P, Navari RM, Williams GR, Bart Rose J, Li Y, Paluri R. Accurate Therapeutic Response Assessment of Pancreatic Ductal Adenocarcinoma Using Quantitative Dynamic Contrast-Enhanced Magnetic Resonance Imaging With a Point-of-Care Perfusion Phantom: A Pilot Study. Invest Radiol 2019;54:16-22. [Crossref] [PubMed]
- Kim H, Thomas JV, Nix JW, Gordetsky JB, Li Y, Rais-Bahrami S. Portable Perfusion Phantom Offers Quantitative Dynamic Contrast-Enhanced Magnetic Resonance Imaging for Accurate Prostate Cancer Grade Stratification: A Pilot Study. Acad Radiol 2021;28:405-13. [Crossref] [PubMed]
- Joerger M, Hundsberger T, Haefliger S, von Moos R, Hottinger AF, Kaindl T, Engelhardt M, Marszewska M, Lane H, Roth P, Stathis A. Safety and anti-tumor activity of lisavanbulin administered as 48-hour infusion in patients with ovarian cancer or recurrent glioblastoma: a phase 2a study. Invest New Drugs 2023;41:267-75. [Crossref] [PubMed]
- Bagley SJ, Binder ZA, Lamrani L, Marinari E, Desai AS, Nasrallah MP, et al. Repeated peripheral infusions of anti-EGFRvIII CAR T cells in combination with pembrolizumab show no efficacy in glioblastoma: a phase 1 trial. Nat Cancer 2024;5:517-31. [Crossref] [PubMed]
- Habibi MA, Mirjani MS, Ahmadvand MH, Delbari P, Alasti O. The safety and efficacy of dabrafenib and trametinib in patients with glioma: A systematic review and meta-analysis. Eur J Clin Pharmacol 2024;80:639-56. [Crossref] [PubMed]
- Wei S, Chang L, Zhong Y. The efficacy and adverse events of bevacizumab combined with temozolomide in the treatment of glioma: a systemic review and meta-analysis of randomized controlled trials. Front Med (Lausanne) 2024;11:1419038. [Crossref] [PubMed]
- Sacolick LI, Wiesinger F, Hancu I, Vogel MW. B1 mapping by Bloch-Siegert shift. Magn Reson Med 2010;63:1315-22. [Crossref] [PubMed]
- Liberman G, Louzoun Y, Ben Bashat D T. 1 mapping using variable flip angle SPGR data with flip angle correction. J Magn Reson Imaging 2014;40:171-80. [Crossref] [PubMed]
- Li Z, Tielbeek JAW, Caan MWA, Puylaert CAJ, Ziech MLW, Nio CY, Stoker J, van Vliet LJ, Vos FM. Expiration-phase template-based motion correction of free-breathing abdominal dynamic contrast enhanced MRI. IEEE Trans Biomed Eng 2015;62:1215-25. [Crossref] [PubMed]
- Bokacheva L, Rusinek H, Chen Q, Oesingmann N, Prince C, Kaur M, Kramer E, Lee VS. Quantitative determination of Gd-DTPA concentration in T1-weighted MR renography studies. Magn Reson Med 2007;57:1012-8. [Crossref] [PubMed]
- Parker GJ, Roberts C, Macdonald A, Buonaccorsi GA, Cheung S, Buckley DL, Jackson A, Watson Y, Davies K, Jayson GC. Experimentally-derived functional form for a population-averaged high-temporal-resolution arterial input function for dynamic contrast-enhanced MRI. Magn Reson Med 2006;56:993-1000. [Crossref] [PubMed]
- Tofts PS. Modeling tracer kinetics in dynamic Gd-DTPA MR imaging. J Magn Reson Imaging 1997;7:91-101. [Crossref] [PubMed]
- Tofts PS, Brix G, Buckley DL, Evelhoch JL, Henderson E, Knopp MV, Larsson HB, Lee TY, Mayr NA, Parker GJ, Port RE, Taylor J, Weisskoff RM. Estimating kinetic parameters from dynamic contrast-enhanced T(1)-weighted MRI of a diffusable tracer: standardized quantities and symbols. J Magn Reson Imaging 1999;10:223-32. [Crossref] [PubMed]
- Li X, Huang W, Yankeelov TE, Tudorica A, Rooney WD, Springer CS Jr. Shutter-speed analysis of contrast reagent bolus-tracking data: Preliminary observations in benign and malignant breast disease. Magn Reson Med 2005;53:724-9. [Crossref] [PubMed]
- Fawcett T. An introduction to ROC analysis. Pattern Recognition Letters 2006;27:861-74.
- Liu A, Schisterman EF, Zhu Y. On linear combinations of biomarkers to improve diagnostic accuracy. Stat Med 2005;24:37-47. [Crossref] [PubMed]
- Abbasi AW, Westerlaan HE, Holtman GA, Aden KM, van Laar PJ, van der Hoorn A. Incidence of Tumour Progression and Pseudoprogression in High-Grade Gliomas: a Systematic Review and Meta-Analysis. Clin Neuroradiol 2018;28:401-11. [Crossref] [PubMed]
- Shalom ES, Kim H, van der Heijden RA, Ahmed Z, Patel R, Hormuth DA 2nd, et al. The ISMRM Open Science Initiative for Perfusion Imaging (OSIPI): Results from the OSIPI-Dynamic Contrast-Enhanced challenge. Magn Reson Med 2024;91:1803-21. [Crossref] [PubMed]
- Ruggiero MR, Baroni S, Pezzana S, Ferrante G, Geninatti Crich S, Aime S. Evidence for the Role of Intracellular Water Lifetime as a Tumour Biomarker Obtained by In Vivo Field-Cycling Relaxometry. Angew Chem Int Ed Engl 2018;57:7468-72. [Crossref] [PubMed]
- Springer CS Jr, Li X, Tudorica LA, Oh KY, Roy N, Chui SY, Naik AM, Holtorf ML, Afzal A, Rooney WD, Huang W. Intratumor mapping of intracellular water lifetime: metabolic images of breast cancer? NMR Biomed 2014;27:760-73. [Crossref] [PubMed]
- Zhang Y, Poirier-Quinot M, Springer CS Jr, Balschi JA. Active trans-plasma membrane water cycling in yeast is revealed by NMR. Biophys J 2011;101:2833-42. [Crossref] [PubMed]
- Tudorica A, Oh KY, Chui SY, Roy N, Troxell ML, Naik A, Kemmer KA, Chen Y, Holtorf ML, Afzal A, Springer CS Jr, Li X, Huang W. Early Prediction and Evaluation of Breast Cancer Response to Neoadjuvant Chemotherapy Using Quantitative DCE-MRI. Transl Oncol 2016;9:8-17. [Crossref] [PubMed]
- Chawla S, Loevner LA, Kim SG, et al. Dynamic Contrast-Enhanced MRI-Derived Intracellular Water Lifetime (τ i): A Prognostic Marker for Patients with Head and Neck Squamous Cell Carcinomas. AJNR Am J Neuroradiol 2018;39:138-44. [Crossref] [PubMed]
- Huang W, Chen Y, Fedorov A, Li X, Jajamovich GH, Malyarenko DI, et al. The Impact of Arterial Input Function Determination Variations on Prostate Dynamic Contrast-Enhanced Magnetic Resonance Imaging Pharmacokinetic Modeling: A Multicenter Data Analysis Challenge. Tomography 2016;2:56-66. [Crossref] [PubMed]
- Huang W, Chen Y, Fedorov A, Li X, Jajamovich GH, Malyarenko DI, et al. The Impact of Arterial Input Function Determination Variations on Prostate Dynamic Contrast-Enhanced Magnetic Resonance Imaging Pharmacokinetic Modeling: A Multicenter Data Analysis Challenge, Part II. Tomography 2019;5:99-109. [Crossref] [PubMed]