Classification of carotid artery plaques: promising alternative methods to computed tomography angiography through radiomics approach using neck non-contrast computed tomography
Introduction
Stroke is the second leading cause of death globally, being responsible for 11.6% of all fatalities (1). Carotid artery plaques (CAPs) substantially contribute stroke risk, accounting for 15–20% of all ischemic strokes (2). Traditionally, the evaluation of CAP risk relies on stenosis quantification (3). However, increasing evidence suggests that ischemic strokes are often caused by arterial thrombi released from vulnerable plaques after the fibrous cap ruptures (4), and plaque stability is closely related to its composition.
The most prevalent low-risk feature of plaques is calcification, whereas non-calcification represents the most common high-risk feature. Non-calcified plaques are characterized by intraplaque hemorrhage, lipid-rich necrotic core, and fibrous elements (5), all of which are closely associated with an increased risk of stroke. Moreover, non-calcified plaques exhibit lower computed tomography (CT) attenuation values ranging from approximately 16 to 90 Hounsfield units (HU) on non-contrast images, and they can manifest individually or in conjunction with calcified plaques. Notably, when non-calcified plaques with peripheral calcification (mean CT attenuation value of roughly 250 HU) are detected at the luminal border, they are deemed high-risk markers (6). Therefore, accurate plaque characterization is paramount for stroke risk prediction. Patients with CAPs frequently remain asymptomatic in the early stages, possibly presenting only mild dizziness or headaches. Therefore, timely and accurate screening for CAPs and identification of their constituents will assist in assessing plaque stability, preventing strokes, and providing a basis for clinical treatment.
Currently, carotid artery ultrasound is the most commonly used method for assessing CAP composition, but it is highly operator-dependent and susceptible to interobserver variability (7). High-resolution magnetic resonance imaging (MRI) can produce various images with different contrasts through different sequences and parameters, offering extremely high soft tissue resolution and sensitivity. This technique can be used to identify hemorrhage, ulceration, lipid core, and inflammation within the plaque (8). However, because of limitations regarding the scanning time and cost, this technique has not been widely used. Computed tomography angiography (CTA) is widely used as a non-invasive diagnostic tool for vascular lesions. However, because of adverse reactions to iodinated contrast agents, longer examination times, and higher costs, it has not become the primary tool for screening CAPs (9). Non-contrast computed tomography (NCCT) has the potential to provide large-scale screening for clinical indications. For example, an artificial intelligence model for the early detection of pancreatic cancer can accurately detect and classify pancreatic lesions using NCCT (10). The use of NCCT in the initial screening of patients with CAPs and identification of patients requiring further examination could greatly improve the utilization of clinical resources and reduce medical costs. However, because of the overlap in CT values between non-calcified plaques and blood, it is challenging to accurately differentiate them on NCCT images via visual inspection alone. Radiomics, unlike traditional medical imaging used for visual interpretation, involves extracting quantitative features (often invisible to the naked eye) from images. These features describe texture patterns or geometric properties within specified regions of interest (ROIs), capturing complex relationships between voxels, and subsequently generating minable data (11). Recently, radiomics-based methods have become reliable tools for evaluating cardiovascular diseases (12), successfully establishing machine learning models based on CTA or MRI to accurately differentiate symptomatic and asymptomatic CAPs, and they have outperformed traditional models in identifying high-risk plaques (8,13).
Therefore, this study utilized radiomics to extract the features of plaques and establish machine learning models to classify CAPs using NCCT. We present this article in accordance with the TRIPOD + AI reporting checklist (available at https://qims.amegroups.com/article/view/10.21037/qims-24-1974/rc).
Methods
Research objectives
This study was approved by the ethics committee of The First Affiliated Hospital of Ningbo University (approval No. 057A) and was performed in compliance with the Declaration of Helsinki and its subsequent amendments. The requirement for informed consent was waived because of the retrospective nature of the study. From January 2020 to December 2022, 500 patients with confirmed CAPs were included in the main cohort at The First Affiliated Hospital of Ningbo University. These patients were randomly divided into the training and internal test sets at a ratio of 8:2. One hundred patients with confirmed CAPs from Ningbo No. 2 Hospital were selected for external validation. The inclusion criteria were as follows: (I) evaluation by neck CTA; (II) images demonstrating the presence of CAPs; (III) availability of complete, high-quality paired NCCT-CTA Digital Imaging and Communications in Medicine (DICOM) data suitable for plaque identification and omics feature extraction; and (IV) body mass index (BMI) of 18.5–24 kg/m2. The exclusion criteria were as follows: (I) poor image quality because of motion artifacts; (II) prior vascular reconstruction for CAPs, including carotid artery stent placement and carotid endarterectomy; (III) diseases other than atherosclerosis, such as arterial aneurysms; (IV) inclusion in other studies; and (V) an insufficient follow-up period.
Data collection
The images for patients in the training and internal test sets were acquired using a 320-row CT scanner (Aquilion One, Toshiba, Japan), whereas those for the external test set were acquired using Siemens (SIEMENS SOMATOM Force) and GE (GE Optima 620) CT scanners. According to the standardized arterial CTA guidelines in China, patients with poor image quality were excluded according to the inclusion and exclusion criteria, resulting in the acquisition of paired neck NCCT–CTA data that met the diagnostic requirements. Initially, the patients underwent non-contrast scanning, followed by CTA of the head and neck. The scan extended from the ascending aorta to the skull vertex. Iohexol (370 mgI/mL), a non-ionic contrast agent, was injected into the antecubital vein of each patient at a rate of 4.0 mL/s (total volume of 80 mL), followed by flushing with 30 mL of 0.9% sodium chloride solution injected at the same rate. For CTA, SureStart contrast agent tracking was used, with the ascending aorta as the ROI trigger point and the trigger threshold set at 200 HU. The tube voltage and tube current were set at 120 kVp and 250 mA, respectively, and the slice thickness and interval were both 1 mm.
Image annotation
NCCT and CTA images were imported into the Union Image Intelligent Research Platform system [uAI Research Portal, version 20240130 (14)] in the DICOM format. On the NCCT images, vascular contours were manually delineated on the slice with the largest carotid plaque area and its adjacent slices of normal carotid artery (Figure 1). According to the proposed plaque classification scheme (15), carotid plaques were categorized as calcified plaques (CT attenuation >130 HU), non-calcified plaques (CT attenuation visually higher than that of surrounding fat but different from that of non-contrast blood in the center of the vessel lumen, excluding artifacts, with consistency compared with lesion morphology on CTA), and mixed plaques (including both calcified and non-calcified components). Differences in the degree of patient obesity can result in variations in perivascular fat gap content, which can affect the delineation of the ROI. Therefore, the BMI of the enrolled patients was maintained within the range of 18.5–24 kg/m2 to ensure the accuracy of ROI delineation. Because of challenges in annotations on NCCT images and concerns about reliability, annotations on NCCT images were independently made by two board-certified radiologists with different levels of expertise in diagnostic neuroradiology [4 years for reader 1 (H.W.) and 6 years for reader 2 (Q.M.)] in reference to images manually marked on registered CTA images. In total, 50 subjects were randomly selected to evaluate intra- and interobserver reproducibility in delineating carotid plaques. To assess intraobserver reproducibility, reader 1 delineated the same patient’s ROI twice within 1 week using the same method. For interobserver reproducibility, reader 2 delineated the ROI and compared the extracted features with those obtained by reader 1 during the initial session. The intraclass correlation coefficient (ICC) was used to measure reproducibility, with an ICC greater than 0.75 indicating good consistency.
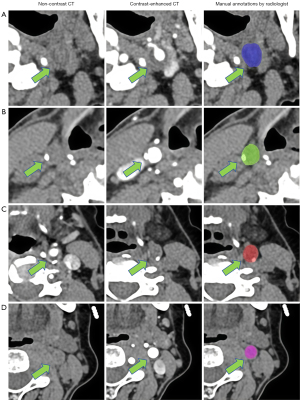
Radiomics feature extraction and selection
To enhance the consistency of image acquisition, all NCCT images were resampled to a uniform voxel size of 1×1×1 mm3. Radiomics features were then extracted from each annotated ROI, encompassing seven types: shape, first order, gray level co-occurrence matrix (GLCM), gray level run length matrix (GLRLM), gray level size zone matrix (GLSZM), neighboring gray tone difference matrix (NGTDM), and gray level dependence matrix (GLDM). To ensure the stability and reproducibility of the model, the ICC was employed to conduct consistency tests on the extracted features, including both intra- and interobserver reliability analyses. Features with ICC >0.75 were retained. Following Z-score normalization, the least absolute shrinkage and selection operator (LASSO) algorithm was utilized for feature selection. Subsequently, based on the selected radiomics features, the radiomics score was calculated.
Establishment of machine learning classifiers
In this study, we introduced two classification models, namely the four-class classification model (FCM) and two-level binary classification model (TBCM), which are designed to categorize CAPs into four distinct subtypes based on the radiomics features extracted from NCCT images. TBCM comprises three binary classifiers. Classifier 1 discriminates the presence of calcification within the carotid artery lumen, functioning as the initial classifier. Subsequently, in cases in which calcification is present, classifier 2 distinguishes between calcified plaques and mixed plaques, whereas in the absence of calcification, classifier 3 differentiates between non-calcified plaques and no plaques, thereby constituting the second level of classification. These models were constructed using five machine learning algorithms: Gaussian processes (GP), support vector machine (SVM), decision tree (DT), logistic regression (LR), and random forest (RF). The optimal model was selected from these algorithms and evaluated in an external test set to assess its generalization capability. Evaluation metrics such as the receiver operating characteristic (ROC) curve, area under the ROC curve (AUC), accuracy, F1 score, precision, recall, and specificity were employed to evaluate the classification performance of each model. The scheme of the study is presented in Figure 2.
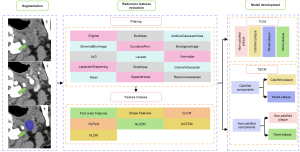
Statistical analysis
Continuous variables were expressed as the mean ± standard deviation (SD) or median (interquartile range), and the normality of the data was analyzed using the Kolmogorov-Smirnov test. Group differences were compared using an independent-samples t-test or a non-parametric test. Categorical data were assessed for group differences using the chi-squared test. The ROC curves of various models were plotted for selected features, and the AUC was calculated. The diagnostic ability of each model was evaluated using accuracy, F1 score, precision, sensitivity, and specificity. The DeLong test was used to analyze the differences in diagnostic performance between ROC curves. P<0.05 was considered statistically significant.
Results
Clinical characteristics of the patients
The clinical characteristics of the three groups of patients are summarized in Table 1. No statistically significant differences were observed regarding sex or age in the training, internal test, and external test groups (P>0.05, Table 1). There were no statistically significant differences in BMI or hemoglobin levels among the four plaque subtype groups (P>0.05, Table 2).
Table 1
Clinical features | Training set | Internal test set | External test set | P |
---|---|---|---|---|
Sex | 0.683 | |||
Male | 325 | 85 | 82 | |
Female | 75 | 15 | 18 | |
Age (years) | 67.9±9.3 | 68.6±10.4 | 70.1±9.9 | 0.057 |
Data are presented as mean ± standard deviation or n.
Table 2
Clinical features | Non-calcified plaques (n=357) | Calcified plaques (n=252) | Mixed plaques (n=325) |
No plaques (n=869) |
P |
---|---|---|---|---|---|
Hemoglobin levels (g/L) | 123.8±12.0 | 126.7±11.6 | 130.1±10.2 | 129.3±13.4 | 0.274 |
BMI (kg/m2) | 23.3±5.8 | 23.6±2.9 | 23.7±3.5 | 23.1±3.7 | 0.369 |
Data are presented as mean ± standard deviation. BMI, body mass index.
Extraction and selection of radiomics features
Following manual segmentation, 1,803 ROIs were delineated in the main cohort, comprising 357 non-calcified plaques (20%), 252 calcified plaques (14%), 325 mixed plaques (18%), and 869 ROIs with no plaques (48%). The external test set consisted of 38 non-calcified plaques (25%), 39 calcified plaques (25%), 36 mixed plaques (24%), and 40 ROIs with no plaques (26%). Table S1 provides an overview of plaque categories for all included patients in this study.
In total, 1,786 radiomics features with ICC >0.75 were extracted from each ROI. The features selected after LASSO varied across different classification tasks. In FCM, 38 features were ultimately chosen, whereas in the first level of TBCM (calcification vs. no calcification), 14 radiomics features were selected. In the second level, 14 features (calcified plaques vs. mixed plaques) and 22 features (non-calcified plaques vs. no plaques) were selected. Figure S1 displays the radiomics features and their corresponding coefficients selected by the three classifiers in LASSO for FCM and TBCM.
Model prediction results in the main cohort
In the internal test cohort, all five machine learning algorithms exhibited outstanding classification performance in both FCM and TBCM. Analysis of the confusion matrix revealed that, excluding the GP model predicting one fewer correct outcome in TBCM than in FCM, the remaining models displayed a higher number of correct predictions in TBCM than in FCM. Therefore, TBCM demonstrated generally superior performance to FCM, indicating a preference toward selecting TBCM for the final model. The confusion matrices of FCM and TBCM for the five machine learning algorithms are illustrated in Figure 3. In TBCM, the GP (with AUCs of 1.000, 0.892, and 0.944 for the three classifiers, respectively) and RF models (with AUCs of 1.000, 0.883, and 0.943 for the three classifiers, respectively) outperformed the other machine learning algorithms, and their AUCs were similar. Therefore, the DeLong test was utilized to assess whether the differences in the ROC curves of the two models and their combined model were statistically significant. A combined model was established using binary logistic regression analysis of the predicted values of the two models. The respective AUCs of the combined model were 1.000, 0.893, and 0.949 for classifiers 1–3, respectively. No statistically significant difference was found in the ROC curves of the GP, RF, and combined models across the three classifiers (P>0.05). Tables S2,S3 present the performance metrics of FCM and TBCM in the internal test cohort, including AUC, sensitivity, specificity, accuracy, precision, and F1 score. The ROC curves of the GP, RF, and combined models of TBCM in the internal test cohort are depicted in Figure 4, with recall, precision, F1 score for each class, and overall accuracy displayed in Table S4.
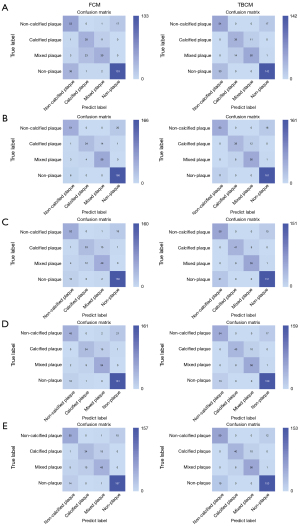
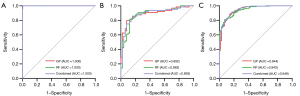
Model prediction results in the external test set
The GP, RF, and combined models of TBCM were externally validated. Similarly, the ROC curves for the three classifiers were compared in the external test set. The AUCs for GP across classifiers 1–3 were 1.000, 0.902, and 0.993, respectively. For RF, the corresponding AUCs were 1.000, 0.939, and 0.998, respectively, and the AUCs for the combined model were 1.000, 0.939, and 0.999, respectively. Table S5 presents the performance metrics of the GP, RF, and combined models of TBCM in the external test set, including AUC, sensitivity, specificity, accuracy, precision, and F1 score. Table S6 displays the results for recall, precision, F1 score for each class, and overall accuracy in the external test set for the GP, RF, and combined models of TBCM. The ROC curves for the GP, RF, and combined models of TBCM in the external test set are depicted in Figure 5. Furthermore, the confusion matrices of the GP, RF, and combined models of TBCM in the external test set are illustrated in Figure 6. No statistically significant differences were found in the ROC curves of the three models across the three classifiers (P>0.05).
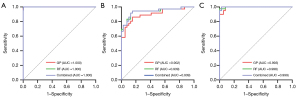
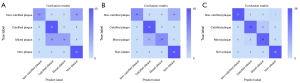
Discussion
This study explored the effectiveness of a radiomics model based on NCCT of the neck in identifying and classifying CAPs. The results revealed that the GP (with AUCs of 1.000, 0.892, and 0.944 for the three classifiers, respectively) and RF models (with AUCs of 1.000, 0.883, and 0.943 for the three classifiers, respectively) of TBCM outperformed the other models, and the differences in the ROC curves between the GP and RF models were not statistically significant, indicating similar predictive performance between the two models. The model combining the GP and RF models displayed improved AUCs of 1.000, 0.893, and 0.949 for classifiers 1–3, respectively. The differences in the ROC curves of the GP, RF, and combined models were not statistically significant, suggesting that all three models have good performance in identifying the composition of CAPs. This study highlighted the efficacy of the radiomics model derived from NCCT images of the neck in discerning the composition of CAPs. It is anticipated to emerge as a novel tool for classifying CAPs, potentially offering an alternative to CTA.
In clinical practice, it is generally easier to diagnose stable calcified plaques because of their higher CT values. However, it can be challenging to diagnose non-calcified plaques with high-risk features because they often display subtle differences from blood on NCCT images. Therefore, calcified plaques are more easily diagnosed on NCCT images than non-calcified plaques. This explains why classifier 1 (presence of calcification) performed better and classifier 3 (non-calcified plaques vs. absence of plaque) is more challenging in TBCM. In clinical practice, although NCCT cannot provide a definitive diagnosis and it only offers information about vascular lesions, CTA is crucial for the accurate diagnosis of vascular diseases. However, more sensitive image analysis techniques can detect subtle differences within the carotid artery lumen, thus extracting distinct radiomics features hidden within images for the construction of machine learning models to differentiate CAPs. Most previous studies primarily focused on the qualitative and quantitative assessment of CAPs using MRI (16-19) or the evaluation of plaque stability by CTA (20,21). Our study is the first to detect and classify CAPs using NCCT images. All developed models demonstrated promising outcomes, highlighting the effectiveness of radiomics features in identifying plaque components on NCCT images. This supports the potential to diagnose vascular diseases without contrast agents. Although NCCT cannot fully replace CTA, utilizing TBCM results for early screening can aid in assessing plaque stability, particularly for patients with iodine allergies or those facing challenges with medical insurance coverage. Moreover, our NCCT-based radiomics model possesses several advantages. First, in addition to the four-category design, we also employed a two-level binary classification approach to simplify the multicategory problem into multiple binary classification tasks. Second, in comparison to the study by Zaccagna et al. (22), which concentrated on CTA-based radiomics for identifying high-risk CAPs, our research incorporated a comprehensive array of radiomics features. Whereas Zaccagna et al. primarily extracted texture features, they overlooked other crucial radiomics features, such as filter transform features. Furthermore, delineating the interactions between radiomics features and identifying the optimal combination for the best performance proved challenging. By contrast, our study successfully extracted a comprehensive set of radiomics features and employed the LASSO algorithm to optimize feature sets tailored to the three classifiers of FCM and TBCM. In addition, previous studies reported a positive correlation between the CT values of blood and hemoglobin concentrations (23). To investigate whether this difference affects omics feature extraction, we compared hemoglobin levels among the four plaque subtypes in the primary cohort and found no statistically significant differences (P>0.05, Table 2). Third, we selected the two best classifiers from the two classification tasks and five machine learning models and subsequently constructed a combined model, thereby enhancing the overall efficiency of the model. Finally, we conducted an external test to validate the generalizability of the combined model, which yielded satisfactory results.
Radiomics employs automated data mining algorithms to convert medical imaging data into high-dimensional quantitative features. First-order statistics can describe the distribution of voxel intensities within an ROI without considering spatial relationships (24). For instance, they can be used to depict the density or variations thereof in CAPs. Two first-order features, namely skewness (measuring the asymmetry of the distribution of means) and the 10th percentile (representing increased plaque density), ranked first in importance for tasks such as distinguishing whether the carotid artery lumen contains calcified components and discriminating between calcified and mixed plaques. This suggests that first-order features make the most significant contribution to classifying calcified and mixed-type plaques. This might be because, even without considering spatial relationships, the locally increased density caused by calcified components in calcified and mixed plaques is sufficient to provide sufficient statistical information about the voxel intensity distribution to distinguish between them. This finding is consistent with that of Yunus et al. (25). Shape features can capture the two-dimensional shape of carotid artery vessel contours. However, our results indicate that the extracted shape features do not provide sufficient information for diagnosing CAPs. Although arteries undergo positive remodeling with the development of atherosclerosis (26), the ROI that we selected is the vessel contour of a specific slice of the carotid artery, and the size of the vessel contour itself varies with its course. Therefore, the influence of plaques on the shape features of carotid artery vessel contours is minimal. Second-order statistical features explore the spatial relationships among voxel contrasts, also referred to as texture features. These encompass GLCM, GLRLM, GLSZM, NGTDM, and GLDM features, which analyze texture variances based on gray-level spatial correlations (27). Our research findings suggest that gldm_dependencevariance and glcm_imc2 exhibit superior diagnostic efficacy in discerning non-calcified plaques from the absence of plaques. This suggests that combining texture features based on voxel spatial distribution improves diagnostic accuracy in classifying non-calcified plaques, potentially revealing underlying pathological changes within these plaques. Furthermore, we extended our investigation to include the entire carotid artery lumen as an ROI to identify the predictive features of CAPs. This departure from previous studies, which focused solely on the plaques themselves, permitted the capture of more comprehensive information beyond the plaque region. This approach acknowledges the potential clinical significance of compensatory changes occurring in the surrounding blood and vessel walls.
This study had several limitations. First, various studies demonstrated that the variability of CT scanners, reconstruction settings, and segmentation methods can influence CT data, consequently influencing radiomics features (28,29). Therefore, larger datasets from multiple centers are necessary to address the heterogeneity of CT acquisition machines. Although our NCCT imaging data were sourced from only two centers, one of which was used for external validation, we achieved relatively satisfactory validation results. Second, this study was retrospective in nature, highlighting the need for prospective studies to ascertain whether the predictive model can serve as a tool to guide clinical decisions. Third, because of the numerous slices in NCCT images and time constraints, we did not utilize three-dimensional segmentation methods, potentially resulting in the loss of valuable three-dimensional information. However, our radiomics analysis was conducted on the slice with the maximum plaque area to comprehensively demonstrate plaque characteristics, minimizing the potential impact of the absence of three-dimensional features on model performance. Fourth, manual segmentation was employed for ROIs in this study, and automation is yet to be implemented. Additionally, plaques with varying degrees of stenosis constitute a significant portion, and they are considered one of the key contributors to stroke (30). Because of the differences between NCCT and CTA, namely that blood vessels are not enhanced on NCCT images, stenosis assessment still relies on CTA. In future model development, we plan to use a knowledge distillation-based plaque recognition framework that leverages CTA images to guide NCCT plaque detection. This approach will transfer CTA image features to NCCT, enabling the creation of a CTA-generative model based on NCCT. This model will facilitate automatic vessel segmentation, overcoming the limitations of manual segmentation. Additionally, we will calculate stenosis levels in various vessel segments using centerline extraction, image rendering, and automated stenosis detection models and integrate these findings with TBCM. Our future goal is to achieve carotid plaque screening and assessment in a single NCCT scan.
Conclusions
In summary, NCCT, as a widely utilized non-invasive diagnostic modality, presents several advantages, including cost-effectiveness, rapid acquisition time, and the absence of contrast agent requirements. These attributes render NCCT particularly suitable for large-scale screening and routine clinical evaluations. This study demonstrated that machine learning models leveraging radiomics features derived from neck NCCT exhibit exceptional performance in identifying the composition of CAPs. Furthermore, our findings indicate that first-order features contribute most significantly to distinguishing between calcified and mixed plaques, whereas second-order features serve as potential predictive markers for the diagnosis of non-calcified plaques. Additionally, the extracted shape features were not found to provide substantial diagnostic value in the assessment of CAPs. Therefore, it is evident that the characterization of carotid plaques must extend beyond visual assessment. Radiomics models based on NCCT offer extensive quantitative data, augmenting current diagnostic and classification capabilities. This approach holds considerable promise as an advanced tool for carotid plaque classification and may serve as a viable alternative to CTA.
Acknowledgments
We thank Medjaden Inc. for scientific editing of this manuscript.
Footnote
Reporting Checklist: The authors have completed the TRIPOD + AI reporting checklist. Available at https://qims.amegroups.com/article/view/10.21037/qims-24-1974/rc
Funding: This study received funding support from
Conflicts of Interest: All authors have completed the ICMJE uniform disclosure form (available at https://qims.amegroups.com/article/view/10.21037/qims-24-1974/coif). E.X. and A.L. are employees of Shanghai United Imaging Intelligence Co., Ltd. The other authors have no conflicts of interest to declare.
Ethical Statement: The authors are accountable for all aspects of the work in ensuring that questions related to the accuracy or integrity of any part of the work are appropriately investigated and resolved. The study was conducted in accordance with the Declaration of Helsinki and its subsequent amendments. The study was approved by the ethics committee of The First Affiliated Hospital of Ningbo University (approval No. 057A). The requirement for informed consent was waived because of the retrospective nature of the study.
Open Access Statement: This is an Open Access article distributed in accordance with the Creative Commons Attribution-NonCommercial-NoDerivs 4.0 International License (CC BY-NC-ND 4.0), which permits the non-commercial replication and distribution of the article with the strict proviso that no changes or edits are made and the original work is properly cited (including links to both the formal publication through the relevant DOI and the license). See: https://creativecommons.org/licenses/by-nc-nd/4.0/.
References
- Global, regional, and national burden of stroke and its risk factors, 1990-2019: a systematic analysis for the Global Burden of Disease Study 2019. Lancet Neurol 2021;20:795-820. [Crossref] [PubMed]
- Baradaran H, Gupta A. Carotid Vessel Wall Imaging on CTA. AJNR Am J Neuroradiol 2020;41:380-6. [Crossref] [PubMed]
- Rothwell PM, Eliasziw M, Gutnikov SA, Fox AJ, Taylor DW, Mayberg MR, Warlow CP, Barnett HJ. Analysis of pooled data from the randomised controlled trials of endarterectomy for symptomatic carotid stenosis. Lancet 2003;361:107-16. [Crossref] [PubMed]
- Miceli G, Basso MG, Pintus C, Pennacchio AR, Cocciola E, Cuffaro M, Profita M, Rizzo G, Tuttolomondo A. Molecular Pathways of Vulnerable Carotid Plaques at Risk of Ischemic Stroke: A Narrative Review. Int J Mol Sci 2024;25:4351. [Crossref] [PubMed]
- Weng ST, Lai QL, Cai MT, Wang JJ, Zhuang LY, Cheng L, Mo YJ, Liu L, Zhang YX, Qiao S. Detecting vulnerable carotid plaque and its component characteristics: Progress in related imaging techniques. Front Neurol 2022;13:982147. [Crossref] [PubMed]
- Henein MY, Vancheri S, Longo G, Vancheri F. The Role of Inflammation in Cardiovascular Disease. Int J Mol Sci 2022;23:12906. [Crossref] [PubMed]
- Saba L, Agarwal N, Cau R, Gerosa C, Sanfilippo R, Porcu M, Montisci R, Cerrone G, Qi Y, Balestrieri A, Lucatelli P, Politi C, Faa G, Suri JS. Review of imaging biomarkers for the vulnerable carotid plaque. JVS Vasc Sci 2021;2:149-58. [Crossref] [PubMed]
- Zhang R, Zhang Q, Ji A, Lv P, Zhang J, Fu C, Lin J. Identification of high-risk carotid plaque with MRI-based radiomics and machine learning. Eur Radiol 2021;31:3116-26. [Crossref] [PubMed]
- Chiu TM, Chu SY. Hypersensitivity Reactions to Iodinated Contrast Media. Biomedicines 2022;10:1036. [Crossref] [PubMed]
- Cao K, Xia Y, Yao J, Han X, Lambert L, Zhang T, et al. Large-scale pancreatic cancer detection via non-contrast CT and deep learning. Nat Med 2023;29:3033-43. [Crossref] [PubMed]
- Hoye J, Solomon JB, Sauer TJ, Samei E. Quantification of Minimum Detectable Difference in Radiomics Features Across Lesions and CT Imaging Conditions. Acad Radiol 2021;28:1570-81. [Crossref] [PubMed]
- Kolossváry M, De Cecco CN, Feuchtner G, Maurovich-Horvat P. Advanced atherosclerosis imaging by CT: Radiomics, machine learning and deep learning. J Cardiovasc Comput Tomogr 2019;13:274-80. [Crossref] [PubMed]
- Lao Q, Zhou R, Wu Y, Feng C, Pang J, Ma L, Yang Y, Ji W. Predicting Vulnerability Status of Carotid Plaques Using CTA-Based Quantitative Analysis. J Cardiovasc Pharmacol 2025;85:217-24. [Crossref] [PubMed]
- Wu J, Xia Y, Wang X, Wei Y, Liu A, Innanje A, Zheng M, Chen L, Shi J, Wang L, Zhan Y, Zhou XS, Xue Z, Shi F, Shen D. uRP: An integrated research platform for one-stop analysis of medical images. Front Radiol 2023;3:1153784. [Crossref] [PubMed]
- Fu F, Shan Y, Yang G, Zheng C, Zhang M, Rong D, Wang X, Lu J. Deep Learning for Head and Neck CT Angiography: Stenosis and Plaque Classification. Radiology 2023;307:e220996. [Crossref] [PubMed]
- Zhang X, Hua Z, Chen R, Jiao Z, Shan J, Li C, Li Z. Identifying vulnerable plaques: A 3D carotid plaque radiomics model based on HRMRI. Front Neurol 2023;14:1050899. [Crossref] [PubMed]
- Chen S, Liu C, Chen X, Liu WV, Ma L, Zha Y. A Radiomics Approach to Assess High Risk Carotid Plaques: A Non-invasive Imaging Biomarker, Retrospective Study. Front Neurol 2022;13:788652. [Crossref] [PubMed]
- Gui C, Cao C, Zhang X, Zhang J, Ni G, Ming D. Radiomics and artificial neural networks modelling for identification of high-risk carotid plaques. Front Cardiovasc Med 2023;10:1173769. [Crossref] [PubMed]
- Cademartiri F, Balestrieri A, Cau R, Punzo B, Cavaliere C, Maffei E, Saba L. Insight from imaging on plaque vulnerability: similarities and differences between coronary and carotid arteries-implications for systemic therapies. Cardiovasc Diagn Ther 2020;10:1150-62. [Crossref] [PubMed]
- Dong Z, Zhou C, Li H, Shi J, Liu J, Liu Q, Su X, Zhang F, Cheng X, Lu G. Radiomics versus Conventional Assessment to Identify Symptomatic Participants at Carotid Computed Tomography Angiography. Cerebrovasc Dis 2022;51:647-54. [Crossref] [PubMed]
- Cilla S, Macchia G, Lenkowicz J, Tran EH, Pierro A, Petrella L, Fanelli M, Sardu C, Re A, Boldrini L, Indovina L, De Filippo CM, Caradonna E, Deodato F, Massetti M, Valentini V, Modugno P. CT angiography-based radiomics as a tool for carotid plaque characterization: a pilot study. Radiol Med 2022;127:743-53. [Crossref] [PubMed]
- Zaccagna F, Ganeshan B, Arca M, Rengo M, Napoli A, Rundo L, Groves AM, Laghi A, Carbone I, Menezes LJ. CT texture-based radiomics analysis of carotid arteries identifies vulnerable patients: a preliminary outcome study. Neuroradiology 2021;63:1043-52. [Crossref] [PubMed]
- Gillies RJ, Kinahan PE, Hricak H. Radiomics: Images Are More than Pictures, They Are Data. Radiology 2016;278:563-77. [Crossref] [PubMed]
- Decker JA, Huber A, Senel F, Bette S, Braun F, Risch F, Woźnicki P, Becker J, Popp D, Haerting M, Jehs B, Rippel K, Wollny C, Scheurig-Muenkler C, Kroencke TJ, Schwarz F. Anemia Detection by Hemoglobin Quantification on Contrast-enhanced Photon-counting CT Data Sets. Radiology 2022;305:650-2. [Crossref] [PubMed]
- Yunus MM, Mohamed Yusof AK, Ab Rahman MZ, Koh XJ, Sabarudin A, Nohuddin PNE, Ng KH, Kechik MMA, Karim MKA. Automated Classification of Atherosclerotic Radiomics Features in Coronary Computed Tomography Angiography (CCTA). Diagnostics (Basel) 2022.
- Watase H, Sun J, Hippe DS, Balu N, Li F, Zhao X, Mani V, Fayad ZA, Fuster V, Hatsukami TS, Yuan C. Carotid Artery Remodeling Is Segment Specific: An In Vivo Study by Vessel Wall Magnetic Resonance Imaging. Arterioscler Thromb Vasc Biol 2018;38:927-34. [Crossref] [PubMed]
- Koçak B, Durmaz EŞ, Ateş E, Kılıçkesmez Ö. Radiomics with artificial intelligence: a practical guide for beginners. Diagn Interv Radiol 2019;25:485-95. [Crossref] [PubMed]
- Shiri I, Rahmim A, Ghaffarian P, Geramifar P, Abdollahi H, Bitarafan-Rajabi A. The impact of image reconstruction settings on 18F-FDG PET radiomic features: multi-scanner phantom and patient studies. Eur Radiol 2017;27:4498-509. [Crossref] [PubMed]
- Altazi BA, Zhang GG, Fernandez DC, Montejo ME, Hunt D, Werner J, Biagioli MC, Moros EG. Reproducibility of F18-FDG PET radiomic features for different cervical tumor segmentation methods, gray-level discretization, and reconstruction algorithms. J Appl Clin Med Phys 2017;18:32-48. [Crossref] [PubMed]
- Huang Z, Cheng XQ, Lu RR, Bi XJ, Liu YN, Deng YB. Incremental Prognostic Value of Carotid Plaque-RADS Over Stenosis Degree in Relation to Stroke Risk. JACC Cardiovasc Imaging 2025;18:77-89. [Crossref] [PubMed]