Identification of intrinsic imaging subtypes using clustering analysis based on dynamic contrast-enhanced magnetic resonance imaging radiomics features for gliomas: preliminary associations with gene expression profiles
Introduction
Diffuse glioma in adults is the most common malignant tumor of the central nervous system (CNS) (1). Due to the heterogeneity of histological subtypes and gene profiles, glioma has a complex immune microenvironment, which can inhibit immune cell function and bring great challenges to treatment (2). Even though there are many treatment methods for glioma, including surgery, chemoradiotherapy, and immunotherapy, among others, its highly aggressive nature truncates the overall survival of glioma patients (3). Overall survival is largely dependent on the genetic and molecular typing of gliomas, which requires surgical and pathological biopsy, representing an invasive means of assessment. The 2021 World Health Organization (WHO) Classification of CNS Tumors has unique insights into the genetic and molecular typing of gliomas, and has expanded the diagnosis of gliomas by molecular detection (4). These innovations can lead to more accurate diagnostic and prognostic information, enabling the selection of optimal treatment. Therefore, there is an urgent need for a reliable and non-invasive technique that can accurately predict glioma genotyping at an early stage to improve patient prognosis.
Conventional imaging techniques are crucial for providing information regarding anatomical location and detailed morphological characteristics. However, there is controversy regarding the diagnosis of gliomas with atypical imaging features using morphological imaging, and their interpretation remains largely visual and subjective, relying on the experience of neuroradiologists. Additionally, for tumors that are not histologically but only molecularly defined [telomerase reverse transcriptase (TERT), epidermal growth factor receptor (EGFR), chromosomes +7/−10], for which imaging remains relatively unknown, morphological imaging is often unreliable (5). Advanced perfusion magnetic resonance imaging (MRI) technology such as dynamic susceptibility contrast perfusion-weighted imaging (DSC-PWI) and dynamic contrast-enhanced (DCE) can reflect the internal pathophysiological characteristics of tumors, including tumor microvascular proliferation and vascular wall permeability, and more truly reflect the tumor microenvironment. In recent years, stratified diagnosis of gliomas has relied on the application of predictive imaging models or parameters to noninvasively assess tumor molecular genotypes based on quantitative imaging biomarkers, providing potential benefits for personalized and effective treatment plans (6). Previous research has demonstrated the potential of DSC-PWI in non-invasively distinguishing between isocitrate dehydrogenase (IDH) mutant grade 4 astrocytoma and IDH-wildtype glioblastoma preoperatively (7). DSC-PWI also holds potential value in differentiating between astrocytoma and oligodendroglioma (8). A systematic review and meta-analysis indicated that DCE has high accuracy in preoperative stratification of gliomas, but it is not commonly used in clinical practice, mainly due to the limitations of post-processing analysis (9).
With the rapid development of artificial intelligence, radiomics and neural network have emerged as important tools for imaging analysis (10-12). Radiomics extracts high-throughput quantifiable information from radiological images which, when combined with machine learning, can identify internal tumor heterogeneity for potential disease prognosis and prediction of response to therapy (13-15). The application of radiomics in the genotyping of gliomas has been established, but most previous studies have focused on supervised machine learning to construct a prediction model (16-18). Also, the radiomics features reflect the intrinsic imaging profile of the gliomas; whether the profiles are meaningful should be tested using unsupervised clustering analysis.
In this study, we aimed to identify the internal image subtypes of glioma by multi-radiomic clustering method based on DCE-MRI, and to explore the correlation between subtypes of glioma and gene expression profiles, so as to extract the internal characteristics of tumors in a non-invasive and comprehensive way, and obtain accurate preoperative diagnosis. We present this article in accordance with the STROBE reporting checklist (available at https://qims.amegroups.com/article/view/10.21037/qims-24-1459/rc).
Methods
Participants enrollment
This retrospective study was conducted in accordance with the Declaration of Helsinki and its subsequent amendments, and was approved by the Ethical Committee of Nanjing Drum Tower Hospital (approval No. NCT05624736). The requirement for individual consent for this retrospective analysis was waived. Patients in this retrospective and consecutive study were recruited from January 2018 to October 2023 from Nanjing Drum Tower Hospital. The inclusion criteria were as follows: (I) patients (>18 years old) diagnosed with WHO grade 2–4 adult diffuse glioma by surgical pathology or stereotactic biopsy; (II) complete preoperative image data [T1-weighted imaging (T1WI), T2-weighted imaging (T2WI), fluid-attenuated inversion recovery (FLAIR), T1 contrast-enhanced (T1CE), and DCE-MRI] and pathological data. The exclusion criteria were as follows: (I) patients lacking pathological diagnosis and molecular detection; (II) poor DCE image quality due to serious image artifacts; (III) preoperative treatment or recurrent glioma; (IV) accompanied by concomitant neurological disorders, such as cerebral infarction or hemorrhage.
Histopathologic analysis
All surgical tissues were histopathologically diagnosed according to the 2021 WHO classification of CNS tumors, and the tumors before 2021 were re-classified according to the new classification criteria. Ki-67 label index (LI) was determined by immunohistochemical staining. The results were interpreted based on the percentage of immunoreactive positive nuclei per 500–1,000 cells in the regions with the highest staining density of the tumor, and the positive cells were brown-yellow nuclei. Ki-67 LI was negatively correlated with survival, and the cut-off value was 20% (19). Other molecular makers were detected by polymerase chain reaction (PCR), DNA sequencing, or fluorescence in situ hybridization (FISH) (20).
Image acquisition, image processing, and tumor delineation
All the MRI measurements were performed with a 3.0 T scanner (uMR 770, United Imaging Healthcare, Shanghai, China) and a 32-channel head coil. The clinically scanning sequences included T1WI, T2WI, FLAIR, T1CE, and DCE. The contrast agent used was Gadodiamide Injection (Omniscan, GE HealthCare, Chicago, IL, USA), administered at an injection rate of 3.5 mL/s and a dosage of 0.2 mL/kg. The DCE sequence included the following parameters: repetition time/echo time (3.47 ms/1.9 ms), field of view (240×220 mm2), slice thickness (5 mm), and flip angle (13°). The contrast sequence consisted of 90 dynamic scans, with a temporal resolution of 4 seconds. The total duration time was 6 minutes.
Standardized pre-processing was assessed on T1CE and T2WI. The applied pre-processing routines included the following: (I) image resampling with linear interpolation; (II) rigid registration to T2WI; and (III) skull-stripping using the Brain Extraction Tool (BET) and a convolutional neural network model. A two-stage optimal mass transport (TSOMT) (21) technique is used to transform raw images into 2×1283 tensors for T1CE and T2WI. Volumes of interest (VOIs) on the tensor were predicted by inputting the tensors into a pre-trained SegResUnet model. Then, the inverse map of two-stage optimal mass transport technique was used to obtain VOIs on T1CE or T2WI. Finally, we registered the VOIs to the DCE-MRI through the projection registration algorithm to obtain the VOIs on DCE-MRI (Figure 1).
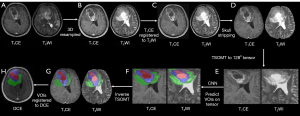
Feature extraction and dynamic feature calculation
To extract the static radiomics features, DCE images at three time points (0, 90, and 300 s) representing the pre-contrast, arterial, and delayed phases, respectively, were utilized. The radiomics feature extraction was performed using the well-established Pyradiomics package (version 3.0.1, available at https://pyradiomics.readthedocs.io/en/latest/). The extraction process encompassed preprocessing and feature computation steps. Preprocessing involved resampling the images to a uniform 1×1×1 mm³ resolution for rotational invariance, discretizing gray values with a bin width of 10 to reduce computational complexity, and normalizing the images using the µ±3σ method to eliminate outlier gray values. Further details on the preprocessing steps can be found in the supplementary file (Appendix 1). Subsequently, radiomics features were computed, resulting in a total of 54 radiomics features being derived.
After that, we introduced a novel dynamic radiomics feature termed temporal anisotropy feature, aimed at capturing the variance of radiomics features across different time points. The formula for this feature was as follows (Eq. [1]):
Where , , and are radiomics features from 3 phases, respectively. is the mean value of the three radiomics features. Totally, we obtained four sets of radiomics features, three of which were static radiomics features and one was dynamic radiomics features.
Clustering analysis
The MOVICS package was employed for conducting clustering analysis (22). Initially, the optimal number of clusters, denoted as k, was determined by testing values ranging from 2 to 8. Subsequently, the Clustering Prediction Index (CPI) was computed to identify the optimal k value corresponding to the maximum CPI value. Following this, 10 advanced multi-omics clustering algorithms, CIMLR, iClusterBayes, MoCluster, COCA, Consensus Clustering, IntNMF, LRAcluster, NEMO, PINSPlus, and SNF, were executed to cluster the dataset using the optimized k. By synthesizing the results from the 10 clustering analyses, a consensus clustering matrix was derived, then a hierarchical clustering was conducted to obtain a stable clustering result (Figure 2). This matrix facilitated the determination of the final clustering outcome, enabling the allocation of patients to their respective clusters, and thereby assigned the patients into different phenotypes.
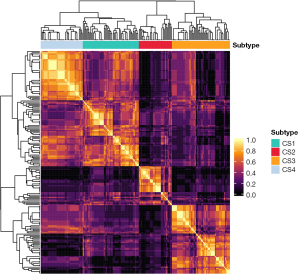
Statistical analyses
The statistical analyses were conducted utilizing R software (version 4.2.1, https://cran.rproject.org) to investigate the associations between radiomics subtypes and clinicopathological information, as well as gene profiles. The clinical characteristics of the ultimate clusters were compared using Fisher’s exact test or the χ2 test for categorical variables, and the Kruskal-Wallis test for continuous data, with a significance level set at P<0.05 indicating a significant difference.
Results
Participant characteristics
Of 250 patients identified with glioma, a total of 189 met the inclusion criteria (61 were excluded) (Figure 3). The median patient age was 53 years [interquartile range (IQR), 51–55 years], and 99 (52.38%) patients were men and 90 (47.62%) were women. Most patients were grade 4 [n=122 (64.55%)], followed by grade 2 [n=45 (23.81%)] and grade 3 [n=22 (11.64%)]. There were 121 patients with high Ki-67 level [n=121 (64.02%)], and 65 patients with low Ki-67 level [n=65 (34.39%)]. According to 2021 WHO Classification of CNS tumor, our results demonstrated that most patients were glioblastoma, IDH wild-type [n=98 (51.85%)], 46 (24.34%) patients were astrocytoma, IDH mutant and 18 (9.52%) patients were oligodendroglioma, IDH mutant, 1p/19q codeleted. The remaining samples [n=27 (14.29%)] were not otherwise specified. The proportion of O6-methylguanine-DNA methyltransferase (MGMT) promoter unmethylation [n=54 (28.57%)] was slightly higher than that of methylation [n=53 (28.04%)]. Among patients, EGFR non-amplification was present in 46 (24.34%) samples, whereas EGFR amplification was present in 31 (16.40%) samples. In addition, EGFR non-mutant was present in 46 (24.34%) samples whereas mutant type was present in only 9 (4.76%) samples. The proportion of patients without cyclin-dependent kinase inhibitor (CDKN) 2A/B homozygous deletion [n=25 (13.23%)] was higher than that of those with deletion [n=15 (7.94%)]. Similarly, the other most frequent molecular markers were 1p19q non-codeleted (n=144 (76.19%)], TERT mutant [n=49 (25.93%)], phosphatase and tensin homolog (PTEN) non-mutant [n=37 (19.58%)], P53 mutant [n=92 (48.68%)], and alpha thalassemia retardation syndrome X-linked (ATRX) non-mutant [n=39 (20.63%)]. There were 11 (5.82%) samples with chromosomes +7/−10 (Table 1).
Table 1
Characteristics | Value (n=189) |
---|---|
Gender | |
Male | 99 (52.38) |
Female | 90 (47.62) |
Age (years), median [IQR] | 53 [51–55] |
Grade | |
G2 | 45 (23.81) |
G3 | 22 (11.64) |
G4 | 122 (64.55) |
Ki-67 | |
≥20% | 121 (64.02) |
<20% | 65 (34.39) |
Unknown | 3 (1.59) |
Integrated classification | |
Glioblastoma, IDH-wild type | 98 (51.85) |
Astrocytoma, IDH-mutant | 46 (24.34) |
Oligodendroglioma, IDH-mutant, 1p/19q codeleted | 18 (9.52) |
NOS | 27 (14.29) |
IDH | |
Wild-type | 101 (53.44) |
Mutant | 73 (38.62) |
Unknown | 15 (7.94) |
lp19q | |
Non-codeleted | 144 (76.19) |
Codeleted | 21 (11.11) |
Unknown | 24 (12.70) |
MGMT | |
Unmethylation | 54 (28.57) |
Methylation | 53 (28.04) |
Unknown | 82 (43.39) |
TERT | |
Wild-type | 42 (22.22) |
Mutant | 49 (25.93) |
Unknown | 98 (51.85) |
EGFRp | |
Non-amplification | 46 (24.34) |
Amplification | 31 (16.40) |
Unknown | 112 (59.26) |
EGFRm | |
Non-mutant | 46 (24.34) |
Mutant | 9 (4.76) |
Unknown | 134 (70.90) |
PTEN | |
Non-mutant | 37 (19.58) |
Mutant | 15 (7.94) |
Unknown | 137 (72.48) |
P53 | |
Wild-type | 56 (29.63) |
Mutant | 92 (48.68) |
Unknown | 41 (21.69) |
ATRX | |
Non-mutant | 39 (20.63) |
Mutant | 14 (7.41) |
Unknown | 136 (71.96) |
CDKN 2A/B | |
Without deletion | 25 (13.23) |
Deletion | 15 (7.94) |
Unknown | 149 (78.83) |
Chromosomes +7/−10 | |
Yes | 11 (5.82) |
No | 18 (9.52) |
Unknown | 160 (84.66) |
Unless otherwise indicated, data are numbers of patients and data in parentheses are percentages. ATRX, alpha thalassemia retardation syndrome X-linked; CDKN, cyclin-dependent kinase inhibitor; EGFRm, epidermal growth factor receptor mutant status; EGFRp, epidermal growth factor receptor amplification status; IDH, isocitrate dehydrogenase; IQR, interquartile range; MGMT, O6-methylguanine-DNA methyltransferase; NOS, not otherwise specified; PTEN, phosphatase and tensin homolog; TERT, telomerase reverse transcriptase.
Consensus clustering associations
As shown in Figure 4, when k=4, the CPI value was the largest, thus, four was chosen as the optimized number of clusters. After clustering analysis, 56 patients were assigned to cluster 1, 33 to cluster 2, 58 to cluster 3, and the remaining patients (n=42) to cluster 4. As shown in Figure 5, the radiomics feature distribution heatmap displayed significantly different values. Demographic, grading, and immunohistochemical markers characteristics by cluster are displayed in Table 2. The patients in cluster 3 were significantly older (57.98±12.55, P=0.002), compared with those in cluster 1 (53.43±14.1), cluster 2 (48.82±15.73), and cluster 4 (49.05±11.6). Cluster 3 and cluster 1 had higher frequencies of grade 4 [51 of 58 (87.93%) and 35 of 56 (62.50%), respectively] compared with cluster 2, which had a higher frequency of grade 2 [13 of 33 (39.40%)] and cluster 4, which had a higher frequency of grade 3 [8 of 42 (19.05%)] (P<0.001). Likewise, the frequency of high Ki-67 level was highest in cluster 3 [49 of 58 (84.48%)], and cluster 2 had the highest frequency of low Ki-67 level [16 of 33 (48.48%)] (P=0.001). Cluster 1 had a higher frequency of high Ki-67 level [32 of 56 (57.14%)], compared with cluster 2 and cluster 4. There was no significant difference in gender between clusters (P=0.37).
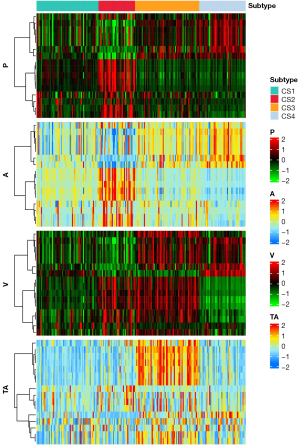
Table 2
Variable | Cluster =1 (n=56) | Cluster =2 (n=33) | Cluster =3 (n=58) | Cluster =4 (n=42) | P value |
---|---|---|---|---|---|
Gender | 0.37 | ||||
Male | 30 (53.57) | 13 (39.39) | 34 (58.62) | 22 (52.38) | |
Female | 26 (46.43) | 20 (60.61) | 24 (41.38) | 20 (47.62) | |
Age (years) | 53.43±14.1 | 48.82±15.73 | 57.98±12.55 | 49.05±11.6 | 0.002 |
Grade | <0.001 | ||||
G2 | 13 (23.21) | 13 (39.40) | 6 (10.35) | 13 (30.95) | |
G3 | 8 (14.29) | 5 (15.15) | 1 (1.72) | 8 (19.05) | |
G4 | 35 (62.50) | 15 (45.45) | 51 (87.93) | 21 (50.00) | |
Ki-67 | 0.001 | ||||
≥20% | 32 (57.14) | 17 (51.52) | 49 (84.48) | 23 (54.76) | |
<20% | 24 (42.86) | 16 (48.48) | 9 (15.52) | 19 (45.24) |
Data are presented as mean ± standard deviation or n (%). Cluster characteristics were compared using ANOVA. Associations between radiomic clusters and clinicopathologic or genomic variables are significant (P<0.05). ANOVA, analysis of variance.
Cluster analysis in the molecular markers (Table 3) showed that cluster 3 and cluster 1 had higher frequencies of glioblastoma IDH wild-type [43 of 58 (74.14%) and 27 of 56 (48.21%), respectively; P=0.022]. Cluster 4 and cluster 2 had higher frequencies of astrocytoma IDH-mutant [14 of 42 (33.33%) and 11 of 33 (33.33%), respectively; P=0.022]. Cluster 4 had the highest frequency of oligodendroglioma IDH-mutant and 1p/19q codeleted [6 of 42 (14.29%); P=0.022]. Similarly, cluster 3 and cluster 1 had higher proportions of MGMT promoter unmethylation [23 of 36 (63.89%) and 21 of 34 (61.76%), respectively; P=0.005], whereas cluster 4 had a higher frequency of MGMT promoter methylation [16 of 21 (76.19%); P=0.005]. Cluster 4 had the highest frequency of EGFR non-amplification [13 of 16 (81.25%); P=0.003], whereas cluster 3 had the highest frequency of EGFR amplification [18 of 26 (69.23%); P=0.003] and EGFR mutant [7 of 16 (43.75%); P=0.005]. Cluster 1 had the highest frequency of EGFR non-mutant [16 of 16 (100%)]. In addition, cluster 2 had a higher frequency of patients without CDKN 2A/B homozygous deletion [7 of 8 (87.50%); P=0.01]. Cluster 3 and cluster 1 had higher proportions of CDKN 2A/B homozygous deletion [9 of 12 (75.00%) and 4 of 15 (26.67%), respectively]. There were no associations for 1p19q (P=0.26), TERT (P=0.25), PTEN (P=0.07), P53 (P=0.99), ATRX (P=0.08), and chromosomes +7/−10 (P=0.74) between clusters. Figure 6 shows the representative cases of the four clusters.
Table 3
Variable | Cluster =1 (n=56) | Cluster =2 (n=33) | Cluster =3 (n=58) | Cluster =4 (n=42) | P value |
---|---|---|---|---|---|
Integrated classification | 0.022 | ||||
Glioblastoma, IDH-wildtype | 27 (48.21) | 13 (39.39) | 43 (74.14) | 15 (35.71) | |
Astrocytoma, IDH-mutant | 14 (25.00) | 11 (33.33) | 7 (12.07) | 14 (33.33) | |
Oligodendroglioma, IDH-mutant, 1p/19q codeleted | 6 (10.71) | 4 (12.12) | 2 (3.44) | 6 (14.29) | |
NOS | 9 (16.08) | 5 (15.16) | 6 (10.35) | 7 (16.67) | |
IDH | <0.001 | ||||
Wild-type | 28 (54.90) | 13 (44.83) | 44 (81.48) | 16 (40.0) | |
Mutant | 23 (45.10) | 16 (55.17) | 10 (18.52) | 24 (60.0) | |
Missing | 5 | 4 | 4 | 2 | |
lp19q | 0.26 | ||||
Non-codeleted | 41 (85.42) | 24 (85.71) | 50 (94.34) | 29 (80.56) | |
Codeleted | 7 (14.58) | 4 (14.29) | 3 (5.66) | 7 (19.44) | |
Missing | 8 | 5 | 5 | 6 | |
MGMT | 0.005 | ||||
Unmethylation | 21 (61.76) | 5 (31.25) | 23 (63.89) | 5 (23.81) | |
Methylation | 13 (38.24) | 11 (68.75) | 13 (36.11) | 16 (76.19) | |
Missing | 22 | 17 | 22 | 21 | |
TERT | 0.25 | ||||
Wild-type | 17 (56.67) | 9 (56.25) | 10 (34.48) | 6 (37.50) | |
Mutant | 13 (43.33) | 7 (43.75) | 19 (65.52) | 10 (62.50) | |
Missing | 26 | 17 | 29 | 26 | |
PTEN | 0.07 | ||||
Non-mutant | 12 (75.0) | 7 (77.78) | 7 (46.67) | 11 (91.67) | |
Mutant | 4 (25.0) | 2 (22.22) | 8 (53.33) | 1 (8.33) | |
Missing | 40 | 24 | 43 | 30 | |
EGFRp | 0.003 | ||||
Non-amplification | 16 (69.57) | 9 (75.00) | 8 (30.77) | 13 (81.25) | |
Amplification | 7 (30.43) | 3 (25.00) | 18 (69.23) | 3 (18.75) | |
Missing | 33 | 21 | 32 | 26 | |
EGFRm | 0.005 | ||||
Non-mutant | 16 (100.0) | 9 (90.00) | 9 (56.25) | 12 (92.31) | |
Mutant | 0 (0.00) | 1 (10.00) | 7 (43.75) | 1 (7.69) | |
Missing | 40 | 23 | 42 | 29 | |
P53 | 0.99 | ||||
Wild-type | 16 (36.36) | 9 (37.50) | 18 (40.0) | 13 (37.14) | |
Mutant | 28 (63.64) | 15 (62.50) | 27 (60.0) | 22 (62.86) | |
Missing | 12 | 9 | 13 | 7 | |
ATRX | 0.08 | ||||
Non-mutant | 8 (50.00) | 8 (88.89) | 12 (80.00) | 11 (84.62) | |
Mutant | 8 (50.00) | 1 (11.11) | 3 (20.00) | 2 (15.38) | |
Missing | 40 | 24 | 43 | 29 | |
CDKN 2A/B | 0.01 | ||||
Without deletion | 11 (73.33) | 7 (87.50) | 3 (25.00) | 4 (80.00) | |
Deletion | 4 (26.67) | 1 (12.50) | 9 (75.00) | 1 (20.00) | |
Missing | 41 | 25 | 46 | 37 | |
Chromosomes +7/−10 | 0.74 | ||||
Yes | 3 (30.00) | 2 (28.57) | 5 (50.00) | 1 (50.00) | |
No | 7 (70.00) | 5 (71.43) | 5 (50.00) | 1 (50.00) | |
Missing | 46 | 26 | 48 | 40 |
Data are numbers of patients and data in parentheses are percentages. Cluster characteristics were compared using ANOVA. ANOVA, analysis of variance; ATRX, alpha thalassemia retardation syndrome X-linked; CDKN, cyclin-dependent kinase inhibitor; EGFRm, epidermal growth factor receptor mutant status; EGFRp, epidermal growth factor receptor amplification status; IDH, isocitrate dehydrogenase; MGMT, O6-methylguanine-DNA methyltransferase; NOS, not otherwise specified; PTEN, phosphatase and tensin homolog; TERT, telomerase reverse transcriptase.
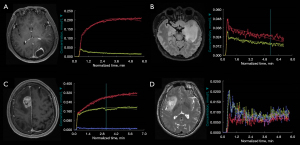
Discussion
The objective of this study was to identify the intrinsic imaging subtypes of gliomas based on multi-phase and temporal anisotropic dynamic radiomics features, investigate the correlation of key gene expression profiles among clusters, and provide a potential method for predicting glioma gene expression before surgery. We found no significant results in gender between clusters. Within four clusters, there was no evidence of association with 1p19q codeleted, TERT, PTEN, P53, ATRX mutant, and chromosomes +7/−10, likely because of the data deviation or pathological molecular detection error.
In our study, cluster 3 was associated with the most aggressive subtype, with the tumor subtype primarily consisting of glioblastoma, IDH wild-type. It is well known that this tumor subtype has the poorest prognosis, with a survival period of only about 15–18 months. Studies have indicated that Ki-67 LI is negatively correlated with survival. In IDH wild-type gliomas, Ki-67 LI is predominantly high, with tumor grade positively correlating with malignancy and thus indicating a poorer prognosis for patients (23). Previous studies have observed that EGFR amplification predominantly occurs in high-grade gliomas, which is also a phenomenon mainly found in IDH wild-type gliomas, with rare occurrences in IDH mutant gliomas (24), which is congruent with our results. MGMT promoter methylation can serve as an independent prognostic factor in glioblastoma, indicating a longer survival. In cluster 3, the majority of tumors are MGMT-unmethylated, which similarly suggests a poor prognosis for this cluster.
In addition, our results indicated that oligodendroglioma, IDH-mutant, and 1p/19q codeleted was representative in cluster 4, providing valuable reference for the early differential diagnosis of oligodendroglioma and astrocytoma, which remains an unresolved challenge in the field of CNS tumors. This tumor subtype is associated with a good response to treatment and is relatively sensitive to radiotherapy and alkylating agents (25). The predominance of MGMT promoter methylation in cluster 4 further supported this finding.
Another tumor subtype, astrocytoma IDH-mutant, showed a similar proportion in both cluster 2 and cluster 4, and our study was unable to make a clear classification. Astrocytoma IDH-mutant grade 4 has been less extensively studied compared to its grade 2–3 counterparts, remaining a major radiological challenge. IDH mutation in WHO grade 4 astrocytoma is associated with CDKN 2A/B deletion, which suggests a poorer prognosis. Recent studies have highlighted the potential of advanced non-invasive MRI techniques in accurately predicting CDKN 2A/B homozygous deletion status, thereby aiding in clinical decision-making (26,27). Our study showed that the proportion of CDKN 2A/B codeleted and MGMT promoter methylation was higher in cluster 4 compared to cluster 2. Our study has not found any significant clinical specificity for cluster 1. Based on the results, we hypothesized that the overall malignancy of tumors in cluster 1 was likely to fall between cluster 3 and the other two clusters.
The current gold standard for the stratified diagnosis and molecular classification of gliomas remains pathological examination. The first-line molecular testing includes immunohistochemistry and FISH. However, DNA sequencing is the preferred method for detecting atypical IDH mutations and other genetic changes involved in classification, but its lack of availability and high cost leads to limited results (28). Furthermore, pathological diagnosis is largely dependent on the subjectivity and visual judgment of the pathologist and is influenced by experience. Therefore, non-invasive early identification of specific molecular markers is crucial for early individualized targeted therapy. Our study provides a new insight into the non-invasive diagnosis of multiple glioma-specific molecular markers, particularly for gliomas with atypical imaging features, allowing for preliminary assessment of tumor malignancy and prognosis, which could aid in early clinical diagnosis and targeted therapy.
Previous studies have used radiomics to predict gene expression and stratified diagnosis of glioma, but radiomics studies based on conventional MRI have not yielded satisfactory results and only a few have tried to predict multiple genes simultaneously (29). In particular, the prediction of molecular markers such as MGMT, EGFR, and CDKN 2A/B is more challenging. Zhu et al. combined structural MRI, apparent diffusion coefficient (ADC), and susceptibility-weighted imaging (SWI), and the results showed no effect in predicting MGMT methylation status. Adding SWI and ADC features did not provide additional information for structural MRI in predicting WHO grade and IDH mutation (30). Based on the pharmacokinetic characteristics of DCE-MRI, our study dynamically extracted multi-phase and multi-temporal omics features, and used cluster analysis to build a data framework to further explore the potential information of images and classify gliomas more comprehensively.
Our study selected DCE imaging features based on previously established diagnostic significance and their correlation with molecular and clinical outcomes. The clustering approach was designed to identify distinct patient subgroups based on these features, which could potentially guide personalized treatment strategies. For example, our findings suggested that cluster 3, with its high frequency of grade 4 and IDH wild-type gliomas, may represent a more aggressive subgroup that could benefit from more aggressive treatment approaches. Conversely, cluster 1, with its high frequency of EGFR non-mutant, may represent a more favorable prognosis group that could benefit from targeted therapies. The biological significance of these clusters is further supported by their association with distinct molecular and clinical features. However, further studies with larger and more diverse patient populations would be needed to confirm these findings and assess their clinical plausibility.
The limitations of our study lie in that, first of all, this was a single-center study with uneven data distribution and a large number of missing values of molecular detection indicators, which also limited our further analysis of the correlation between the clustering of molecular markers. Additionally, the detection of molecular markers was via obtainment of tumor tissue through surgical biopsy, and the tumors were highly heterogeneous. The results obtained largely depend on the subjective initiative of pathologists, which limits the diagnostic accuracy. Last but not least, the extraction of dynamic image features is affected by image quality and contrast agent dynamic/vascular characteristics changes. Both head movements and individual factors during the examination may lead to differences in results. Future research will be necessary to correlate dynamic radiomics features to baseline clinical characteristics, molecular markers, and overall survival in a multicenter database.
Conclusions
In summary, we have identified significant associations between DCE-MRI dynamic radiomics features and age, grading, and immunohistochemical and molecular markers in patients with glioma. These associations provided a stratified classification of key preoperative predictors of glioma. The extraction of these DCE-MRI-based dynamic radiomics features offers potential approaches for glioma diagnosis and prognosis, and may ultimately influence individualized treatment strategies in the future.
Acknowledgments
None.
Footnote
Reporting Checklist: The authors have completed the STROBE reporting checklist. Available at https://qims.amegroups.com/article/view/10.21037/qims-24-1459/rc
Funding: This work was supported by
Conflicts of Interest: All authors have completed the ICMJE uniform disclosure form (available at https://qims.amegroups.com/article/view/10.21037/qims-24-1459/coif). The authors have no conflicts of interest to declare.
Ethical Statement: The authors are accountable for all aspects of the work in ensuring that questions related to the accuracy or integrity of any part of the work are appropriately investigated and resolved. This retrospective study was conducted in accordance with the Declaration of Helsinki and its subsequent amendments, and was approved by the Ethical Committee of Nanjing Drum Tower Hospital (approval No. NCT05624736). The requirement for individual consent for this retrospective analysis was waived.
Open Access Statement: This is an Open Access article distributed in accordance with the Creative Commons Attribution-NonCommercial-NoDerivs 4.0 International License (CC BY-NC-ND 4.0), which permits the non-commercial replication and distribution of the article with the strict proviso that no changes or edits are made and the original work is properly cited (including links to both the formal publication through the relevant DOI and the license). See: https://creativecommons.org/licenses/by-nc-nd/4.0/.
References
- Global, regional, and national burden of brain and other CNS cancer, 1990-2016: a systematic analysis for the Global Burden of Disease Study 2016. Lancet Neurol 2019;18:376-93. [Crossref] [PubMed]
- Kang W, Mo Z, Li W, Ma H, Zhang Q. Heterogeneity and individualized treatment of microenvironment in glioblastoma (Review). Oncol Rep 2023;50:217. [Crossref] [PubMed]
- Weller M, van den Bent M, Preusser M, Le Rhun E, Tonn JC, Minniti G, et al. EANO guidelines on the diagnosis and treatment of diffuse gliomas of adulthood. Nat Rev Clin Oncol 2021;18:170-86. [Crossref] [PubMed]
- Louis DN, Perry A, Wesseling P, Brat DJ, Cree IA, Figarella-Branger D, Hawkins C, Ng HK, Pfister SM, Reifenberger G, Soffietti R, von Deimling A, Ellison DW. The 2021 WHO Classification of Tumors of the Central Nervous System: a summary. Neuro Oncol 2021;23:1231-51. [Crossref] [PubMed]
- Pons-Escoda A, Majos C, Smits M, Oleaga L. Presurgical diagnosis of diffuse gliomas in adults: Post-WHO 2021 practical perspectives from radiologists in neuro-oncology units. Radiologia (Engl Ed) 2024;66:260-77. [Crossref] [PubMed]
- Gore S, Chougule T, Jagtap J, Saini J, Ingalhalikar M. A Review of Radiomics and Deep Predictive Modeling in Glioma Characterization. Acad Radiol 2021;28:1599-621. [Crossref] [PubMed]
- Pons-Escoda A, Naval-Baudin P, Viveros M, Flores-Casaperalta S, Martinez-Zalacaín I, Plans G, Vidal N, Cos M, Majos C. DSC-PWI presurgical differentiation of grade 4 astrocytoma and glioblastoma in young adults: rCBV percentile analysis across enhancing and non-enhancing regions. Neuroradiology 2024;66:1267-77. [Crossref] [PubMed]
- Pons-Escoda A, Garcia-Ruiz A, Naval-Baudin P, Martinez-Zalacain I, Castell J, Camins A, Vidal N, Bruna J, Cos M, Perez-Lopez R, Oleaga L, Warnert E, Smits M, Majos C. Differentiating IDH-mutant astrocytomas and 1p19q-codeleted oligodendrogliomas using DSC-PWI: high performance through cerebral blood volume and percentage of signal recovery percentiles. Eur Radiol 2024;34:5320-30. [Crossref] [PubMed]
- Okuchi S, Rojas-Garcia A, Ulyte A, Lopez I, Ušinskienė J, Lewis M, Hassanein SM, Sanverdi E, Golay X, Thust S, Panovska-Griffiths J, Bisdas S. Diagnostic accuracy of dynamic contrast-enhanced perfusion MRI in stratifying gliomas: A systematic review and meta-analysis. Cancer Med 2019;8:5564-73. [Crossref] [PubMed]
- Chen S, Xu Y, Ye M, Li Y, Sun Y, Liang J, Lu J, Wang Z, Zhu Z, Zhang X, Zhang B. Predicting MGMT Promoter Methylation in Diffuse Gliomas Using Deep Learning with Radiomics. J Clin Med 2022;11:3445. [Crossref] [PubMed]
- Di Salle G, Tumminello L, Laino ME, Shalaby S, Aghakhanyan G, Fanni SC, Febi M, Shortrede JE, Miccoli M, Faggioni L, Cosottini M, Neri E. Accuracy of Radiomics in Predicting IDH Mutation Status in Diffuse Gliomas: A Bivariate Meta-Analysis. Radiol Artif Intell 2024;6:e220257. [Crossref] [PubMed]
- Xiong Z, Qiu J, Liang Q, Jiang J, Zhao K, Chang H, Lv C, Zhang W, Li B, Ye J, Li S, Peng S, Sun C, Chen S, Long D, Shu X. Deep learning models for rapid discrimination of high-grade gliomas from solitary brain metastases using multi-plane T1-weighted contrast-enhanced (T1CE) images. Quant Imaging Med Surg 2024;14:5762-73. [Crossref] [PubMed]
- Chen Q, Wang L, Deng Z, Wang R, Wang L, Jian C, Zhu YM. Cooperative multi-task learning and interpretable image biomarkers for glioma grading and molecular subtyping. Med Image Anal 2025;101:103435. [Crossref] [PubMed]
- Dercle L, Fronheiser M, Lu L, Du S, Hayes W, Leung DK, Roy A, Wilkerson J, Guo P, Fojo AT, Schwartz LH, Zhao B. Identification of Non-Small Cell Lung Cancer Sensitive to Systemic Cancer Therapies Using Radiomics. Clin Cancer Res 2020;26:2151-62. [Crossref] [PubMed]
- Li D, Hu W, Ma L, Yang W, Liu Y, Zou J, Ge X, Han Y, Gan T, Cheng D, Ai K, Liu G, Zhang J. Deep learning radiomics nomograms predict Isocitrate dehydrogenase (IDH) genotypes in brain glioma: A multicenter study. Magn Reson Imaging 2025;117:110314. [Crossref] [PubMed]
- Rameh V, Vajapeyam S, Ziaei A, Kao P, London WB, Baker SJ, Chiang J, Lucas J, Tinkle CL, Wright KD, Poussaint TY. Correlation between Multiparametric MR Imaging and Molecular Genetics in Pontine Pediatric High-Grade Glioma. AJNR Am J Neuroradiol 2023;44:833-40. [Crossref] [PubMed]
- Wang H, Zhang S, Xing X, Yue Q, Feng W, Chen S, Zhang J, Xie D, Chen N, Liu Y. Radiomic study on preoperative multi-modal magnetic resonance images identifies IDH-mutant TERT promoter-mutant gliomas. Cancer Med 2023;12:2524-37. [Crossref] [PubMed]
- Jian A, Jang K, Manuguerra M, Liu S, Magnussen J, Di Ieva A. Machine Learning for the Prediction of Molecular Markers in Glioma on Magnetic Resonance Imaging: A Systematic Review and Meta-Analysis. Neurosurgery 2021;89:31-44. [Crossref] [PubMed]
- Henker C, Kriesen T, Schneider B, Glass Ä, Scherer M, Langner S, Erbersdobler A, Piek J. Correlation of Ki-67 Index with Volumetric Segmentation and its Value as a Prognostic Marker in Glioblastoma. World Neurosurg 2019;125:e1093-103. [Crossref] [PubMed]
- Capper D, Reifenberger G, French PJ, Schweizer L, Weller M, Touat M, Niclou SP, Euskirchen P, Haberler C, Hegi ME, Brandner S, Le Rhun E, Rudà R, Sanson M, Tabatabai G, Sahm F, Wen PY, Wesseling P, Preusser M, van den Bent MJ. EANO guideline on rational molecular testing of gliomas, glioneuronal, and neuronal tumors in adults for targeted therapy selection. Neuro Oncol 2023;25:813-26. [Crossref] [PubMed]
- Lin WW, Juang C, Yueh MH, Huang TM, Li T, Wang S, Yau ST. 3D brain tumor segmentation using a two-stage optimal mass transport algorithm. Sci Rep 2021;11:14686. [Crossref] [PubMed]
- Lu X, Meng J, Zhou Y, Jiang L, Yan F. MOVICS: an R package for multi-omics integration and visualization in cancer subtyping. Bioinformatics 2021;36:5539-41. [Crossref] [PubMed]
- Fudaba H, Momii Y, Matsuta H, Onishi K, Kawasaki Y, Sugita K, Shimomura T, Fujiki M. Perfusion Parameter Obtained on 3-Tesla Magnetic Resonance Imaging and the Ki-67 Labeling Index Predict the Overall Survival of Glioblastoma. World Neurosurg 2021;149:e469-80. [Crossref] [PubMed]
- Hölzl D, Hutarew G, Zellinger B, Alinger-Scharinger B, Schlicker HU, Schwartz C, Sotlar K, Kraus TFJ. EGFR Amplification Is a Phenomenon of IDH Wildtype and TERT Mutated High-Grade Glioma: An Integrated Analysis Using Fluorescence In Situ Hybridization and DNA Methylome Profiling. Biomedicines 2022;10:794. [Crossref] [PubMed]
- van den Bent MJ, Smits M, Kros JM, Chang SM. Diffuse Infiltrating Oligodendroglioma and Astrocytoma. J Clin Oncol 2017;35:2394-401. [Crossref] [PubMed]
- Yang H, Zhu Z, Long C, Niu F, Zhou J, Chen S, Ye M, Peng S, Zhang X, Chen Y, Wei L, Wang H, Liu D, Yao M, Zhang X, Zhang B. Quantitative and Qualitative Parameters of DCE-MRI Predict CDKN2A/B Homozygous Deletion in Gliomas. Acad Radiol 2024;31:3355-65. [Crossref] [PubMed]
- Park YW, Park KS, Park JE, Ahn SS, Park I, Kim HS, Chang JH, Lee SK, Kim SH. Qualitative and Quantitative Magnetic Resonance Imaging Phenotypes May Predict CDKN2A/B Homozygous Deletion Status in Isocitrate Dehydrogenase-Mutant Astrocytomas: A Multicenter Study. Korean J Radiol 2023;24:133-44. [Crossref] [PubMed]
- Barresi V, Eccher A, Simbolo M, Cappellini R, Ricciardi GK, Calabria F, Cancedda M, Mazzarotto R, Bonetti B, Pinna G, Sala F, Ghimenton C, Scarpa A. Diffuse gliomas in patients aged 55 years or over: A suggestion for IDH mutation testing. Neuropathology 2020;40:68-74. [Crossref] [PubMed]
- Sohn B, An C, Kim D, Ahn SS, Han K, Kim SH, Kang SG, Chang JH, Lee SK. Radiomics-based prediction of multiple gene alteration incorporating mutual genetic information in glioblastoma and grade 4 astrocytoma, IDH-mutant. J Neurooncol 2021;155:267-76. [Crossref] [PubMed]
- Zhu Z, Shen J, Liang X, Zhou J, Liang J, Ni L, Wang H, Ye M, Chen S, Yang H, Chen Q, Li X, Zhang W, Lu J, Ge D, Fu L, Zhu Y, Zhang X, Sun Y, Zhang B. Radiomics for predicting grades, isocitrate dehydrogenase mutation, and oxygen 6-methylguanine-DNA methyltransferase promoter methylation of adult diffuse gliomas: combination of structural MRI, apparent diffusion coefficient, and susceptibility-weighted imaging. Quant Imaging Med Surg 2024;14:9276-89. [Crossref] [PubMed]