Time-variant and tissue-level collaterals predict postoperative neurological recovery and clinical outcomes of patients with endovascular thrombectomy
Introduction
Endovascular thrombectomy (EVT) has been demonstrated to be highly effective for patients suffering from acute ischemic stroke caused by anterior large vessel occlusion, and it enables numerous patients to achieve clinical neurological recovery and improved functional outcome (1-5). Patients with well-developed collaterals have been shown to have smaller infarct core and exhibit a more favorable prognosis after treatment (6). However, the compensatory effect of collaterals on ischemia is a dynamic process with complex mechanisms. Therefore, more accurate and comprehensive assessments of collateral status are critical for the postoperative management and improvement of prognosis in patients undergoing EVT.
Arterial collaterals on computed tomography (CT) angiography have been recognized as a criterion for inclusion in the EVT treatment in previous research (1,7). Traditionally, single-phase CT angiography (CTA) was conventionally recruited for assessing the arterial collateral status with single scanning at the peak of contrast agent in clinical routine. However, this approach is likely to underestimate the intracranial arterial compensation (8). In contrast, multiphase CTA (mCTA), which typically encompasses three phases, facilitates superior localization of arterial occlusion and provides both spatial and temporal assessments of collateral status. The representation of arteries through delayed filling enables a more precise evaluation of collaterals via mCTA than that is achievable with single-phase CTA (9). Furthermore, an innovative post-processing tool of mCTA called color-coded map, serves to condense all phases of mCTA into a singular series (10), and the additional time-variant color-coded map may assist in enhancing the assessment of collateral status (11). Nevertheless, the potential association of collateral status with time-variant and clinical outcome of EVT patients remains to be elucidated.
In addition, tissue-level collateral assessed by CT or magnetic resonance imaging (MRI) perfusion imaging can be evaluated and standardized by hypoperfusion intensity ratio (HIR) (12,13). Previous studies showed that HIR was correlated with hypoperfusion volumes, status of collaterals, and infarct progression during patients’ transfer to comprehensive stroke centers (14-16). Conversely, early neurological improvement after reperfusion treatment is a marker of favorable clinical outcome, but it is limited in reflecting the trend in neurological function changes after EVT. Continuous tracking of the National Institutes of Health Stroke Scale (NIHSS) at designated time points (TPs) is relevant for assessing of treatment results and postoperative management in thrombolysis treatment (17), but it has not been applied in EVT treatment before. We believe that it is also critical to explore the impact of collateral status on continuous postoperative neurological function trends in patients with EVT.
Machine learning (ML) is effecting a transformation in clinical interventions. ML algorithms demonstrate a significantly enhanced capability to utilize high-dimensional data to develop precise patient risk assessment models. These models surpass conventional logistic regression (LR)-based tools by incorporating a greater number of predictors and identifying intricate, nonlinear relationships (18). Such capabilities augment the precision and reliability of prognoses, which are essential for clinical decision-making (19).
Consequently, this study employs ML methodologies to forecast clinical outcomes for patients undergoing endovascular therapy, with particular emphasis on collateral status. Furthermore, we aspire to investigate the disparities in neurological recovery based on varying collateral-driven patient stratification. We present this article in accordance with the STROBE reporting checklist (available at https://qims.amegroups.com/article/view/10.21037/qims-24-1073/rc).
Methods
Patients
The study was conducted in accordance with the Declaration of Helsinki and its subsequent amendments. The study was approved by the institutional ethics board of Tianjin Huanhu Hospital (No. 2024-038) and informed consent was taken from all the patients. Patients with acute ischemic stroke due to anterior large vessel occlusion who underwent EVT were included between May 2020 and December 2022. The inclusion criteria were as follows: (I) patients underwent baseline mCTA and CT perfusion (CTP) examinations; (II) patients had large vessel occlusion of anterior circulation [occlusion of the intracranial segment of the internal carotid artery, or middle cerebral artery (MCA) M1 or M2 segment]; and (III) they underwent EVT treatment within 24 hours after stroke onset. Exclusion criteria were as follows: (I) age <18 years old; (II) occlusion of the extracranial segment of the internal carotid artery or posterior cerebral arteries; (III) stroke was caused by other determined factors, such as moyamoya disease, arteritis, and vascular malformation; (IV) pre-stroke modified Rankin Scale (mRS) ≥3; (V) lack of complete clinical information; (VI) absence of 90-day mRS; and (VII) poor mCTA or CTP image quality to patient motion or failed contrast material bolus. Clinical information, including age, sex, onset time, and cerebrovascular risk factors, were collected. For patients whose stroke onset was not observed, onset time was assessed as midway between the time of last known well and the first showing of stroke symptoms. Treatment information, including thrombolysis or not before EVT, modified thrombolysis in cerebral infarction score, and symptomatic intracranial hemorrhage, were collected.
Imaging acquisition and postprocessing
All CT examinations were obtained with the multi-detector CT scanner (Revolution CT, GE HealthCare, Milwaukee, WI, USA). All patients underwent multimodal CT imaging, including non-contrast CT, mCTA, and CTP before EVT. Detailed imaging parameters are provided in Appendix 1. Iopromide (GE HealthCare, 370 mgI/mL) was used for mCTA and CTP examination.
Time-variant collateral score was evaluated on the color-coded axial mCTA summation maps on a workstation using the ColorViz module of FastStroke research prototype (AW 4.7; GE HealthCare). Based on the adaptive threshold technique, color-coding of the collaterals was accomplished. Red indicated vessels with maximum enhancement in the arterial phase; green and blue signified those with maximum enhancement in the peak venous phase and late venous phase. The post-processing was fully automated within 10–15 s. Based on the collateral score of mCTA described by the previous study (20), we designed a 6-point scale system to evaluate the extent and delay of arterial filling on color-coded maps, which defined Collateral Score on Color-Coded summation maps (CSCC): Score 5, all arterial fillings were red color-coded and normal extent; Score 4, red and green color-coding in the filling of peripheral vessels, but prominence and extent is the same; Score 3, existing of blue color-coded vessels with normal extent or appearing of only red color-coded vessels and significantly reduced number or vessels; Score 2, existing of blue color-coded vessels with decreased prominence and extent, or only green vessels exist and appearance of some no vessels regions; Score 1, just a few vessels visible in the maps; Score 0, no vessels visible in the maps (Figure S1). The measurement of CSCC was independently performed and in randomized order by S.L. and T.Y. (with 6- and 15-year experience interpreting acute stroke studies, respectively). Blinding of treatment assignment, temporal factors, and clinical information was implemented for all imaging analyses. In instances of disagreement among readers, consensus was achieved regarding all image parameters.
RAPID (RApid Processing of perfusIon and Diffusion; version 4.5.0; iSchemaView, Menlo Park, CA, USA) was utilized to generate maps of ischemic cores and hypoperfusion from CTP automatically. HIR, which defined as tissue-level collateral characteristic, was calculated as the volume of the time to maximum (Tmax) more than 10 s (Tmax >10 s) lesion divided by the volume of the Tmax >6 s lesion (Figure S1): HIR = Tmax >10 s volume/Tmax >6 s volume. Alberta Stroke Program Early CT Score (ASPECTS) was automatically obtained from preoperative non-contrast CT images using the RAPID software.
Outcomes measures
The clinical outcome was defined as the mRS score at 90 days after EVT, with good clinical outcomes defined as a score of 0 to 2 and poor outcomes defined as a score of 3 to 6. NIHSS scores were measured at multiple TPs, including baseline (TP1), 24 hours (TP2), 48 hours (TP3), 72 hours (TP4), 7 days (TP5) after EVT, and discharge (TP6). Blinding to all imaging information, mRS, and NIHSS scores were evaluated by two neurologists with 8- and 15-year experience (L.L. and M.W.) through face-to-face communications or telephone interviews.
Feature selection and ML
All patients were randomly divided into a 70% (n=89) training set and a 30% (n=39) testing set. A five-fold cross-validation approach was used to ensure that both the training and testing sets were representative of the overall dataset and maintained the same proportion of clinical outcome classes. The training set was employed for model development and optimization, and the test set was utilized to evaluate and compare model performance. Among all the variables, the overall rate of missing data was 0.098%, a k-nearest-neighbor algorithm was performed to impute the missing data for continuous variables. To select potential features, the univariate regression analysis was performed. Subsequently, the retained significant variables (P<0.1) were incorporated into a multivariable LR analysis, and the final significant variables were identified with a significance level of P<0.05.
We used five ML models, including LR, support vector machine (SVM), random forest (RF), decision tree (DT), and eXtreme gradient boosting (XGBoost) to predict functional outcomes for patients with EVT, and selected the optimal model. The LR predicts the probability of the binary dependent variable using maximum likelihood estimation to determine the regression coefficient. SVM identifies a hyperplane for binary classification, which is represented in a higher-dimensional space according to the input data. RF is an ensemble learning method that constructs multiple DTs to improve prediction accuracy and prevent over-fitting by averaging their outputs. A DT method is a tree-like model of decisions that can predict the best choice mathematically. And XGBoost builds DTs sequentially, correcting errors of prior trees through gradient descent to optimize performance on complex datasets. The class weight technique was employed to solve the issue of data imbalance. Optuna, a hyperparameter optimization framework based on random search, was used to optimize the ML models (21).
ML models performance
The area under the receiver operating characteristic (ROC) curve (AUC) and precision-recall (PR) curves was used to describe tools for diagnostic testing or the identification accuracy of predictive models. Additionally, various performance metrics including sensitivity, specificity, positive predictive value (PPV), negative predictive value (NPV), accuracy, precision, Recall, and F1-score were scrutinized. The evaluation of clinical utility was performed by decision curve analysis. For model explanations, we used Shapley Additive exPlanations (SHAP) values to explain how different ML models had worked.
Statistical analysis
The statistical analysis was performed by using R language (version 4.2.1; R Foundation for Statistical Computing, Vienna, Austria). The distribution was assumed non-parametric on the basis of the Shapiro-Wilk tests. The χ2 test and Fisher exact test were used for categorical variables, and Mann-Whitney test and independent samples t-test were applied to continuous variables. Mixed effect models were established to estimate the fixed effects and group × time interaction effects of CSCC and HIR stratification on the NIHSS change. All statistical tests were two sided and were performed at a P value less than 0.05.
Results
Patients characteristics
Overall, among 825 patients who underwent EVT, 128 patients were eligible [97 men (75.8%)] (Figure 1). The median age was 64.0 years [range, 27–84 years; interquartile range (IQR), 57.3–69.0 years]. The median interval between the symptom onset and admission CT examination was 11 hours (range, 4–24 hours; IQR, 8–17 hours). Eleven patients (8.6%) underwent intravenous thrombolysis prior to receiving EVT. One hundred and 6 (82.8%) patients achieved successful recanalization (modified thrombolysis in cerebral infarction score 2b or 3). Good functional outcome was achieved in 50.0% (n=64) of patients. Patients with good outcomes were younger, more likely to achieve successful recanalization, more frequently symptomatic intracranial hemorrhage, and had lower baseline NIHSS. We found no significant differences between the good outcome group and poor outcome group in clinical risk factors. Table 1 summarizes the detailed baseline characteristics of all patients and provides a comparison based on outcomes. Case examples in the good outcome group and poor outcome group are shown in Figure 2.
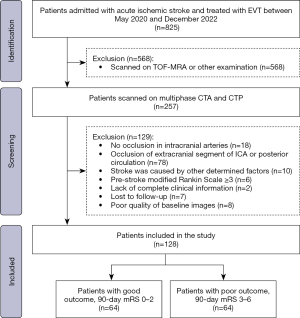
Table 1
Characteristics | All patients (n=128) | Good outcome group (n=64) | Poor outcome group (n=64) | P |
---|---|---|---|---|
Demographic characteristics | ||||
Age (years) | 64.0 [57.3, 69.0] | 63.0 [55.3, 67.0] | 66.0 [60.0, 71.0] | 0.002** |
Men | 97 (75.8) | 48 (75.0) | 49 (76.6) | >0.99 |
Hypertension | 86 (67.2) | 43 (67.2) | 43 (67.2) | >0.99 |
Diabetes mellitus | 25 (19.5) | 12 (18.8) | 13 (20.3) | >0.99 |
Dyslipidemia | 42 (32.8) | 25 (39.1) | 17 (26.6) | 0.186 |
Atrial fibrillation | 21 (16.4) | 8 (12.5) | 13 (20.3) | 0.340 |
Smoking history | 62 (48.4) | 32 (50.0) | 30 (46.9) | 0.860 |
Alcohol intake | 49 (38.3) | 26 (40.6) | 23 (35.9) | 0.716 |
Ischemic heart disease | 26 (20.3) | 10 (15.6) | 16 (25.0) | 0.272 |
Previous stroke | 20 (15.6) | 9 (14.1) | 11 (17.2) | 0.808 |
Clinical information | ||||
Onset time (hours) | 11 [8, 17] | 12 [8, 18] | 11 [7, 16] | 0.626 |
Thrombolysis before EVT | 11 (8.6) | 7 (10.9) | 4 (6.3) | 0.530 |
Modified thrombolysis in cerebral infarction score ≥ 2b | 106 (82.8) | 57 (89.1) | 32 (50.0) | <0.001** |
NIHSS score on admission | 13.6±5.4 | 11.9±5.2 | 15.4±5.0 | <0.001** |
Symptomatic intracranial hemorrhage | 40 (31.3) | 18 (28.1) | 32 (50.0) | 0.018* |
Imaging features | ||||
Tandem occlusion | 27 (21.1) | 14 (21.9) | 13 (20.3) | >0.99 |
Clot burden | 6 [6, 8] | 7 [6, 8] | 6 [6, 8] | 0.413 |
CSCC | 0.001** | |||
5 | 0 (0.0) | 0 (0.0) | 0 (0.0) | |
4 | 23 (18.0) | 17 (26.6) | 6 (9.4) | |
3 | 75 (58.6) | 37 (57.8) | 28 (43.8) | |
2 | 30 (23.4) | 8 (12.5) | 21 (32.8) | |
1 | 11 (8.6) | 2 (3.1) | 9 (14.1) | |
0 | 0 (0.0) | 0 (0.0) | 0 (0.0) | |
ASPECTS | 7 [6, 8] | 7 [6, 9] | 7 [4, 8] | 0.003** |
Tmax (mL) | ||||
>4 s volume | 320 [227, 456] | 299 [199, 403] | 370 [228, 484] | 0.055 |
>6 s volume | 157 [104, 222] | 134 [69, 194] | 169 [128, 255] | <0.001** |
>8 s volume | 92 [38, 142] | 69 [18, 117] | 109 [65, 182] | <0.001** |
>10 s volume | 50 [13, 89] | 35 [4, 63] | 70 [36, 132] | <0.001** |
HIR | 0.310 [0.166, 0.487] | 0.236 [0.078, 0.375] | 0.434 [0.220, 0.556] | <0.001** |
Data are presented a n (%) for categorical variables and median [IQR] for continuous variables, except for NIHSS score on admission, which is presented as mean ± SD. *, P<0.05; **, P<0.01. ASPECTS, Alberta Stroke Program Early CT Score; CSCC, Collateral Score on Color-Coded summation maps; EVT, endovascular thrombectomy; HIR, hypoperfusion intensity ratio; IQR, interquartile range; mRS, modified Rankin Scale; NIHSS, National Institutes of Health Stroke Scale; SD, standard deviation; Tmax, time to maximum.
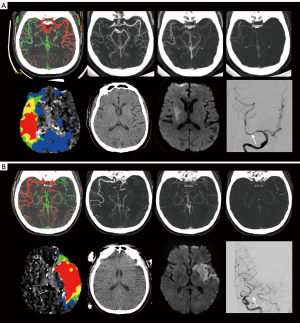
The baseline characteristics of the training set [70% (n=89) and test set [30% (n=39)] are detailed in Table S1. There is no significant difference between the two sets in the baseline characteristics. The results of post-hoc sample size calculation, intra-observer and inter-observer reproducibility for CSCC assessment were shown in Appendix 2.
Feature selection and ML model performance
In the training set, univariable regression was used to identify 10 significant risk factors, including age, symptomatic intracranial hemorrhage, ASPECTS, modified Thrombolysis in cerebral infarction score after EVT, CSCC, Tmax >4 s volume, Tmax >6 s volume, Tmax >8 s volume, Tmax >10 s volume, and HIR. Subsequently, these were used to determine independent risk factors using multivariate regression, and results showed that age [odds ratio (OR) =1.073; 95% confidence interval (CI): 1.008, 1.154; P=0.040], ASPECTS (OR =0.742; 95% CI: 0.546, 0.975; P=0.040), CSCC (OR =0.468; 95% CI: 0.213, 0.953; P=0.044), and HIR (OR =56.666; 95% CI: 3.843, 1,156.959; P=0.005) were associated with functional outcome. The results of the univariable and multivariable analyses in the training set are presented in Table S2.
Based on the above four significant variables identified through multivariable regression analysis in the training set, LR, SVM, RF, and XGBoost models were developed for the prediction of good functional outcomes, respectively. Among them, the RF model was identified as the most effective predictive model for the clinical outcome in the training set (AUC =0.964; 95% CI: 0.902, 0.992), displaying sensitivity of 0.800, specificity of 0.977, accuracy of 0.888, and F1-score of 0.878. The comparisons of AUC, sensitivity, specificity, accuracy, F1-score, PPV and NPV between various ML models were depicted in Table 2 and Figures 3,4. In the testing set, the RF model also achieved the highest AUC (AUC =0.837; 95% CI: 0.684, 0.935), with a sensitivity of 0.421, specificity of 0.950, accuracy of 0.692, and F1-score of 0.571. In PR curves, RF also achieved the highest AUCs in both training set (AUC of PR =0.968; 95% CI: 0.852, 0.994) and testing set (AUC of PR =0.858; 95% CI: 0.624, 0.956) (Figure S2). Based on the decision curve analysis, the RF model persistently provided the highest net benefit compared with other models in both the training and testing sets (Figure 3). Figure 5 shows the confusion matrix and SHAP plot to illustrate the contribution of each feature to the clinical outcome in the RF model. Among the selected features, HIR had the highest SHAP values, showing the importance in the clinical outcome of patients with EVT. The results of predictive performance of variables in the total cohort, comparison of predictive performance for clinical outcome between HIRs with different thresholds, and five-fold validation of the RF model were shown in Appendix 2 and Figures S3,S4.
Table 2
Algorithms | AUC (95% CI) | Sensitivity | Specificity | PPV | NPV | Accuracy | Precision | Recall | F1-score |
---|---|---|---|---|---|---|---|---|---|
Training set | |||||||||
LR | 0.833 (0.739, 0.904) | 0.778 | 0.796 | 0.796 | 0.778 | 0.787 | 0.778 | 0.795 | 0.787 |
RF | 0.964 (0.902, 0.992) | 0.800 | 0.977 | 0.973 | 0.827 | 0.888 | 0.800 | 0.973 | 0.878 |
SVM | 0.825 (0.730, 0.897) | 0.689 | 0.909 | 0.886 | 0.741 | 0.798 | 0.689 | 0.886 | 0.775 |
DT | 0.853 (0.762, 0.919) | 0.711 | 0.864 | 0.842 | 0.745 | 0.787 | 0.711 | 0.842 | 0.771 |
XGBoost | 0.767 (0.666, 0.850) | 0.689 | 0.727 | 0.721 | 0.696 | 0.708 | 0.689 | 0.721 | 0.705 |
Testing set | |||||||||
LR | 0.789 (0.629, 0.903) | 0.684 | 0.650 | 0.650 | 0.684 | 0.667 | 0.684 | 0.650 | 0.667 |
RF | 0.837 (0.684, 0.935) | 0.421 | 0.950 | 0.889 | 0.633 | 0.692 | 0.421 | 0.888 | 0.571 |
SVM | 0.726 (0.560, 0.856) | 0.526 | 0.800 | 0.714 | 0.640 | 0.667 | 0.526 | 0.714 | 0.606 |
DT | 0.726 (0.560, 0.856) | 0.526 | 0.850 | 0.769 | 0.654 | 0.692 | 0.526 | 0.769 | 0.625 |
XGBoost | 0.718 (0.552, 0.850) | 0.632 | 0.700 | 0.667 | 0.667 | 0.667 | 0.632 | 0.667 | 0.649 |
AUC, area under the ROC curve; CI, confidence interval; DT, decision tree; LR, logistic regression; ML, machine learning; NPV, negative predictive value; PPV, positive predictive value; RF, random forest; ROC, receiver operator characteristic; SVM, support vector machine; XGBoost, eXtreme gradient boosting.
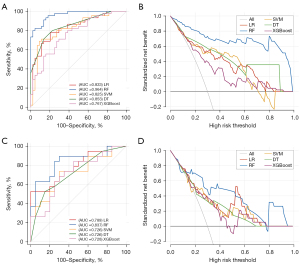
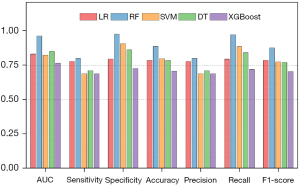
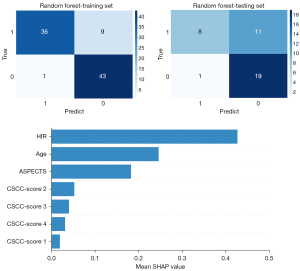
Effect of CSCC and HIR stratifications on the postoperative neurological recovery
According to the cut-off value of ROC analysis (Figure 3 and Table S3), patients were divided into two stratifications based on CSCC and HIR, respectively. The significant fixed effects were found in both CSCC stratification (TP4 to TP5: β=−1.330, P=0.023; TP5 to TP6: β=−1.875, P=0.001) and HIR stratification (TP4 to TP5: β=−2.143, P<0.001; TP5 to TP6: β=−2.868, P<0.001). Furthermore, significant group × time interaction effects were observed from TP4 to TP5 (β=2.105, P=0.043) in CSCC stratification, and at all TPs in HIR stratification (detailed results are shown in Table 3). Akaike information criterion (AIC) suggested that HIR stratification is the better indicator in the determination of postoperative neurological recovery after EVT treatment (AICHIR =4,599.577 vs. AICCSCC =4,648.707).
Table 3
Predictors | CSCC stratification (CSCC ≥3 vs. <3) |
HIR stratification (HIR <0.459 vs. ≥0.459) |
|||
---|---|---|---|---|---|
β (SE) | P | β (SE) | P | ||
Fixed effects | |||||
Group | 1.580 (1.488) | 0.291 | 2.868 (1.425) | 0.046* | |
TP1 − TP2 | 0.330 (0.581) | 0.571 | −0.330 (0.558) | 0.555 | |
TP2 − TP3 | 0.057 (0.581) | 0.922 | −0.527 (0.558) | 0.345 | |
TP3 − TP4 | −0.295 (0.581) | 0.611 | −1.033 (0.558) | 0.065 | |
TP4 − TP5 | −1.330 (0.581) | 0.023* | −2.143 (0.558) | <0.001** | |
TP5 − TP6 | −1.875 (0.581) | 0.001** | −2.868 (0.558) | <0.001** | |
Group × TP1 − TP2 | 1.070 (1.039) | 0.304 | 3.438 (1.04) | 0.001** | |
Group × TP2 − TP3 | 1.067 (1.039) | 0.305 | 3.176 (1.04) | 0.002** | |
Group × TP3 − TP4 | 2.020 (1.039) | 0.524 | 4.736 (1.04) | <0.001** | |
Group × TP4 − TP5 | 2.105 (1.039) | 0.043 | 5.089 (1.04) | <0.001** | |
Group × TP5 − TP6 | 1.625 (1.039) | 0.119 | 5.192 (1.04) | <0.001** | |
Constant | 13.170 (0.832) | <0.001** | 12.835 (0.77) | <0.001** | |
Random effects | |||||
Residual | 3.824 | – | 3.737 | – | |
AIC | 4,648.707 | – | 4,599.577 | – | |
BIC | 4,713.720 | – | 4,664.590 | – |
Values signify the estimated effects (β) and corresponding SE. Mixed effect model was performed to analyzed the main effects and group × time interaction effects in NIHSS at multiple TPs, including baseline (TP1), 24 hours (TP2), 48 hours (TP3), 72 hours (TP4), 7 days after treatment (TP5), and discharge (TP6). *, P<0.05; **, P<0.01. AIC, Akaike information criterion; BIC, Bayesian information criterion; CSCC, Collateral Score on Color-Coded summation maps; HIR, hypoperfusion intensity ratio; NIHSS, National Institutes of Health Stroke Scale; SE, standard error; TP, time point.
Line charts and three-dimensional simulations vividly demonstrated that the variation of postoperative NIHSS was different in CSCC stratification and HIR stratification (Figure 6). NIHSS scores in patients with CSCC <3 trended to increase after EVT but returned to the baseline level, whereas decreased steadily over time in patients with CSCC ≥3. In HIR stratification, NIHSS scores decreased gradually after EVT in patients with HIR <0.459, but increased within 24 hours after EVT and then remained stable. The difference in NIHSS between patients with HIR <0.459 and those with HIR ≥0.459 became increasingly larger over time. The spaghetti plots with individual longitudinal trajectories in CSCC and HIR stratifications are shown in Figure S5. The results of association between collaterals and NIHSS at different TPs were shown in Appendix 2 and Figures S6,S7.
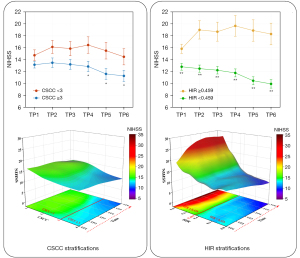
Discussion
Our research elucidated numerous collateral features, including time-resolved resolution, morphology, and tissue-level characteristics, through a comprehensive multimodal CT scan aimed at investigating their predictive value for postoperative neurological recovery and clinical outcomes in patients undergoing EVT. We employed various ML models to evaluate the predictive efficiency of clinical and multiple collateral features, and found that the RF model based on CSCC and HIR demonstrated superior performance in predicting functional outcomes compared to other models. Furthermore, it was revealed that HIR stratification was more effective in predicting the continuous variation trend of postoperative neurological recovery than CSCC, thus offering significant insights for clinical decision-making.
A comprehensive assessment of cerebral collaterals may yield an enhanced comprehension of the mechanism of collateral perfusion in patients with acute large vessel occlusion (22-24). Well-developed arterial collaterals are essential for maintaining compensatory blood supply to ischemic regions, thereby preventing the progression to an established infarct core (25,26). Moreover, collaterals based on single-phase CTA have been used as an inclusion criterion for EVT (1). The effect of collateral status on infarct growth and functional outcomes has been thoroughly reviewed (6). Nevertheless, mCTA has been shown to increase the detection of distal-vessel occlusion compared with single-phase CTA (27). Menon et al. described collateral evaluation by mCTA with a six-point scale, and the ability to predict clinical outcome was better than that of single-phase CTA and CTP (20). The additional color-indicator effect achieving from a color-coded display format could further facilitate detection and localization of distal criminal vessel on mCTA, thereby enhancing the precision of assessing the true extent of collateral status (11,28). Furthermore, it allows for the visualization of arteries exhibiting delayed filling through a tri-color display, illustrating the time-resolved characteristics of pial arteries. We devised a time- variant collateral score utilizing a six-point scale grounded in the color-coded maps of mCTA, delineating comprehensive information regarding the time variance in pial artery filling. The AUC of CSCC for 90-day mRS increased 19% (0.691 vs. 0.58) when compared to the results presented by Menon (20). Our findings suggest that the concept of time-variant collaterals may serve as a precise and valuable tool for accurately representing collateral status and predicting clinical outcomes in patients undergoing EVT treatment.
The diagnostic efficiency of the HIR concerning clinical outcomes was demonstrated, revealing superior discrimination for 90-day functional outcomes compared to the CSCC (AUC, 0.731 vs. 0.691), despite the absence of significant differences between the two AUCs. Our findings are consistent with recent studies that have highlighted the importance of mCTA and HIR in forecasting the clinical prognosis of patients undergoing EVT (29-31). The capacity of the HIR to differentiate between favorable and unfavorable outcomes could potentially improve patient selection and facilitate clinical decision-making in the context of EVT. The substantial importance of the ASPECTS and the CSCC, in conjunction with HIR, underscores the combined significance of both macroscopic and microscopic perfusion evaluations in assessing cerebral tissue damage and the prospects for neurological recovery. We propose that a system incorporating a thorough evaluation of collateral status, which includes both temporal, morphological, and tissue-level characteristics, may yield vital predictive value concerning the functional outcomes of patients undergoing EVT.
Among the various ML models, RF demonstrated the highest efficacy in predicting outcomes for patients undergoing EVT. A significant advantage of the RF algorithm is its ensemble learning methodology, which constructs numerous DTs and aggregates their predictions. This approach mitigates the risk of overfitting and enhances the precision of the predictions (32). This aspect is crucial in clinical practice, where datasets often involve complex, non-linear relationships among variables. Moreover, the capability of RF to manage high-dimensional data and rank feature importance, which was shown on SHAP plots, facilitates interpretability by identifying key contributors to clinical outcomes (33). This study identifies variables such as the HIR and CSCC as significant predictors of outcomes. The robustness and interpretability of RF render it an effective instrument for facilitating clinical decision-making, with the potential to enable the early identification of patients at a heightened risk of adverse outcomes, thereby informing personalized interventions and enhancing prognosis following EVT.
We also investigated the variation trends in the neurological function of patients following EVT across different types of collateral stratifications. Early neurological improvement, defined as NIHSS decrease ≥4 between admission and 24 hours or NIHSS 0–1 at 24 hours, was considered as the strong predictor of long-term clinical outcome in patients with acute ischemic stroke in previous studies (34-37). In this study, we assessed not only NIHSS on admission and 24 hours after EVT, but also NIHSS scores at 48 hours, 72 hours, 7 days after EVT, and at discharge. According to the results derived from mixed effect models in CSCC and HIR stratifications, the HIR stratification demonstrates superior predictive value for continuous variations in NIHSS scores post-EVT compared to CSCC. We propose that enhanced permeability signifies an increased uptake of oxygen-rich blood in the hypoperfused brain tissue when successful reperfusion is achieved, and that HIR possesses a superior capacity to illustrate whether blood successfully permeates into the cerebral tissue from the pial collateral network (16,38). Consequently, we determined that the ongoing alterations in the NIHSS following EVT hold significant value in guiding clinical decision-making for patients undergoing EVT. Furthermore, the preoperative HIR stratification may offer predictive insights regarding the trajectory of neurological recovery, thereby facilitating the development of individualized treatment plans for patients receiving EVT.
The clinical implications of our findings are of considerable significance. Firstly, the assessment of both CSCC and HIR provides a comprehensive evaluation of time-variant and tissue-level collateral circulation, which may enhance predictions regarding short-term neurological recovery and long-term functional outcomes. Secondly, the RF model, noted for its superior predictive capabilities, serves as a promising tool for clinicians to estimate functional outcomes with greater accuracy, ultimately contributing to the optimization of individualized treatment strategies for patients experiencing acute ischemic stroke patients.
Our research presents several limitations. Firstly, the small sample size and the retrospective design conducted at a single center may introduce potential selection bias. It is imperative to conduct additional validation and further apply our findings in larger, multi-center cohorts. Nevertheless, our analysis benefited from a dataset derived from patients who underwent continuous NIHSS evaluations at six distinct TPs throughout their entire hospitalization, a methodology that is relatively rare in previous literature. Secondly, to control for variables, all enrolled patients were scanned using a single CT scanner. Future studies should incorporate other scanners to enhance the generalizability of our results. Thirdly, the parameter employed to assess tissue-level collaterals is the HIR, as opposed to the Perfusion Computed Tomography Index (39), which is adjusted for relative cerebral blood volume and is more close to the collateral status as assessed by digital subtraction angiography (DSA). The subsequent step involves a more in-depth examination of both indicators to evaluate their distinct predictive value regarding short-term and long-term outcomes. Fourthly, the implementation of delay and dispersion correction during CTP processing may improve the accuracy of estimating hypoperfusion and collateral circulation. Lastly, the absence of follow-up treatment following EVT presents a potential confounding factor influencing the trend of neurological recovery.
Conclusions
Our study demonstrates that HIR and CSCC are significant predictors of functional outcomes in patients undergoing EVT. The RF model, with its robust performance, could be utilized as a decision-support tool to predict clinical outcomes, potentially improving treatment selection and optimizing stroke care. Furthermore, HIR stratification may serve as a pathway for the enhancement and personalization of care for patients undergoing EVT by determining whether a patient is at an increased risk for neurological deterioration or possesses the potential for neurological recovery.
Acknowledgments
We would like to acknowledge all technicians, Radiology Department of Tianjin Huanhu Hospital, for patient recruitment.
Footnote
Reporting Checklist: The authors have completed the STROBE reporting checklist. Available at https://qims.amegroups.com/article/view/10.21037/qims-24-1073/rc
Funding: This work was supported by
Conflicts of Interest: All authors have completed the ICMJE uniform disclosure form (available at https://qims.amegroups.com/article/view/10.21037/qims-24-1073/coif). L.W. is from CT Imaging Research Center, GE Healthcare China. The other authors have no conflicts of interest to declare.
Ethical Statement: The authors are accountable for all aspects of the work in ensuring that questions related to the accuracy or integrity of any part of the work are appropriately investigated and resolved. The study was conducted in accordance with the Declaration of Helsinki and its subsequent amendments. The study was approved by the institutional ethics board of Tianjin Huanhu Hospital (No. 2024-038) and informed consent was taken from all the patients.
Open Access Statement: This is an Open Access article distributed in accordance with the Creative Commons Attribution-NonCommercial-NoDerivs 4.0 International License (CC BY-NC-ND 4.0), which permits the non-commercial replication and distribution of the article with the strict proviso that no changes or edits are made and the original work is properly cited (including links to both the formal publication through the relevant DOI and the license). See: https://creativecommons.org/licenses/by-nc-nd/4.0/.
References
- Goyal M, Demchuk AM, Menon BK, Eesa M, Rempel JL, Thornton J, et al. Randomized assessment of rapid endovascular treatment of ischemic stroke. N Engl J Med 2015;372:1019-30. [Crossref] [PubMed]
- Nogueira RG, Jadhav AP, Haussen DC, Bonafe A, Budzik RF, Bhuva P, et al. Thrombectomy 6 to 24 Hours after Stroke with a Mismatch between Deficit and Infarct. N Engl J Med 2018;378:11-21. [Crossref] [PubMed]
- LeCouffe NE, Kappelhof M, Treurniet KM, Rinkel LA, Bruggeman AE, Berkhemer OA, et al. A Randomized Trial of Intravenous Alteplase before Endovascular Treatment for Stroke. N Engl J Med 2021;385:1833-44. [Crossref] [PubMed]
- Yang P, Zhang Y, Zhang L, Zhang Y, Treurniet KM, Chen W, et al. Endovascular Thrombectomy with or without Intravenous Alteplase in Acute Stroke. N Engl J Med 2020;382:1981-93. [Crossref] [PubMed]
- Renú A, Millán M, San Román L, Blasco J, Martí-Fàbregas J, Terceño M, et al. Effect of Intra-arterial Alteplase vs Placebo Following Successful Thrombectomy on Functional Outcomes in Patients With Large Vessel Occlusion Acute Ischemic Stroke: The CHOICE Randomized Clinical Trial. JAMA 2022;327:826-35. [Crossref] [PubMed]
- Bang OY, Saver JL, Kim SJ, Kim GM, Chung CS, Ovbiagele B, Lee KH, Liebeskind DS. Collateral flow predicts response to endovascular therapy for acute ischemic stroke. Stroke 2011;42:693-9. [Crossref] [PubMed]
- Chen Z, Ying Y, Lu X, Yu C, Wang J, Shao J, Jia Q, Li P, Chen L. Direct endovascular treatment may be more appropriate for patients with good collateral circulation: a retrospective case-control study. Quant Imaging Med Surg 2024;14:8915-26. [Crossref] [PubMed]
- Fasen BACM, Heijboer RJJ, Hulsmans FH, Kwee RM. Diagnostic performance of single-phase CT angiography in detecting large vessel occlusion in ischemic stroke: A systematic review. Eur J Radiol 2021;134:109458. [Crossref] [PubMed]
- Wang Z, Xie J, Tang TY, Zeng CH, Zhang Y, Zhao Z, Zhao DL, Geng LY, Deng G, Zhang ZJ, Ju SH, Teng GJ. Collateral Status at Single-Phase and Multiphase CT Angiography versus CT Perfusion for Outcome Prediction in Anterior Circulation Acute Ischemic Stroke. Radiology 2020;296:393-400. [Crossref] [PubMed]
- Ospel JM, Cimflova P, Volny O, Qiu W, Hafeez M, Mayank A, Najm M, Chung K, Kashani N, Almekhlafi MA, Menon BK, Goyal M. Utility of Time-Variant Multiphase CTA Color Maps in Outcome Prediction for Acute Ischemic Stroke Due to Anterior Circulation Large Vessel Occlusion. Clin Neuroradiol 2021;31:783-90. [Crossref] [PubMed]
- Ospel JM, Volny O, Qiu W, Najm M, Kashani N, Goyal M, Menon BK. Displaying Multiphase CT Angiography Using a Time-Variant Color Map: Practical Considerations and Potential Applications in Patients with Acute Stroke. AJNR Am J Neuroradiol 2020;41:200-5. [Crossref] [PubMed]
- Faizy TD, Kabiri R, Christensen S, Mlynash M, Kuraitis G, Broocks G, Hanning U, Nawabi J, Lansberg MG, Marks MP, Albers GW, Fiehler J, Wintermark M, Heit JJ. Perfusion imaging-based tissue-level collaterals predict ischemic lesion net water uptake in patients with acute ischemic stroke and large vessel occlusion. J Cereb Blood Flow Metab 2021;41:2067-75. [Crossref] [PubMed]
- de Havenon A, Mlynash M, Kim-Tenser MA, Lansberg MG, Leslie-Mazwi T, Christensen S, McTaggart RA, Alexander M, Albers G, Broderick J, Marks MP, Heit JJ. DEFUSE 3 Investigators. Results From DEFUSE 3: Good Collaterals Are Associated With Reduced Ischemic Core Growth but Not Neurologic Outcome. Stroke 2019;50:632-8. [Crossref] [PubMed]
- Guenego A, Fahed R, Albers GW, Kuraitis G, Sussman ES, Martin BW, Marcellus DG, Olivot JM, Marks MP, Lansberg MG, Wintermark M, Heit JJ. Hypoperfusion intensity ratio correlates with angiographic collaterals in acute ischaemic stroke with M1 occlusion. Eur J Neurol 2020;27:864-70. [Crossref] [PubMed]
- Guenego A, Mlynash M, Christensen S, Kemp S, Heit JJ, Lansberg MG, Albers GW. Hypoperfusion ratio predicts infarct growth during transfer for thrombectomy. Ann Neurol 2018;84:616-20. [Crossref] [PubMed]
- Olivot JM, Mlynash M, Inoue M, Marks MP, Wheeler HM, Kemp S, Straka M, Zaharchuk G, Bammer R, Lansberg MG, Albers GW. DEFUSE 2 Investigators. Hypoperfusion intensity ratio predicts infarct progression and functional outcome in the DEFUSE 2 Cohort. Stroke 2014;45:1018-23. [Crossref] [PubMed]
- Powers WJ, Rabinstein AA, Ackerson T, Adeoye OM, Bambakidis NC, Becker K, Biller J, Brown M, Demaerschalk BM, Hoh B, Jauch EC, Kidwell CS, Leslie-Mazwi TM, Ovbiagele B, Scott PA, Sheth KN, Southerland AM, Summers DV, Tirschwell DLAmerican Heart Association Stroke Council. 2018 Guidelines for the Early Management of Patients With Acute Ischemic Stroke: A Guideline for Healthcare Professionals From the American Heart Association/American Stroke Association. Stroke 2018;49:e46-110. Erratum in: Stroke 2018;49:e138 Erratum in: Stroke 2018;49:e233-4.
- Greener JG, Kandathil SM, Moffat L, Jones DT. A guide to machine learning for biologists. Nat Rev Mol Cell Biol 2022;23:40-55. [Crossref] [PubMed]
- Haug CJ, Drazen JM. Artificial Intelligence and Machine Learning in Clinical Medicine, 2023. N Engl J Med 2023;388:1201-8. [Crossref] [PubMed]
- Menon BK, d'Esterre CD, Qazi EM, Almekhlafi M, Hahn L, Demchuk AM, Goyal M, Multiphase CT. Angiography: A New Tool for the Imaging Triage of Patients with Acute Ischemic Stroke. Radiology 2015;275:510-20. [Crossref] [PubMed]
- Yesilyaprak A, Kumar AK, Agrawal A, Furqan MM, Verma BR, Syed AB, Majid M, Akyuz K, Rayes DL, Chen D, Kai Ming Wang T, Cremer PC, Klein AL. Predicting Long-Term Clinical Outcomes of Patients With Recurrent Pericarditis. J Am Coll Cardiol 2024;84:1193-204. [Crossref] [PubMed]
- Faizy TD, Mlynash M, Kabiri R, Christensen S, Kuraitis G, Meyer L, Bechstein M, Van Horn N, Lansberg MG, Albers G, Fiehler J, Wintermark M, Heit JJ. Favourable arterial, tissue-level and venous collaterals correlate with early neurological improvement after successful thrombectomy treatment of acute ischaemic stroke. J Neurol Neurosurg Psychiatry 2022; Epub ahead of print. [Crossref]
- Faizy TD, Mlynash M, Kabiri R, Christensen S, Kuraitis GM, Mader MM, Flottmann F, Broocks G, Lansberg MG, Albers GW, Marks MP, Fiehler J, Wintermark M, Heit JJ. The Cerebral Collateral Cascade: Comprehensive Blood Flow in Ischemic Stroke. Neurology 2022;98:e2296-306. [Crossref] [PubMed]
- Faizy TD, Kabiri R, Christensen S, Mlynash M, Kuraitis GM, Broocks G, Flottmann F, Marks MP, Lansberg MG, Albers GW, Fiehler J, Wintermark M, Heit JJ. Favorable Venous Outflow Profiles Correlate With Favorable Tissue-Level Collaterals and Clinical Outcome. Stroke 2021;52:1761-7. [Crossref] [PubMed]
- Rao VL, Mlynash M, Christensen S, Yennu A, Kemp S, Zaharchuk G, Heit JJ, Marks MP, Lansberg MG, Albers GW. Collateral status contributes to differences between observed and predicted 24-h infarct volumes in DEFUSE 3. J Cereb Blood Flow Metab 2020;40:1966-74. [Crossref] [PubMed]
- Liu L, Su X, Zhang L, Li Z, Bu K, Yuan S, Wang Q, Wang Y, Aime NJ, Liu Z, Zhou C, Yu J, Tan G, Guo L, Liu X. Cerebral collateral circulation as an independent predictor for in-stent restenosis after carotid artery stenting. Quant Imaging Med Surg 2023;13:2941-52. [Crossref] [PubMed]
- Yu AY, Zerna C, Assis Z, Holodinsky JK, Randhawa PA, Najm M, Goyal M, Menon BK, Demchuk AM, Coutts SB, Hill MD. Multiphase CT angiography increases detection of anterior circulation intracranial occlusion. Neurology 2016;87:609-16. [Crossref] [PubMed]
- Byrne D, Sugrue G, Stanley E, Walsh JP, Murphy S, Kavanagh EC, MacMahon PJ. Improved Detection of Anterior Circulation Occlusions: The "Delayed Vessel Sign" on Multiphase CT Angiography. AJNR Am J Neuroradiol 2017;38:1911-6. [Crossref] [PubMed]
- Shi F, Gong X, Liu C, Zeng Q, Zhang M, Chen Z, Yan S, Lou M. Acute Stroke: Prognostic Value of Quantitative Collateral Assessment at Perfusion CT. Radiology 2019;290:760-8. [Crossref] [PubMed]
- Agarwal S, Bivard A, Warburton E, Parsons M, Levi C. Collateral response modulates the time-penumbra relationship in proximal arterial occlusions. Neurology 2018;90:e316-22. [Crossref] [PubMed]
- Liebeskind DS, Saber H, Xiang B, Jadhav AP, Jovin TG, Haussen DC, Budzik RF, Bonafe A, Bhuva P, Yavagal DR, Hanel RA, Ribo M, Cognard C, Sila C, Hassan AE, Smith WS, Saver JL, Nogueira RG. DAWN Investigators. Collateral Circulation in Thrombectomy for Stroke After 6 to 24 Hours in the DAWN Trial. Stroke 2022;53:742-8. [Crossref] [PubMed]
- Niu Y, Zhou Y, Chen R, Yin P, Meng X, Wang W, Liu C, Ji JS, Qiu Y, Kan H, Zhou M. Long-term exposure to ozone and cardiovascular mortality in China: a nationwide cohort study. Lancet Planet Health 2022;6:e496-503. [Crossref] [PubMed]
- Lin CH, Hsu KC, Johnson KR, Fann YC, Tsai CH, Sun Y, Lien LM, Chang WL, Chen PL, Lin CL, Hsu CYTaiwan Stroke Registry Investigators. Evaluation of machine learning methods to stroke outcome prediction using a nationwide disease registry. Comput Methods Programs Biomed 2020;190:105381. [Crossref] [PubMed]
- Heit JJ, Mlynash M, Kemp SM, Lansberg MG, Christensen S, Marks MP, Ortega-Gutierrez S, Albers GW. Rapid Neurologic Improvement Predicts Favorable Outcome 90 Days After Thrombectomy in the DEFUSE 3 Study. Stroke 2019;50:1172-7. [Crossref] [PubMed]
- Meyer L, Broocks G, Bechstein M, Flottmann F, Leischner H, Brekenfeld C, Schön G, Deb-Chatterji M, Alegiani A, Thomalla G, Fiehler J, Kniep H, Hanning U. Early clinical surrogates for outcome prediction after stroke thrombectomy in daily clinical practice. J Neurol Neurosurg Psychiatry 2020;91:1055-9. [Crossref] [PubMed]
- Weyland CS, Mokli Y, Vey JA, Kieser M, Herweh C, Schönenberger S, Bendszus M, Möhlenbruch MA, Ringleb PA, Nagel S. Predictors for Failure of Early Neurological Improvement After Successful Thrombectomy in the Anterior Circulation. Stroke 2021;52:1291-8. [Crossref] [PubMed]
- Agarwal S, Scher E, Lord A, Frontera J, Ishida K, Torres J, Rostanski S, Mistry E, Mac Grory B, Cutting S, Burton T, Silver B, Liberman AL, Lerario MP, Furie K, Grotta J, Khatri P, Saver J, Yaghi S. Redefined Measure of Early Neurological Improvement Shows Treatment Benefit of Alteplase Over Placebo. Stroke 2020;51:1226-30. [Crossref] [PubMed]
- Guenego A, Marcellus DG, Martin BW, Christensen S, Albers GW, Lansberg MG, Marks MP, Wintermark M, Heit JJ. Hypoperfusion Intensity Ratio Is Correlated With Patient Eligibility for Thrombectomy. Stroke 2019;50:917-22. [Crossref] [PubMed]
- Nael K, Doshi A, De Leacy R, Puig J, Castellanos M, Bederson J, Naidich TP, Mocco J, Wintermark M. MR Perfusion to Determine the Status of Collaterals in Patients with Acute Ischemic Stroke: A Look Beyond Time Maps. AJNR Am J Neuroradiol 2018;39:219-25. [Crossref] [PubMed]