Artificial intelligence electrocardiogram as a novel screening tool to detect and longitudinally monitor high-risk echocardiographic features in hypertrophic cardiomyopathy
Introduction
Hypertrophic cardiomyopathy (HCM) is one of the most prevalent genetic diseases, with an estimated prevalence of 1:200 in the general population (1). It is the leading cause of sudden cardiac death (SCD) in young people, and patients with HCM also have an increased risk of heart failure with a worse long-term prognosis (2,3). Improvements in targeted medical therapy and internal cardioverter defibrillator (ICD) implantation have provided effective protection in patients with HCM (4,5). Therefore, early identification of high-risk patients with HCM who may benefit from myosin inhibitor and ICD therapy can significantly reduce HCM-related morbidity and mortality (6,7).
Echocardiography is the first-line imaging modality for HCM diagnosis, providing valuable information on hemodynamic severity and risk status (8). The current American Society of Echocardiography (ASE) practice protocol for HCM recommends a specific echocardiographic protocol during periodic follow-up, and integrates crucial high-risk echocardiographic features into patient management to assess changes in phenotype severity (9), including left ventricular outflow tract obstruction (LVOTO) and risk markers for SCD [massive left ventricular hypertrophy (LVH), left ventricular (LV) apical aneurysm, and LV ejection fraction (LVEF) <50%]. However, the high cost and limited resource coverage of echocardiography make its use as a widespread screening and routine management tool in rural communities difficult (10).
Electrocardiogram (ECG) is a cost-effective and widely available diagnostic tool that is often considered the initial procedure in cardiology and can be performed in any clinical setting. Recently, artificial intelligence (AI) technologies have demonstrated their feasibility for ECG-based disease diagnosis, achieving remarkable accuracy in a wide range of cardiovascular conditions, such as cardiac systolic dysfunction (11), hemodynamic abnormalities (12), and structural heart disease including HCM (13,14). Therefore, the aim of this study was to develop an AI-ECG model for the identification of high-risk echocardiographic features and to explore the potential of the model for longitudinal monitoring of the risk status of patients with HCM, allowing prioritization of echocardiographic services. This approach will contribute to reducing diagnostic delays and facilitate effective stratified management in the community. We present this article in accordance with the TRIPOD+AI reporting checklist (available at https://qims.amegroups.com/article/view/10.21037/qims-24-1638/rc).
Methods
Data collection
Patients aged 18 years or older who underwent comprehensive echocardiography and 12-lead ECG within a 14-day window with a clinical diagnosis of HCM at the First Hospital of China Medical University between January 2013 and June 2023 were retrospectively identified [China Medical University (CMU) cohort]. Patients with HCM were defined as those with a maximum LV wall thickness exceeding 15 mm, or exceeding 13 mm with a family history of HCM and no other cardiac or systemic diseases that could cause LVH (8). For patients with multiple ECG-echocardiogram pairs, only the earliest pair with the smallest time interval was used for model development. ECGs with the presence of ventricular pacing, left bundle branch block, poor diagnostic quality, and echocardiograms showing myectomy or myocardial ablation were excluded. All eligible patients in the CMU cohort were randomized into training (70%), validation (10%), and testing (20%) sets, with no patient overlap among the three groups. An independent cohort of patients from the Dalian Municipal Friendship Hospital was evaluated for external validation (Friendship cohort). Details are described in Figure 1.
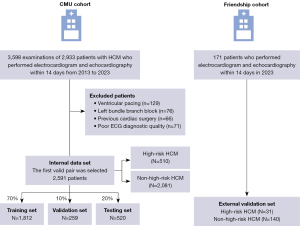
Standard 10-second, 12-lead ECG data recorded at a frequency of 500 Hz were obtained in XML format and exported from the MUSE ECG database. These files contained demographic information and specific details of the ECG, such as unique identifiers, age, sex, heart rate, PR interval, QRS duration, QT interval, and QTc (using Bazett’s correction; QT/RR1/2).
Echocardiographic data were extracted from echocardiographic reports using a unique patient identifier and manually validated. This dataset encompassed measurements of interventricular septal thickness, LV posterior wall thickness, maximum wall thickness, LVEF, observations regarding the HCM hemodynamic phenotype (obstructive or non-obstructive), presence or absence of LV apical aneurysm.
The study was conducted according to the ethical guidelines of Declaration of Helsinki (as revised in 2013). The study protocol was approved by the Ethics Committee of the First Hospital of China Medical University (No. 2022-116) and individual consent for this retrospective analysis was waived.
Cohort definitions and labeling
High-risk echocardiographic features
Echocardiographic high-risk features were further defined according to the 2023 ASE practical imaging approach and the 2020 American College of Cardiology/American Heart Association (ACC/AHA) enhanced strategy (7,9).
- Resting LVOTO (defined as LV outflow pressure gradient ≥30 mmHg under resting condition).
- Massive LVH (defined as maximum LV wall thickness ≥30 mm).
- LV apical aneurysm.
- LVEF ≤50%.
Patients with HCM who had a positive echocardiogram for any of the above four criteria were defined as the high-risk group. Patients with HCM who did not meet all of the above four criteria were referred to as the non-high-risk group.
Model development
The 10-second, 12-lead ECG data at a frequency of 500 Hz were converted into a 5,000×12 matrix. A Butterworth bandpass filter with a cutoff frequency range of 1–40 Hz was applied to all ECG signals. We developed a multi-attention convolutional neural network (MANet), leveraging a ResNet-18 backbone with one-dimensional residual convolutional blocks (15). The MANet incorporates both local and global spatial attention mechanisms to fuse information from 12-lead ECG data and produce a probability score between zero and one corresponding to high-risk echocardiographic features (Figure S1). This design promotes the enhanced learning of key ECG signal attributes, including both amplitude and waveform characteristics. The details of MANet development and training are provided in the supplementary methods (Appendix 1).
Model evaluation
Initial analyses focused on the performance of the model in identifying high-risk echocardiographic features in HCM using 12-lead ECG. Optimal thresholds for corrected disease classification were calculated using Youden’s J index in the validation set. The performance of the model in detecting any of the four high-risk features was subsequently analyzed on the testing set from the CMU cohort, together with an external validation set from the Friendship cohort. Patient clinical and ECG characteristics were compared among the true-positive, false-positive, true-negative, and false-negative groups in the testing set. Additionally, the same MANet architecture and datasets from the CMU cohort were used for training and testing using single-lead ECG (lead I), which is most commonly used in wearable monitoring devices.
Subgroup analyses evaluated model performance using 12-lead ECG in subsets defined by sex and age groups in the testing set. In addition, the trained model was also tested on resting LVOTO subtype and risk marker subtype (including any of massive LVH, LV apical aneurysm, and LVEF ≤50%).
Model explainability
To investigate the effect of voltage features on the decision-making ability of the MANet model for the detection of high-risk echocardiographic features, saliency mapping was used to highlight the patterns of activation.
Model performance in longitudinal monitoring high-risk echocardiographic features
To access the model’s performance in monitoring longitudinal changes in HCM risk status over time, patients in the testing set who were followed with more than two pairs of ECG-echocardiogram data were tested. We examined the longitudinal associations between the model’s predicted probability score and echocardiographically-derived risk status. In addition, all patients with negative echocardiograms were categorized as either model-derived false-positives or true-negatives, and we tested the incidence of development of high-risk echocardiographic features over time for both groups.
Statistical analysis
The performance of the ECG model in detecting high-risk echocardiographic features in HCM patients was evaluated by calculating the area under the receiver operating characteristic curve (AUROC), area under the precision-recall curve (AUPRC), sensitivity, specificity, positive predictive value (PPV), negative predictive value (NPV), and accuracy. Two-sided 95% confidence intervals (95% CIs) were calculated. The diagnostic odd ratio was computed as the ratio of the positive likelihood ratio to the negative likelihood ratio.
Continuous variables were presented as mean ± standard deviation and compared using the one-way analysis of variance (ANOVA) test or paired t-test. Categorical variables were presented as frequencies (percentages) and evaluated using the χ2 test or the Fisher exact test. The log-rank test was applied and hazard ratios (HRs) were calculated to quantify the time difference between model-derived false-positive and true-negative and the development of echocardiographically-derived high-risk HCM. A two-side P<0.05 was considered statistically significant. All statistical analyses were performed with Python version 3.7.13 and R 4.3.0.
Results
Study population
A total of 2,591 patients with HCM who had valid ECG-echocardiogram pairs were retrospectively identified in the CMU cohort. The median interval between ECG and echocardiography was zero days. In the entire cohort of patients with HCM, high-risk echocardiographic features were observed in 510 patients (19.7%), including 430 (16.6%) with resting LVOTO, 63 (2.4%) with massive LVH, 10 (0.4%) with LV apical aneurysm, and 43 (1.7%) with LVEF ≤50%. Of these, a total of 33 patients exhibited at least one high-risk feature. Patients with high-risk features were younger and had a higher proportion of New York Heart Association (NYHA) functional class III/IV (P<0.05). Patient characteristics remained consistent across training, validation and testing sets. External validation was performed using the Friendship cohort of 171 patients with HCM, of whom 31 (18.1%) were classified as high-risk and the remaining 140 (81.9%) were classified as non-high-risk based on echocardiographic findings. Patient characteristics are shown in Table 1.
Table 1
Characteristics | CMU cohort (n=2,591) | Friendship cohort (n=171) | |||||
---|---|---|---|---|---|---|---|
High-risk (n=510) |
Non-high-risk (n=2,081) |
P value | High-risk (n=31) |
Non-high-risk (n=140) |
P value | ||
Age, years | 54.4±13.8 | 57.3±13.9 | 0.005** | 58.5±10.8 | 61.3±11.8 | 0.238 | |
Males | 283 (55.5) | 1,419 (68.2) | <0.001*** | 16 (51.6) | 106 (75.7) | 0.007** | |
NYHA class I/II | 299 (58.6) | 1,518 (72.9) | <0.001*** | 19 (61.3) | 110 (78.6) | 0.043* | |
NYHA class III/IV | 211 (41.4) | 563 (27.1) | <0.001*** | 12 (38.7) | 30 (21.4) | 0.043* | |
ECG features | |||||||
Heart rate (b/min) | 75.9±15.1 | 74.2±15.4 | 0.005** | 75.5±12.0 | 72.9±12.6 | <0.001*** | |
SL ECG criteria for LVH | 240 (47.1) | 901 (43.3) | 0.125 | 19 (61.3) | 66 (47.1) | 0.154 | |
“Normal ECG” | 19 (3.7) | 128 (6.2) | 0.034* | 0 (0.0) | 10 (7.1) | – | |
Atrial fibrillation | 38 (7.5) | 183 (8.8) | 0.331 | 2 (6.5) | 8 (5.7) | 0.876 | |
Echocardiographic features | |||||||
Maximum LV wall thickness, mm | 20.7±4.9 | 18.2±3.2 | <0.001*** | 21.7±4.8 | 18.3±2.7 | <0.001*** | |
Septum thickness, mm | 19.8±5.0 | 16.7±4.3 | <0.001*** | 20.9±5.1 | 16.3±3.8 | <0.001*** | |
Posterior thickness, mm | 11.6±3.0 | 10.9±2.9 | <0.001*** | 12.7±3.5 | 10.8±2.4 | <0.001*** | |
LV diastolic diameter, mm | 46.2±5.1 | 47.5±4.9 | <0.001*** | 46.1±3.8 | 48.0±3.9 | 0.038* | |
Left atrial diameter, mm | 43.4±6.0 | 41.6±6.1 | <0.001*** | 43.3±5.6 | 41.7±6.2 | 0.105 | |
LVEF, % | 61.5±7.4 | 63.0±4.3 | 0.002** | 62.2±3.6 | 62.6±3.2 | 0.394 | |
Resting LVOTO | 430 (84.3) | 0 (0.0) | – | 25 (80.6) | 0 (0.0) | – | |
Massive LV hypertrophy | 63 (12.4) | 0 (0.0) | – | 2 (6.5) | 0 (0.0) | – | |
LV apical aneurysm | 10 (2.0) | 0 (0.0) | – | 2 (6.5) | 0 (0.0) | – | |
LVEF <50% | 43 (8.4) | 0 (0.0) | – | 3 (9.7) | 0 (0.0) | – |
Data are presented as mean ± standard deviation or n (%). *, P<0.05; **, P<0.01; ***, P<0.001. CMU, China Medical University; NYHA, New York Heart Association; ECG, electrocardiogram; SL, Sokolow-Lyon criteria; LVH, left ventricular hypertrophy; LV, left ventricular; LVEF, left ventricular ejection fraction; LVOTO, left ventricular outflow tract obstruction.
Model performance in identifying high-risk echocardiographic features
The threshold for optimal probability of classification of the composite high-risk echocardiographic features was 19.6%. The AUROC and AUPRC for identifying any of the high-risk features were 0.81 (95% CI: 0.76–0.86) and 0.54 (95% CI: 0.45–0.64), respectively (Figure 2A,2B, Table S1). Out of the 520 patients in the testing set, 186 patients were predicted to have high-risk features with a sensitivity of 80%, specificity of 76%, PPV of 47% and NPV of 93%. There were 16.7% (n=87) true-positives, 60.0% (n=312) true negatives, 19.0% (n=99) false-positives, and 4.2% (n=22) false-negatives. The specific characteristics of the MANet model for the detection of high-risk features were further compared among the four groups (Table 2). The ECG characteristics of pathologic q-waves and QT interval were significantly different (P<0.001), but those of QTc were not statistically significant among the four groups.
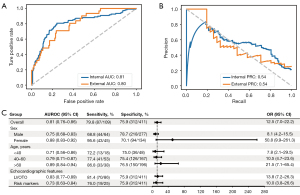
Table 2
Characteristics | True positive (n=87) | True negative (n=312) | False positive (n=99) | False negative (n=22) | P value |
---|---|---|---|---|---|
Age, years | 54.8±13.9 | 58.3±13.6† | 57.3±13.9 | 50.4±12.7‡§ | 0.02* |
Males | 44 (50.6) | 217 (69.6)† | 59 (59.6) | 20 (90.9)†‡§ | <0.001*** |
Heart rate (b/min) | 78.1±18.2 | 71.9±14.6† | 78.6±16.2‡ | 71.0±12.9§ | <0.001*** |
SL ECG criteria for LVH | 39 (44.8) | 141 (45.2) | 37 (37.4) | 12 (54.5) | 0.398 |
Atrial fibrillation | 4 (4.6) | 21 (6.7) | 8 (8.1) | 3 (13.6) | 0.520 |
Right bundle branch block | 1 (1.1) | 29 (9.3)† | 3 (3.0) | 1 (4.5) | 0.007** |
Pathologic q-wave | 22 (25.3) | 35 (11.2)† | 24 (24.2)‡ | 1 (4.5)†§ | <0.001*** |
Atrioventricular block | 8 (9.2) | 28 (9.0) | 7 (7.1) | 1 (4.5) | 0.816 |
PR interval, ms | 161.3±25.6 | 164.4±30.9 | 165.2±23.3 | 154.2±21.3 | 0.326 |
QRS interval, ms | 108.2±11.4 | 108.0±17.9 | 106.7±17.4 | 110.7±12.5 | 0.759 |
QT interval, ms | 392.7±37.2 | 409.5±43.7† | 389.9±39.2‡ | 421.3±36.1†§ | <0.001*** |
QTc interval, ms | 442.1±28.4 | 442.6±32.2 | 438.3±23.3 | 452.0±31.4 | 0.256 |
Data are presented as mean ± standard deviation or n (%). *, P<0.05, **, P<0.01, ***, P<0.001. †, compared with true positive group, P<0.05; ‡, compared with true negative group, P<0.05; §, compared with false positive group, P<0.05. SL, Sokolow-Lyon criteria; ECG, electrocardiogram; LVH, left ventricular hypertrophy; QTc, corrected QT interval.
Further analysis stratifying by sex and age revealed that the accuracy of MANet was higher in women than in men. Additionally, there was a noticeable trend toward improved sensitivity and specificity with increasing age. Model performance was then evaluated separately in patients with the resting LVOTO subtype and the SCD-related risk marker subtype, as these subtypes are associated with different clinical management decisions. The AUROC values were 0.83 (95% CI: 0.77–0.89) and 0.73 (95% CI: 0.63–0.84) for each subtype, respectively. The results of the subgroup analysis are shown in Figure 2C.
Independent validation using the Friendship cohort demonstrated that the model remained discriminatory with an AUROC of 0.80 (95% CI: 0.72–0.87) and an AUPRC of 0.54 (95% CI: 0.41–0.67), as shown in Figure 2A,2B, and Table S1.
Considering that wearable ECG monitoring devices typically capture less than 12 leads, the model was further trained exclusively on ECG lead I. It exhibited an only slightly lower AUROCs of 0.73 (95% CI: 0.67–0.87) and 0.74 (95% CI: 0.65–0.82) in both the testing and external validation sets, respectively (Figure S2 and Table S1).
Model explainability
To gain a deeper understanding of the features for model prediction, we applied the saliency map to 100 ECGs with and without high-risk echocardiographic features. In general, the saliency map showed a primary focus on the QRS complex and relatively less attention to the T-wave region when predicting high-risk features (Figure 3A). In cases lacking high-risk features, the model appears to focus solely on the QRS complex (Figure 3B).
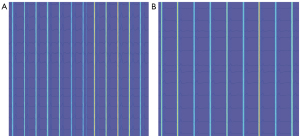
Model performance with serial ECG analysis in longitudinal disease monitoring
Of the 411 patients in the testing group who did not exhibit high-risk echocardiographic features at initial assessment, 30 had follow-up ECG-echocardiogram pairs. By applying MANet to the ECGs of patients with HCM at both time points, we obtained a progression of probability scores over time corresponding to their risk status (Figure 4A). The blue dots represent the initial high-risk scores when HCM was first diagnosed, while the red dots represent the most recent risk scores. Among these patients, ten developed new high-risk echocardiographic features during a median follow-up of 37 months (interquartile range, 30–53 months), as indicated by an increasing trend in the predicted probability score (19.7% vs. 30.2%, P<0.05). Patients whose risk status remained unchanged had stable scores within a narrow range (11.3% vs. 12.6%, P>0.05).
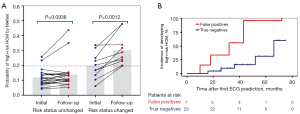
Furthermore, among the 30 individuals without high-risk features, there were 23 true-negatives and 7 false-positives in the initial MANet classification. Notably, the false-positive group had a threefold increased risk of developing high-risk echocardiographic features compared with the true-negative group (HR 3.36, 95% CI: 0.77–14.65; P=0.037), as shown in Figure 4B.
Discussion
As far as we know, the present study is the first exploratory investigation using an AI-enabled ECG model to identify high-risk echocardiographic features in patients with HCM for risk stratification, including resting LVOTO, massive LVH, LV apical aneurysm and LVEF ≤50%. The most important finding was that the MANet exhibited good performance in identifying any of the aforementioned high-risk echocardiographic features using both 12-lead and single-lead ECG. Meanwhile, the model also performed well in an independent validation set. Additionally, the predicted probability score of the model was well aligned with the changes of risk status in patients with HCM.
AI-ECG model can accurately identify high-risk echocardiographic features
The current ASE practice protocol and AHA/ACC guidelines for HCM recommend the integration of crucial echocardiographic features (8,9), including comprehensive assessment of LVOTO and high-risk features predictive of SCD, into the routine management of HCM patients. Unfortunately, not all patients with HCM have access to specialized echocardiography services at initial evaluation and regular follow-up every one to two years thereafter, as obtaining all these data necessitates specialists with expertise in echocardiography, which are not readily available in underdeveloped areas. As a simple and reproducible method, ECG is widely used to detect cardiovascular disease, even in rural areas and health clinics. Previous studies have demonstrated the potential utility of AI-ECG in detecting HCM (13,14). However, simple detection of HCM is not always sufficient, a comprehensive assessment of echocardiographic features for risk stratification is crucial for appropriate management. For the first time, our research considered the existing disparities in the diagnosis and management of HCM in the community and employed AI-ECG to evaluate the integrated high-risk echocardiographic features and guide the appropriate allocation of echocardiography for downstream clinical management (10). Despite the relatively limited sample size of patients with HCM, our model also performed well in identifying these high-risk echocardiographic features, achieving a sensitivity of 80% and demonstrating its effectiveness in screening the majority of high-risk patients. Another important clinical value of our model is its high NPV of 0.93, indicating that the model could be used to exclude patients without high-risk features and facilitate effective stratified management.
Although the model remained accurate across all age cohorts, diagnostic performance was optimal in older patients, particularly those over 60 years of age. It should be noted that LV remodeling and cardiac function alteration may manifest more dramatically on ECG signals, as evidenced by their correlation with advancing age and longer disease duration. According to recent data, elderly patients with HCM exhibit a relatively lower SCD event rate (16), but a more unfavorable prognosis for heart failure during long-term follow-up (17). Current guidelines present a “grey zone” for risk assessment regarding ongoing cardiac imaging in this subset of patients. Although individualized decision making is recommended, no tools are provided for such personalized assessment (8). Therefore, our model may serve as a supportive tool for this subset of patients and guide the decision to undergo echocardiography. In addition, women with HCM appear to have a significantly greater degree of remodeling and a higher risk of adverse cardiovascular events (10,18,19). The finding of more sensitive predictive performance in women suggests that a significant number of female cases with poor outcome could be identified in time (20).
It is noteworthy that SCD-related cardiovascular events are the most serious adverse outcomes, while their low incidence poses a challenge in predicting SCD, which occurs at a rate of only 0.3–3% per 1,000 person-years (21). To overcome the limitation of a low event rate, we employed the composite endpoint comprising high-risk echocardiographic features that characterize adverse outcomes associated with SCD (8). A recent study confirmed the potential of screening cardiac imaging features using AI-ECG to refine the selection process for cardiac magnetic resonance (22). Our results corroborate this association and demonstrate favorable performance in subtype analysis to identify SCD-related risk markers, achieving an AUROC of 0.73.
In addition to the most severe SCD-related events, patients with heart failure also experience a poor prognosis. Symptoms of heart failure are usually due to long-term LVOTO (23). Accurate detection of LVOTO is crucial to guide clinical decision making for effective targeted treatment of symptomatic obstructive HCM (24,25). While a previous study validated that the AI-ECG score for predicting HCM correlates with the obstructive status in patients with HCM (26), the model reported here is the first attempt to explore the potential utility of AI-ECG in directly detecting LVOTO. To our satisfaction, our model exhibited excellent diagnostic performance in identifying the resting LVOTO subtype with an AUROC of 0.83, which may serve as an effective screening tool to prevent delayed referral in resource-limited settings. Notably, this was a retrospective study, some patients may not have been adequately provoked during previous examinations. Therefore, we specifically focused on resting LVOTO cases, and some potentially high-risk patients based on provocation may have been misclassified. However, resting ECG data are consistent with the measurement status of echocardiographic parameters, and resting LVOTO is associated with worse clinical outcomes (27,28).
In an AI model, it is important to know which ECG features contribute to the model’s prediction. A comparative analysis of ECG characteristics revealed significant differences only in the QT interval, while no variation was observed in the QTc, suggesting its limited utility in identifying high-risk HCM. Further analysis using the saliency map showed a robust correlation between the QRS complex and a positive label, consistent with previous evidence that abnormalities in the QRS complex may predict an increased risk of SCD (29).
Clinical utility of the AI-ECG model
Although the AI-ECG model does not play a central role in the risk stratification of patients with HCM, it can provide an additional method to monitor disease progression and assist clinicians in determining the need for further echocardiography and prompt reassessment (7). There is a positive correlation between the probability score and the risk status of patients over long-term follow-up, i.e., distinct ECG changes develop as the severity of the status progresses. Consequently, our model has the potential to serve as a novel predictor of future high-risk features and may provide a viable approach for longitudinal follow-up. Furthermore, patients with false-positive results have an increased risk of developing high-risk features in the future compared to those with true-positive results. This suggests that patients with positive AI-ECG results warrant more frequent surveillance. Another important clinical benefit of this finding is the ability to identify high-risk patients earlier in their disease course, in line with clinical needs and advancing risk management, thereby reducing the likelihood of high-risk individuals missing the optimal time for referral to specialist clinics and improving outcomes (30).
Moreover, the consistent diagnostic accuracy in detecting high-risk echocardiographic features in an independent external validation set suggests that the AI-ECG model is a robust classifier with high generalizability. Further, the promising performance of our model trained on single-lead ECG greatly extends its potential utility beyond the clinical setting, as it can be integrated into the increasingly used wearable ECG monitoring devices.
Limitations
There are some limitations in this study. First, this study used echocardiographic determination, a small number of high-risk patients may have been unidentified based on echocardiographic results. However, the echocardiography is the first-line test and a clinically accepted tool for HCM diagnosis and has been adopted in ACC/AHA guidelines. Second, this was a retrospective study, some patients were not adequately provoked during previous examinations, which may have led to misclassification of potentially high-risk patients. In a future prospective study, we will attempt to confirm the feasibility of the model in predicting latent LVOTO using ECG. Third, because of the low incidence of SCD-related or heart failure-related adverse cardiovascular events, the ECG model was trained only to identify high-risk echocardiographic features. It would be more convincing to evaluate the model’s performance in risk stratification by monitoring patient adverse outcomes during long-term follow-up.
Conclusions
In conclusion, our AI-ECG model can accurately identify and longitudinally monitor high-risk echocardiographic features, making it a powerful community screening tool that can optimize the timing of intervention and prevent adverse events.
Acknowledgments
We thank Editage for English language review.
Footnote
Reporting Checklist: The authors have completed the TRIPOD+AI reporting checklist. Available at https://qims.amegroups.com/article/view/10.21037/qims-24-1638/rc
Funding: This work was supported by
Conflicts of Interest: All authors have completed the ICMJE uniform disclosure form (available at https://qims.amegroups.com/article/view/10.21037/qims-24-1638/coif). All authors report that this study was supported by the National Natural Science Foundation of China (grant No. U21A20387), Science and the Technology Funding Projects to Support the High-Quality Development of China Medical University (grant No. 2023JH2/20200066), and the Basic Research Project for Higher Education Institutions by the Department of Education of Liaoning Province (grant No. LJ 232410159055). The authors have no other conflicts of interest to declare.
Ethical Statement: The authors are accountable for all aspects of the work in ensuring that questions related to the accuracy or integrity of any part of the work are appropriately investigated and resolved. This study was conducted in accordance with the Declaration of Helsinki (as revised in 2013). The study protocol was approved by the Ethics Committee of the First Hospital of China Medical University (No. 2022-116) and individual consent for this retrospective analysis was waived.
Open Access Statement: This is an Open Access article distributed in accordance with the Creative Commons Attribution-NonCommercial-NoDerivs 4.0 International License (CC BY-NC-ND 4.0), which permits the non-commercial replication and distribution of the article with the strict proviso that no changes or edits are made and the original work is properly cited (including links to both the formal publication through the relevant DOI and the license). See: https://creativecommons.org/licenses/by-nc-nd/4.0/.
References
- Maron BJ. Clinical Course and Management of Hypertrophic Cardiomyopathy. N Engl J Med 2018;379:655-68. [Crossref] [PubMed]
- Maron BJ, Haas TS, Murphy CJ, Ahluwalia A, Rutten-Ramos S. Incidence and causes of sudden death in U.S. college athletes. J Am Coll Cardiol 2014;63:1636-43. [Crossref] [PubMed]
- Maron BJ, Rowin EJ, Udelson JE, Maron MS. Clinical Spectrum and Management of Heart Failure in Hypertrophic Cardiomyopathy. JACC Heart Fail 2018;6:353-63. [Crossref] [PubMed]
- Olivotto I, Oreziak A, Barriales-Villa R, Abraham TP, Masri A, Garcia-Pavia P, et al. Mavacamten for treatment of symptomatic obstructive hypertrophic cardiomyopathy (EXPLORER-HCM): a randomised, double-blind, placebo-controlled, phase 3 trial. Lancet 2020;396:759-69. [Crossref] [PubMed]
- Maron BJ, Spirito P, Ackerman MJ, Casey SA, Semsarian C, Estes NA 3rd, et al. Prevention of sudden cardiac death with implantable cardioverter-defibrillators in children and adolescents with hypertrophic cardiomyopathy. J Am Coll Cardiol 2013;61:1527-35. [Crossref] [PubMed]
- Authors/Task Force members. 2014 ESC Guidelines on diagnosis and management of hypertrophic cardiomyopathy: the Task Force for the Diagnosis and Management of Hypertrophic Cardiomyopathy of the European Society of Cardiology (ESC). Eur Heart J 2014;35:2733-79. [Crossref] [PubMed]
- Ommen SR, Mital S, Burke MA, Day SM, Deswal A, Elliott P, Evanovich LL, Hung J, Joglar JA, Kantor P, Kimmelstiel C, Kittleson M, Link MS, Maron MS, Martinez MW, Miyake CY, Schaff HV, Semsarian C, Sorajja P. 2020 AHA/ACC Guideline for the Diagnosis and Treatment of Patients With Hypertrophic Cardiomyopathy: Executive Summary: A Report of the American College of Cardiology/American Heart Association Joint Committee on Clinical Practice Guidelines. J Am Coll Cardiol 2020;76:3022-55. [Crossref] [PubMed]
- Ommen SR, Ho CY, Asif IM, Balaji S, Burke MA, et al. 2024 AHA/ACC/AMSSM/HRS/PACES/SCMR Guideline for the Management of Hypertrophic Cardiomyopathy: A Report of the American Heart Association/American College of Cardiology Joint Committee on Clinical Practice Guidelines. J Am Coll Cardiol 2024;83:2324-405. [Crossref] [PubMed]
- Mitchell CC, Frye C, Jankowski M, Symanski J, Lester SJ, Woo A, Gilliland Y, Dragulescu A, Abraham T, Desai M, Martinez MW, Nagueh SF, Phelan D. A Practical Approach to Echocardiographic Imaging in Patients With Hypertrophic Cardiomyopathy. J Am Soc Echocardiogr 2023;36:913-32. [Crossref] [PubMed]
- Johnson DY, Waken RJ, Fox DK, Hammond G, Joynt Maddox KE, Cresci S. Inequities in Treatments and Outcomes Among Patients Hospitalized With Hypertrophic Cardiomyopathy in the United States. J Am Heart Assoc 2023;12:e029930. [Crossref] [PubMed]
- Attia ZI, Kapa S, Lopez-Jimenez F, McKie PM, Ladewig DJ, Satam G, Pellikka PA, Enriquez-Sarano M, Noseworthy PA, Munger TM, Asirvatham SJ, Scott CG, Carter RE, Friedman PA. Screening for cardiac contractile dysfunction using an artificial intelligence-enabled electrocardiogram. Nat Med 2019;25:70-4. [Crossref] [PubMed]
- Aras MA, Abreau S, Mills H, Radhakrishnan L, Klein L, Mantri N, Rubin B, Barrios J, Chehoud C, Kogan E, Gitton X, Nnewihe A, Quinn D, Bridges C, Butte AJ, Olgin JE, Tison GH. Electrocardiogram Detection of Pulmonary Hypertension Using Deep Learning. J Card Fail 2023;29:1017-28. [Crossref] [PubMed]
- Ko WY, Siontis KC, Attia ZI, Carter RE, Kapa S, Ommen SR, Demuth SJ, Ackerman MJ, Gersh BJ, Arruda-Olson AM, Geske JB, Asirvatham SJ, Lopez-Jimenez F, Nishimura RA, Friedman PA, Noseworthy PA. Detection of Hypertrophic Cardiomyopathy Using a Convolutional Neural Network-Enabled Electrocardiogram. J Am Coll Cardiol 2020;75:722-33. [Crossref] [PubMed]
- Goto S, Solanki D, John JE, Yagi R, Homilius M, Ichihara G, Katsumata Y, Gaggin HK, Itabashi Y, MacRae CA, Deo RC. Multinational Federated Learning Approach to Train ECG and Echocardiogram Models for Hypertrophic Cardiomyopathy Detection. Circulation 2022;146:755-69. [Crossref] [PubMed]
- He K, Zhang X, Ren S, Sun J. Deep Residual Learning for Image Recognition. IEEE Conference on Computer Vision and Pattern Recognition (CVPR), Las Vegas, NV, USA; 2016:770-78.
- Maron BJ, Rowin EJ, Casey SA, Haas TS, Chan RH, Udelson JE, Garberich RF, Lesser JR, Appelbaum E, Manning WJ, Maron MS. Risk stratification and outcome of patients with hypertrophic cardiomyopathy >=60 years of age. Circulation 2013;127:585-93. [Crossref] [PubMed]
- Kitaoka H, Kubo T, Okawa M, Hitomi N, Furuno T, Doi YL. Left ventricular remodeling of hypertrophic cardiomyopathy: longitudinal observation in rural community. Circ J 2006;70:1543-9. [Crossref] [PubMed]
- Lu DY, Ventoulis I, Liu H, Kudchadkar SM, Greenland GV, Yalcin H, Kontari E, Goyal S, Corona-Villalobos CP, Vakrou S, Zimmerman SL, Abraham TP, Abraham MR. Sex-specific cardiac phenotype and clinical outcomes in patients with hypertrophic cardiomyopathy. Am Heart J 2020;219:58-69. [Crossref] [PubMed]
- Chen YZ, Zhao XS, Yuan JS, Zhang Y, Liu W, Qiao SB. Sex-related differences in left ventricular remodeling and outcome after alcohol septal ablation in hypertrophic obstructive cardiomyopathy: insights from cardiovascular magnetic resonance imaging. Biol Sex Differ 2022;13:37. [Crossref] [PubMed]
- Geske JB, Ong KC, Siontis KC, Hebl VB, Ackerman MJ, Hodge DO, Miller VM, Nishimura RA, Oh JK, Schaff HV, Gersh BJ, Ommen SR. Women with hypertrophic cardiomyopathy have worse survival. Eur Heart J 2017;38:3434-40. [Crossref] [PubMed]
- Aro AL, Nair SG, Reinier K, Jayaraman R, Stecker EC, Uy-Evanado A, Rusinaru C, Jui J, Chugh SS. Population Burden of Sudden Death Associated With Hypertrophic Cardiomyopathy. Circulation 2017;136:1665-7. [Crossref] [PubMed]
- Carrick RT, Ahamed H, Sung E, Maron MS, Madias C, Avula V, Studley R, Bao C, Bokhari N, Quintana E, Rajesh-Kannan R, Maron BJ, Wu KC, Rowin EJ. Identification of high-risk imaging features in hypertrophic cardiomyopathy using electrocardiography: A deep-learning approach. Heart Rhythm 2024;21:1390-7. [Crossref] [PubMed]
- Maron MS, Olivotto I, Zenovich AG, Link MS, Pandian NG, Kuvin JT, Nistri S, Cecchi F, Udelson JE, Maron BJ. Hypertrophic cardiomyopathy is predominantly a disease of left ventricular outflow tract obstruction. Circulation 2006;114:2232-9. [Crossref] [PubMed]
- Spertus JA, Fine JT, Elliott P, Ho CY, Olivotto I, Saberi S, Li W, Dolan C, Reaney M, Sehnert AJ, Jacoby D. Mavacamten for treatment of symptomatic obstructive hypertrophic cardiomyopathy (EXPLORER-HCM): health status analysis of a randomised, double-blind, placebo-controlled, phase 3 trial. Lancet 2021;397:2467-75. [Crossref] [PubMed]
- Maron BJ, Desai MY, Nishimura RA, Spirito P, Rakowski H, Towbin JA, Dearani JA, Rowin EJ, Maron MS, Sherrid MV. Management of Hypertrophic Cardiomyopathy: JACC State-of-the-Art Review. J Am Coll Cardiol 2022;79:390-414. [Crossref] [PubMed]
- Tison GH, Siontis KC, Abreau S, Attia Z, Agarwal P, Balasubramanyam A, Li Y, Sehnert AJ, Edelberg JM, Friedman PA, Olgin JE, Noseworthy PA. PIONEER-OLE Investigators. Assessment of Disease Status and Treatment Response With Artificial Intelligence-Enhanced Electrocardiography in Obstructive Hypertrophic Cardiomyopathy. J Am Coll Cardiol 2022;79:1032-4. [Crossref] [PubMed]
- Elliott PM, Gimeno JR, Tomé MT, Shah J, Ward D, Thaman R, Mogensen J, McKenna WJ. Left ventricular outflow tract obstruction and sudden death risk in patients with hypertrophic cardiomyopathy. Eur Heart J 2006;27:1933-41. [Crossref] [PubMed]
- Maron MS, Olivotto I, Betocchi S, Casey SA, Lesser JR, Losi MA, Cecchi F, Maron BJ. Effect of left ventricular outflow tract obstruction on clinical outcome in hypertrophic cardiomyopathy. N Engl J Med 2003;348:295-303. [Crossref] [PubMed]
- Biagini E, Pazzi C, Olivotto I, Musumeci B, Limongelli G, Boriani G, Pacileo G, Mastromarino V, Bacchi Reggiani ML, Lorenzini M, Lai F, Berardini A, Mingardi F, Rosmini S, Resciniti E, Borghi C, Autore C, Cecchi F, Rapezzi C. Usefulness of Electrocardiographic Patterns at Presentation to Predict Long-term Risk of Cardiac Death in Patients With Hypertrophic Cardiomyopathy. Am J Cardiol 2016;118:432-9. [Crossref] [PubMed]
- Papolos A, Narula J, Bavishi C, Chaudhry FA, Sengupta PP U.S.. Hospital Use of Echocardiography: Insights From the Nationwide Inpatient Sample. J Am Coll Cardiol 2016;67:502-11. [Crossref] [PubMed]