Prediction of early renal allograft dysfunction using multiparametric magnetic resonance imaging
Introduction
Chronic kidney disease (CKD) is a significant public health issue, affecting approximately 10% of the global population (1). It is responsible for millions of deaths each year, and hundreds of thousands of CKD patients require dialysis to sustain life (2). Kidney transplantation is the main treatment of choice for end-stage CKD patients. However, acute rejection threatens the transplanted kidney in about 8.4% of patients at 3 months post-transplantation (3). Renal allograft injuries are caused by various insults that lead to renal fibrosis, which is pathologically characterized by the extensive deposition of the extracellular matrix, and an imbalance between tubular injury-related repair mechanisms and chronic injury stimuli (4). Evaluating the burden of fibrosis can help identify high-risk patients who may develop significant renal allograft dysfunction, guide appropriate anti-fibrosis interventions, and monitor treatment outcomes (5).
Currently, renal allograft biopsy is the gold standard for detecting renal fibrosis in kidney recipients. However, as an invasive procedure, it carries risks such as bleeding, infections, perirenal hematomas, and even death, making it impractical for frequent clinical use (6). Additionally, biopsies are subject to sampling errors and may not accurately represent the overall state of the kidney. Therefore, non-invasive imaging biomarkers have been proposed to evaluate fibrosis and inflammation in renal allografts.
Multiparametric magnetic resonance imaging (mpMRI), which includes techniques such as diffusion-weighted imaging (DWI), apparent diffusion coefficient (ADC) maps, longitudinal relaxation time (T1) mapping, and arterial spin labeling (ASL) perfusion (7), provides a comprehensive evaluation of the renal structure, microstructure, and hemodynamics. Preliminary findings suggest that ASL can quantify renal blood flow (RBF), which is associated with the plasma clearance of aminopurine acid (8). Non-invasive tissue characterization through voxel-wise mapping of the T1 can indicate alterations in tissue fibrosis (9). Renal allograft injury is usually accompanied by changes in renal blood perfusion and microstructure, which can be detected by ASL and T1 mapping (10,11). The clinical application of ASL to transplanted kidneys raises several technical considerations, such as ensuring that the tagging of the RBF is orthogonal to the transplanted renal artery, and minimizing motion artifacts to reduce misregistration for the reliable quantification of cerebral blood flow (12).
Recently, T1-rho (T1ρ) imaging has emerged as a viable approach for tissue characterization. T1ρ, also known as the spin-lattice relaxation time in the rotating frame, provides a magnetic resonance (MR) contrast mechanism that can probe slow molecular motional processes in the kilohertz range, including proteins such as collagen and amyloid. Unlike conventional T1 and T2 mapping, T1ρ imaging can detect interstitial fibrosis directly (rather than indirectly) through its effects on water. It has been used in ischemic heart disease to differentiate between normal and infarcted myocardium, and has shown the ability to detect ventricular fibrosis (13). T1ρ imaging may be useful in identifying the early stages of renal fibrosis, and may serve as a more sensitive and specific biomarker than traditional techniques (14). It has been used in animal models, and is considered a promising imaging biomarker for renal allograft fibrosis (15).
This study aimed to apply mpMRI to kidney transplant recipients and evaluate which MR imaging (MRI) techniques, including DWI, T1ρ mapping, and ASL, could serve as non-invasive biomarkers for predicting renal allograft dysfunction at an early stage. We present this article in accordance with the STARD reporting checklist (available at https://qims.amegroups.com/article/view/10.21037/qims-24-2182/rc).
Methods
Study participants
This single-center prospective study was approved by the Ethics Committee of the China-Japan Friendship Hospital (No. 2022-KY-236), and informed consent was obtained from all participants. The study was conducted in accordance with the Declaration of Helsinki and its subsequent amendments. To be eligible for inclusion in the study, the patients had to meet the following inclusion criteria: (I) have early graft function after kidney transplantation; (II) have undergone mpMRI 1 month after transplantation; and (III) have had their creatinine levels continuously monitored for 3 months at our outpatient department. From September 2022 to July 2024, 37 adult kidney transplant recipients were identified for inclusion in the study. Of the 37 patients initially identified, six were excluded (one refused to provide informed consent, one had claustrophobia, three could not complete the examination due to physical discomfort, and one had poor respiratory coordination resulting in image artifacts) (Figure 1).
The serum creatinine levels of each patient were recorded at the baseline (1 month after transplantation) and at follow-up (3 months after transplantation) (16). The patients were divided into a stable group (SG) and an impaired group (IG) based on whether the serum creatinine level at 3 months post-transplantation increased by more than 25% compared to that at the first month post-transplantation (17).
MRI protocols
MRI was performed approximately 1 month after transplantation using a 1.5-T MR scanner (Ingenia Ambition, Philips Healthcare, Best, Netherlands), equipped with a 32-channel body coil. For the morphologic evaluation, axial T2- and T1-weighted images were acquired, with the scan range extending from the L3 vertebra to the pubic symphysis. For functional MRI, three-dimensional (3D) pulsed ASL [label duration: 1,800 ms; post-label delay (PLD): 1,600 ms], axial DWI (b values: 0 and 800 s/mm2) and T1ρ mapping [time of spin locking (TSL): 0, 13.3, 26.6, and 40 ms] were performed. For the pseudo-continuous ASL (pCASL) sequence, 3D gradient and spin-echo (SE) acquisition was performed with a two-dimensional SE echo-planar imaging sequence with background suppression. The labeling plane was 8 cm above the transplanted kidney, oriented axially and perpendicular to the abdominal aorta. Labeling was applied for 1.8 seconds followed by a PLD of 1,600 ms before image acquisition. The mpMRI images of the transplanted kidneys were all acquired in the axial planes. The image parameters for pCASL, T1ρ mapping, and DWI are listed in Table 1.
Table 1
Parameters | 3D pCASL | T1ρ | DWI |
---|---|---|---|
TR/TE (ms) | 3,746/13 | 2.5/1.24 | 1,200/78 |
FOV (mm2) | 240×240 | 360×297 | 400×328 |
Matrix size | 68×60 | 180×148 | 132×110 |
Slice thickness (mm) | 8 | 6 | 6 |
b value | – | – | 0/800 |
PLD/label duration time (ms) | 1,600/1,800 | – | – |
Spin-locking frequency (Hz) | – | 500 | – |
TSL (ms) | – | 0, 13.3, 26.6, 40 | – |
Scan time | 4 min 52 s | 12 s (one breath hold) | 1 min 34 s |
3D, three-dimensional; ASL, arterial spin labeling; DWI, diffusion-weighted imaging; FOV, field of view; PLD, post-labeling delay; T1ρ, T1-rho; TR/TE, time of repetition/time of echo; TSL, time of spin locking.
Imaging analysis
ADC maps, RBF maps, and T1ρ maps were generated automatically with Intellispace Portal (version 10, Philips Healthcare) for parameter measurement. Two radiologists, with 8 and 10 years of abdominal imaging experience, independently and randomly analyzed all the images without referring to the clinical or pathological data. To measure ADC, RBF, and T1ρ, the RBF maps, ADC maps, and T1ρ maps were first registered to T2-weighted images. Three irregular regions of interest (ROIs), ranging from 110 to 150 mm2, were then drawn on the cortex area of the T2-weighted images outlining the configuration of the cortex, and copied onto the functional maps for measurement (Figure 2). For each transplanted kidney, three ROIs were drawn at the upper pole, interpolar portion, and lower pole, and measurements were undertaken at three middle continuous slices. Areas with blood vessels, hematomas, and cysts were excluded from the ROIs. The values from the total nine ROIs were averaged for each transplanted kidney for the statistical analysis.
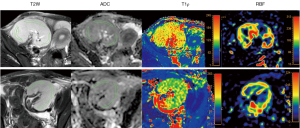
Statistical analysis
Each patient was independently assessed by two radiologists. The mean value from both readers was used as the final value for each patient. SPSS (SPSS 29.0, SPSS Inc., Chicago, IL, USA), MedCalc (version 15.0, Mariakerke, Belgium), and GraphPad Prism (version 10.4.1, GraphPad Software) were used for statistical analysis and plotting. Data distribution was assessed for normality using the normality test and distribution curve before further statistical analysis. The data are presented as the mean ± standard deviation. The Chi-squared test was used to examine the gender ratio. The t-test was used to compare the two groups. The Chi-squared test was used to assess differences among the three groups. A logistic regression analysis was used to investigate the association between the MRI parameters and serum creatinine levels at follow-up. Odds ratios (ORs) and their 95% confidence intervals (CIs) were calculated. A receiver operating characteristic (ROC) curve analysis was used to evaluate the sensitivity, specificity, and cut-off values of the MRI parameters for the prognostic indices. Interobserver reproducibility was assessed using the intraclass correlation coefficient (ICC), with values less than 0.5, values between 0.5 and 0.75, values between 0.75 and 0.9, and values greater than 0.90 indicating poor, moderate, good, and excellent reliability, respectively (18). A P value <0.05 was considered statistically significant.
Results
Patient characteristics
MpMRI was performed on 31 patients (25 males and 6 females, mean age: 44.4±10.9 years), including 20 with stable graft function (the SG) and 11 with impaired graft function (the IG). The mean serum creatinine levels 1 and 3 months after transplantation were 196.1±77.8 and 183.1±116.4 µmol/L, respectively. The mean serum estimated glomerular filtration rate (eGFR) 1 and 3 months after transplantation were 44.4±11.1 and 49.2±11.2 µmol/L, respectively. At 1 month post-transplantation, there were no significant differences between the two groups in terms of age, gender, serum creatinine level, or eGFR (P>0.05). At 3 months post-transplantation, the serum creatinine levels were significantly higher and the eGFR was lower in the IG than the SG (P<0.001). The demographic and clinical information of the patients are presented in Table 2.
Table 2
Variables | Total | SG (n=20) | IG (n=11) | P |
---|---|---|---|---|
Age (years) | 44.4±10.9 | 45.2±11.8 | 43.0±9.3 | 0.39 |
Gender (male:female) | 25:6 | 15:5 | 10:1 | 0.28 |
Creatinine (μmol/L) | ||||
At 1 month after transplantation | 196.1±77.8 | 183.2±83.7 | 230.1±47.2 | 0.07 |
At 3 months after transplantation | 183.1±116.4 | 127.1±23.9 | 304.5±142.2 | <0.001 |
eGFR (mL/min/1.73 m2) | ||||
At 1 month after transplantation | 44.4±11.1 | 46.8±9.1 | 43.3±11.8 | 0.05 |
At 3 months after transplantation | 49.2±11.2 | 51.7±11.4 | 43.8±8.8 | <0.001 |
Values are expressed as the number or mean ± standard deviation. eGFR, estimated glomerular filtration rate; IG, impaired group; SG, stable group.
The stability of the T1ρ measurements
The stability of T1ρ measurements was assessed across different renal regions, including the upper, interpolar, and lower poles. Chi-squared tests were used to compare the average T1ρ values of the upper, interpolar, and lower poles, and the results revealed no statistically significant differences (108±13.2 vs. 113±21.1 vs. 105±15.4 ms, F=1.835, P=0.17) among them.
Comparative analysis of the clinical characteristics and MRI parameters
The mean ADC value of all 31 patients was 2.0±0.20−3 mm2/s, the mean RBF was 242.3±64.5 mL/100 g/min, and the mean T1ρ mapping was 109.8±18.9 ms. All parameters had good inter-observer reproducibility (ICCs of 0.86, 0.93, and 0.91 for ADC, RBF, and T1ρ, respectively). As Figure 3 shows, at 1 month post-transplantation, the ADC values did not differ significantly between the two groups (P>0.05). However, significant differences in RBF (261.1±56.4 vs. 208.2±66.6 mL/100 g/min, P=0.04) and T1ρ (116.1±20.0 vs. 98.3±9.7 ms, P=0.01) were observed between the SG and IG. The MRI parameters of the two groups are summarized in Table 3.
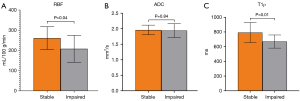
Table 3
Variables | Total | SG (n=20) | IG (n=11) | P |
---|---|---|---|---|
RBF (ASL) (mL/100 g/min) | 242.3±64.5 | 261.1±56.4 | 208.2±66.6 | 0.04 |
T1ρ (ms) | 109.8±18.9 | 116.1±20.0 | 98.3±9.7 | 0.01 |
ADC value (mm2/s) | 2.0±0.20−3 | 2.0±0.2−3 | 1.9±0.2−3 | 0.84 |
Values are expressed as the mean ± standard deviation. ADC, apparent diffusion coefficient; ASL, arterial spin; IG, impaired group; MRI, magnetic resonance imaging; RBF, renal blood flow; SG, stable group.
The prognostic evaluation value of the MRI parameters
In the binary logistic regression analysis, among all the measured imaging parameters (i.e., RBF, ADC, and T1ρ), RBF and T1ρ were significantly correlated with renal function at 3 months post-transplantation (P<0.05) (Table 4). The ROC curve analysis revealed that RBF values greater than 192.4 mL/100 g and T1ρ values greater than 103.2 ms significantly decreased the risk of renal dysfunction, yielding the highest Youden index values. For RBF with a cut-off value of 192.4 mL/100 g, the area under the curve (AUC), sensitivity, and specificity were 0.739, 100%, and 55%, respectively. For T1ρ with a cut-off value of 103.2 ms, the AUC, sensitivity, and specificity were 0.845, 85%, and 73%, respectively (Table 4). The AUC for the combination of RBF and T1ρ was 0.927 (Figure 4).
Table 4
Variables | B | SE | Wald | OR | P | 95% CI |
---|---|---|---|---|---|---|
T1ρ | −0.188 | 0.077 | 5.913 | 0.845 | 0.02 | 0.709–0.982 |
RBF (ASL) | −0.166 | 0.083 | 3.976 | 0.739 | 0.05 | 0.563–0.915 |
ADC value | −0.047 | 0.214 | 0.048 | 0.954 | 0.83 | 0.628–1.450 |
ADC, apparent diffusion coefficient; ASL, arterial spin labeling; CI, confidence interval; MRI, magnetic resonance imaging; OR, odds ratio; RBF, renal blood flow; SE, standard error; T1ρ, T1-rho.
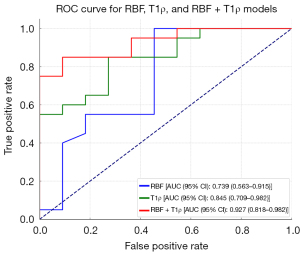
Discussion
In this study, we investigated the value of DWI, ASL, and T1ρ mapping in predicting short-term allograft dysfunction. We found that RBF and T1ρ at 1 month post-transplantation were associated with impaired allograft function 2 months later. A single time value related to renal allograft fibrosis is clinically significant; however, monitoring allograft fibrosis over time could be even more relevant for clinical decisions and predicting disease progression. These results highlight the potential role of ASL and T1ρ mapping in assessing allograft dysfunction.
High renal perfusion is crucial for maintaining stable glomerular filtration (19), particularly in renal allografts. Acute rejection, which typically occurs early after kidney transplantation, is often linked to allograft ischemia or hypoperfusion. Changes in renal perfusion are among the earliest markers of renal damage (20). Therefore, assessing renal perfusion can help identify patients with early allograft injuries. ASL-MRI is a reliable tool for detecting RBF with low variability. Previous studies have shown that quantifying changes in perfusion and diffusion may predict long-term renal allograft outcomes (21-23). Compared to reduced field of view intravoxel incoherent motion (IVIM) DWI, ASL may be more effective at non-invasively distinguishing different degrees of renal allograft fibrosis (4). Consistent with previous research, our results also showed that the baseline RBF is associated with short-term allograft dysfunction and has good diagnostic performance. A recent study also found that RBF has high diagnostic and prognostic value for detecting graft dysfunction early after transplantation (16). In our study, the reduced RBF in the allograft kidneys at the baseline might have indicated acute rejection, as we excluded recipients with delayed graft function. However, some studies suggest that while ASL perfusion can indicate renal function, it may not be able to be used to differentiate between fibrotic burden and other damage factors, such as inflammation (4,24,25). These confounding effects may reduce the diagnostic efficiency of RBF for renal allograft fibrosis.
Our results also indicated that T1ρ mapping was effective in predicting short-term renal allograft dysfunction. The use of T1ρ mapping in evaluating myocardial fibrosis is increasingly being studied. Research has revealed a significant correlation between fibrosis fractions measured histologically in ex vivo human hearts and T1ρ values (26). However, studies on T1ρ mapping in renal transplantation are relatively limited. A previous cross-sectional study assessed the utility of T1ρ mapping in evaluating fibrosis in renal allografts, and found that it had an AUC of 0.77 for differentiating between functional and fibrotic allografts. The study showed that cortical T1ρ was significantly correlated with Masson’s trichrome-stained fractions and the eGFR (27). The present study did not include pathological evidence; however, it showed the utility of T1ρ mapping in predicting allograft dysfunction through follow-up. Further, the predictive power of T1ρ mapping appeared to be superior to that of ASL. Notably, ASL reflects allograft perfusion, while T1ρ mapping captures macromolecule-water interactions and collagen content. Thus, complicated structural abnormalities may begin early during acute rejection, affecting the change in T1ρ. However, as biopsies were not performed at this early stage, the extent of renal fibrosis formation could not be determined.
Contrary to the findings of previous studies (28), in the present study, ADC was not found to be significantly associated with renal allograft function. Friedli et al. found that in animal models, both T1 and ADC were correlated with fibrosis and inflammation based on histological findings (29). However, for kidney allograft recipients, the absolute cortical ADC or T1 values were poorly correlated with interstitial fibrosis assessed by biopsy. Conversely, the corticomedullary ADC difference was independently associated with kidney fibrosis (30), and changes in this difference paralleled increases in fibrosis identified by biopsies (31). Image data for the renal medulla were available in our study; however, we chose to focus on the cortical measurements. The glomeruli and proximal tubules are primarily located in the renal cortex, which receives 85–90% of the kidney’s total blood flow. This high perfusion renders the cortex particularly susceptible to ischemic injury and toxic insults. Dysfunction of the glomeruli leads to a decline in the filtration rate, as seen in acute kidney injury, while damage to the proximal tubules impairs reabsorption, resulting in abnormal urine composition (32). Thus, renal dysfunction typically begins in these two critical structures. Given this, we chose to focus exclusively on measuring the ADC of the cortex of the transplanted kidney.
The combination of RBF and T1ρ was better able to predict allograft dysfunction than either parameter alone. Our findings align with a recent study that showed that mpMRI significantly enhances diagnostic and prognostic values for detecting graft dysfunction compared to the eGFR (31). Despite the high diagnostic and prognostic potential of mpMRI, technical factors and clinical convenience must be considered. A fixed PLD of 1,600 ms was selected in our study based on a previous study (33) that identified 1,600 ms as optimal for assessing renal perfusion in transplanted kidneys. Coronal oblique slices (along the major axis of the kidneys) are recommended for renal ASL (34) but are not suitable for transplanted kidneys. Axial planes may not strictly align with the major axis of the transplanted kidneys. Therefore, there may be technical errors regarding ASL data acquisition. Moreover, ASL sequences, high-resolution DWI, and IVIM imaging require several minutes to collect raw data, and motion artifacts can lead to quantification inaccuracies during postprocessing. Conversely, T1ρ mapping, which generates tissue contrasts through endogenous T1ρ relaxation during a breath hold, is relatively rapid and convenient for clinical use. The stability of T1ρ measurements was also shown across different renal regions, including the upper, interpolar, and lower poles.
This study had several limitations. First, the relatively small sample size might have introduced sampling errors into the statistical analysis. Larger studies need to be conducted to confirm the role of T1ρ as a biomarker for renal fibrosis. Second, our study relied on serum creatinine levels as an indicator of graft function, but these levels might not fully represent the underlying tissue state. While T1ρ mapping and ASL provide non-invasive assessments of fibrosis and perfusion, their correlation with histopathological changes requires further validation. Future studies incorporating biopsy data need to be conducted to establish a direct relationship between T1ρ mapping and renal tissue characteristics. Finally, renal medullary values were not measured in this study. It has been reported that medullary measurements have relatively lower reproducibility than cortical measurements (21). Nonetheless, we believe that cortical parameters are adequate for assessing renal allograft function.
Conclusions
Our study used mpMRI to assess renal allograft dysfunction. RBF and T1ρ measured 1 month after transplantation demonstrated good performance in predicting early allograft dysfunction, potentially due to acute rejection. T1ρ mapping in particular shows promise for evaluating early renal structural changes, as it outperforms ASL and is more convenient for clinical application.
Acknowledgments
None.
Footnote
Reporting Checklist: The authors have completed the STARD reporting checklist. Available at https://qims.amegroups.com/article/view/10.21037/qims-24-2182/rc
Funding: None.
Conflicts of Interest: All authors have completed the ICMJE uniform disclosure form (available at https://qims.amegroups.com/article/view/10.21037/qims-24-2182/coif). X.Y. is an employee of Philips Healthcare. The other authors have no conflicts of interest to declare.
Ethical Statement: The authors are accountable for all aspects of the work in ensuring that questions related to the accuracy or integrity of any part of the work are appropriately investigated and resolved. The study was approved by the Ethics Committee of the China-Japan Friendship Hospital (No. 2022-KY-236), and was conducted in accordance with the Declaration of Helsinki and its subsequent amendments. Informed consent was obtained from all patients.
Open Access Statement: This is an Open Access article distributed in accordance with the Creative Commons Attribution-NonCommercial-NoDerivs 4.0 International License (CC BY-NC-ND 4.0), which permits the non-commercial replication and distribution of the article with the strict proviso that no changes or edits are made and the original work is properly cited (including links to both the formal publication through the relevant DOI and the license). See: https://creativecommons.org/licenses/by-nc-nd/4.0/.
References
- Zhang J, Yu Y, Liu X, Tang X, Xu F, Zhang M, Xie G, Zhang L, Li X, Liu ZH. Evaluation of Renal Fibrosis by Mapping Histology and Magnetic Resonance Imaging. Kidney Dis (Basel) 2021;7:131-42. [Crossref] [PubMed]
- Naber T, Purohit S. Chronic Kidney Disease: Role of Diet for a Reduction in the Severity of the Disease. Nutrients 2021;13:3277. [Crossref] [PubMed]
- Švachová V, Krupičková L, Novotný M, Fialová M, Mezerová K, Čečrdlová E, Lánská V, Slavčev A, Viklický O, Viklický O, Stříž I. Changes in phenotypic patterns of blood monocytes after kidney transplantation and during acute rejection. Physiol Res 2021;70:709-21. [Crossref] [PubMed]
- Yu YM, Wang W, Wen J, Zhang Y, Lu GM, Zhang LJ. Detection of renal allograft fibrosis with MRI: arterial spin labeling outperforms reduced field-of-view IVIM. Eur Radiol 2021;31:6696-707. [Crossref] [PubMed]
- Lovisa S, LeBleu VS, Tampe B, Sugimoto H, Vadnagara K, Carstens JL, Wu CC, Hagos Y, Burckhardt BC, Pentcheva-Hoang T, Nischal H, Allison JP, Zeisberg M, Kalluri R. Epithelial-to-mesenchymal transition induces cell cycle arrest and parenchymal damage in renal fibrosis. Nat Med 2015;21:998-1009. [Crossref] [PubMed]
- Moledina DG, Luciano RL, Kukova L, Chan L, Saha A, Nadkarni G, Alfano S, Wilson FP, Perazella MA, Parikh CR. Kidney Biopsy-Related Complications in Hospitalized Patients with Acute Kidney Disease. Clin J Am Soc Nephrol 2018;13:1633-40. [Crossref] [PubMed]
- Buchanan CE, Mahmoud H, Cox EF, McCulloch T, Prestwich BL, Taal MW, Selby NM, Francis ST. Quantitative assessment of renal structural and functional changes in chronic kidney disease using multi-parametric magnetic resonance imaging. Nephrol Dial Transplant 2020;35:955-64. [Crossref] [PubMed]
- Cai YZ, Li ZC, Zuo PL, Pfeuffer J, Li YM, Liu F, Liu RB. Diagnostic value of renal perfusion in patients with chronic kidney disease using 3D arterial spin labeling. J Magn Reson Imaging 2017;46:589-94. [Crossref] [PubMed]
- Franke M, Baeßler B, Vechtel J, Dafinger C, Höhne M, Borgal L, Göbel H, Koerber F, Maintz D, Benzing T, Schermer B, Persigehl T. Magnetic resonance T2 mapping and diffusion-weighted imaging for early detection of cystogenesis and response to therapy in a mouse model of polycystic kidney disease. Kidney Int 2017;92:1544-54. [Crossref] [PubMed]
- Jiang B, Li J, Wan J, Tian Y, Wu P, Xu R, Yu Y, Wang X, Hu L, Zhu M. Arterial spin labeling combined with T1 mapping for assessment of kidney function and histopathology in patients with long-term renal transplant survival after kidney transplantation. Quant Imaging Med Surg 2024;14:2415-25. [Crossref] [PubMed]
- Wei CG, Zeng Y, Zhang R, Zhu Y, Tu J, Pan P, Ma Q, Wei LY, Zhao WL, Shen JK. Native T(1) mapping for non-invasive quantitative evaluation of renal function and renal fibrosis in patients with chronic kidney disease. Quant Imaging Med Surg 2023;13:5058-71. [Crossref] [PubMed]
- Odudu A, Nery F, Harteveld AA, Evans RG, Pendse D, Buchanan CE, Francis ST, Fernández-Seara MA. Arterial spin labelling MRI to measure renal perfusion: a systematic review and statement paper. Nephrol Dial Transplant 2018;33:ii15-21. [Crossref] [PubMed]
- Haris M, Singh A, Cai K, Kogan F, McGarvey J, Debrosse C, Zsido GA, Witschey WR, Koomalsingh K, Pilla JJ, Chirinos JA, Ferrari VA, Gorman JH, Hariharan H, Gorman RC, Reddy R. A technique for in vivo mapping of myocardial creatine kinase metabolism. Nat Med 2014;20:209-14. [Crossref] [PubMed]
- Suraj D. Serai KD. T1ρ Mapping and Its Applications for Assessment of Renal Fibrosis. Switzerland: Springer Nature Switzerland AG; 2023.
- Min S, Chang D, Wang YC, Xu TT, Ge H, Zhang J, Wang B, Ju S. Novel small-molecule compound VCP979 attenuates renal fibrosis in male rats with unilateral ureteral obstruction. Exp Biol Med (Maywood) 2023;248:327-38. [Crossref] [PubMed]
- Echeverria-Chasco R, Martin-Moreno PL, Aramendía-Vidaurreta V, Garcia-Ruiz L, Mora-Gutiérrez JM, Vidorreta M, Villanueva A, Cano D, Bastarrika G, Garcia-Fernandez N, Fernández-Seara MA. Diagnostic and Prognostic Potential of Multiparametric Renal MRI in Kidney Transplant Patients. J Magn Reson Imaging 2024;60:1650-63. [Crossref] [PubMed]
- Wiebe C, Gibson IW, Blydt-Hansen TD, Pochinco D, Birk PE, Ho J, Karpinski M, Goldberg A, Storsley L, Rush DN, Nickerson PW. Rates and determinants of progression to graft failure in kidney allograft recipients with de novo donor-specific antibody. Am J Transplant 2015;15:2921-30. [Crossref] [PubMed]
- Koo TK, Li MY. A Guideline of Selecting and Reporting Intraclass Correlation Coefficients for Reliability Research. J Chiropr Med 2016;15:155-63. [Crossref] [PubMed]
- Burke M, Pabbidi MR, Farley J, Roman RJ. Molecular mechanisms of renal blood flow autoregulation. Curr Vasc Pharmacol 2014;12:845-58. [Crossref] [PubMed]
- James MT, Hemmelgarn BR, Tonelli M. Early recognition and prevention of chronic kidney disease. Lancet 2010;375:1296-309. [Crossref] [PubMed]
- Echeverria-Chasco R, Martin-Moreno PL, Garcia-Fernandez N, Vidorreta M, Aramendia-Vidaurreta V, Cano D, Villanueva A, Bastarrika G, Fernández-Seara MA. Multiparametric renal magnetic resonance imaging: A reproducibility study in renal allografts with stable function. NMR Biomed 2023;36:e4832. [Crossref] [PubMed]
- Ix JH, Katz R, Bansal N, Foster M, Weiner DE, Tracy R, Jotwani V, Hughes-Austin J, McKay D, Gabbai F, Hsu CY, Bostom A, Levey AS, Shlipak MG. Urine Fibrosis Markers and Risk of Allograft Failure in Kidney Transplant Recipients: A Case-Cohort Ancillary Study of the FAVORIT Trial. Am J Kidney Dis 2017;69:410-9. [Crossref] [PubMed]
- Oberbauer R. Progression of Interstitial Fibrosis in Kidney Transplantation. Clin J Am Soc Nephrol 2016;11:2110-2. [Crossref] [PubMed]
- Mendichovszky I, Pullens P, Dekkers I, Nery F, Bane O, Pohlmann A, et al. Technical recommendations for clinical translation of renal MRI: a consensus project of the Cooperation in Science and Technology Action PARENCHIMA. MAGMA 2020;33:131-40. [Crossref] [PubMed]
- Selby NM, Blankestijn PJ, Boor P, Combe C, Eckardt KU, Eikefjord E, et al. Magnetic resonance imaging biomarkers for chronic kidney disease: a position paper from the European Cooperation in Science and Technology Action PARENCHIMA. Nephrol Dial Transplant 2018;33:ii4-ii14. [Crossref] [PubMed]
- van Oorschot JW, Güçlü F, de Jong S, Chamuleau SA, Luijten PR, Leiner T, Zwanenburg JJ. Endogenous assessment of diffuse myocardial fibrosis in patients with T1ρ -mapping. J Magn Reson Imaging 2017;45:132-8. [Crossref] [PubMed]
- Hectors SJ, Bane O, Kennedy P, El Salem F, Menon M, Segall M, Khaim R, Delaney V, Lewis S, Taouli B. T1ρ mapping for assessment of renal allograft fibrosis. J Magn Reson Imaging 2019;50:1085-91. [Crossref] [PubMed]
- Beck-Tölly A, Eder M, Beitzke D, Eskandary F, Agibetov A, Lampichler K, Hamböck M, Regele H, Kläger J, Nackenhorst M, Böhmig GA. Magnetic Resonance Imaging for Evaluation of Interstitial Fibrosis in Kidney Allografts. Transplant Direct 2020;6:e577. [Crossref] [PubMed]
- Friedli I, Crowe LA, Berchtold L, Moll S, Hadaya K, de Perrot T, Vesin C, Martin PY, de Seigneux S, Vallée JP. New Magnetic Resonance Imaging Index for Renal Fibrosis Assessment: A Comparison between Diffusion-Weighted Imaging and T1 Mapping with Histological Validation. Sci Rep 2016;6:30088. [Crossref] [PubMed]
- Berchtold L, Friedli I, Crowe LA, Martinez C, Moll S, Hadaya K, de Perrot T, Combescure C, Martin PY, Vallée JP, de Seigneux S. Validation of the corticomedullary difference in magnetic resonance imaging-derived apparent diffusion coefficient for kidney fibrosis detection: a cross-sectional study. Nephrol Dial Transplant 2020;35:937-45. [Crossref] [PubMed]
- Berchtold L, Crowe LA, Friedli I, Legouis D, Moll S, de Perrot T, Martin PY, Vallée JP, de Seigneux S. Diffusion magnetic resonance imaging detects an increase in interstitial fibrosis earlier than the decline of renal function. Nephrol Dial Transplant 2020;35:1274-6. [Crossref] [PubMed]
- O'Connor PM. Renal oxygen delivery: matching delivery to metabolic demand. Clin Exp Pharmacol Physiol 2006;33:961-7. [Crossref] [PubMed]
- Ahn HS, Yu HC, Kwak HS, Park SH. Assessment of Renal Perfusion in Transplanted Kidney Patients Using Pseudo-Continuous Arterial Spin Labeling with Multiple Post-Labeling Delays. Eur J Radiol 2020;130:109200. [Crossref] [PubMed]
- Nery F, Buchanan CE, Harteveld AA, Odudu A, Bane O, Cox EF, et al. Consensus-based technical recommendations for clinical translation of renal ASL MRI. MAGMA 2020;33:141-61. [Crossref] [PubMed]