The rationale for proposing a magnetic resonance slow diffusion metric and its proof-of-concept testing showing spleen parenchyma and hepatocellular carcinoma have faster diffusion than liver parenchyma
Introduction
Diffusion weighted imaging (DWI) plays a pivotal role in magnetic resonance imaging (MRI) evaluation of a variety of pathologies. However, clinical application of the DWI derived quantitative metrics of apparent diffusion coefficient (ADC) and intravoxel incoherent motion (IVIM) parameters [perfusion fraction (PF), Dslow, Dfast] have not been very successful. ADC is indeed widely used, while it is generally regarded by physicians and radiologists that its role is only ‘supportive’ rather than ‘confirmative’. IVIM technique has largely remained in the research phase.
In the literature reported in vivo ADC results, there exists a ‘T2-ADC curve’, with ADC measure strongly affected by T2 relaxation time (T2) (1-6). T2 can be divided into short T2 band [<60 millisecond (ms)], intermediate T2 band (60–80 ms), and long T2 band (>80 ms, all 3T values). For the short T2 time band, there is a negative correlation between T2 and ADC. For the long T2 time band, there is a positive correlation between T2 and ADC. A tissue likely measures a low ADC if its T2 is close to 70 ms (such as the cases of lymphoma, abscess liquid, and the acute phase of brain ischemic stroke). The spleen (with T2 of around 60 ms) and parotid gland Warthin’ tumors (with T2 of around 80 ms) have a low ADC despite having rich blood perfusion. On the other hand, a tissue is likely to measure a high ADC if its T2 is far away from 70 ms, such as the cases of cartilage (with very short T2 and high ADC), myxoma and chondrosarcoma (both with very long T2 and very high ADC) (4). It appears to be unreasonable that the spleen has an ADC of only around 0.8×10−3 mm2/s while chondrosarcoma has an ADC of around 2.3×10−3 mm2/s (4). This ‘T2-ADC curve’ is graphically shown in Figure 1 (2,4,5,7). Uterine myometrium has T2 and ADC similar to that of skeletal muscles (5). A synthetic analysis based on the articles of DeMulder et al. (8), Bura et al. (9), and Barral et al. (10) shows that, relative to the myometrium T2 and ADC, myometrium tumors with shorter T2 are associated with higher ADC, myometrium tumors with longer T2 are associated with lower ADC, and myometrium tumors with very longer T2 (cystic degenerated leiomyoma and myxoid degenerated leiomyoma) are associated with high ADC, thus being very consistent with the ‘T2-ADC curve’ [see the supplementary figure in (5)]. This high dependency of ADC on T2 suggests that, though ADC can be contributed to a certain degree by tissue diffusion, it is overall not a good biomarker to reliably reflect true tissue diffusion (6).
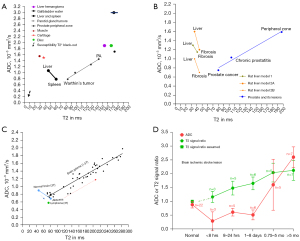
We have recently proposed the metric of diffusion derived ‘vessel density’ (DDVD) (11-13), which is derived from the equation:
where ROIarea0 and ROIarea1 refer to the number of pixels in the selected region of interest (ROI) on b=0 and b=1 s/mm2 diffusion weighted (DW) image, respectively. S(b0) refers to the measured sum signal intensity within the ROI when b=0, and S(b1) refers to the measured sum signal intensity within the ROI when b=1 s/mm2, thus S(b)/ROIarea equates to the mean signal intensity within the ROI. S(b1) and ROIarea1 can also be approximated by other low b-values (such as b=10 s/mm2) DW image. We have demonstrated that, for the liver and spleen DDVD measurement, when the time of echo (TE) is short or modest and time of repetition (TR) is long (such as TR >1,600 ms, TE =60 ms) and the 2ndb-value is 1 or 2 mm2/s, DDVDliver is approximately similar to DDVDspleen which agrees with the known physiology that liver and spleen have similar amount of blood perfusion (14). According to the analysis in (14), a liver DDVD value of 11 au/pixel at 1.5T or 35 au/pixel at 3.0T will be approximately equivalent to computed tomography (CT) perfusion of blood volume of 18 mL/100 mL with a blood flood speed of around 1.2 mL/min/mL. This also correlates with the liver IVIM-PF measure of about 18% reported by us (15). In physiological studies, the hepatic blood volume including that of the large vessels is about 25 mL/100 g and blood flow is about 1.04 mL/min/mL (16). When a TE of 59 ms (TR =1,600 ms, free breathing acquisition) and b=0, 2 mm2/s were used for the DDVD calculation of 26 cases of hepatocellular carcinoma (HCC), we measured a mean DDVDHCC/DDVDliver ratio of 1.42. This value agrees well with the perfusion CT blood volume literature results median ratio of 1.38, while the perfusion CT blood flow literature results mean ratio is 1.92 (Figure 2) (17). Our study of parotid tumor DDVD also showed parotid tumor DDVD ratio measures were consistent with CT measured blood volume ratios (18). One of the initial goals of using DDVD was to minimize the T2 effect (which is defined as MRI signal differences contributed by T2 relaxation time difference). This is approximately achieved when TR is long and TE is short or modest (17). DDVD is less sensitive to TE selection and the ‘T2 effect’ compared to that of ADC and IVIM (17).
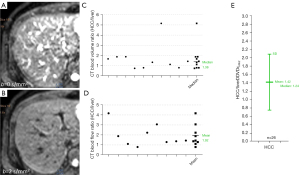
As in vivo diffusion metrics can be only measured with MRI thus external validation is not possible for diffusion metrics, it will be convenient and practical for us to consider liver/spleen parenchyma as the reference tissues. The spleen has been known to have a much lower ADC than the liver. However, the liver and spleen have similar amounts of blood perfusion per tissue volume per minute (14), and the spleen is waterier than the liver (the spleen has longer T1 and longer T2 than the liver, and the spleen has lower CT density than the liver). The magnetization transfer signal ratio (MTR) measure shows a higher proportion of water molecules in the liver are bound to other macro-molecules than the water molecules in the spleen (19), which supports that water molecules in the spleen have a greater extent of free diffusion. Free water molecules also allow longer T1 and T2 relaxation times than bounded water molecules. The spleen is also an organ with the function of storing blood (20). The spleen can respond to sympathetic stimulation by contracting its fibroelastic capsule and trabeculae to increase systemic blood supply. Recently, Yeung et al. (21) reported that spleen tissue had a higher contrast-enhanced CT extracellular volume fraction (ECV) than the liver, in healthy tissues and in tissues with systemic amyloid light-chain amyloidosis. We consider that it is unreasonable that spleen ADC is much lower than liver ADC. As HCCs are mostly associated with increased blood supply and increased proportion of arterial blood supply and higher water content (i.e., edema, as shown with higher signal on T2-weighted image and with lower density on X-ray CT), we consider that it is also unreasonable that HCC has a lower ADC than adjacent liver parenchyma. We argue that the lower ADC of the spleen and HCC relative to liver parenchyma is due to their longer T2 relative to liver parenchyma (6).
Another two entities with confusing ADC measures are liver hemangioma and simple liver cyst. Cysts are known to have a longer T2 and a higher ADC than hemangioma (Figure 3) (22-28). We also hypothesize that the higher ADC of liver simple cysts are due to their longer T2 (6). Due to the ‘flushing’ of blood flow inside the hemangioma, we hypothesize that actual diffusion of hemangioma liquid is faster than the more ‘static’ liquid of the cysts. Indeed, in a gadolinium-enhanced dynamic MRI study, Nam et al. (29) reported that ADC values were higher in the rapidly contrast enhancing hemangiomas than in the intermediately or the slowly contrast enhancing hemangiomas.
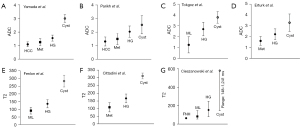
During the course of our research activities and following the same concept as DDVD, we found that we can use a straightforward method to calculate a metric reflecting tissue slow diffusion, and we term this metric as ‘slow diffusion coefficient (SDC)’:
where b1 and b2 refers to a high b-value (e.g., 400 mm2/s for the case of liver DWI) and a higher b-value respectively (e.g., 600 mm2/s for the case of liver DWI), where S(b1) and S(b2) denote the image signal intensity acquired at the high b-value and the higher b-value respectively (Figure 4). In this study, we explain the rationale of why we propose such a diffusion metric and attempt to conduct proof-of-concept testing with the liver, spleen, HCC, liver hemangioma, and simple liver cysts for such a metric. As ADC measure is heavily affected by T2 (6), we test whether SDC can minimize the T2 effect.
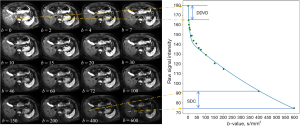
Methods
This is a retrospective analysis of previously prospectively acquired liver IVIM data. The study was conducted in accordance with the Declaration of Helsinki (as revised in 2013). All IVIM data were acquired with institutional ethical approval and with informed consent obtained from individual participants. 1.5T MRI was performed with a Philips scanner (Achieva, Philips Healthcare, Best, Netherlands). The IVIM diffusion imaging was based on a single-shot spin-echo type echo-planar sequence, with 15 b-values of 0, 1, 2, 15, 20, 30, 45, 50, 60, 80, 100, 200, 300, 600, and 800 s/mm2. Number-of-excitation (NEX) was 2 for all image acquisition. The TR was 1,600 ms and the TE was 63 ms. SPIR technique (Spectral Pre-saturation with Inversion-Recovery) was used for fat suppression. Respiratory-gating was applied in all scan participants. Other parameters included slice thickness =7 mm and inter-slice gap 1 mm, matrix =124×97, field of view (FOV) =375 mm × 302 mm, number of slices =6. 3.0T MRI was performed with a Philips scanner (Ingenia, Philips Healthcare, Best, Netherlands). The IVIM diffusion imaging was based on a single-shot spin-echo type echo-planar sequence, with 16 b-values of 0 (NEX =5), 2 (NEX =5), 4 (NEX =1), 7 (NEX =1), 10 (NEX =1), 15 (NEX =1), 20 (NEX =1), 30 (NEX =1), 46 (NEX =1), 60 (NEX =3), 72(NEX =1), 100 (NEX =1), 150 (NEX =1), 200 (NEX =1), 400 (NEX =2), and 600 (NEX =2) s/mm2. The default SPIR technique was used for fat suppression. The TR was 1,600 ms and the TE was 59 ms. Data was acquired with free breathing. Other parameters included slice thickness =7 mm and inter-slice gap =1.5 mm, matrix =123×124, FOV =372 mm × 341 mm, number of slices =20.
All image processing was implemented in a custom program developed on MATLAB (Mathworks, Natick, MA, USA). For the analysis of liver and spleen parenchyma, on each DW image for the liver/spleen, ROIs were drawn to cover a large portion of the right liver parenchyma and spleen parenchyma while avoiding large vessels and artifacts. Based on Eq. [1], for 1.5T data, DDVDb0b1, DDVDb0b2, DDVDb0b15, DDVDb0b20, and DDVDb0b30, DDVDb0b45, DDVDb0b50, DDVDb0b60, DDVDb0b80, DDVDb0b100, DDVDb0b200, DDVDb0b300, DDVDb0b600, and DDVDb0b800, were calculated from b=0 and b=1, b=0 and b=2, b=0 and b=15, b=0 and b=20, b=0 and b=30, b=0 and b=45, b=0 and b=50, b=0 and b=60, b=0 and b=80, b=0 and b=100, b=0 and b=200, b=0 and b=300, b=0 and b=600, and b=0 and b=800 s/mm2 images, respectively. For 3.0T data, DDVDb0b2, DDVDb0b4, DDVDb0b7, DDVDb0b10, DDVDb0b15, DDVDb0b20, and DDVDb0b30, DDVDb0b46, DDVDb0b60, DDVDb0b72, DDVDb0b100, DDVDb0b150, DDVDb0b200, DDVDb0b400, and DDVDb0b600, were calculated from b=0 and b=2, b=0 and b=4, b=0 and b=7, b=0 and b=10, b=0 and b=15, b=0 and b=20, b=0 and b=30, b=0 and b=46, b=0 and b=60, b=0 and b=72, b=0 and b=100, b=0 and b=150, b=0 and b=200, b=0 and b=400, and b=0 and b=600 s/mm2 images, respectively.
For 1.5T data, SDCb600b800 was calculated according to Eq. [2], where b2 and b1 refer to 800 and 600 s/mm2, respectively, and S(b2) and S(b1) denote the signal intensity on b=800 and b=600 s/mm2 images, respectively. For 3.0T data, SDCb400b600 was calculated according to Eq. [2], where b2 and b1 refer to 600 and 400 s/mm2, respectively, and S(b2), and S(b1) denote the signal intensity on b=600 and on b=400 s/mm2 images, respectively. With 1.5T data, SDCb600b800 was calculated for 10 healthy volunteer cases’ liver and spleen parenchyma, as well as two simple liver cysts and their corresponding liver parenchyma. With 3.0T data, SDCb400b600 was calculated for 14 healthy volunteer cases’ liver and spleen parenchyma, as well as 13 HCC masses, 9 simple liver cysts, 13 hemangiomas and their corresponding liver parenchyma. These HCC, hemangioma, and cysts happened to exist in our datasets, and a random selection for these lesions was only based on that they were of reasonable sizes for measurement. Measurement of HCC excluded apparent necrotic areas whenever possible.
Based on the ROI drawn for DDVD analysis, conventional ADC was calculated according to:
where b2 and b1 refers to the high b-value and low b-value respectively, where S(b2) and S(b1) denote the image signal intensity acquired at the high b-value and low b-value respectively.
Based on Eq [3], for 1.5T data, ADCb1b800, ADCb2b800, ADCb15b800, ADCb20b800, and ADCb30b800, ADCb45b800, ADCb50b800, ADCb60b800, ADCb80b800, ADb100b800, ADCb200b800, ADCb300b800, and ADCb600b800, were calculated from b=1 and b=800, b=2 and b=800, b=15 and b=800, b=20 and b=800, b=30 and b=800, b=45 and b=800, b=50 and b=800, b=60 and b=800, b=80 and b=800, b=100 and b=800, b=200 and b=800, b=300 and b=800, and b=600 and b=800 s/mm2 images, respectively. For 3.0T, ADCb2b600, ADCb4b600, ADCb7b600, ADCb10b600, ADCb15b600, ADCb20b600, and ADCb30b600, ADCb46b600, ADCb60b600, ADCb72b600, ADCb100b600, ADCb150b600, ADCb200b600, and ADCb400b600, were calculated from b=2 and b=600, b=4 and b=600, b=7 and b=600, b=10 and b=600, b=15 and b=600, b=20 and b=600, b=30 and b=600, b=46 and b=800, b=60 and b=600, b=72 and b=600, b=100 and b=600, b=150 and b=600, b=200 and b=600, and b=400 and b=600 s/mm2 images, respectively.
For each case, the mean signal intensity of each ROI was weighted by the number of pixels included in each ROI in different slices, then the average of the weighted DDVDs was calculated to obtain the value for each case. The measures of liver parenchyma were used to normalize the measures of spleen parenchyma, HCC, cysts, and hemangiomas. Data are presented graphically. For statistics, SDC values were tested by Mann-Whitney U test. A P value <0.05 was considered statistically significant, >0.1 as not significant, and between 0.05 and 0.1 as with a trend of significance.
Results
The DDVDspleen/DDVDliver ratio results with the 2ndb-value started being very low and increased to 800 s/mm2 for 1.5T data and 600 s/mm2 for 3.0T are shown in Figure 5. For 1.5T’s DDVDb0b1, the ratio of DDVDspleen/DDVDliver was 1.24; from DDVDb0b15 to DDVDb0b80, the ratio of DDVDspleen/DDVDliver stayed around or slightly below 1. For 3.0T, from DDVDb0b2 to DDVDb0b100, the ratio of DDVDspleen/DDVDliver stayed around or slightly below 1. From DDVDb0b100 to DDVDb0b800 for 1.5T and from DDVDb0b150 to DDVDb0b600 for 3.0T, DDVDspleen/DDVDliver ratio became increasingly higher.
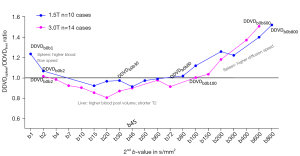
The ratios of SDCspleen/SDCliver and SDCcyst/SDCliver for 1.5T data are shown in Figure 6A, and the ratios of SDCspleen/SDCliver, SDCHCC/SDCliver, SDCcyst/SDCliver, and SDChemangioma/SDCliver for 3.0T data are shown in Figure 6B. For both 1.5T and 3.0T data, SDCspleen/SDCliver was >1, and a trend was noted with ‘SDChemangioma/SDCliver’ > ‘SDCcyst/SDCliver’ > ‘SDCHCC/SDCliver’ > ‘SDCspleen/SDCliver’. Absolute SDC values of the above data are shown in Figure 7, with the hemangiomas having the highest median SDC value. Parametric visualizations of these results are shown in Figures 8-11.
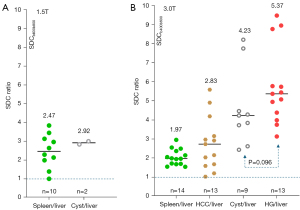
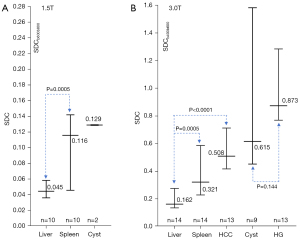
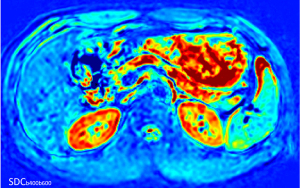
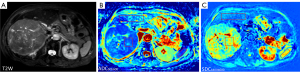
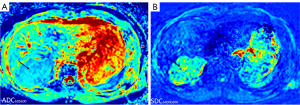
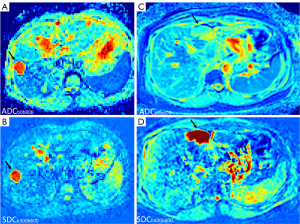
ADCspleen/ADCliver ratio from ADCb1b800 to ADCb600b800 for 1.5T data and from ADCb2b600 to ADCb400b600 for 3.0T data are shown in Figure 12, with all the ratio values being <0.81.
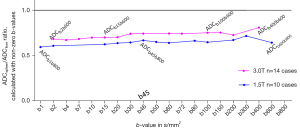
Discussion
The results in Figure 5 show the change of DDVD values following the increase of the 2ndb-value. Note that, an application of the diffusion gradients will lead to a decrease in observed T2 for tissues, which can be interpreted as an application of diffusion gradients is associated with a longer TE for data acquisition (30,31). Our modeling analysis shows that, when the 2ndb-value is very low (such as 1 s/mm2), liver and spleen DDVD measures are contributed by both blood pool volume and blood flow speed; however, when the 2ndb-value is only low (such as 10 or 20 s/mm2), then liver and spleen DDVD measures are more contributed by blood pool volume (13). It has been reviewed that the blood pool volume of the liver is slightly larger than that of the spleen, while the blood flow speed of the spleen is slightly faster than that of the liver [see Tab. 1 and Tab. 2 in (14)]. Figure 5 shows that, when the 2ndb-value was 1 s/mm2, DDVDspleen/DDVDliver was slightly larger than 1. When the 2ndb-value was between >2 and 100 s/mm2, a shorter T2 of the liver than that of the spleen (liver T2 =40 ms, spleen T2 =60 ms, 3.0T data) might have led to a faster initial signal decay during DWI signal acquisition and promotes ‘fast compartment’ measurement (6,31), and with these 2ndb-value ranges, DDVDspleen/DDVDliver was around 0.9 (Figure 5). This remains also reasonable since liver blood pool volume is indeed slightly larger than that of the spleen. Following the 2ndb-value being higher and higher, liver and spleen DDVD values were contributed more and more by slow diffusion (13). DDVDspleen/DDVDliver ratio returned to 1 when the 2ndb-value was around 80 s/mm2 for 1.5T and around 100 s/mm2 for 3.0T. After the 2ndb-value was ≥150 s/mm2, the slow diffusion’s contribution to DDVD further built up, and the measure for spleen became higher than the measure for liver. According to the IVIM theory, the perfusion contribution would already have been very small at the stage when the 2ndb-value is ≥150 s/mm2. In addition, since liver and spleen have similar amounts of perfusion, data in Figure 5 show that when the 2ndb-value was sufficiently large, slow diffusion started to dominate the DDVD value resulting in DDVDspleen being measured higher than DDVDliver. Such a DDVD observation suggests that it is possible to predominately measure the slow diffusion, and thus the conceiving of the SDC metric (Figure 4). In Figure 5, the changes of the trends were more ‘substantial’ for 3.0T data than for 1.5T data. This is likely due to that the T2 relaxation times were shorter at 3.0T than at 1.5T.
In this study, as shown in Figures 6,7, we confirmed that SDCspleen was higher than SDCliver. This is consistent with the fact that spleen has a higher extent of free water (thus likely with a faster slow diffusion than that of the liver). We also confirmed that SDCHCC was higher than SDCliver, and this agrees with the facts that HCC tumorous tissue has a higher water extent, being higher perfused and being perfused mainly with arterial blood (while liver parenchyma is perfused about 3/4 with venous blood). Another interesting observation is that SDChemangioma is higher than SDCcyst. Earlier literature reported substantially higher ADCs for cysts than for hemangiomas (Figure 3). Due to the ‘flushing’ of blood flow inside the hemangioma, it is reasonable that the diffusion of hemangiomas liquid is faster than that of the more ‘static’ liquid of the cysts. Note that, the size of a water molecule is 280 picometers. If we consider that the size of a hepatocyte is 25 µm in diameter, then the size ratio of water molecule to hepatocyte is about 1/100,000. If we consider that the size of a spleen cell is 6.5 µm in diameter, then the size ratio of water molecule to spleen cell is about 1/26,000. Hepatic sinusoids (about 10–15 µm in diameter) are also quite ‘big’ considering the size of a water molecule. Water molecules may be freer to move inside in vivo tissue than we anticipated.
As ADC measure is heavily affected by T2 (6), in addition to minimizing the perfusion information, one of the goals of using SDC is also to minimize the T2 effect. For tissues with T2 less than 70 ms (such as spleen and HCC), the advantage of SDC over ADC has been demonstrated (Figures 6,7). For these tissues’ ADC, we consider them to be ‘fast diffusion compartment dominant’, and longer T2 depresses the measure for ADC’s ‘fast diffusion compartment’ (6). By focusing on the ‘slow diffusion compartment’, SDC measured higher values for the spleen and HCC than for the liver. This is likely to reflect truly faster ‘slow diffusion’ of the spleen and HCC than that of the liver. For tissues with T2 of more than 80 ms (such as cyst), longer T2 promotes the measure for ADC’s ‘slow diffusion compartment’ (6). For SDC, despite cysts having longer T2 than hemangiomas (Figure 3), SDChemangioma was higher than SDCcyst, thus SDC is not dominated by the T2 effect. The T2 effect would ever exist, but this might be further mitigated by only using a small to moderate interval between the high b-value and the higher b-value for SDC calculation. Note that liver hemangiomas have a very high DDVD measure while the DDVD of liver cysts is close to zero when properly measured (14,32).
As the liver has a rather short T2, DW images of the liver with higher b-values are commonly associated with a low signal-to-noise ratio. This study used historical liver IVIM data, and we did not purposely try to optimize image quality with high b-values. For the absolute SDC values, the 95% confidence intervals as shown in Figure 7 were overall large. This is partly due to some of the lesion sizes being small. Figure 7 also shows absolute SDC values from different scanners/different magnetic fields may not be directly comparable. In our study, TE for 1.5T acquisition (=63 ms) and for 3.0T acquisition (=59 ms) are slightly different. A small but anticipated difference in Δ (time interval between the two motion probing pulses) and δ (time duration of the motion probing pulses) among different scanner models might also have contributed to the absolute SDC value difference between 1.5T results and 3.0T results. Our earlier experience with DDVD is that the ratio results were more comparable (14), and this is also tentatively shown in Figure 6. For DDVD-based differential diagnosis with the same MRI scanner, DDVD ratio results have been shown to be ‘somewhat’ better, but not massively better, than absolute DDVD values at a group level (33-35).
In this study, we tested whether ADC values calculated using Eq. [3] but with various combinations of high b-values could play a similar role as SDC. However, with all the combinations of liver DWI clinically applicable b-values as shown in Figure 12, spleen ADCs were all lower than liver ADCs. Therefore, ADC value calculated with a combination of high b-values cannot replace SDC. The literature results of IVIM-Dslow also differ from SDC. IVIM-Dslow and IVIM-PF for the spleen have been consistently measured much lower than that of the liver (15). In most literature reports, IVIM-Dslow for HCC has been measured lower than that of the surrounding liver parenchyma (36-38). Therefore, IVIM-Dslow also cannot replace SDC. Note that, though in principle SDC and IVIM-Dslow measure the same phenomenon, the actual calculation approaches are different. In its most simplified term, IVIM-Dslow is calculated according to:
S(b0) is affected by T2 of the target tissues. Therefore, the compounding factor of S(b0) is introduced. Though histology and imaging data such as those of perfusion CT and DDVD show HCCs tend to be hypervascular, in most IVIM literature, PF for HCC has been reported to be lower than PF of the adjacent liver (33,36,38). For IVIM analysis excluding b=0 s/mm2 data, as such when curving fitting starts from b=2 s/mm2, IVIM results are still heavily affected T2 (39). It has also been described that IVIM analysis poorly characterizes liver hemangioma (40).
There are many limitations to this study. The assumptions of: (I) the spleen has a faster diffusion than the liver; (II) HCC has a faster diffusion than the surrounding liver parenchyma; and (III) liver hemangioma has a faster diffusion than liver cyst, are all based on theorization, rather than actual experimental validation. While MRI perfusion measures can be validated such as the results in Figure 2 and the liver IVIM-PF being approximately 20% (15,36), diffusion measures cannot be validated (41). This study aimed only to prove the principle that the spleen and HCC have a faster diffusion than liver parenchyma, and hemangiomas have a faster diffusion than the simple liver cysts, while we did not pre-calculate the statistical power for significance of among various entities’ SDC. For the 3.0T data ratio difference of SDChemangioma/SDCliver and SDCcyst/SDCliver, the P value was only 0.096. However, we highly anticipate that, given sufficient sample size, the observed trend will be statistically significant. For the patient studies with HCC, potential variability such as liver fibrosis will affect ratios. The primary goal of this study was not to recommend the wider adoption of the diffusion metric SDC, which will require more testing with various organs and with different pathological entities. Data acquisition optimization for SDC also remains to be further explored. How SDC is sensitive to the TE parameter, as compared with that of ADC, should be explored in future studies. We also anticipate that further improvement of DWI data acquisition techniques will enhance the stability of DDVD and SDC measurement.
Conclusions
In conclusion, with the diffusion metrics SDC, in this study we achieved our primary goal of confirming that, the spleen has a faster diffusion than the liver, HCC tumorous tissues on average have a faster diffusion than the surrounding liver parenchyma, and hemangiomas have a faster diffusion than the simple liver cysts. There is a possibility that SDC will be a useful diffusion metric in clinical practice. Theoretically, an application of four DWI b-values, such as b=0, 10, 600, 800 s/mm2, will allow the generation of three parameters, i.e., DDVD, SDC, and ADC, with ADC considered a composite biomarker commonly dominated by T2 effect and with its features in various disease entities well characterized. This may further improve disease classifications.
Acknowledgments
None.
Footnote
Funding: This study received funding from a
Conflicts of Interest: All authors have completed the ICMJE uniform disclosure form (available at https://qims.amegroups.com/article/view/10.21037/qims-2025-537/coif). Y.X.J.W. serves as the Editor-in-Chief of Quantitative Imaging in Medicine and Surgery. Y.X.J.W. is the founder of Yingran Medicals Ltd., which develops medical image-based diagnostics software. B.H.X. contributed to the development of Yingran Medicals Ltd. The other author has no conflicts of interest to declare.
Ethical Statement: The authors are accountable for all aspects of the work in ensuring that questions related to the accuracy or integrity of any part of the work are appropriately investigated and resolved. This is a retrospective analysis of previously prospectively acquired liver IVIM data. The study was conducted in accordance with the Declaration of Helsinki (as revised in 2013). All IVIM data were acquired with institutional ethical approval and with informed consent obtained from individual participants.
Open Access Statement: This is an Open Access article distributed in accordance with the Creative Commons Attribution-NonCommercial-NoDerivs 4.0 International License (CC BY-NC-ND 4.0), which permits the non-commercial replication and distribution of the article with the strict proviso that no changes or edits are made and the original work is properly cited (including links to both the formal publication through the relevant DOI and the license). See: https://creativecommons.org/licenses/by-nc-nd/4.0/.
References
- Wáng YXJ, Zhao KX, Ma FZ, Xiao BH. The contribution of T2 relaxation time to MRI-derived apparent diffusion coefficient (ADC) quantification and its potential clinical implications. Quant Imaging Med Surg 2023;13:7410-6. [Crossref] [PubMed]
- Wáng YXJ, Ma FZ. A tri-phasic relationship between T2 relaxation time and magnetic resonance imaging (MRI)-derived apparent diffusion coefficient (ADC). Quant Imaging Med Surg 2023;13:8873-80. [Crossref] [PubMed]
- Wáng YXJ. The very low magnetic resonance imaging apparent diffusion coefficient (ADC) measure of abscess is likely due to pus's specific T2 relaxation time. Quant Imaging Med Surg 2023;13:8881-5. [Crossref] [PubMed]
- Wáng YXJ, Aparisi Gómez MP, Ruiz Santiago F, Bazzocchi A. The relevance of T2 relaxation time in interpreting MRI apparent diffusion coefficient (ADC) map for musculoskeletal structures. Quant Imaging Med Surg 2023;13:7657-66. [Crossref] [PubMed]
- Wáng YXJ. Natural course of apparent diffusion coefficient (ADC) change after brain ischemic stroke: an alternative explanation by the triphasic relationship between T2 and ADC. Quant Imaging Med Surg 2024;14:9848-55. [Crossref] [PubMed]
- Wáng YXJ. An explanation for the triphasic dependency of apparent diffusion coefficient (ADC) on T2 relaxation time: the multiple T2 compartments model. Quant Imaging Med Surg 2025;15:3779-91. [Crossref]
- Lutsep HL, Albers GW, DeCrespigny A, Kamat GN, Marks MP, Moseley ME. Clinical utility of diffusion-weighted magnetic resonance imaging in the assessment of ischemic stroke. Ann Neurol 1997;41:574-80. [Crossref] [PubMed]
- DeMulder D, Ascher SM. Uterine Leiomyosarcoma: Can MRI Differentiate Leiomyosarcoma From Benign Leiomyoma Before Treatment? AJR Am J Roentgenol 2018;211:1405-15. [Crossref] [PubMed]
- Bura V, Pintican RM, David RE, Addley HC, Smith J, Jimenez-Linan M, Lee J, Freeman S, Georgiu C. MRI findings in-between leiomyoma and leiomyosarcoma: a Rad-Path correlation of degenerated leiomyomas and variants. Br J Radiol 2021;94:20210283. [Crossref] [PubMed]
- Barral M, Placé V, Dautry R, Bendavid S, Cornelis F, Foucher R, Guerrache Y, Soyer P. Magnetic resonance imaging features of uterine sarcoma and mimickers. Abdom Radiol (NY) 2017;42:1762-72. [Crossref] [PubMed]
- Wáng YXJ. Living tissue intravoxel incoherent motion (IVIM) diffusion MR analysis without b=0 image: an example for liver fibrosis evaluation. Quant Imaging Med Surg 2019;9:127-33. [Crossref] [PubMed]
- Xiao BH, Huang H, Wang LF, Qiu SW, Guo SW, Wáng YXJ. Diffusion MRI Derived per Area Vessel Density as a Surrogate Biomarker for Detecting Viral Hepatitis B-Induced Liver Fibrosis: A Proof-of-Concept Study. SLAS Technol 2020;25:474-83. [Crossref] [PubMed]
- Ma FZ, Xiao BH, Wáng YXJ. MRI signal simulation of liver DDVD (diffusion derived 'vessel density') with multiple compartments diffusion model. Quant Imaging Med Surg 2025;15:1710-8. [Crossref] [PubMed]
- Ju ZG, Leng XM, Xiao BH, Sun MH, Huang H, Hu GW, Zhang G, Sun JH, Zhu MSY, Guglielmi G, Wáng YXJ. Influences of the second motion probing gradient b-value and T2 relaxation time on magnetic resonance diffusion-derived 'vessel density' (DDVD) calculation: the examples of liver, spleen, and liver simple cyst. Quant Imaging Med Surg 2025;15:74-87. [Crossref] [PubMed]
- Yu WL, Xiao BH, Ma FZ, Zheng CJ, Tang SN, Wáng YXJ. Underestimation of the spleen perfusion fraction by intravoxel incoherent motion MRI. NMR Biomed 2023;36:e4987. [Crossref] [PubMed]
- Greenway CV, Stark RD. Hepatic vascular bed. Physiol Rev 1971;51:23-65. [Crossref] [PubMed]
- Wáng YXJ, Li CY, Yao DQ, Tang SN, Xiao BH. The impacts of time of echo (TE) and time of repetition (TR) on diffusion-derived ‘vessel density’ (DDVD) measurement: examples of liver, spleen, and hepatocellular carcinoma. Quant Imaging Med Surg 2025;15:3771-8. [Crossref]
- Yao DQ, King AD, Zhang R, Xiao BH, Wong LM, Wáng YXJ. Assessing parotid gland tumor perfusion with a new imaging biomarker DDVD (diffusion-derived vessel density): promising initial results. Rofo 2025; Epub ahead of print. [Crossref] [PubMed]
- Martirosian P, Boss A, Deimling M, Kiefer B, Schraml C, Schwenzer NF, Claussen CD, Schick F. Systematic variation of off-resonance prepulses for clinical magnetization transfer contrast imaging at 0.2, 1.5, and 3.0 tesla. Invest Radiol 2008;43:16-26. [Crossref] [PubMed]
. Physiology, Spleen.Kapila V Wehrle CJ Tuma F 2025 .- Yeung J, Sivarajan S, Treibel TA, Rosmini S, Fontana M, Gillmore JD, Hawkins PN, Punwani S, Moon JC, Taylor SA, Bandula S. Measurement of liver and spleen interstitial volume in patients with systemic amyloid light-chain amyloidosis using equilibrium contrast CT. Abdom Radiol (NY) 2017;42:2646-51. [Crossref] [PubMed]
- Yamada I, Aung W, Himeno Y, Nakagawa T, Shibuya H. Diffusion coefficients in abdominal organs and hepatic lesions: evaluation with intravoxel incoherent motion echo-planar MR imaging. Radiology 1999;210:617-23. [Crossref] [PubMed]
- Parikh T, Drew SJ, Lee VS, Wong S, Hecht EM, Babb JS, Taouli B. Focal liver lesion detection and characterization with diffusion-weighted MR imaging: comparison with standard breath-hold T2-weighted imaging. Radiology 2008;246:812-22. [Crossref] [PubMed]
- Tokgoz O, Unlu E, Unal I, Serifoglu I, Oz I, Aktas E, Caglar E. Diagnostic value of diffusion weighted MRI and ADC in differential diagnosis of cavernous hemangioma of the liver. Afr Health Sci 2016;16:227-33. [Crossref] [PubMed]
- Erturk SM, Ichikawa T, Kaya E, Yapici O, Ozel A, Mahmutoglu AS, Basak M. Diffusion tensor imaging of cysts, hemangiomas, and metastases of the liver. Acta Radiol 2014;55:654-60. [Crossref] [PubMed]
- Fenlon HM, Tello R, deCarvalho VL, Yucel EK. Signal characteristics of focal liver lesions on double echo T2-weighted conventional spin echo MRI: observer performance versus quantitative measurements of T2 relaxation times. J Comput Assist Tomogr 2000;24:204-11. [Crossref] [PubMed]
- Cittadini G, Santacroce E, Giasotto V, Rescinito G. Focal liver lesions: characterization with quantitative analysis of T2 relaxation time in TSE sequence with double echo time. Radiol Med 2004;107:166-73. [PubMed]
- Cieszanowski A, Szeszkowski W, Golebiowski M, Bielecki DK, Grodzicki M, Pruszynski B. Discrimination of benign from malignant hepatic lesions based on their T2-relaxation times calculated from moderately T2-weighted turbo SE sequence. Eur Radiol 2002;12:2273-9. [Crossref] [PubMed]
- Nam SJ, Park KY, Yu JS, Chung JJ, Kim JH, Kim KW. Hepatic cavernous hemangiomas: relationship between speed of intratumoral enhancement during dynamic MRI and apparent diffusion coefficient on diffusion-weighted imaging. Korean J Radiol 2012;13:728-35. [Crossref] [PubMed]
- Egnell L, Jerome NP, Andreassen MMS, Bathen TF, Goa PE. Effects of echo time on IVIM quantifications of locally advanced breast cancer in clinical diffusion-weighted MRI at 3 T. NMR Biomed 2022;35:e4654. [Crossref] [PubMed]
- Führes T, Riexinger AJ, Loh M, Martin J, Wetscherek A, Kuder TA, Uder M, Hensel B, Laun FB. Echo time dependence of biexponential and triexponential intravoxel incoherent motion parameters in the liver. Magn Reson Med 2022;87:859-71. [Crossref] [PubMed]
- Hu GW, Li CY, Zhang G, Zheng CJ, Ma FZ, Quan XY, Chen W, Sabarudin A, Zhu MSY, Li XM, Wáng YXJ. Diagnosis of liver hemangioma using magnetic resonance diffusion-derived vessel density (DDVD) pixelwise map: a preliminary descriptive study. Quant Imaging Med Surg 2024;14:8064-82. [Crossref] [PubMed]
- Li XM, Yao DQ, Quan XY, Li M, Chen W, Wáng YXJ. Perfusion of hepatocellular carcinomas measured by diffusion-derived vessel density biomarker: Higher hepatocellular carcinoma perfusion than earlier intravoxel incoherent motion reports. NMR Biomed 2024;37:e5125. [Crossref] [PubMed]
- Lu BL, Yao DQ, Wáng YXJ, Zhang ZW, Wen ZQ, Xiao BH, Yu SP. Higher perfusion of rectum carcinoma relative to tumor-free rectal wall: quantification by a new imaging biomarker diffusion-derived vessel density (DDVD). Quant Imaging Med Surg 2024;14:3264-74. [Crossref] [PubMed]
- Li CY, Chen L, Ma FZ, Chen JQ, Zhan YF, Wáng YXJ. High performance of the diffusion magnetic resonance imaging biomarker diffusion-derived 'vessel density' (DDVD) for separating placentas associated with pre-eclampsia from placentas in normal pregnancy. Quant Imaging Med Surg 2025;15:1-14. [Crossref] [PubMed]
- Li YT, Cercueil JP, Yuan J, Chen W, Loffroy R, Wáng YX. Liver intravoxel incoherent motion (IVIM) magnetic resonance imaging: a comprehensive review of published data on normal values and applications for fibrosis and tumor evaluation. Quant Imaging Med Surg 2017;7:59-78. [Crossref] [PubMed]
- Yue X, Lu Y, Jiang Q, Dong X, Kan X, Wu J, Kong X, Han P, Yu J, Li Q. Application of Intravoxel Incoherent Motion in the Evaluation of Hepatocellular Carcinoma after Transarterial Chemoembolization. Curr Oncol 2022;29:9855-66. [Crossref] [PubMed]
- Ma FZ, Wáng YXJ T. (2) relaxation time elongation of hepatocellular carcinoma relative to native liver tissue leads to an underestimation of perfusion fraction measured by standard intravoxel incoherent motion magnetic resonance imaging. Quant Imaging Med Surg 2024;14:1316-22. [Crossref] [PubMed]
- Xiao BH, Wáng YXJ. Different tissue types display different signal intensities on b = 0 images and the implications of this for intravoxel incoherent motion analysis: Examples from liver MRI. NMR Biomed 2021;34:e4522. [Crossref] [PubMed]
- Wáng YXJ, Sabarudin A. Underestimation of liver hemangioma perfusion fraction by standard intravoxel incoherent motion diffusion magnetic resonance imaging. Quant Imaging Med Surg 2024;14:2128-35. [Crossref] [PubMed]
- Le Bihan D, Turner R, Moonen CT, Pekar J. Imaging of diffusion and microcirculation with gradient sensitization: design, strategy, and significance. J Magn Reson Imaging 1991;1:7-28. [Crossref] [PubMed]