3D computed tomography density of stage T1 lung cancer, which value is most stable for predicting its invasiveness?
Introduction
Predicting the invasiveness of pulmonary nodules through preoperative imaging is a clinical difficulty, yet it is extremely important for preoperative planning. The most commonly used indicators in the clinic to identify the subtypes of early lung adenocarcinoma are the specific conditions of the size of pulmonary nodules, computed tomography (CT) value, volume and proportion of solid components, and morphological characteristics of pulmonary nodules (such as spiculation sign, pleural indentation sign, etc.) (1-6). The measurement of CT value is rather convenient. It can not only quantify the density of the lesion, serve as one of the criteria for determining whether the pulmonary nodule progresses during the follow-up period, but also predict the infiltration degree of early lung adenocarcinoma. However, it should be noted that when measuring, the solid components, vacuoles, and bronchi in the lesion should be avoided to ensure its accuracy.
Early lung adenocarcinoma is divided into four stages: atypical adenomatous hyperplasia (AAH), adenocarcinoma in situ (AIS), minimally invasive carcinoma (MIA), and invasive adenocarcinoma (IAC). During the progressive process, the tumor cells continuously increase and become denser, and the growth pattern also changes from mainly lepidic growth to acinar, papillary, micropapillary, and solid types, and the density increases accordingly. Besides the tumor cells, the alveolar septum also continuously thickens, also showing an increase in density. Correspondingly, the gas content in the lesion lung continuously decreases, which is manifested as an increase in CT value on CT.
Preoperative assessment of the subtype of lung adenocarcinoma is of crucial importance in the planning of the surgical resection range. The average CT value, as the arithmetic average of all CT values within a lung cancer lesion, provides a comprehensive reflection of the tumor’s overall density, influenced by the CT values of all pixels within the tumor, offering a general description of the tumor’s density characteristics, is the most widely used indicator currently.
However, compared with the maximum CT value, median CT value, 90th percentile of CT value, 10th percentile of CT value, and minimum CT value, each parameter possesses a distinct significance and function within the realm of CT imaging. The maximum CT value, for instance, reflects the area of highest density within a lesion, potentially corresponding to the most dense components within a tumor, such as calcifications or hemorrhages. This parameter aids in discerning the special structures or components within a tumor. The 90th percentile of CT values represents the higher end of the CT value distribution, highlighting the areas of relatively high density within a tumor. This can be utilized to assess tumor heterogeneity, which refers to the variation in density across different regions of the tumor. In contrast, the median CT value, which is the middle value when the tumor’s CT values are sorted in ascending order, is less affected by extreme values and thus represents the central tendency of tumor CT values more robustly, particularly in cases of uneven data distribution. The 10th percentile of CT values focuses on the lower density areas within a tumor, assisting in understanding the distribution of cystic, necrotic, or fatty components with lower densities within the tumor. Lastly, the minimum CT value reflects the area of lowest density within a lung cancer lesion, potentially corresponding to necrotic, cystic, or mucinous components with low density, which is also of significant importance for determining the internal structure and composition of the tumor. which CT density measurement value is the most stable in the differentiation of T1 stage lung adenocarcinoma subtypes? Whether the average CT value is the best indicator in clinical application? No relevant consensus or guideline has been seen to recommend it. The three-dimensional (3D) CT value seems to be more accurate than the two-dimensional (2D) CT value (7,8).
Based on the Shukun artificial intelligence workstation, this paper quantitatively analyzes the above-mentioned characteristics related to the CT density of the clinical data and thin-layer CT images of some patients with AAH, AIS, MIA, and IAC confirmed by surgery in six centers including Zhengzhou People’s Hospital, Cancer Hospital Chinese Academy of Medical Sciences, The First Affiliated Hospital of Zhengzhou University, Huadong Hospital Affiliated to Fudan University, Beijing Liangxiang Hospital, and the Ninth People’s Hospital of Zhengzhou, hoping to find the CT density parameters that can stably predict invasiveness to help clinical make more accurate surgical planning. We present this article in accordance with the STROBE reporting checklist (available at https://qims.amegroups.com/article/view/10.21037/qims-24-2127/rc).
Methods
Clinical data
This study retrospectively analyzed clinical data from six medical centers: Zhengzhou People’s Hospital, Cancer Hospital Chinese Academy of Medical Sciences, The First Affiliated Hospital of Zhengzhou University, Huadong Hospital Affiliated to Fudan University, Beijing Liangxiang Hospital, and the Ninth People’s Hospital of Zhengzhou. The study focused on surgically confirmed cases of AAH, AIS, MIA, and IAC. The study was conducted in accordance with the Declaration of Helsinki (as revised in 2013). The study was approved by the Institutional Review Board of The Fifth Clinical Medical College of Henan University of Chinese Medicine (Zhengzhou People’s Hospital) (No. 2024011150), and the others hospitals was informed and agreed with the study. The requirement for individual consent for this retrospective analysis was waived due to the retrospective nature of the study.
A total of 2,080 nodules were selected, with the following distribution: Zhengzhou People’s Hospital (n=931), Cancer Hospital of the Chinese Academy of Medical Sciences (n=564), The First Affiliated Hospital of Zhengzhou University (n=266), Huadong Hospital affiliated with Fudan University (n=243), Beijing Liangxiang Hospital (n=62), and Zhengzhou Ninth People’s Hospital (n=14). The temporal distribution of nodules ranged from November 2017 to November 2023 for Zhengzhou People’s Hospital, August 2023 to April 2024 for Cancer Hospital of the Chinese Academy of Medical Sciences, June 2023 to December 2023 for The First Affiliated Hospital of Zhengzhou University, May 2012 to September 2018 for Huadong Hospital affiliated with Fudan University, May 2017 to December 2023 for Beijing Liangxiang Hospital, and November 2017 to January 2024 for Zhengzhou Ninth People’s Hospital.
Chest CT imaging was performed using one of eight CT systems: Siemens Somatom Definition (Germany), Siemens Somatom Force (Germany), Siemens Somatom Definition AS (Germany), Philips Brilliance 16 (The Netherlands), Philips IQon Spectral CT (The Netherlands), Philips Healthcare (The Netherlands), GE Discovery CT750 HD (the United States), and NeuViz 16Classic (China). Nodules were imaged using multirow (64 rows) spiral CT (at least 16 rows) with a slice thickness ≤1.5 mm.
Inclusion criteria
Lung nodules with a maximum 3D diameter ≤3 cm. Chest CT scans with a slice thickness of <1.5 cm obtained within one month prior to surgery in the supine position. No prior neoadjuvant therapy (including chemotherapy, targeted therapy, immunotherapy, radiation therapy, etc.). All images were accurately identified and quantifiable for detailed relevant parameters using the Shukun Technology Full Chest Diagnostic Module. All were surgically resected and pathologically confirmed as AAH, AIS, MIA, and IAC.
Exclusion criteria
Lung nodules with a maximum 3D diameter >3 cm were excluded; CT scans with a slice thickness >1.5 cm or with significant respiratory or motion artifacts that compromised diagnosis were excluded. Patients who had received any form of neoadjuvant therapy prior to surgery were excluded. Lung nodules with unclear pathological types or with disputed diagnoses were excluded. Cases where the Shukun Technology Full Chest Diagnostic Module could not accurately identify the nodule or quantify the relevant parameters were excluded. Incomplete clinical data were excluded.
Data collection and grouping
DICOM (Digital Imaging and Communications in Medicine) format data of thin-section imaging that met the inclusion and exclusion criteria were imported into the Shukun artificial intelligence workstation. The Shukun AI workstation is crucial for quantifying pulmonary nodules. Its Lung Nodule Intelligent Auxiliary Analysis System uses computer vision and deep learning to quickly detect and identify nodules from chest CT scans. It can outline suspected nodules, precisely measure them, and generate automated diagnostic data, presenting clear results on the interface for physicians. The surgically resected nodules were identified, and the CT density-related features recognized by the software, including maximum, 90th percentile, mean, median, 10th percentile, and minimum, were downloaded and summarized. These features were then combined with basic clinical history, including patient age, sex, lobar distribution, maximum 3D diameter, and postoperative pathology, for comprehensive organization. All experimental data were independently collected and organized by two separate researchers. For unavoidable missing data, we employed the direct deletion method. Data collected were divided into non-invasive and invasive groups based on the presence or absence of surrounding tissue invasion in the postoperative pathology, with AAH, AIS, and MIA collectively referred to as the non-invasive group (1,297 cases), and IAC as the invasive group (783 cases). Although AAH and AIS are categorized as glandular precursor lesions, from the vantage point of the extant research landscape and practicing surgeons alike, MIA exhibits certain similarities in surgical resection modalities with glandular precursor lesions. Hence, this paper zeroes in on the comparison between invasive adenocarcinoma, regarded as an independent cohort, and the other three lesion categories, namely AAH, AIS, and MIA. We posit that such a grouping strategy aligns more closely with the exigencies of clinical practice, facilitating a more profound comprehension of the biological behaviors as well as the determination of optimal surgical intervention timing. Subgroup analysis was performed based on size, with T1a ≤10 mm, 10< T1b ≤20 mm, and 20< T1c ≤30 mm (Figure 1). One typical case was shown in Figure 2.
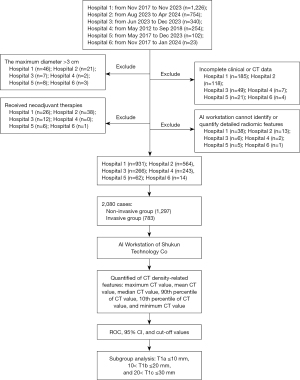
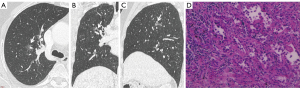
Statistical methods
Data analysis was performed using R 4.2.1. Continuous data are presented as mean ± standard deviation, and independent samples t-tests were used to compare CT density-related features between the two groups. When the data do not follow a normal distribution, the Wilcoxon method is used for the comparison between two groups. Categorical variables were compared using chi-square tests for differences in group characteristics. Receiver operating characteristics (ROC) curves for CT density-related features were plotted using SPSS 23.0, and the area under the curve (AUC) and 95% confidence intervals (CIs) were calculated, followed by subgroup analyses for T1a, T1b, and T1c groups. A P value of <0.05 was considered to indicate statistical significance.
Results
Intergroup comparison of relevant characteristics between non-invasive and invasive groups
The study population comprised 690 males (33.17%) and 1,390 females (66.83%), with an average age of 56.54±11.31 years. Nodule distribution by lung lobe included: right upper lobe (RUL) (n=728, 35.00%), right middle lobe (RML) (n=117, 5.62%), right lower lobe (RLL) (n=393, 18.89%), left upper lobe (LUL) (n=531, 25.53%), and left lower lobe (LLL) (n=311, 14.95%). A total of 2,080 nodules were analyzed, with 1,297 in the non-invasive group and 783 in the invasive group; The mean age in the non-invasive group was 54.08±11.67 years, and in the invasive group, it was 60.62±9.37 years. There were 378 males and 919 females in the non-invasive group, and 312 males and 471 females in the invasive group; The maximum 3D diameter in the non-invasive group was 10.51±4.41 mm, and in the invasive group, it was 17.71±5.76 mm. A detailed comparison of characteristics is presented in Table 1, showing statistically significant differences in patient age, maximum 3D diameter, gender, maximum CT value, 90th percentile of CT values, mean CT value, median CT value, and 10th percentile of CT values between the two groups (P<0.05).
Table 1
Variables | Total (n=2,080) | Non-invasive (n=1,297) | Invasive (n=783) | Statistic | P |
---|---|---|---|---|---|
Age (years) | 56.54±11.31 | 54.08±11.67 | 60.62±9.37 | t=−14.03 | <0.001 |
Maximum 3D diameter (mm) | 13.22±6.07 | 10.51±4.41 | 17.71±5.76 | t=−30.03 | <0.001 |
CT value | |||||
10th percentile (HU) | −649.48±180.43 | −713.52±102.21 | −543.38±226.20 | t=−19.86 | <0.001 |
90th percentile (HU) | −293.37±239.21 | −409.88±175.29 | −100.39±203.46 | t=−35.37 | <0.001 |
Maximum (HU) | −43.49±263.84 | −159.15±227.70 | 148.10±200.50 | t=−32.15 | <0.001 |
Mean (HU) | −461.66±232.93 | −566.19±141.13 | −288.50±251.18 | t=−28.35 | <0.001 |
Median (HU) | −487.38±227.03 | −587.75±132.21 | −321.14±252.36 | t=−27.38 | <0.001 |
Minimum (HU) | −811.93±132.25 | −813.45±103.81 | −809.42±169.21 | t=−0.60 | 0.548 |
Sex | χ2=25.23 | <0.001 | |||
Male | 690 (33.17) | 378 (29.14) | 312 (39.85) | ||
Female | 1,390 (66.83) | 919 (70.86) | 471 (60.15) | ||
Lobe | χ2=1.28 | 0.865 | |||
RUL | 728 (35.00) | 458 (35.31) | 270 (34.48) | ||
RML | 117 (5.63) | 75 (5.78) | 42 (5.36) | ||
RLL | 393 (18.89) | 241 (18.58) | 152 (19.41) | ||
LUL | 531 (25.53) | 336 (25.91) | 195 (24.90) | ||
LLL | 311 (14.95) | 187 (14.42) | 124 (15.84) |
Data are presented as mean ± standard deviation or number (percentage). t, t-test; χ2, Chi-squared test. 3D, three-dimensional; CT, computed tomography; HU, Hounsfield unit; LLL, left lower lobe; LUL, left upper lobe; RLL, right lower lobe; RML, right middle lobe; RUL, right upper lobe.
ROC curve analysis of CT density-related features
ROC curves were constructed using CT density-related features, including the maximum CT value, 90th percentile of CT values, mean CT value, median CT value, 10th percentile of CT values, and minimum CT value. The 90th percentile of CT values demonstrated the largest AUC of 0.863, with a 95% CI of 0.864 to 0.879. At the maximum Youden’s index (0.5788), the cut-off value for the 90th percentile of CT values was −241.5 HU, with a sensitivity of 82.652%, specificity of 75.223%, and accuracy of 79.856%. Subsequently, the maximum CT value, mean CT value, median CT value, and 10th percentile of CT values, as well as the minimum CT value, were assessed, with the ROC curves depicted in Figure 3. The 90th percentile and the maximum CT value showed no statistically significant difference after being tested by DeLong’s test.
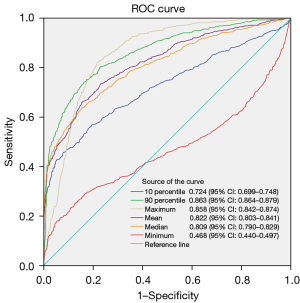
Subgroup analysis of T1a category
In the T1a subgroup, there were a total of 864 nodules (778 in the non-invasive group and 86 in the invasive group), Patient age, maximum 3D diameter, maximum CT value, 90th percentile of CT values, mean CT value, median CT value, 10th percentile of CT values, and minimum CT value all showed statistically significant differences (P<0.05). Subgroup analysis of the T1a category and the re-construction of ROC curves for CT density-related features revealed that the 90th percentile of CT values had the largest AUC of 0.830, with a 95% CI of 0.778 to 0.881. At the maximum Youden’s index (0.5657), the cut-off value for the 90th percentile of CT values was −294.5 HU, with a sensitivity of 77.907%, specificity of 78.663%, and accuracy of 78.588%. Subsequently, the maximum CT value, mean CT value AUC, median CT value, and 10th percentile of CT values were assessed, with the maximum CT value having an AUC of 0.825 (0.778–0.881), a cut-off value of −47.5 HU, a sensitivity of 76.744%, and a specificity of 79.563%. Details are provided in Table 2 and Figure 4. The 90th percentile and other CT density values (excluding the maximum CT value) were found to have statistically significant differences after being tested by DeLong’s test.
Table 2
Variables | Total (n=864) | Non-invasive (n=778) | Invasive (n=86) | Statistic | P |
---|---|---|---|---|---|
Age (years) | 52.11±11.64 | 51.53±11.66 | 57.30±10.19 | t=−4.41 | <0.001 |
Maximum 3D diameter (mm) | 7.80±1.64 | 7.70±1.64 | 8.73±1.38 | t=−6.39 | <0.001 |
CT value | |||||
10th percentile (HU) | −680.33±118.17 | −694.66±100.19 | −550.65±176.38 | t=−7.44 | <0.001 |
90th percentile (HU) | −388.56±193.58 | −415.26±171.57 | −147.09±214.21 | t=−11.22 | <0.001 |
Maximum (HU) | −204.96±225.98 | −232.54±208.48 | 44.57±226.27 | t=−11.60 | <0.001 |
Mean (HU) | −531.25±168.31 | −554.88±142.42 | −317.41±225.40 | t=−9.56 | <0.001 |
Median (HU) | −550.59±155.55 | −572.41±130.69 | −353.20±213.90 | t=−9.31 | <0.001 |
Minimum (HU) | −773.69±108.85 | −781.36±97.68 | −704.28±166.32 | t=−4.22 | <0.001 |
Sex | χ2=1.26 | 0.262 | |||
Male | 190 (21.99) | 167 (21.47) | 23 (26.74) | ||
Female | 674 (78.01) | 611 (78.53) | 63 (73.26) | ||
Lobe | χ2=2.14 | 0.711 | |||
RUL | 274 (31.71) | 249 (32.01) | 25 (29.07) | ||
RML | 55 (6.37) | 47 (6.04) | 8 (9.30) | ||
RLL | 175 (20.25) | 158 (20.31) | 17 (19.77) | ||
LUL | 212 (24.54) | 193 (24.81) | 19 (22.09) | ||
LLL | 148 (17.13) | 131 (16.84) | 17 (19.77) |
Data are presented as mean ± standard deviation or number (percentage). t, t-test; χ2, Chi-squared test. 3D, three-dimensional; CT, computed tomography; HU, Hounsfield unit; LLL, left lower lobe; LUL, left upper lobe; RLL, right lower lobe; RML, right middle lobe; RUL, right upper lobe.
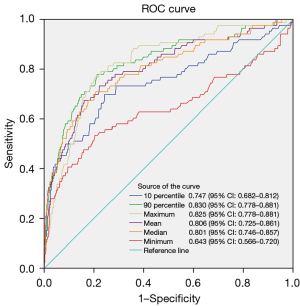
Subgroup analysis of T1b category
In the T1b subgroup, there were a total of 922 nodules (472 in the non-invasive group and 450 in the invasive group). Patient age, maximum 3D diameter, maximum CT value, 90th percentile of CT values, mean CT value, median CT value, 10th percentile of CT values, minimum CT value, and lobar distribution all exhibited statistically significant differences (P<0.05). Subgroup analysis of the T1b category and the re-construction of ROC curves for CT density-related features indicated that the 90th percentile of CT values had the highest AUC of 0.843, with a 95% CI of 0.817 to 0.868. At the maximum Youden’s index (0.5426), the cut-off value for the 90th percentile of CT values was −241.5 HU, with a sensitivity of 73.111%, specificity of 81.144%, and accuracy of 77.223%. Subsequently, the mean CT value AUC, median CT value, maximum CT value, 10th percentile of CT values, and minimum CT value were assessed, with specific details provided in Table 3 and Figure 5. The 90th percentile and other CT density values all showed statistically significant differences after being tested by DeLong’s test.
Table 3
Variables | Total (n=922) | Non-invasive (n=472) | Invasive (n=450) | Statistic | P |
---|---|---|---|---|---|
Age (years) | 58.95±10.18 | 57.72±10.71 | 60.24±9.43 | t=−3.80 | <0.001 |
Maximum 3D diameter (mm) | 14.71±2.81 | 13.78±2.41 | 15.68±2.88 | t=−10.84 | <0.001 |
CT value | |||||
10th percentile (HU) | −642.66±195.29 | −737.83±100.14 | −542.83±219.55 | t=−17.21 | <0.001 |
90th percentile (HU) | −260.42±242.51 | −401.75±180.33 | −112.18±208.56 | t=−22.51 | <0.001 |
Maximum (HU) | 33.70±225.43 | −65.94±205.62 | 138.21±196.21 | t=−15.43 | <0.001 |
Mean (HU) | −442.39±246.66 | −580.72±140.24 | −297.30±250.92 | t=−21.03 | <0.001 |
Median (HU) | −472.01±243.27 | −607.69±133.72 | −329.69±250.95 | t=−20.85 | <0.001 |
Minimum (HU) | −825.82±136.88 | −854.34±93.27 | −795.91±166.01 | t=−6.55 | <0.001 |
Sex | χ2=0.65 | 0.422 | |||
Male | 377 (40.89) | 187 (39.62) | 190 (42.22) | ||
Female | 545 (59.11) | 285 (60.38) | 260 (57.78) | ||
Lobe | χ2=12.18 | 0.016 | |||
RUL | 329 (35.68) | 184 (38.98) | 145 (32.22) | ||
RML | 48 (5.21) | 27 (5.72) | 21 (4.67) | ||
RLL | 177 (19.20) | 81 (17.16) | 96 (21.33) | ||
LUL | 239 (25.92) | 128 (27.12) | 111 (24.67) | ||
LLL | 129 (13.99) | 52 (11.02) | 77 (17.11) |
Data are presented as mean ± standard deviation or number (percentage). t, t-test; χ2, Chi-squared test. 3D, three-dimensional; CT, computed tomography; HU, Hounsfield unit; LLL, left lower lobe; LUL, left upper lobe; RLL, right lower lobe; RML, right middle lobe; RUL, right upper lobe.
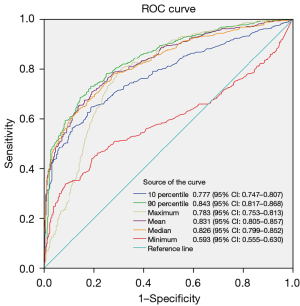
Subgroup analysis of T1c category
In the T1c subgroup, there were a total of 294 nodules (47 in the non-invasive group and 247 in the invasive group). Patient age, maximum CT value, 90th percentile of CT values, mean CT value, median CT value, 10th percentile of CT values, and minimum CT value all showed statistically significant differences (P<0.05). Subgroup analysis of the T1c category and the re-construction of ROC curves for CT density-related features revealed that the 90th percentile of CT values maintained the highest AUC of 0.893, with a 95% CI of 0.850 to 0.936. At the maximum Youden’s index (0.6355), the cut-off value for the 90th percentile of CT values was −241 HU, with a sensitivity of 82.979%, specificity of 80.567%, and accuracy of 80.952%. Subsequently, the mean CT value AUC, median CT value, 10th percentile of CT values, maximum CT value, and minimum CT value were assessed, with specific details provided in Table 4 and Figure 6. After being tested by DeLong’s test, there was no statistically significant difference among the 90th percentile, mean, and median. However, there were statistically significant differences between the 90th percentile and the other two values.
Table 4
Variables | Total (n=294) | Non-invasive (n=47) | Invasive (n=247) | Statistic | P |
---|---|---|---|---|---|
Age (years) | 62.01±8.74 | 59.70±9.33 | 62.45±8.58 | t=−1.98 | 0.048 |
Maximum 3D diameter (mm) | 24.47±2.80 | 24.18±3.12 | 24.52±2.73 | t=−0.77 | 0.439 |
CT value | |||||
10th percentile (HU) | −580.19±249.43 | −781.55±72.66 | −541.87±252.77 | t=−12.44 | <0.001 |
90th percentile (HU) | −116.97±222.28 | −402.45±185.21 | −62.65±184.12 | t=−11.59 | <0.001 |
Maximum (HU) | 188.98±184.18 | 119.72±184.25 | 202.16±181.56 | t=−2.85 | 0.005 |
Mean (HU) | −317.59±272.07 | −607.55±105.21 | −262.41±258.79 | t=−15.33 | <0.001 |
Median (HU) | −349.87±277.39 | −641.38±99.18 | −294.40±265.49 | t=−15.60 | <0.001 |
Minimum (HU) | −880.75±143.67 | −933.87±63.06 | −870.64±152.31 | t=−4.73 | <0.001 |
Sex | χ2=1.96 | 0.162 | |||
Male | 123 (41.84) | 24 (51.06) | 99 (40.08) | ||
Female | 171 (58.16) | 23 (48.94) | 148 (59.92) | ||
Lobe | χ2=6.99 | 0.137 | |||
RUL | 125 (42.52) | 25 (53.19) | 100 (40.49) | ||
RML | 14 (4.76) | 1 (2.13) | 13 (5.26) | ||
RLL | 41 (13.95) | 2 (4.26) | 39 (15.79) | ||
LUL | 80 (27.21) | 15 (31.91) | 65 (26.32) | ||
LLL | 34 (11.56) | 4 (8.51) | 30 (12.15) |
Data are presented as mean ± standard deviation or number (percentage). t, t-test; χ2, Chi-squared test. 3D, three-dimensional; CT, computed tomography; HU, Hounsfield unit; LLL, left lower lobe; LUL, left upper lobe; RLL, right lower lobe; RML, right middle lobe; RUL, right upper lobe.
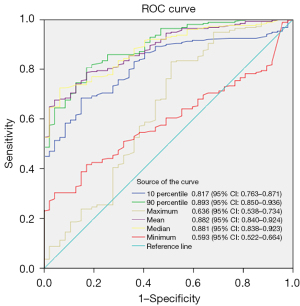
Discussion
Ground-glass opacity (GGO) lung cancer is a specialized variant of pulmonary adenocarcinoma, characterized by its unique radiographic presentation. The evaluation of its invasive potential is pivotal for determining the optimal timing for intervention and for devising surgical strategies (3,9-14). In clinical practice, the assessment is predominantly conducted through the interpretation of CT imaging features, which are utilized to make empirical judgments. CT features, including diameter, density, and morphological characteristics, have all been utilized in the prediction of lung adenocarcinoma invasiveness. However, due to potential inconsistencies among physicians regarding morphological features, diameter and density exhibit greater repeatability. Quantitative parameters from artificial intelligence, with their precision, have also seen numerous applications compared to manual measurements (1,15-17). The precision of quantitative parameters from artificial intelligence surpasses manual measurements, making them widely used in this context. Tamura et al. (18) analyzed 494 patients with stage IA lung cancer (140 in the pre-invasive group, including AAH and AIS, and 354 in the invasive group, including MIA and IAC) and found that the mean CT value for the pre-invasive group was 643.6±9.4 HU, compared to 365.9±11.4 HU for the invasive group. This suggests that mean CT values can predict the invasiveness of stage IA lung adenocarcinoma and aid in preoperative decisions regarding sublobar resection. He et al. (19) conducted a meta-analysis confirming that a mean CT value of −557 HU effectively differentiates between pre-invasive and invasive groups of lung adenocarcinoma, and a mean CT value of −484 HU can distinguish between MIA and IAC. After analyzing 180 cases of pure ground-glass nodule (GGN) (9 AAH, 108 AIS, 56 MIA, and 7 IAC), Ichinose et al. (20) discovered that a maximum CT value of ≥−300 HU is also a useful predictor of histological invasiveness, effectively distinguishing between pre-invasive and invasive groups. We found that multiple CT density metrics, including the maximum CT value, average CT value, median CT value, 90th percentile of CT value, 10th percentile of CT value, and minimum CT value, all have value in predicting the invasiveness of lung adenocarcinoma. However, there have been no reports on which CT density measurement is the most stable for predicting T1 stage lung adenocarcinoma.
In medical imaging, 3D and 2D CT values refer to measurements of tissue density obtained through CT scans. 2D CT values are derived from the two-dimensional (planar) images of CT scans, commonly used to assess tissue density on a single slice and a frequently utilized parameter by radiologists in the diagnostic process. The aforementioned studies (18-20) were all based on 2D CT values. In contrast, 3D CT values are obtained from the three-dimensional volumetric data of CT scans. Kitazawa et al. (8) analyzed 66 cases of non-invasive and 30 cases of invasive adenocarcinoma and found that the mean 3D CT density value had an AUC of 0.838 for predicting invasive adenocarcinoma, with a cut-off value of −489 HU, outperforming the mean 2D CT density value. 3D CT values appear to offer more precise measurements, especially when assessing tumor volume, shape, and other three-dimensional characteristics. The CT density-related features used in this study are all based on 3D CT values from the Shukun Artificial Intelligence workstation, including maximum CT value, 90th percentile of CT values, mean CT value, median CT value, 10th percentile of CT value, and minimum CT value.
Our data revealed that among the 3D CT density-related features for predicting the invasiveness of stage T1 lung adenocarcinoma, the 90th percentile of CT values demonstrated the best performance. Unlike the commonly used mean CT value, the 90th percentile refers to a value below which 90% of all measured pixel values fall, representing the top 10% of pixels with the highest density. This appears to offer stronger representativeness. The 90th percentile of CT values at −241.5 HU was identified as the optimal threshold for predicting T1 stage invasiveness.
Subsequently, the mean and median CT values showed relatively stable performance, with the mean CT value slightly outperforming the median. The maximum CT value exhibited the least stability, with predictive performance second only to the 90th percentile in the T1a subgroup, but regressing to second to last among the seven features in the T1c subgroup. The 10th percentile and minimum CT values showed poor predictive performance and are not recommended for predicting the invasiveness of stage T1 lung adenocarcinoma.
Study limitation
There are certain limitations in this study. The data used in this study are all retrospective, which may introduce a certain degree of selection bias. The data sources of this text are based on the imaging quantification results from various centers, we did not conduct random reviews on the acquired data, which may lead to certain biases to some extent. Additionally, there may be variations in CT equipment, image scanning parameters, post-image processing across different centers, and patient’s body habitus which may have an impact on the accuracy of the results. In this study, cases of AAH, AIS, and MIA were classified as non-invasive, while IAC was classified as invasive, this classification may be more beneficial for surgical planning. However, there are also studies that classify AAH and AIS as pre-invasive groups and MIA and IAC as invasive groups. Further analysis is needed to clarify the most appropriate classification approach.
Conclusions
In summary, even though the 90th percentile does not always show an advantage over other CT density values as tested by DeLong’s test, regardless of the magnitude of the differences, it remains the most stable predictor of the invasiveness of stage T1 lung adenocarcinoma, with the cut-off value of −241.5 HU. The performance of mean and median CT values was relatively stable. The predictive performance of the maximum CT value significantly decreased as the nodule diameter increased. Based on subgroup analysis, we recommend using the 90th percentile and maximum CT values for prediction when the pulmonary nodule diameter is ≤10 mm. For nodules with a diameter between >10 mm and ≤30 mm, assessment should be conducted using the 90th percentile and mean CT values. Regarding the comparison between single variables and combinations of multiple variables, we will conduct further investigations in subsequent research.
Acknowledgments
We would like to express our sincere appreciation and gratitude to those who helped to coordinate the study.
Footnote
Reporting Checklist: The authors have completed the STROBE reporting checklist. Available at https://qims.amegroups.com/article/view/10.21037/qims-24-2127/rc
Funding: None.
Conflicts of Interest: All authors have completed the ICMJE uniform disclosure form (available at https://qims.amegroups.com/article/view/10.21037/qims-24-2127/coif). L.Z. is an employee of Shukun (Beijing) Technology Co., Ltd., which provided data analysis and interpretation for this research. But the company had no role in the study design, data collection, analysis, or manuscript preparation. The other authors have no conflicts of interest to declare.
Ethical Statement: The authors are accountable for all aspects of the work in ensuring that questions related to the accuracy or integrity of any part of the work are appropriately investigated and resolved. The study was conducted in accordance with the Declaration of Helsinki (as revised in 2013). The study was approved by the Institutional Review Board of The Fifth Clinical Medical College of Henan University of Chinese Medicine (Zhengzhou People’s Hospital) (No. 2024011150), and the others hospitals was informed and agreed with the study. The requirement for individual consent for this retrospective analysis was waived due to the retrospective nature of the study.
Open Access Statement: This is an Open Access article distributed in accordance with the Creative Commons Attribution-NonCommercial-NoDerivs 4.0 International License (CC BY-NC-ND 4.0), which permits the non-commercial replication and distribution of the article with the strict proviso that no changes or edits are made and the original work is properly cited (including links to both the formal publication through the relevant DOI and the license). See: https://creativecommons.org/licenses/by-nc-nd/4.0/.
References
- Liu J, Yang X, Li Y, Xu H, He C, Zhou P, Qing H. Predicting the Invasiveness of Pulmonary Adenocarcinomas in Pure Ground-Glass Nodules Using the Nodule Diameter: A Systematic Review, Meta-Analysis, and Validation in an Independent Cohort. Diagnostics (Basel) 2024;14:147. [Crossref] [PubMed]
- Yang Y, Xu J, Wang W, Zhao J, Yang Y, Wang B, Ye L. Meta-analysis of the correlation between CT-based features and invasive properties of pure ground-glass nodules. Asian J Surg 2023;46:3405-16. [Crossref] [PubMed]
- Liu J, Yang X, Li Y, Xu H, He C, Qing H, Ren J, Zhou P. Development and validation of qualitative and quantitative models to predict invasiveness of lung adenocarcinomas manifesting as pure ground-glass nodules based on low-dose computed tomography during lung cancer screening. Quant Imaging Med Surg 2022;12:2917-31. [Crossref] [PubMed]
- Chu ZG, Li WJ, Fu BJ, Lv FJ. CT Characteristics for Predicting Invasiveness in Pulmonary Pure Ground-Glass Nodules. AJR Am J Roentgenol 2020;215:351-8. [Crossref] [PubMed]
- Heidinger BH, Anderson KR, Nemec U, Costa DB, Gangadharan SP, VanderLaan PA, Bankier AA. Lung Adenocarcinoma Manifesting as Pure Ground-Glass Nodules: Correlating CT Size, Volume, Density, and Roundness with Histopathologic Invasion and Size. J Thorac Oncol 2017;12:1288-98. [Crossref] [PubMed]
- Zhang D, Li C, Luo QQ, Wang G. Preinvasive and Invasive Lung Adenocarcinoma Appearing as Part-Solid Ground-Glass Nodules: Differentiation with CT Value and Solid-Part Diameter. Journal of Medical Imaging and Health Informatics 2017;7:1817-22. [Crossref]
- Xie XJ, Liu SY, Chen JY, Zhao Y, Jiang J, Wu L, Zhang XW, Wu Y, Duan H, He B, Luo H, Han D. Development of unenhanced CT-based imaging signature for BAP1 mutation status prediction in malignant pleural mesothelioma: Consideration of 2D and 3D segmentation. Lung Cancer 2021;157:30-9. [Crossref] [PubMed]
- Kitazawa S, Saeki Y, Kobayashi N, Kikuchi S, Goto Y, Sato Y. Three-dimensional mean CT attenuation value of pure and part-solid ground-glass lung nodules may predict invasiveness in early adenocarcinoma. Clin Radiol 2019;74:944-9. [Crossref] [PubMed]
- Liu L, Ni Z, Zhang J, Zhao J, Shen J. Application of artificial intelligence-based dual source CT scanning in the differentiation of lung adenocarcinoma in situ and minimally invasive adenocarcinoma. Pak J Med Sci 2024;40:271-6. [PubMed]
- Wu FZ, Wu YJ, Tang EK. An integrated nomogram combined semantic-radiomic features to predict invasive pulmonary adenocarcinomas in subjects with persistent subsolid nodules. Quant Imaging Med Surg 2023;13:654-68. [Crossref] [PubMed]
- Gao R, Gao Y, Zhang J, Zhu C, Zhang Y, Yan C. A nomogram for predicting invasiveness of lung adenocarcinoma manifesting as pure ground-glass nodules: incorporating subjective CT signs and histogram parameters based on artificial intelligence. J Cancer Res Clin Oncol 2023;149:15323-33. [Crossref] [PubMed]
- Willner J, Narula N, Moreira AL. Updates on lung adenocarcinoma: invasive size, grading and STAS. Histopathology 2024;84:6-17. [Crossref] [PubMed]
- Wang YF, Deng HY, Huang W, Zhou Q. Segmentectomy for early-stage non-small-cell lung cancer with invasive characteristics: the definitions of invasiveness and feasibility of segmentectomy. Eur J Cardiothorac Surg 2022;62:ezac236. [Crossref] [PubMed]
- Huo J, Luo T, Lv F, Li Q. Clinicopathological and computed tomography features associated with recurrence-free survival of patients with small-sized peripheral invasive lung adenocarcinoma after sublobectomy. Quant Imaging Med Surg 2023;13:8144-56. [Crossref] [PubMed]
- Kudo Y, Nakamura T, Matsubayashi J, Ichinose A, Goto Y, Amemiya R, Park J, Shimada Y, Kakihana M, Nagao T, Ohira T, Masumoto J, Ikeda N. AI-driven Characterization of Solid Pulmonary Nodules on CT Imaging for Enhanced Malignancy Prediction in Small-sized Lung Adenocarcinoma. Clin Lung Cancer 2024;25:431-9. [Crossref] [PubMed]
- Zhang H, Wang D, Li W, Tian Z, Ma L, Guo J, Wang Y, Sun X, Ma X, Ma L, Zhu L. Artificial intelligence system-based histogram analysis of computed tomography features to predict tumor invasiveness of ground-glass nodules. Quant Imaging Med Surg 2023;13:5783-95. [Crossref] [PubMed]
- Zhang B, Yu F, Peng M. Artificial intelligence-assisted CT characterizations and quantitative analysis for differentiating pre-invasive lesions from invasive adenocarcinomas in pulmonary subsolid nodules ≤ 2cm. J Clin Oncol 2020;38:e21614. [Crossref]
- Tamura M, Matsumoto I, Saito D, Yoshida S, Kakegawa S, Takemura H. Mean Computed Tomography Value to Predict the Tumor Invasiveness in Clinical Stage IA Lung Cancer. Ann Thorac Surg 2017;104:261-6. [Crossref] [PubMed]
- He S, Chen C, Wang Z, Yu X, Liu S, Huang Z, Chen C, Liang Z, Chen C. The use of the mean computed-tomography value to predict the invasiveness of ground-glass nodules: A meta-analysis. Asian J Surg 2023;46:677-82. [Crossref] [PubMed]
- Ichinose J, Kawaguchi Y, Nakao M, Matsuura Y, Okumura S, Ninomiya H, Oikado K, Nishio M, Mun M. Utility of Maximum CT Value in Predicting the Invasiveness of Pure Ground-Glass Nodules. Clin Lung Cancer 2020;21:281-7. [Crossref] [PubMed]