The role of artificial intelligence in the diagnosis of diabetic retinopathy through retinal lesion features: a narrative review
Introduction
According to the World Health Organization (WHO), diabetic retinopathy (DR) is a common complication of diabetes (1) and is the leading cause of blindness in working-age adults. When the blood sugar level increases in the body, the blood vessels are damaged in the retina, located in the backside of the eye. This damage can cause blood vessels to leak fluid or bleed, leading to swelling and distortion of the retina (2). Diabetic patients can have a risk of developing DR, and the risk increases with the duration of diabetes. The risk of developing DR can also be influenced by factors such as, high cholesterol, high blood pressure, poor blood sugar control, and smoking (3).
DR can be classified into two types: non-proliferative DR (NPDR) and proliferative DR (PDR). NPDR is the early stage of the disease and is characterized by the presence of small, dilated blood vessels in the retina, as well as tiny deposits of fluid or blood. PDR, on the other hand, is a more advanced stage and is characterized by the growth of new, abnormal blood vessels in the retina (4). If left untreated, DR can lead to complete vision loss. However, due to accurate and early identification and treatment of DR, the risk of blindness can be greatly reduced. Treatment options include laser surgery to seal leaking blood vessels and injections of medication to stop the growth of abnormal blood vessels. People with diabetes should have regular eye examinations to monitor for the development of DR (5). Automatic retina screening is highly recommended not only to save manual tasks because the cost of manual tasks is very high and time-consuming, but also to scale up the tasks (6). In addition, non-invasive procedure for DR screening is more suitable, because most of the patients are above 45 years of age. According to medical researchers and ophthalmologists, fundus scans are a comfortable and non-invasive technique that optometrists use during DR screening. There are different parameters and retinal abnormalities that indicate DR, namely as neovascularization, hemorrhages, microaneurysms, cotton wool spots, and exudates, are examined for the screening of DR (7). Figure 1 shows the difference between normal retina and DR (8).
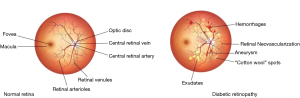
According to the international clinical DR (ICDR), a standardized scaling protocol mostly used for DR grading including proliferative, severe, moderate, mild, and normal, and also for clinical scales. Most artificial intelligence (AI)-based approaches used ICDR for the classification and grading of DR (9). In general, DR is subdivided into two main categories which are NPDR and PDR. The non-proliferative is also divided into severe, moderate, and mild NPDR (10). The details of benchmark features for DR are provided in Table 1 (11). The outflow of the blood vessels into the retinal part is considered an abnormal feature of DR represented as NPDR and there is an inflamed and wet condition at the end retina. The abnormal features of the retina include microaneurysms, exudates, and hemorrhage which can be found at the level of NPDR. The retinal hemorrhages are considered as blood dots presented on the retina. Similarly, exudates are also the basic cause of two types of DR, where hard retinal exudates are represented as yellow waxy spots, whereas soft retinal exudates are identified as the small size of white or yellow dots having distracted edges in the back side of the eye (12). Furthermore, microaneurysms are considered early symptoms of DR because of new abnormal retinal neovascularization. Figure 2 represents the different levels of DR; the images taken from publicly available Indian diabetic retinopathy image dataset (IDRiD) (13).
Table 1
Standard features of DR | ICDR scaling |
---|---|
Neovascularization (new vessels); vitreous/pre-retinal hemorrhage | PDR |
More than 20 intra-retinal hemorrhages in all four quadrants; definite venous beading in two or more quadrants; prominent intra-retinal micro-vascular abnormality in one or more quadrants; no signs of PDR | Severe NPDR |
Number of signs of micro-aneurysms, dot or blot hemorrhages, and HEs or cotton wool spots, no signs of severe NPDR | Moderate NPDR |
The basic signs of retinal micro-aneurysms | Mild NPDR |
No abnormalities | No DR |
DR, diabetic retinopathy; HE, hard exudate; ICDR, international clinical DR; NPDR, non-proliferative DR; PDR, proliferative DR.

Recently, the development of medical image processing has modernized the sectors of computer vision and healthcare. The improved level of medical imaging provides clinical experts with the supreme vision of human organs to help them with accurate diagnosis, which leads toward optimal and early treatment plans. The high-resolution images captured on both soft and hard tissue structures provide valuable insights (14). Medical image analysis helps resolve the clinical challenges through applying different kinds of image processing techniques to deliver the detailed analysis of medical scans, supporting applications in diagnosis, treatment, and patient monitoring. The general process of medical image analysis based on different stages includes image preprocessing involving image de-noising, image enhancement and color space transformation, while image segmentation is for detect boundaries and objects, whereas feature extraction stage is to capture or extract the meaningful information from the images and image classification stage is used to classify different levels of disease or to handle the grading tasks of medical imaging based challenges (15).
Artificial intelligence (AI) has the potential to solve the problems of ophthalmology including screening in resource-limiting regions, and rising eye care costs (16) by providing accurate and efficient diagnoses with telemedicine, remote care and reduced cost, personalized treatment plans, and better patient outcomes. Particularly, AI-enabled models integrate various machine learning (ML) and deep learning (DL) algorithms to enhance the efficacy of system architectures deployed commercially for detecting DR disease (17). AI-based algorithms can be used for the analysis of retinal images for early and accurate detection of DR to help ophthalmologists for timely treatment of the disease. Furthermore, AI can help to accelerate the discovery of new drugs for ophthalmic diseases by analyzing large datasets and predicting the efficacy of potential drug candidates (18). In DR management, early detection and timely treatment can reduce the level of DR. Currently, AI performing a significant role along with advanced approaches of ML and DL to empower the systems for DR screening in an accurate manner. The automated DR detection (19) approaches can reduce the cost and effort, as well as providing more effective way of DR diagnosis than traditional schemes (20). Generally, there are three basic applications of AI applied through supervised learning mechanisms, namely segmentation, classification, and prediction (21). Supervised learning-based segmentation is a machine-learning mechanism that can be used for the automated detection and segmentation of various features in DR images. This approach requires a dataset of labeled images, where each image is annotated with information about the location and boundaries of specific features, such as exudates, hemorrhages, and cotton wool spots. Whereas, supervised learning-based classification supports the automatic categorization of DR scans into different classes based on their severity. This approach requires a dataset of labeled images, where each image is annotated with information about its severity level, such as mild, moderate, or severe DR. Supervised learning-based prediction can be used for the prediction of DR progression in individual patients on the basis of their medical history and clinical data. This approach requires a dataset of labeled patient data, where each patient is classified into different categories based on their DR progression, such as stable, progressing, or advanced stages of the disease (21). The basic concept of the DR diagnostic process is presented in Figure 3 (22).
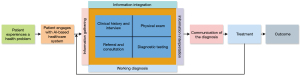
The world’s latest survey by the American Academy of Ophthalmology reported that 103.12 million adults are diagnosed with DR and this number could be increased to 160.50 million by 2045 (23). For early identification and better management of DR, AI-based methods are applied for retinal abnormality detection, but still, there is also a lot of AI potential that needs to be discovered with advanced DL approaches, because, AI with related techniques would take part in the eye care and treatment and a major contribution in healthcare business. As a result, there is a lot of interest in AI, due to lowering costs in healthcare and improvement in eye treatment. The review covers together technical and clinical perspectives of using the AI-based systems in clinical institutes to help the ophthalmologists by interpreting and diagnosis of DR at the early stages. Furthermore, the key challenges of interpreting AI predictions in clinical settings and the potential for integrating explainable AI (XAI) approaches and clinical implications are covered to improve the acceptance and trust of ophthalmologists on AI applications. The review would also explain the importance of retinal multimodal imaging, datasets, AI-based approaches, and evaluation metrics to analyze the performance of the methodologies in the case of DR segmentation and classification. The main purpose of this review article is to help young research experts in the area of ophthalmology and AI for a better understanding of retinal issues and to continue their work to solve real-world problems. We present this article in accordance with the Narrative Review reporting checklist (available at https://qims.amegroups.com/article/view/10.21037/qims-24-1791/rc).
The major contributions of the study are as follows:
- The detailed review covers more than one decade to analyze the automated DR diagnosis through retinal lesion features.
- The details of private and publicly available datasets used for DR segmentation, classification, and prediction are provided with possible accessibility and limitations concerning dataset size, diversity, and the generalizability of models are presented.
- A detailed review of traditional and advanced AI-based approaches for DR segmentation, classification, and prediction has been incorporated with comparative analysis to identify the particular gaps in existing studies.
- AI-based DR segmentation, classification, and prediction approaches have been evaluated and various performance metrics are provided with descriptions and formulas to guide the readers.
- Moreover, the authors performed critical analysis to understand the practical deployment challenges in diverse clinical settings and provided possible solutions to address the research problems regarding DR diagnostics.
- Finally, a freely available repository is created to facilitate the researchers with relevant up-to-date articles and open-source implementations in the field of DR diagnosis at https://github.com/muhammadmateen319/progress-of-diabetic-retinopathy-screening.
Research methodology
This section is composed of the basic components of the DR diagnostic system, in the initial stage; we provided the details of DR-related datasets and the accessibility criteria. After that, AI-based methods related to the diagnosis of DR, collected through Web of Science, Google Scholar, and PubMed are evaluated and critical analysis of the existing studies is performed. In the next phase, performance evaluation metrics used in the diagnosis of DR, are presented with a description. The summary of the search strategy is presented in Table 2. The eligible criterion was fixed on the experimental and clinical outcomes of DR, including retinal lesion features. Moreover, ML and DL approaches for DR detection and classification. The keywords used to search the related information were included as DR, microaneurysms, exudates, retinal hemorrhage, neovascularization, ML, and advanced DL approaches. The extracted information taken from each paper was saved in a separate document, where findings were related to experimental and clinical articles, datasets, methodologies, and outcomes of technical literature with evaluation metrics, while few studies contained insufficient information, so couldn’t be accessed the complete version of those studies.
Table 2
Items | Specification |
---|---|
Date of search | May 25th, 2024 |
Databases and other sources searched | Web of Science, Google Scholar, and PubMed |
Search terms used | The keywords used to search the related information were included as DR, microaneurysms, exudates, retinal hemorrhage, neovascularization, ML, and advanced DL approaches |
Timeframe | From 2015 to 2024 |
Inclusion criteria | Restricted to English articles |
Selection process | D.X. and B.N. independently participated in the selection process of relevant studies and Tao Peng served as an arbitrator, the inclusion of studies carried out through the agreement of all three authors |
DL, deep learning; DR, diabetic retinopathy; ML, machine learning.
Datasets
The dataset is a basic component of the proposed framework used for the analysis of the specified task. The collection of data is the collection of records that preserve useful information, for example, medical or insurance records used by a specified system to complete the assigned task (24). There are several types of retinal imaging techniques used in ophthalmology to visualize the retina, and diagnose and monitor eye diseases. Multimodal retinal imaging can assist diagnostic approaches for more accurate detection of retinal abnormalities including DR (25). The applications of multimodal imaging are becoming more useful due to advanced AI-based disease diagnostic and retinal multimodal imaging techniques are invaluable tools for ophthalmologists in diagnosing and monitoring a wide range of eye diseases and can help improve patient outcomes and quality of life. An et al. (26) applied multimodal imaging to diagnose glaucoma with optical coherence tomography (OCT) and fundus scans retinal modalities. Whereas, Vaghefi et al. (27) achieved better accuracy using multimodal imaging with OCT and fundus scans to diagnose dry age-related macular degeneration (AMD). The details of the most common retinal imaging modalities are given and shown in Figure 4.
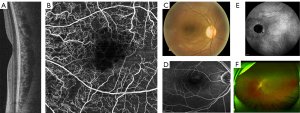
OCT is a non-invasive imaging method that creates high-resolution photographs of the retina. It is mostly used for the detection and monitoring the abnormal conditions such as diabetic macular edema (DME), macular degeneration, and glaucoma. OCT angiography (OCTA) is an emerging non-invasive imaging modality technique that has great potential to support the diagnosis of retinal vascular disease (30). Fundus photography (FP) is also a familiar technique that uses a specialized camera to take high-resolution color images of the retina. These images can provide a detailed view of the retina and are useful for diagnosing and monitoring abnormal conditions of retinal detachment, DR, and macular degeneration, while, fluorescein angiography (FA) is a technique that involves injecting a fluorescent dye into a patient’s arm and taking a series of photographs of retina. This technique is very useful for DR diagnosis. Indocyanine green angiography (ICGA) uses a tricarbocyaninedye that is better suited for imaging deeper blood vessels and evaluating choroidal vasculature. It is particularly useful for diagnosing and monitoring conditions such as choroidal neovascularization (CNV) (31). Whereas, scanning laser ophthalmoscopy (SLO) is a technique that uses a laser beam to create high-resolution images of the retina. It is particularly useful for macular degeneration and DR diagnosis (32). The datasets of DR contain information on various retinal modalities, including OCT and fundus scans mostly used for the analysis of DR. In the collection of datasets, it has come to know that most research experts used publicly available databases, which are available through specific sources. The datasets are generally split into training, and testing parts for the assessment of the proposed methodologies. In the training and testing of the proposed model, there are more important concerns or limitations need to be considered including size, diversity, and generalizability regarding datasets. Dataset size contains the basic significance while training and testing the model because insufficient data may lead towards overfitting of the model, where the model can perform well on training data, while fails to perform on external dataset. Moreover, small amount of datasets may not be able to cover sufficient variations regarding input features, which may lead to dropout the knowledge of the DL models.
The second concern of dataset is about the lack of diversity (population samples, cultural context) in the data, may lead towards the biased prediction. Furthermore, the trained model with narrow contexts would not be applicable to handle the real-world scenarios. The third important concern about the selection of the dataset is to train the model with sufficient generalizability in the data. In the case of small amount of dataset use for training the model, resultant into overfitting, limited task transfer, and real-world failures due to non-generalizability in the data and it can be handled through increase the diversity feature in the datasets (33). For example, DRIVE dataset that contains only 40 scans is considered as the small dataset labeled and annotated with high quality, which outweighs the quantity. In the DR diagnosis, DRIVE is a well-known dataset towards the researchers to segment the retinal vessels as provided high quality and richness of annotations for segmentation tasks (34,35). Furthermore, Messidor-2 dataset is also a famous dataset containing 1,748 high-quality fundus images with wide range of DR severity used for DR classification, while having lack of lesion level annotation, limited diversity, and class imbalance problem. IDRiD dataset containing 516 fundus images for diverse tasks with comprehensive notations but having image quality variability (33).
The list of datasets with possible availability status of fundus and OCT modalities is given in Table 3, whereas Figure 5 represents the citation ratio of datasets in the collection of existing studies.
Table 3
Sr.# | Database | Status | ||
---|---|---|---|---|
Freely available | On-demand | Not available | ||
Fundus datasets | ||||
1 | DR1 | √ | ||
2 | DR2 | √ | ||
3 | ARIA | √ | ||
4 | FAZ | √ | ||
5 | CHASE-DB1 | √ | ||
6 | Messidor | √ | ||
7 | Messidor-2 | √ | ||
8 | DRIVE | √ | ||
9 | DIARETDB0 | √ | ||
10 | STARE | √ | ||
11 | DIARETDB1 | √ | ||
12 | ROC | √ | ||
13 | Kaggle | √ | ||
14 | E-Ophtha | √ | ||
15 | DRiDB | √ | ||
16 | AGAR300 | √ | ||
17 | APTOS 2019 Blindness Detection | √ | ||
18 | Diabetic retinopathy detection | √ | ||
19 | IDRiD | √ | ||
20 | Diabetic retinopathy debrecen | √ | ||
21 | Retinal-lesions | √ | ||
22 | RFMiD | √ | ||
23 | FGADR | √ | ||
24 | Kaggle EyePACS | √ | ||
25 | DDR-dataset | √ | ||
26 | TMUMDH | √ | ||
27 | Moorfields Eye Hospital | √ | ||
28 | CLEOPATRA | √ | ||
29 | JMU | √ | ||
30 | SNDRSP | √ | ||
31 | LECHC DR India | √ | ||
32 | KMCM, India | √ | ||
33 | Southwest Medical University | √ | ||
34 | Rawalpindi Medical University, Pakistan | √ | ||
35 | University of California | √ | ||
36 | Silchar Medical College and Hospital | √ | ||
37 | Aravind Eye Hospital, Coimbatore | √ | ||
38 | 2nd Affiliated Hospital, Eye Center at Zhejiang University | √ | ||
39 | HUPM, Cádiz, Spain | √ | ||
OCT datasets | ||||
1 | BIOMISA | √ | ||
2 | Dukes | √ | ||
3 | OPTIMA cyst segmentation challenge | √ | ||
4 | NEH | √ | ||
5 | Mendeley (Kermany dataset) | √ | ||
6 | Dukes2 | √ | ||
7 | Retinal OCT classification challenge | √ | ||
8 | RETOUCH | √ | ||
9 | OCTID | √ | ||
10 | Zhang dataset | √ | ||
11 | Rabbani | √ | ||
12 | Diabetic retinopathy retinal OCT images | √ | ||
13 | ROAD (OCT-A dataset) | √ | ||
14 | University of Louisville Hospital (OCT data) | √ |
AGAR, Agarwal; APTOS, Asia Pacific Tele-Ophthalmology Society; ARIA, automated retinal image analysis; BIOMISA, biomedical image and signal analysis; CHASE-DB1, child health and study England-database 1; CLEOPATRA, CLinical Evaluation Of Pertuzumab And TRAstuzumab; DDR, dataset for diabetic retinopathy; DIARETDB, diabetic retinopathy database; DR, diabetic retinopathy; DRiDB, diabetic retinopathy image database; DRIVE, digital retinal images for vessel extraction; E-Ophtha, ophthalmology; EyePACS, eye picture archive communication system; FAZ, foveal avascular zone; FGADR, fine-grained annotated diabetic retinography; HUPM, Hospital Universitario Puerta del Mar; IDRiD, Indian diabetic retinopathy image dataset; JMU, Jichi Medical University; KMCM, Kasturba Medical College, Manipal; LECHC, Lotus Eye Care Hospital Coimbatore; Messidor, methods to evaluate segmentation and indexing techniques in the field of retinal ophthalmology; NEH, Noor Eye Hospital; OCT, optical coherence tomography; OCT-A, OCT-angiography; OCTID, OCT image retinal database; OPTIMA, ophthalmic image analysis; RETOUCH, retinal OCT fluid challenge; RFMiD, retinal fundus multi-disease image dataset; ROC, retinopathy online challenge; ROAD, retinal OCT-A diabetic retinopathy; SNDRSP, Singapore National Diabetic Retinopathy Screening Program; STARE, structured analysis of the retina; TMUMDH, Tianjin Medical University Metabolic Diseases Hospital.
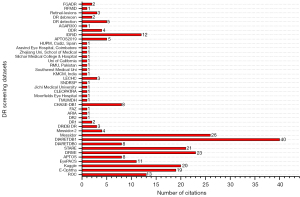
AI-based diagnosis of DR through retinal lesion features
In the diagnosis of DR, AI has also utilized the features of retinal lesions and played an essential role in classifying the stages of DR and allowing timely treatment for the patients. Many AI-based approaches have been reported for the detection of DR based on retinal lesion features using different retinal imaging modalities. This section of the review focuses on the traditional and advanced AI-based approaches from the last decade in the area of DR segmentation and detection with the most relevant retinal lesion features including microaneurysms, exudates, hemorrhages, and neovascularization as shown in Figure 6.
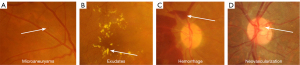
Microaneurysms detection
In DR, microaneurysms are the micro spots and basic signs that appear at an early stage in fundus images. The main reason for microaneurysms is the leakage of blood vessels in the retina, in which are later shaped as red rounded spots. The identification of microaneurysms is a critical task because of their small size and difficulty to distinguish from the surface of the retina and is important to diagnosis at the early stage of DR (36). In the domain of ophthalmology, DR is a main issue to address and various AI-based applications have been developed to diagnose the microaneurysms by identifying the red spots on the surface of retina. A few of them are going to be presented in this section to understand the development of AI for the analysis of DR. There are several classifiers have been applied for the classification of retinal abnormalities, where Lam et al. introduced an image patches-based lesion identification model using DL (37), where a dataset of 243 fundus images was used for the testing phase and examined by two eye medical experts. The Kaggle dataset was used as an input in the reported approach, which was further subdivided into retinal patches including retinal secularization, normal-appearing structures, exudates, hemorrhages, and microaneurysms. The convolutional neural network (CNN)-based approach was designed and applied for the identification and classification of lesions in five types. Furthermore, to identify the abnormality from retinal images, Chowdhury et al. (38) presented a new approach based on a random forest classifier (RFC) for accurate classification of red lesions and help the ophthalmologists. In the reported approach, ML and K-means clustering methodologies have been used to identify and classify retinal abnormalities. The classification performance of the reported approach was presented as the RFC classifier provided 93.58% of classification accuracy, which is comparatively better than 83.63% provided by Naïve Bayes. Similarly, to identify the red lesions, a new method has been introduced by Orlando et al. (39), using domain knowledge and DL approaches. The incorporation of handcrafted features learned from CNN had been performed in the reported approach to identify the red lesions and further for the classification of red lesions a RFC had been used. Moreover, an ML-based method was used to classify the DR through microaneurysm detection by Cao et al. (40). The reported approach also included principal component analysis (PCA) for the identification of microaneurysms in an appropriate manner. In this framework, data augmentation is performed and patches of 25×25 pixels were applied as input data for the identification of microaneurysms using fundus photographs. In the experimental phase, the DIARETDB1 dataset was used for the analysis of the presented approach, where PCA was used for dimensionality reduction, while random forest, support vector machine (SVM), and neural network were applied to perform the classification through the useful extracted features.
A few of them are going to be presented in Table 4 to understand the development of AI for the analysis of DR. It is notable that the highest result of microaneurysms detection in terms of sensitivity was reported 98.74% by Steffi and Sam Emmanuel (43) using ML-based resilient back-propagation approach, whereas specificity was presented 97.5% by Prasad et al. using segmentation and morphological operations (56).
Table 4
Year | Reference | Methodologies | Database | Results with evaluation metrics |
---|---|---|---|---|
2024 | Jayachandran and Ratheesh Kumar (41) | Auto weight dilated with CNN used to learnable set of parameters | RIM-ONE-R1, ORIGA, Messidor | Accuracy: 98.76%, 98.95%, 99.28% |
2024 | Alotaibi (42) | CNN, Inception-V3, transfer learning, (MA-RTCNN-Inception-V3-EOA), equilibrium optimization algorithm | E-Ophtha | Sensitivity: 75.45%; specificity: 67.63%; accuracy: 82.61%; F-measure: 77.61% |
2024 | Steffi and Sam Emmanuel (43) | ML-based resilient back-propagation to detect shape and texture features | ROC, DIARETDB1, E-Ophtha-MA, Messidor | Specificity: 97.12%; sensitivity: 98.74%; accuracy: 98.01%; AUC: 91.72% |
2023 | Alam et al. (44) | Fully CNN enhances approach, a modified GoogLeNet architecture | Kaggle, EyePACS | Mean accuracy: 87.71% |
2023 | Nunez do Rio et al. (45) | DLS, ResNet34 architectures, image preprocessing through subtraction of local average color, normalizing | SM1, SM2 | Sensitivity: 93.86%; specificity: 96.00% |
2022 | Du et al. (46) | Multi-context ensemble learning and TS | ROC, DIARETDB1, E-Ophtha-MA | FROC: 0.306, 0.429, 0.518 |
2022 | Hervella et al. (47) | Generative adversarial networks, adversarial multimodal reconstruction | DDR, ROC, E-Ophtha | Average precision: 33.55%, 31.36%, 64.90% |
2022 | Deng et al. (48) | LSAMFC, ring gradient descriptor, gradient boosting decision tree | E-Ophtha-MA, ROC | AUC: 0.9751, 0.9409 |
2021 | Ayala et al. (49) | CNN model, DenseNet121 model having three dense-blocks | Kaggle, APTOS, Messidor | Accuracy: 97.78% |
2021 | Zhang et al. (50) | Feature-distance-based algorithm, feature-transfer network | ROC, DB1, E-Ophtha-MA, RetinaCheck | Sensitivity: 98.3%, 100%, 99.3%, 100%, 96.5% |
2021 | Dai et al. (51) | DeepDR system, ResNet, mask RCNN, sub networks for assessment of image quality, lesion awareness, and DR grading | SIM cohort | AUC: 90.1% |
2020 | Deepa and Narayanan (52) | Feed forward neural networks | DIARETDB1 | Accuracy: 98.89% |
2020 | Du et al. (53) | LCT, under sampling boosting based classifier | ROC, DIARETDB1, E-Ophtha-MA | FROC: 0.293, 0.402, 0.516 |
2019 | Mateen et al. (8) | VGG-19, SVD, and PCA | Kaggle | Accuracy: 98.34% |
2018 | Lam et al. (37) | Standard five CNN models include Inception-V3, ResNet, GoogLeNet, AlexNet | E-Ophtha | AUC: 95% |
2017 | Gondal et al. (54) | CNNs for generate the class activation mappings | DIARETDB1 | AUC: 95.4% |
2016 | Paing et al. (55) | Artificial neural networks and ROI localization | DIARETDB1 | Accuracy: 96% |
2015 | Prasad et al. (56) | Segmentation approaches, morphological operations, one rule and BPNN classifier algorithms | DIARETDB1 | Specificity: 97.5%; AUC: 97%; accuracy: 97.75%; sensitivity: 97.8% |
AUC, area under the curve; APTOS, Asia Pacific Tele-Ophthalmology Society; BPNN, back-propagation neural network; CNN, convolutional neural network; DB, database; DDR, dataset for diabetic retinopathy; DIARETDB, diabetic retinopathy database; DLS, deep learning system; DR, diabetic retinopathy; E-Ophtha, ophthalmology; EOA, equilibrium optimization algorithm; EyePACS, eye picture archive communication system; FROC, free response operating characteristic; LCT, local cross-section transformation; LSAMFC, local structure awareness-based multi-feature combination; MA, microaneurysms; MA-RTCNN, micro aneurysm detection using residual-based temporal attention convolutional neural network; Messidor, methods to evaluate segmentation and indexing techniques in the field of retinal ophthalmology; ML, machine learning; ORIGA, online retinal fundus image database for glaucoma analysis and research; PCA, principal component analysis; RCNN, residual-based convolutional neural network; RIM-ONE-R1, retinal image database for optic nerve evaluation-release 1; ROC, retinopathy online challenge; ROI, region of interest; SIM, Shanghai Integrated Model; SM, SMART-India; SVD, singular value decomposition; TS, transformation splicing; VGG, visual geometry group.
Exudates detection
In the DR, exudates are also basic signs and serious retinal abnormality. There are two kinds of exudates, one is known as hard exudate (HE) and the other is called soft exudate (SE). The yellow waxy parts on the surface of the retina are known as HE whereas cotton wool spots can be considered as SE, which are white or light-yellow shape regions. The varieties of methodologies to identify the SE and HE are reported in this portion (57). Timely detection of exudate is very significant for better treatment and recovery of the affected people of DR. In this way, a study is reported to diagnose retinal exudate using residual networks (ResNet-50) and SVM for better classification outcomes (58). The authors applied various kinds of CNN approaches and then finalized the comparatively better approach for the identification of exudates with an accuracy of 98%. Furthermore, for DR analysis, an exudate segmentation approach has been introduced by Kaur and Mittal (59) to assist the retinal experts for early diagnosis, and better treatment. The discussed approach applied a dynamic decision technique for an accurate and reliable way of exudate segmentation. The reported approach was a robust method with a choice of threshold values in a dynamic way. In the defined model, 1,307 retinal images were used as input with a diversity of shapes, colors, sizes, and locations. Furthermore, a new exudate identification technique based on deep CNNs was introduced by Prentašić and Lončarić (60). Moreover, it is helpful to detect anatomical landmarks through DL approaches. The reported framework helps ophthalmologists for early detection of exudates using fundus images. A novel approach (61) has been designed to obtain information on hyper-reflective dots and HEs in OCT images for DME subjects. Bland Altman and dice coefficient were used for the evaluation of the developed approach designed for clinical practices. OCT and fundus photographs were used to detect and classify the DR and DME through two kinds of existing AI-based models, and model evaluation was performed via specificity and sensitivity (62). The relevant approaches of exudate detection are further discussed in Table 5. In the case of exudate detection, it is noteworthy that Thulkar et al. (77) achieved the highest score of specificity as 97.1% using tree-based forward search approach, while achieved 100% score of sensitivity. Furthermore, the highest results of precision with 84.03% and F1-score with 77% are achieved by Tang et al. (68) and Prentašić and Lončarić (78), respectively.
Table 5
Year | Reference | Methods | Database | Results with evaluation metrics |
---|---|---|---|---|
2024 | Van Do et al. (63) | Two-stage approach with CNN, VGGUnet, and RITM model | DDR, IDRiD | Mean Dice: 60.8%, 76.6%; mean IoU scores: 45.5%, 62.4% |
2024 | Guo et al. (64) | Deep multi-scale model, FCN for lesion segmentation | E-Ophtha, DDR, DIARETDB1, IDRiD | DSC: 69.31%; AUPR: 68.84% |
2024 | Maiti et al. (65) | Enriched encoder-decoder model with CLSTM and RES unit | DIARETDB0, Messidor, DIARETDB1, IDRiD | Overall accuracy: 97.7%; while accuracies of individual dataset respectively: 97.81%, 98.01%, 98.23%, 96.76% |
2023 | Farahat et al. (66) | U-Net and YOLOv5, Leaky ReLU | Cheikh Zaïd Foundation’s Ophthalmic Center | Specificity: 85%; sensitivity: 85%; accuracy: 99% |
2024 | Naik et al. (67) | DenseNet121 | APTOS-2019 | Accuracy: 96.64% |
2023 | Tang et al. (68) | Patch-wise density loss, global segmentation loss, discriminative edge inspection | IDRiD | Precision: 84.03%; recall: 65.14%; F1-score: 68.54 |
2022 | Reddy and Gurrala (69) |
Hybrid DLCNN-MGWO-VW, DSAM, and DDAM | IDRiD | Accuracy: 96.0% |
2022 | Hussain et al. (70) | YOLOv5M, classification-extraction-superimposition mechanism | EyePACS | Accuracy: 100% |
2023 | Sangeethaa and Jothimani (71) |
Median filtering, CLAHE | Local dataset: Aravind Eye Hospital, Coimbatore | Accuracy: 95% |
2021 | Sudha and Ganeshbabu (72) | Deep neural network, VGG-19, gradient descent, structure tensor | Kaggle | Sensitivity: 82%; accuracy: 96% |
2021 | Cincan et al. (73) | GoogLeNet, SqueezeNet, and ResNet50 models | SUSTech_SYSU | Accuracy: 0.928% |
2021 | Kurilová et al. (74) | Faster R-CNN, ResNet-50, region proposed network | DIARETDB1, Messidor, E-Ophtha-EX | AUC: 97.27%, 88.5%, 100% |
2020 | Pan et al. (75) | ResNet50, VGG-16, DenseNet, multi-label classification | 2nd Affiliated Hospital, Eye Center at Zhejiang University Hospital | AUC: 96.53% |
2020 | Theera-Umpon et al. (76) | Adaptive histogram equalization, hierarchical ANFIS, multilayer perceptron | DIARETDB1 | AUC: 99.8% |
2020 | Thulkar et al. (77) | Tree-based forward search approach, SVM | DIARETDB1, IDRiD, IDEDD | Specificity: 97.1%; sensitivity: 100% |
2019 | Khojasteh et al. (58) | DRBM, ResNet-50, CNNs | E-Ophtha, DIARETDB1 | Sensitivity: 99%; accuracy: 98% |
2019 | Chowdhury et al. (38) | Random forest and Naïve Bayes classifier approach | DIARETDB1, DIARETDB0 | Accuracy: 93.58% |
2018 | Lamet al. (37) | CNNs, AlexNet, GoogLeNet, VGG16, Inception-V3, ResNet | E-Ophtha | AUC: 95% |
2017 | Gondal et al. (54) | CNNs, for generate the class activation mappings | DIARETDB1 | AUC: 95.4% |
2016 | Paing et al. (55) | Artificial neural networks, and ROI iocalization | DIARETDB1 | Accuracy: 96% |
2015 | Prentašić and Lončarić (78) | Deep CNNs | DIARETDB1 | PPV: 77%; sensitivity: 77%; F1-score: 77% |
ANFIS, adaptive network-based fuzzy inference system; APTOS, Asia Pacific Tele-Ophthalmology Society; AUC, area under the curve; AUPR, area under the precision-recall curve; CLAHE, contrast-limited adaptive histogram equalization; CLSTM, contextual long-short term memory; CNN, convolutional neural network; DDAM, disease-dependent attention module; DLCNN, deep learning convolutional neural network; DRBM, discriminative restricted Boltzmann machine; DSC, dice similarity coefficient; DSAM, disease-specific attention module; DDR, dataset for diabetic retinopathy; DIARETDB, diabetic retinopathy database; E-Ophtha, ophthalmology; EX, exudates; EyePACS, eye picture archive communication system; FCN, fully convolutional network; IDEDD, Indian diabetic eye diseases dataset; IDRiD, Indian diabetic retinopathy image dataset; IoU, intersection over union; Messidor, methods to evaluate segmentation and indexing techniques in the field of retinal ophthalmology; MGWO, modified grey-wolf optimizer; PPV, positive predictive value; R-CNN, region-based convolutional neural network; ReLU, rectified linear unit; RES, residual extended skip; RITM, reviving iterative training with mask guidance; ROI, region of interest; SUS, Southern University of Science and Technology; SVM, support vector machine; SYSU, Sun Yat-sen university; VGG, visual geometry group; VW, variable weight; YOLOv5, you only look once version 5; YOLOv5M, you only look once version 5 medium.
Hemorrhages detection
Retinal hemorrhage is considered a basic and severe cause of DR, which exists in retinal imaging. In this scenario, accurate and early diagnosis of retinal hemorrhage is extremely helpful and important in the process of timely recovery of affected persons. The retinal hemorrhages are basically present in the deep and shallow retina. In fundus photographs, bright red lesions are presented in the backside of the eye known as the shallow retina, whereas dark red lesions exist in the deep retinal part. The identification of retinal hemorrhages is performed through different kinds of approaches which are going to be discussed in this portion (79). Hemorrhage detection for DR was performed by Wu et al. (80), where a new method has been introduced with the combination of dual approaches. The hemorrhage classification was performed using background estimation and watershed segmentation approaches. Initially, the preprocessing approach was performed through an adaptive histogram of the retinal digital fundus photographs. In the next phase of the proposed methodology, feature extraction was initiated for the purpose of segmentation. Dual approaches were performed to visualize the feature extraction of retinal hemorrhages. At the last stage, visual features were used to obtain the accuracy of hemorrhage detection, which was observed as 95.42%. The traditional data augmentation approaches namely geometric and color transformations were used by Ayhan and Berens (81) for fundus image segmentation. The author used the DNN approach for the prediction of abnormalities Kaggle database to achieve comparatively better uncertain estimation. The retinal fundus image segmentation for hemorrhage detection was performed (82) with the help of the R-sGAN technique. The presented approach followed supervised learning for synthetic fundus photographs and achieved comparatively better segmentation outcomes after the training process of the synthetic dataset. The authors (83) presented CNN’s performance enhancement approach to overcome the experimental time of the whole part to analyze the fundus images. The identification, as well as classification process of hemorrhage in retinal images, was based on heuristical samples. Furthermore, a classification approach was introduced for the detection of hemorrhages (84). The authors used a supervised approach for the overlapping segmentation for partitions of retinal color images. The feature extraction process was done through each splat, which defines features towards its neighbors and used a filter-based wrapper technique for the interaction of neighbor splats. Maqsood et al. (85) proposed a novel approach to identify the retinal hemorrhage using fundus images. Initially, contrast enhancement approach performed to get the details of edges in fundus images. The remaining stages of the reported work were based on three-dimensional (3D) CNNs for feature extraction, feature fusion approach used to fuse the extracted features and then best selective features through multi-logistic regression were used to detect the retinal hemorrhage. The reported work also focused on limitations found in the literature work including poor contrast, false hazard, disparate sizes of hemorrhage, and microaneurysms, and addressed the related issues with superior results and less computation time. The hemorrhage identification methods are discussed in Table 6. The traditional and advanced-based hemorrhage detection approaches are reported to compare the performances of the techniques by highlighting the obtained results of both trends. Guo et al. (100) used multi-class discriminant analysis and obtained 90.9% accuracy, while Sathiyaseelan et al. (86) achieved 98.6% accuracy using fast-CNN and modified U-Net architecture. Furthermore, the recent approach of Xia et al. (94) used multi-scale gated network (MGNet) with modified U-Net also achieved better results in terms of accuracy of 99.12%.
Table 6
Year | Reference | Methods | Database | Results with evaluation metrics |
---|---|---|---|---|
2024 | Sathiyaseelan et al. (86) |
Fast-CNN, modified U-Net, enhanced machine-based diagnostic | IDRiD, DIARETDB1 | Specificity: 99.6%; sensitivity: 80%; accuracy: 98.6% |
2024 | Biju and Shanthi (87) | Enhanced visual geometry group model, data augmentation, Gabor transform, hemorrhages segmentation, and classification module | HRF, DIARETDB1 | Specificity: 98.71%, 98.44%; sensitivity: 98.59%, 98.37%; accuracy: 98.66%, 98.74% |
2024 | Atlas et al. (88) | Maximally stable extremal regions approach, CNN, ELSTM, DPFE | DIARETDB2 | Specificity: 98.91%; sensitivity: 98.67% |
2023 | Li et al. (89) | CNN, ResNet-50, customized computer vision algorithm | Local dataset: University of California | Specificity: 74.5%; sensitivity: 100%; F1-score: 0.932; accuracy: 90.7% |
2023 | Alwakid et al. (90) | CLAHE filtering approach and ESRGAN network, Inception-V3 model | APTOS datasets | Case1: accuracy: 98.7%; case 2: accuracy: 80.87% |
2023 | Saranya et al. (91) | CNN, U-Net, morphological operations | Messidor, STARE DIARETDB1, IDRiD | Specificity: 99%; sensitivity: 89%; accuracy: 95.65% |
2023 | Kiliçarslan (92) | ResNet-50, YOLOv5, and VGG-19 | EyePACS | Accuracy: 93.38%, 94.75%, 91.72% |
2022 | Mondal et al. (93) | ResNeXt, DenseNet101, CLAHE | DIARETDB1, APTOS19 | Accuracy: 96.98%; precision: 0.97; recall: 0.97 |
2023 | Xia et al. (94) | MGNet, U-Net | IDRiD, DIARETDB1 | Accuracy: 99.12%; sensitivity: 53.53%; specificity: 99.66% |
2021 | Goel et al. (95) | DL, transfer learning, VGG16 | IDRiD | Accuracy: 91.8%; F1-score: 81.5%; precision: 81.5% |
2022 | Zhang et al. (96) | Inception-V3 model, class activation mapping | Kaggle | Sensitivity: 0.925; specificity: 0.907; harmonic mean: 0.916; AUC: 0.968 |
2020 | Hacisoftaoglu et al. (97) |
ResNet50, GoogLeNet, AlexNet models | Messidor-2, EyePACS, Messidor, IDRiD | Specificity: 99.1%; sensitivity: 98.2%; accuracy: 98.6% |
2020 | Li et al. (98) | DL system, inception ResNet-2 | Local dataset: ZOC | Sensitivity: 97.6%; specificity: 99.4%; AUC: 99.9% |
2019 | Chowdhury et al. (38) | Classifier: random forest and Naïve Bayes classifier approach | Messidor, Tele-Ophtha, DIARETDB1, DIARETDB0 | Accuracy: 93.58% |
2018 | Suriyal et al. (99) | DeepCNNs, MobileNets | Kaggle | Accuracy: 73.3% |
2017 | Gondal et al. (54) | CNNs, for generate the class activation | DIARETDB1 | AUC: 95.4% |
2016 | Paing et al. (55) | Artificial neural networks, and ROI localization | DIARETDB1 | Accuracy: 96% |
2015 | Guo et al. (100) | Multi-class discriminant analysis, wavelet transformation, and discrete cosine transformation | Real-world dataset | Accuracy: 90.9% |
APTOS, Asia Pacific Tele-Ophthalmology Society; AUC, area under the curve; CLAHE, contrast limited adaptive histogram equalization; CNN, convolutional neural network; DIARETDB, diabetic retinopathy database; DL, deep learning; DPFE, double-pierced feature extraction; ELSTM, enhanced long short-term memory; ESRGAN, enhanced super-resolution generative adversarial network; EyePACS, eye picture archive communication system; HRF, high-resolution fundus; IDRiD, Indian diabetic retinopathy image dataset; Messidor, methods to evaluate segmentation and indexing techniques in the field of retinal ophthalmology; MGNet, multi-scale gated network; ROI, region of interest; STARE, structured analysis of the retina; VGG, visual geometry group; YOLOv5, you only look once version 5; ZOC, Zhongshan Ophthalmic Center.
Neovascularization detection
The neovascularization detection and segmentation are the critical steps for the identification and diagnosis of DR to discriminate the blood vessels from neovascularization. Moreover, identification and segmentation of retinal blood vessels are also considered windows to diagnose brain and heart stroke, which abnormalities found through the vascular system of the retina. The segmentation of retinal blood vessels is more significant and helpful in the non-invasive fundus photograph modality (101). In the case of DR, noise removal and contrast improvement approaches were used by Sahu et al. (102) through digital color fundus images. The presented approach focused on resolving the de-noising and enhancement problems using different performance parameters namely correlation coefficient, structural similarity index, peak signal-to-noise ratio (PSNR), and edge preservation index. Moreover, Leopold et al. (103) performed better retinal segmentation based on various kinds of performance indicators. The Pixel BNN model was introduced by authors to perfume better segmentation of fundus images and the model evaluation is performed through CHASE_DB1, STARE, and DRIVE databases. The evaluation metrics namely F1-score were used to calculate the performance and computational speed of the defined model. In medical image analysis, a novel framework as a multi-stage structure has been designed by Mahapatra et al. (104) based on triplet loss function (TLF) to gradually improve image quality. Furthermore, TLF helps to achieve the quality output of the preceding photograph, which can be the input of the next photograph. The implementation of TLF obtains a higher level of image resolution, to assist in the diagnosis of eye disease through the fundus photographs. A new approach for the segmentation of retinal vessels was introduced by Wang to identify blood vessels through a complex database (105). The three different kinds of datasets have been used by authors and obtained an accuracy of 95% to 96% of vessel segmentation, which is considered as better performance than the existing relevant approaches. The authors also mentioned that the presented approach would be applicable to relevant tasks of image analysis with pattern recognition. An image matting approach was applied by a fast approach to segment the blood vessels (106). Initially, the authors used an automated tri-map for the utilization of regional features of retinal vessels. Furthermore, a hierarchical approach was applied through image matting to obtain informative data relevant to vessel pixels using region of interest (ROI). The defined approach utilized minimum computational time and achieved better performance to segment the retinal vessels. Hossain and Reza (107) presented a novel approach for DR to segment the retinal images based on Markov random field (MRF). In the reported method, MRF is adopted for experiments to get high performance of segmentation as compared to the complex other relevant segmentation approaches, and in the reported work, fundus photographs are used in blood vessel segmentation using MRF. Feng et al. (108) designed a fusion network based on pyramidal modules in U-shape architecture to achieve the challenging tasks namely retinal edema and linear lesion segmentation. Moreover, Xi et al. (109) focused on two kinds of challenges faced in CNV in the context of difficulty in small objects for effective model training and better segmentation. The reported CNN model was designed based on an attention mechanism for ROI localization and discrimination. The neovascularization detection approaches are presented in Table 7. Neovascularization detection approaches played a vital role to discriminate the blood vessels including recent advance level approach introduced by Vij and Arora (110) used hybrid deep transfer learning to perform the segmentation task to help the accurate DR diagnosis and achieved accuracy of 98.16%, while highest accuracy of 99.71% is obtained by Singh et al. (121) using enhanced R2-ATT U-Net model.
Table 7
Year | Reference | Methods | Database | Results with evaluation metrics |
---|---|---|---|---|
2024 | Vij and Arora (110) | Hybrid deep transfer learning approaches, ResNet34 + U-Net | HRF, DRIVE | Accuracy: 98.16%, 98.53%; recall: 98.28%, 98.53%; precision: 98.33%, 98.81% |
2024 | Singh et al. (111) | DL, DenseU-Net, U-Net, ATTU-Net, R2U-Net, LadderNet, R2-ATT U-Net | STARE | Accuracy: 97.1% |
2024 | Ma and Li (112) | U-Net, DFM, SFM, context squeeze, and excitation module | CHASE-DB1, STARE, DRIVE | Accuracy: 96.67%, 96.60%, 95.65% |
2023 | Kumar and Singh (113) | Ensemble based DL, ResNet-152, VGG-16, efficient Net B0, PCA | STARE | F-measure: 99.22%; recall: 98.25%; precision: 98.63%; accuracy: 99.71% |
2022 | Bhardwaj et al. (114) | Nature-inspired swarm approach, TDCN models | APTOS 2019 | Cohen’s kappa: 96.7%; accuracy: 90.3%; AUC: 95.6% |
2022 | Sethuraman and Palakuzhiyil Gopi (115) | Supervised CNN, Staircase-Net with series of transformations | DRIVE | Drive: accuracy: 97.76%; sensitivity: 94.83%; specificity: 98.71% |
2024 | Sanjeewani et al. (116) | U-Net, GAN, RNN, SVM | DRIVE | Specificity: 98.38%; sensitivity: 74.36%; accuracy: 95.77%; F1-score: 79.31% |
2021 | Li et al. (117) | U-Net, Dense-Net, Dice loss function | DRIVE | Specificity: 98.96%; accuracy: 96.98%; sensitivity: 79.31% |
2020 | Gao et al. (118) | Multiscale vascular enhancement, filtering approach | STARE | Accuracy: 94.01%; sensitivity: 75.81%; specificity: 95.50% |
2020 | Chen et al. (119) | Semi-supervised learning, U-Net | DRIVE | Accuracy: 0.9631; AUC: 0.976 |
2019 | Xia et al. (105) | One-pass feed forward process, cascade classification framework | DRIVE, STARE, and CHASE-DB1 | Accuracy: 96.03%, 96.40%, 95.41% |
2018 | Fan et al. (106) | Hierarchical image matting model | CHASE-DB1, DRIVE, STARE | Accuracy: 95.7%, 96.0%, 95.1% |
2018 | Adal et al. (120) | Blobness technique (multi-scale measured), SVM | Rotterdam Eye Hospital | Sensitivity: 98% |
2016 | Paing et al. (55) | Artificial neural networks, and ROI iocalization | DIARETDB1 | Accuracy: 96% |
2015 | Guo et al. (100) | Wavelet transformation and discrete cosine transformation, multiclass discriminant analysis | Real-world dataset | Accuracy: 90.9% |
APTOS, Asia Pacific Tele-Ophthalmology Society; AUC, area under the curve; CHASE-DB1, child health and study England-database 1; CNN, convolutional neural network; DFM, decoder fusion module; DIARETDB, diabetic retinopathy database; DL, deep learning; DRIVE, digital retinal images for vessel extraction; GAN, generative adversarial network; HRF, high-resolution fundus; PCA, principal component analysis; R2-ATT, recurrent residual attention; RNN, recurrent neural network; ROI, region of interest; SFM, supervised fusion module; STARE, structured analysis of the retina; SVM, support vector machine; TDCN, tailored deep convnets; VGG, visual geometry group.
Evaluation metrics
For accurate detection of DR using retinal images captured by a fundus camera, needs few steps of preprocessing approaches before proceeding with image analysis techniques. There are several preprocessing techniques namely adaptive histogram equalization, contrast adjustment; average filtering, homomorphism, and median filtering are applied for fundus photographs in the benchmark datasets. After performing an algorithmic approach on retinal photographs, PSNR and mean square error (MSE) are applied to analyze the performance of the technique (122). In the area of healthcare and medicine, there are two classes of data used for experiment purposes and medical treatment; one is about data without disease, and the other about with disease. The authenticity level of the experiments regarding the treatment of patients is evaluated through sensitivity and specificity measures (123). In retinal image analysis, DR detection and classification are mostly done through digital fundus images, which are determined through the sensitivity and specificity of every image. The improvement of treatment is directly proportional to the values of specificity and sensitivity, if the values are higher, then it is considered as a better treatment approach. The true positive determines the lesion pixels and the true negative shows the non-lesion pixels in the digital fundus photographs. Similarly, false positives show non-lesion pixels that are captured in the wrong way by the designed technique and false negatives represent lesion pixels not captured through the technique. Table 8 represents the variety of performance evaluation metrics used in the literature and can also help the research experts regarding availability of formulas, terms of choice, and the importance of the various types of evaluation metrics. Figure 7 mentions the evaluation metrics and demonstrates the citations of the evaluation metrics in the entire study.
Table 8
Evaluation metrics of performance | Calculating formula |
---|---|
Accuracy (124) | |
Sensitivity (94) | |
Specificity (125) | |
Precision (126) | |
PSNR (127) | |
TPR (128) | |
FPR (120) | |
AUC (129) | |
SSI (102) | |
F1-score (130) | |
PPV (80) | |
E (131) | |
A (131) | |
MCC (103) | |
G-means (103) | |
Overlap score (132) | |
EPI (102) | |
KC (133) | |
CoC (102) |
A, balanced accuracy; AUC, area under the curve; CoC, correlation coefficient; DR, diabetic retinopathy; E, overlapping error; EPI, edge preservation index; FN, false negative; FP, false positive; FPR, false positive rate; KC, kappa score; MCC, Mathews correlation coefficient; MSE, mean square error; PPV, positive predictive value; PSNR, peak signal-to-noise ratio; SSI, structural similarity index; TN, true negative; TP, true positive; TPR, true positive rate.
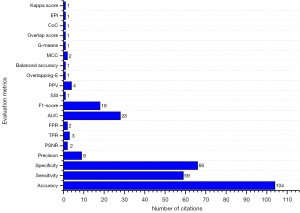
DR with XAI
In the recent development of artificial intelligence-based systems, XAI has also become an evolving technology (134). XAI is an advanced feature, which refers to the development and implementation of AI-based systems that helps to provide transparent and understandable explanations for their decisions and actions. While traditional AI models, such as DL neural networks, can be highly accurate in their predictions, they often function as black boxes, making it challenging to understand the underlying factors that contribute to their outputs. XAI aims to address this “black box” problem by incorporating transparency and interpretability into understandable AI models (134).
XAI enables the trust of users, regulators, and developers by providing transparency in the decision-making procedure of AI systems. The researchers have introduced different kinds of XAI tools, which are supportive and applicable in various kinds of domains to make the AI system understandable and transparent (134). Some methods involve designing models that are inherently interpretable, such as decision trees or rule-based systems. Other approaches focus on creating post-hoc explanations by analyzing and visualizing the internal workings of complex AI models. This could involve techniques like feature importance analysis, attention mechanisms, or generating textual or visual explanations. XAI has gained importance due to ethical, legal, and regulatory considerations. It helps ensure accountability, fairness, and trustworthiness in AI systems. Additionally, XAI has practical applications in various domains, including healthcare, finance, autonomous vehicles, and criminal justice, where clear and interpretable explanations are crucial for decision-making and risk assessment. However, it’s important to note that achieving full transparency and interpretability in AI models can be challenging, especially in complex DL architectures and XAI-based systems should be equipped with the advanced level of resources to compete with the challenges of black-box AI to make it more trustable and transparent towards decisions and conclusions (135).
Recently, ML-based predictions faced different kinds of scepticism, particularly in the area of healthcare for early prediction of serious disease and generated lot of discussion about explainable ML-based predictions (135,136). XAI can also play a significant role in the diagnosis and treatment of DR, a common complication of diabetes that affects the eyes (134). The graphical representation of XAI for DR diagnosis is presented in Figure 8.
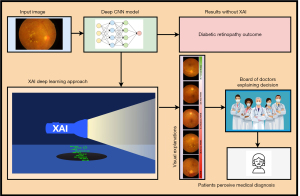
There are a several approaches of XAI (137) which can be applied in the context of DR, such as “decision support” which supports the clinicians and patients to get answer their questions like why a certain diagnosis was made? Moreover, “feature support” is also another way, which can help the researchers to know which retinal lesion is mostly involved in the current stage of DR and this transparency and information may improve the medical treatment and give clear directions for future research efforts. The “visual explanations” is an effective approach of XAI to generate visual details by offering highly intuitive understanding of DR diagnosis through implementation of heat maps instead of complex mathematical models on retinal images to make it easier for clinical inspection and validation. XAI also provides informative approach related with “error analysis” in the case of failure or incorrect predictions of AI based models for DR diagnosis. The information can be applied to refine the AI models to reduce the potential risks. The XAI approach namely “regulatory compliance” is also becoming more important for regulatory bodies and ethics committees in healthcare related applications, which may need AI-based systems having transparency and explanations in their decisions before clinical use.
XAI can enhance the understanding, trust, and adoption of AI systems in diagnosing and managing DR (138). By providing explanations for AI predictions and highlighting relevant features, XAI empowers clinicians, researchers, and patients to make informed decisions and contribute to improved patient outcomes in the field of ophthalmology.
Clinical implications for AI-based diagnosis of DR
In the field of DR screening, clinical implications are significant for early diagnosis, cost-effective, and better management of DR to prevent the vision loss. In the development of AI-based models, overall stakeholders must know the requirements of the clinical practice to address the challenges of healthcare delivery. Secondly, there is also important to have a proper validation process for AI-based models through a board of clinical and AI experts to check the biasness of datasets, whether it is complex, sufficient, and heterogeneous according to the specification of the institute (139). The AI-based systems should be monitored to carry out the reliability and safety by clinical decision-making, the trade-offs between sensitivity (reducing false negatives) and specificity (reducing false positives), and the ethical considerations of relying on automated systems in healthcare. Thirdly, the AI-based models must be carefully tested before deployment at the treatment point for safety and competency, similar to the medical devices, medications, and other relevant procedures. In the case of adopting, deciding on, and evaluating of the AI-based systems, the hospitals and healthcare delivery boards will need to brief the post-development technical issues, organizational governance, and clinical concerns for effective implementation (140). Furthermore, the administrative and clinical leadership of healthcare models along with participation of all the concerned stakeholders, including general public and patients must explain the future essentials to improve the clinical outcomes. In this way, AI-based systems can influence a positive change in the domain of healthcare through clearly define these objective states.
Discussion
The detailed research studies demonstrate the application of various computational approaches namely ML, DL, and image analysis for the diagnosis of DR using multimodal imaging, mostly including fundus and OCT images. The proper way of DR lesion segmentation including microaneurysms, hemorrhages, and exudates was carried out through automatic diagnosis systems. The efficiency of AI-based systems for DR detection depends on feature segmentation from retinal images related to DR, but the process is prone to error and also computationally expensive and the consistency of the whole system moves down by implementing this step. Furthermore, in most cases of literature work, without quantitative measurements, traditional image processing and ML techniques have been applied based on large-scale datasets. In the case of DR diagnosis, several detection techniques have been presented to discriminate the normal and DR real-time grading levels are rare for DR classification with five types of different classes. In recent work, many research experts made contributions to the assessment of DR classification into five categories but some of them worked to analyze and observe the retinal abnormalities based on existing domain expert knowledge. In the context of DR grading, many ML-based approaches have been reported instead of focusing on the whole features of DR. Moreover, it is noted that the outdated hardware was also an obstacle to the development of a diagnostic system of DR with DL approaches. However, nowadays the capacity of hardware has been increased and can now able to achieve outstanding outcomes through the combination of advanced DL techniques including meta-learning, multitask learning, incremental learning and domain adaptation to detect the retinal abnormalities including DR.
In the future, the overall development of DR detection and classification can be improved through advanced DR diagnostic approaches with the following suggestions:
- The input data for computer-aided diagnosis systems should contain high-resolution images and also be gathered from different kinds of regions and societies on a large scale.
- The dynamic features fusion could be applied through hand-crafted and automated features to obtain better classification performance, specifically in the case of severe DR levels.
- Implement several novel color space and appearance techniques for better performance of classification in the situation of complex patterns.
- The database of four levels of DR could be replaced with five levels.
- The examination of DME is also very essential to care about eye vision because DME is also a common reason of complete vision loss in diabetic patients.
- It is recommended that DME diagnosis should be performed using retinal imaging multimodalities including OCT and fundus scans instead of a single modality.
- It is suggested to design a meta-learning-based framework to facilitate ophthalmologist having less amount of dataset, which would also present more generalization and accuracy.
- It is suggested to combine the strength of ophthalmologists and AI for DR diagnosis.
- It is suggested to incorporate XAI-based approaches to overcome the potential challenges of DR misdiagnosis and also recommended to have human-in-the-loop (HITL) in AI-based decisions.
Recently, researchers introduced medical features-based extraction parameters for retinal abnormalities detection namely Diabetes Retinopathy Risk Index (DRRI) and Standards for Reporting Diagnostic accuracy studies (STARD). These parameters are based on the numerical value of different levels of thresholds to identify the stages of retinal disease. Moreover, these are also useful for the classification of DR through quantitative measurements (141). The review article provides a detailed review of the relevant studies in the area of DR, which completed more than a past decade. Figure 9 presents the major contributions of the researchers performed in the last decade using AI models for the progress of DR diagnosis with multimodal imaging (142-151).

AI with DL techniques, presented a significant role to achieve the excellent performance in medical image analysis, especially in DR screening using digital retinal images (152). However, still there is a need to discuss more about the important issues and basic development of DL. In the area of computer vision, advanced AI-based applications have been developed to solve the complex problems with new directions using DL. Nevertheless, it is necessary to know about the background knowledge of DL before going to implement it, such as amount of pooling and Convolutional levels, and amount of nodes on each level to get required outcome, and about the selection of model. The DL-based approaches perform the wonderful task for the training of a large scale of datasets. Generally, noted about the low quantity of images may confine and affect training task and learning procedure DL. In the recent studies, the two kinds of suggestions were presented to increase the scale of training data, where one to focus on data augmentation approaches to make the dataset more generic and the other is about to data gathering on the basis of weakly supervised learning approaches. In the area of medical image analysis, it is a significant task to overcome the demand of well annotated and large-scale of training data. To address this problem, research experts have introduced different kinds of modern DL techniques namely, meta-learning (153,154), incremental learning (155), multitask learning (156), and domain adaptation (157). In the same way, numerous research experts focused and divert their attention towards the utilization of such advanced techniques for the screening and grading of the retinal pathologies (158). Particularly, He et al. (159) worked in the context of unsupervised domain adaptation using adversarial network which is an appreciable task to segment the structure of retinal image using OCT scans, which are basically attained by Spectralis and Cirrus machines.
Comparative performance analysis
Numerous experts used traditional DL models with one fundus or OCT imaging modality at a time, which needs a large amount of data and also requires high computational power for training the dataset, furthermore, feature extraction through a single retinal modality could be less helpful to achieve better prediction results. To overcome this gap of research work and deficiencies, there could be a research direction using meta-learning based on clinically significant DR diagnosis from fused retinal imaging multimodalities including fundus and OCT imagery; would have tremendous performance, because, in the field of medical imaging, meta-learning is very useful and beneficial, containing unique features including few-shot learning (160) which can learn to generalize to new tasks using only a few annotated examples. The basic reason for the selection of meta-learning (161) in DR diagnosis is to improve the performance and efficiency of retinal imaging multimodality analysis, especially when faced with limited annotated data and computational power, but high levels of variability and uncertainty. The comparative analysis of the traditional and advanced AI-based techniques is explained in the following:
- The applications of DL require common weights for DNNs to handle their choices, which were not available in the existing models.
- The more generalized models are achieved through the integration of non-hand-crafted and handcrafted features.
- Implementation of the feature learning through layers technique as every level of layered neuron can be trained from previous layered attributes.
- In the case of DL architectures, the generalization feature can be enhanced by increasing the size with the addition of levels and units in each layer. For example, a DL model such as GoogLeNet has 22 layers.
The DR diagnosis with a comparison of several traditional and advanced AI with DL methods has been presented in Table 9, which shows the performance results for the identification of DR lesions as microaneurysms, hemorrhage, and exudates, where advanced AI-based techniques performed better than the traditional handcrafted techniques.
Table 9
Methods | Literature | Abnormalities | Performance (accuracy) (%) |
---|---|---|---|
Meta-learning | |||
Traditional | Morphological operations (56) | Retinal micro-aneurysms | 97.75 |
Advanced | DRNet (162) | 98.18 | |
Domain adaptation | |||
Traditional | RFC (38) | Retinal hemorrhages | 93.58 |
Advanced | Multi-instance learning (163) | 95.8 | |
Multi-tasking | |||
Traditional | Fuzzy techniques (164) | Retinal exudates | 93 |
Advanced | Modified U-Net multi-tasking (165) | 99.42 |
AI, artificial intelligence; RFC, random forest classifier.
In the case of retinal abnormality detection and treatment, retinal image registration process performs a vital role. In this context, there are few advanced methodologies are introduced including novel 3D (design, detection, deformation) registration approach for OCT images to deal with serious pathological subjects (166). Furthermore, transformation and feature based image registration approaches have been reported (167). Moreover, multimodal imaging registration approaches (168) contains multi-color scanning laser (MCSL) and ICGA have been introduced with coarse and fine registration stages. Model agnostic meta-learning technique (169) has been utilized by Hasan et al. (153) for the registration of multivendor retinal photographs through refinement a transformation matrix in an adversarial manner using spatial transformer. An automatic fast-focusing approach through fringes magnitudes of retinal layers introduced by Liu et al. (170) to identify the appropriate position of optical lens without any assistance of OCT and fundus imaging. Recently, you only look once (Yolo)also performed well and provided outstanding results in the case of DR detection using fundus images (171). Wang et al. (172) introduced a novel approach for speckle noise de-noising using OCT scans to enhance the performance of model to capture sparse and complex features. Furthermore, Wang et al. (173) highlighted the challenges of drusen segmentation for the identification of AMD in OCT images. The authors designed a novel U-shaped architecture-based framework contains multi-scale transformer module to capture non-local features. Moreover, Ju et al. (174) used adversarial domain adaptation for the achievement of cross-domain shifts between ultra-wide-field and normal fundus images for the analysis of glaucoma, AMD and DR. Meng et al. (158) proposed ADINet model known as attribute-driven incremental network for learning retinal abnormalities detection tasks using fundus images. An advanced DL-based model is introduced by Peng et al. (175) for the detection of Retinopathy of Prematurity (RoP). The presented work is based on five levels of RoP staging handled through EfficientNetB2, DenseNet121, and ResNet18 frameworks for feature extraction.
Future directions and challenges
Currently, AI and image processing have performed an imperative role in DR diagnostic systems to diagnose retinal abnormalities in DR. Several optimal solutions can help for the smart utilization of DL techniques with a small amount of annotated data. The recent studies presented CNN-based models for the development of deep multi-layer frameworks designed for DR detection through retinal fundus images. In contrast, retinal experts and ophthalmologists need to perform retinal image analysis and annotation which is quite expensive as well as a time-consuming task. For that reason, there is a need for effective and advanced deep-learning techniques that can perform well with a limited amount of annotated data. Furthermore, the traditional DL models can be performed well by applying a fusion of dynamic-sized DL-based models sequentially. For this reason, it can overcome the large training requirement and decrease the computational cost in the case of individual DL-based models to better complete the task independently. Moreover, advanced DL approaches including, meta-learning, multitask learning, incremental learning, and domain adaptation can perform better in the case of model generalization with less amount of annotated data. In real-world clinical settings, AI serves as a tool of assistance that reduces the manual workload but cannot fully ignore and eliminate it. Data preprocessing is significant to make sure that the inputs are accurate, whereas, the post-processing validation is useful to confirm that the AI-based outcomes are clinically applicable. In this way, the combination of human expertise and automation, commonly known as HITL in AI, is important for high-standard clinical workflows and for maintaining healthcare. There are still two important challenges regarding poor generalization and lack of reproducibility, which are affecting publications in the domain of AI. To address these serious problems, Barberis et al. (176) designed a framework for generalized analysis of software implementation through resampling of training and testing data, to ensure the reproducibility and robustness of AI models. Gundersen and Kjensmo (177) focused on the importance of reproducibility in AI models and discussed three degrees of reproducibility proposed by Goodman et al. (178) are methods, results, and inferential reproducibility. The methods reproducibility is referred to the implementation ability towards the computational and experimental tasks through the same tools and set of data to achieve the same results. The second degree of reproducibility is focused on the results reproducibility to verify the results on the new study by using the same computational and experimental approaches. The third degree of reproducibility is about inferential highlight the similar conclusions on the reanalysis of the novel work or through the independent study with replication. It is suggested that to enhance the transparency in the AI models, it is desirable from the regulator authority using publicly available datasets. Moreover, it is essential to test the medical devices through as much populations and cultural contexts as possible (179).
Conclusions
In the early diagnosis of DR, AI-based approaches play a vital role in the use of retinal imaging. This comprehensive review reported the relevant studies obtained from prestigious and popular repositories of Web of Science, Google Scholar, and PubMed to provide rich information for researchers. The review covered the related private and publicly available datasets with accessibility details, challenges, and suggestions to overcome the biases in the datasets and presented the AI-based DR lesions identification approaches for evaluating retinal abnormality in diabetic patients. Moreover, evaluation metrics are presented with descriptions and formulas for particular tasks of DR detection, classification, and segmentation. In ML-based approaches, statistical-based features including structure and shape provide better performance and in the case of DL models, CNNs are considered as a primary component for automatically better performing the extraction and categorization of DR images. In the context of performance evaluation metrics, area under the curve, specificity, sensitivity, and accuracy are mostly used in DR diagnosis. The critical analysis presents a deep summary of the DR-related topics, which would be helpful and assisting the research experts and clinical professionals working in this domain. Advanced AI-based concepts including, yolo, vision transformers, XAI, meta-learning, incremental learning, multitask learning, and domain adaptation could be used for the early and accurate detection of DR and also useful to overcome the limitations on areas such as integrating domain knowledge, using multimodal data, or employing transfer learning for resource-constrained settings. AI-based health caring techniques with specified clinical implications can minimize the time to identify the DR and can reduce the cost of DR diagnosis to facilitate the patients and ophthalmologists. The authors are confident that a rich informative review can facilitate medical practitioners and scientists towards the automated DR diagnosis. The readers of this review study would get the required information and achieve future research directions; to further improve research work and progress of DR diagnosis and treatment.
Acknowledgments
We extend our gratitude to the clinical experts from the hospitals for their assistance in private data annotation.
Footnote
Reporting Checklist: The authors have completed the Narrative Review reporting checklist. Available at https://qims.amegroups.com/article/view/10.21037/qims-24-1791/rc
Funding: This study was funded by
Conflicts of Interest: All authors have completed the ICMJE uniform disclosure form (available at https://qims.amegroups.com/article/view/10.21037/qims-24-1791/coif). The authors have no conflicts of interest to declare.
Ethical Statement: The authors are accountable for all aspects of the work in ensuring that questions related to the accuracy or integrity of any part of the work are appropriately investigated and resolved.
Open Access Statement: This is an Open Access article distributed in accordance with the Creative Commons Attribution-NonCommercial-NoDerivs 4.0 International License (CC BY-NC-ND 4.0), which permits the non-commercial replication and distribution of the article with the strict proviso that no changes or edits are made and the original work is properly cited (including links to both the formal publication through the relevant DOI and the license). See: https://creativecommons.org/licenses/by-nc-nd/4.0/.
References
- World Health Organization. Diabetic retinopathy screening: a short guide: increase effectiveness, maximize benefits and minimize harm. 2020. Available online: https://iris.who.int/bitstream/handle/10665/336660/9789289055321-eng.pdf
- Sawhney R, Malik A, Sharma S, Narayan V. A comparative assessment of artificial intelligence models used for early prediction and evaluation of chronic kidney disease. Decision Analytics Journal 2023;6:100169. [Crossref]
- World Health Organization. World report on vision. 2019. Available online: https://iris.who.int/bitstream/handle/10665/328717/9789240017184-ara.pdf
- Mushtaq G, Siddiqui F. Detection of diabetic retinopathy using deep learning methodology. In: IOP Conference Series: Materials sScience and Engineering. IOP Publishing; 2021;1070:012049.
- Taylor R, Batey D. Handbook of retinal screening in diabetes: diagnosis and management. 2nd ed. Oxford: John Wiley & Sons; 2012.
- Gupta A, Chhikara R. Diabetic retinopathy: Present and past. Procedia Comput Sci 2018;132:1432-40. [Crossref]
- Lin J, Yu L, Weng Q, Zheng X. Retinal image quality assessment for diabetic retinopathy screening: A survey. Multimed Tools Appl 2020;79:16173-99. [Crossref]
- Mateen M, Wen J. Nasrullah, Song S, Huang Z. Fundus image classification using VGG-19 architecture with PCA and SVD. Symmetry 2018;11:1. [Crossref]
- Li X, Li X. The Antidepressant Effect of Light Therapy from Retinal Projections. Neurosci Bull 2018;34:359-68. [Crossref] [PubMed]
- Aiello LP. The potential role of PKC beta in diabetic retinopathy and macular edema. Surv Ophthalmol 2002;47:S263-9. [Crossref] [PubMed]
- Jagan Mohan N, Murugan R, Goel T. Deep learning for diabetic retinopathy detection: Challenges and opportunities. In: Tripathy BK, Lingras P, Kar AK, Chowdhary CL. editors. Next Generation Healthcare Informatics. Springer; 2022:213-32.
- Krause J, Gulshan V, Rahimy E, et al. Grader Variability and the Importance of Reference Standards for Evaluating Machine Learning Models for Diabetic Retinopathy. Ophthalmology 2018;125:1264-72. [Crossref] [PubMed]
- Porwal P, Pachade S, Kokare M, et al. IDRiD: Diabetic Retinopathy - Segmentation and Grading Challenge. Med Image Anal 2020;59:101561. [Crossref] [PubMed]
- Umirzakova S, Ahmad S, Khan LU, Whangbo T. Medical image super-resolution for smart healthcare applications: A comprehensive survey. Information Fusion 2024;103:102075. [Crossref]
- Alnaggar OAMF, Jagadale BN, Saif MAN, Ghaleb OAM, Ahmed AAQ, Aqlan HAA, Esmail Al-Ariki HDE. Efficient artificial intelligence approaches for medical image processing in healthcare: comprehensive review, taxonomy, and analysis. Artificial Intelligence Review 2024;57:221. [Crossref]
- Balyen L, Peto T. Promising Artificial Intelligence-Machine Learning-Deep Learning Algorithms in Ophthalmology. Asia Pac J Ophthalmol (Phila) 2019;8:264-72. [PubMed]
- Senapati A, Tripathy HK, Sharma V, Gandomi AH. Artificial intelligence for diabetic retinopathy detection: a systematic review. Inform Med Unlocked 2024;45:101445. [Crossref]
- Kumar R, Pal R. India achieves WHO recommended doctor population ratio: A call for paradigm shift in public health discourse! J Family Med Prim Care 2018;7:841-4. [Crossref] [PubMed]
- Scotland GS, McNamee P, Fleming AD, et al. Costs and consequences of automated algorithms versus manual grading for the detection of referable diabetic retinopathy. Br J Ophthalmol 2010;94:712-9. [Crossref] [PubMed]
- Kumar G, Chatterjee S, Chattopadhyay C. DRISTI: a hybrid deep neural network for diabetic retinopathy diagnosis. Signal Image Video Process 2021;15:1679-86. [Crossref] [PubMed]
- Schmidt-Erfurth U, Sadeghipour A, Gerendas BS, et al. Artificial intelligence in retina. Prog Retin Eye Res 2018;67:1-29. [Crossref] [PubMed]
- Balogh EP, Miller BT, Ball JR. editors. Improving diagnosis in health care. Washington: The National Academies Press; 2015.
- Teo ZL, Tham YC, Yu M, et al. Global Prevalence of Diabetic Retinopathy and Projection of Burden through 2045: Systematic Review and Meta-analysis. Ophthalmology 2021;128:1580-91. [Crossref] [PubMed]
- IBM. What is a data set? Available online: https://www.ibm.com/support/knowledgecenter/zosbasics/com.ibm.zos.zconcepts/zconc_datasetintro.htm
- Kang EY, Yeung L, Lee YL, et al. A Multimodal Imaging-Based Deep Learning Model for Detecting Treatment-Requiring Retinal Vascular Diseases: Model Development and Validation Study. JMIR Med Inform 2021;9:e28868. [Crossref] [PubMed]
- An G, Omodaka K, Hashimoto K, et al. Glaucoma Diagnosis with Machine Learning Based on Optical Coherence Tomography and Color Fundus Images. J Healthc Eng 2019;2019:4061313. [Crossref] [PubMed]
- Vaghefi E, Hill S, Kersten HM, et al. Multimodal Retinal Image Analysis via Deep Learning for the Diagnosis of Intermediate Dry Age-Related Macular Degeneration: A Feasibility Study. J Ophthalmol 2020;2020:7493419. [Crossref] [PubMed]
- APTOS Ophthalmology Society Big Data Competition 2023. Available online: https://tianchialiyuncom/competition/entrance/532160.
- UWF4DR2024 Challenge public dataset. Available online: https://codalablisnupsaclayfr/competitions/18605.
- Ma F, Liu X, Wang S, et al. CSANet: a lightweight channel and spatial attention neural network for grading diabetic retinopathy with optical coherence tomography angiography. Quant Imaging Med Surg 2024;14:1820-34. [Crossref] [PubMed]
- Delgado-Tirado S, Gonzalez-Buendia L, Kim LA. Indocyanine Green Angiography. In: Albert and Jakobiec's Principles and Practice of Ophthalmology. Cham: Springer International Publishing; 2022:2683-711.
- Webb RH, Hughes GW. Scanning laser ophthalmoscope. IEEE Trans Biomed Eng 1981;28:488-92. [Crossref] [PubMed]
- Khan SM, Liu X, Nath S, et al. A global review of publicly available datasets for ophthalmological imaging: barriers to access, usability, and generalisability. Lancet Digit Health 2021;3:e51-66. [Crossref] [PubMed]
- Chalakkal RJ, Abdulla W. Automatic segmentation of retinal vasculature. In: 2017 IEEE International Conference on Acoustics, Speech and Signal Processing (ICASSP). IEEE; 2017:886-90.
- Xu X, Ding W, Wang X, et al. Smartphone-Based Accurate Analysis of Retinal Vasculature towards Point-of-Care Diagnostics. Sci Rep 2016;6:34603. [Crossref] [PubMed]
- Melo T, Mendonça AM, Campilho A. Microaneurysm detection in color eye fundus images for diabetic retinopathy screening. Comput Biol Med 2020;126:103995. [Crossref] [PubMed]
- Lam C, Yu C, Huang L, et al. Retinal Lesion Detection With Deep Learning Using Image Patches. Invest Ophthalmol Vis Sci 2018;59:590-6. [Crossref] [PubMed]
- Chowdhury AR, Chatterjee T, Banerjee S. A Random Forest classifier-based approach in the detection of abnormalities in the retina. Med Biol Eng Comput 2019;57:193-203. [Crossref] [PubMed]
- Orlando JI, Prokofyeva E, Del Fresno M, et al. An ensemble deep learning based approach for red lesion detection in fundus images. Comput Methods Programs Biomed 2018;153:115-27. [Crossref] [PubMed]
- Cao W, Czarnek N, Shan J, et al. Microaneurysm Detection Using Principal Component Analysis and Machine Learning Methods. IEEE Trans Nanobioscience 2018;17:191-8. [Crossref] [PubMed]
- Jayachandran A, Ratheesh Kumar S. Microaneurysm classification system in color fundus images using auto-weight dilated convolutional network. Int J Imaging Syst Technol 2024;34:e22950. [Crossref]
- Alotaibi NS. Micro aneurysm detection using optimized residual-based temporal attention Convolutional Neural Network with Inception-V3 transfer learning. Microsc Res Tech 2024;87:908-21. [Crossref] [PubMed]
- Steffi S, Sam Emmanuel WR. Resilient back-propagation machine learning-based classification on fundus images for retinal microaneurysm detection. Int Ophthalmol 2024;44:91. [Crossref] [PubMed]
- Alam M, Zhao EJ, Lam CK, et al. Segmentation-Assisted Fully Convolutional Neural Network Enhances Deep Learning Performance to Identify Proliferative Diabetic Retinopathy. J Clin Med 2023;12:385. [Crossref] [PubMed]
- Nunez do Rio JM, Nderitu P, Raman R, et al. Using deep learning to detect diabetic retinopathy on handheld non-mydriatic retinal images acquired by field workers in community settings. Sci Rep 2023;13:1392. [Crossref] [PubMed]
- Du J, Zou B, Ouyang P, Zhao R. Retinal microaneurysm detection based on transformation splicing and multi-context ensemble learning. Biomed Signal Process Control 2022;74:103536. [Crossref]
- Hervella Á S, Rouco J, Novo J, Ortega M. Retinal microaneurysms detection using adversarial pre-training with unlabeled multimodal images. Information Fusion 2022;79:146-61. [Crossref]
- Deng J, Tang P, Zhao X, et al. Local Structure Awareness-Based Retinal Microaneurysm Detection with Multi-Feature Combination. Biomedicines 2022;10:124. [Crossref] [PubMed]
- Ayala A, Ortiz Figueroa T, Fernandes B, Cruz F. Diabetic retinopathy improved detection using deep learning. Applied Sciences 2021;11:11970. [Crossref]
- Zhang X, Wu J, Meng M, Sun Y, Sun W. Feature-transfer network and local background suppression for microaneurysm detection. Mach Vis Appl 2021;32:1-13. [Crossref]
- Dai L, Wu L, Li H, et al. A deep learning system for detecting diabetic retinopathy across the disease spectrum. Nat Commun 2021;12:3242. [Crossref] [PubMed]
- Deepa R, Narayanan NK. Detection of microaneurysm in retina image using machine learning approach. In: 2020 International Conference on Innovative Trends in Information Technology (ICITIIT). IEEE; 2020.
- Du J, Zou B, Chen C, et al. Automatic microaneurysm detection in fundus image based on local cross-section transformation and multi-feature fusion. Comput Methods Programs Biomed 2020;196:105687. [Crossref] [PubMed]
- Gondal WM, Köhler JM, Grzeszick R, Fink GA, Hirsch M. Weakly-supervised localization of diabetic retinopathy lesions in retinal fundus images. In: 2017 IEEE International Conference on Image Processing (ICIP). IEEE; 2017:2069-73.
- Paing MP, Choomchuay S, Yodprom MDR. Detection of lesions and classification of diabetic retinopathy using fundus images. In: 2016 9th Biomedical Engineering International Conference (BMEiCON). IEEE; 2016.
- Prasad DK, Vibha L, Venugopal KR. Early detection of diabetic retinopathy from digital retinal fundus images. In: 2015 IEEE Recent Advances in Intelligent Computational Systems (RAICS). IEEE; 2015:240-5.
- Pereira J, Colomer A, Naranjo V. Comparison of local analysis strategies for exudate detection in fundus images. In: Intelligent Data Engineering and Automated Learning-IDEAL 2018: 19th International Conference, Madrid, Spain, November 21-23, 2018, Proceedings, Part I 19. Springer International Publishing; 2018:174-83.
- Khojasteh P, Passos Júnior LA, Carvalho T, et al. Exudate detection in fundus images using deeply-learnable features. Comput Biol Med 2019;104:62-9. [Crossref] [PubMed]
- Kaur J, Mittal D. A generalized method for the segmentation of exudates from pathological retinal fundus images. Biocybernetics and Biomedical Engineering 2018;38:27-53. [Crossref]
- Prentašić P, Lončarić S. Detection of exudates in fundus photographs using deep neural networks and anatomical landmark detection fusion. Comput Methods Programs Biomed 2016;137:281-92. [Crossref] [PubMed]
- Huang H, Zhu L, Zhu W, et al. Algorithm for Detection and Quantification of Hyperreflective Dots on Optical Coherence Tomography in Diabetic Macular Edema. Front Med (Lausanne) 2021;8:688986. [Crossref] [PubMed]
- Liu R, Li Q, Xu F, et al. Application of artificial intelligence-based dual-modality analysis combining fundus photography and optical coherence tomography in diabetic retinopathy screening in a community hospital. Biomed Eng Online 2022;21:47. [Crossref] [PubMed]
- Van Do Q, Hoang HT, Van Vu N, Andrade De Jesus D, Sanchez Brea L, Nguyen HX, Nguyen ATL, Le TN, Dinh DTM, Nguyen MTB, Nguyen HC, Bui ATV, Le HV, Gillen K, Vu TT, Luu HM. Segmentation of hard exudate lesions in color fundus image using two-stage CNN-based methods. Expert Syst Appl 2024;241:122742. [Crossref]
- Guo T, Yang J, Yu Q. Diabetic retinopathy lesion segmentation using deep multi-scale framework. Biomed Signal Process Control 2024;88:105050. [Crossref]
- Maiti S, Maji D, Dhara AK, Sarkar G. An attention enriched encoder-decoder architecture with CLSTM and RES unit for segmenting exudate in retinal images. Signal Image Video Process 2024;18:3329-39. [Crossref]
- Farahat Z, Zrira N, Souissi N, et al. Application of Deep Learning Methods in a Moroccan Ophthalmic Center: Analysis and Discussion. Diagnostics (Basel) 2023;13:1694. [Crossref] [PubMed]
- Naik S, Kamidi D, Govathoti S, Cheruku R, Mallikarjuna Reddy A. Efficient diabetic retinopathy detection using convolutional neural network and data augmentation. Soft Computing 2024;28:617. [Crossref]
- Tang W, Cui K, Chan RH. A global and patch-wise contrastive loss for accurate automated exudate detection. arXiv:2302.11517 [Preprint]. 2023. Available online: https://arxiv.org/abs/2302.11517
- Reddy VPC, Gurrala KK. Joint DR-DME classification using deep learning-CNN based modified grey-wolf optimizer with variable weights. Biomed Signal Process Control 2022;73:103439. [Crossref]
- Hussain M, Al-Aqrabi H, Munawar M, Hill R, Parkinson S. Exudate regeneration for automated exudate detection in retinal fundus images. IEEE Access 2022;11:83934-45.
- Sangeethaa SN, Jothimani S. Detection of exudates from clinical fundus images using machine learning algorithms in diabetic maculopathy. Int J Diabetes Dev Ctries 2023;43:25-35. [Crossref]
- Sudha V, Ganeshbabu TR. A Convolutional Neural Network Classifier VGG-19 Architecture for Lesion Detection and Grading in Diabetic Retinopathy Based on Deep Learning. Computers, Materials & Continua 2021;66:827-42. [Crossref]
- Cincan RG, Popescu D, Ichim L. Exudate Detection in Diabetic Retinopathy Using Deep Learning Techniques. In: 2021 25th International Conference on System Theory, Control and Computing (ICSTCC). IEEE; 2021:473-7.
- Kurilová V, Goga J, Oravec M, et al. Support vector machine and deep-learning object detection for localisation of hard exudates. Sci Rep 2021;11:16045. [Crossref] [PubMed]
- Pan X, Jin K, Cao J, et al. Multi-label classification of retinal lesions in diabetic retinopathy for automatic analysis of fundus fluorescein angiography based on deep learning. Graefes Arch Clin Exp Ophthalmol 2020;258:779-85. [Crossref] [PubMed]
- Theera-Umpon N, Poonkasem I, Auephanwiriyakul S, Patikulsila D. Hard exudate detection in retinal fundus images using supervised learning. Neural Comput Appl 2020;32:13079-96. [Crossref]
- Thulkar D, Daruwala R, Sardar N. An integrated system for detection exudates and severity quantification for diabetic macular edema. J Med Biol Eng 2020;40:798-820. [Crossref]
- Prentašić P, Lončarić S. Detection of exudates in fundus photographs using convolutional neural networks. In: 2015 9th International Symposium on Image and Signal Processing and Analysis (ISPA). IEEE; 2015:188-92.
- Kaur N, Chatterjee S, Acharyya M, Kaur J, Kapoor N, Gupta S. A supervised approach for automated detection of hemorrhages in retinal fundus images. In: 2016 5th International Conference on Wireless Networks and Embedded Systems (WECON). IEEE; 2016.
- Wu J, Zhang S, Xiao Z, Zhang F, Geng L, Lou S, Liu M. Hemorrhage detection in fundus image based on 2D Gaussian fitting and human visual characteristics. Optics & Laser Technology 2019;110:69-77. [Crossref]
- Ayhan MS, Berens P. Test-time data augmentation for estimation of heteroscedastic aleatoric uncertainty in deep neural networks. In: Medical Imaging with Deep Learning. 2018.
- Zhao H, Li H, Maurer-Stroh S, et al. Supervised Segmentation of Un-Annotated Retinal Fundus Images by Synthesis. IEEE Trans Med Imaging 2019;38:46-56. [Crossref] [PubMed]
- van Grinsven MJ, van Ginneken B, Hoyng CB, et al. Fast Convolutional Neural Network Training Using Selective Data Sampling: Application to Hemorrhage Detection in Color Fundus Images. IEEE Trans Med Imaging 2016;35:1273-84. [Crossref] [PubMed]
- Nisha JU, Rufus HNA. Splat Feature Classification With Application to Retinal Hemorrhage Detection in Fundus Images. The International Journal of Science and Technoledge 2014;2:338.
- Maqsood S, Damaševičius R, Maskeliūnas R. Hemorrhage Detection Based on 3D CNN Deep Learning Framework and Feature Fusion for Evaluating Retinal Abnormality in Diabetic Patients. Sensors (Basel) 2021;21:3865. [Crossref] [PubMed]
- Sathiyaseelan R, Ranganathan K, Ramamoorthy R, et al. Haemorrhage diagnosis in colour fundus images using a fast-convolutional neural network based on a modified U-Net. Network 2025;36:198-219. [Crossref] [PubMed]
- Biju J, Shanthi D. Performance Analysis of Diabetic Retinopathy and Hemorrhages Segmentation System Using Enhanced Visual Geometry Group (EVGG) Architecture. Journal of Electrical Engineering & Technology 2024;19:3527-38. [Crossref]
- Atlas LG, Arjun KP, Kumar KS, Dhanaraj RK, Nayyar A. Deep learning enabled hemorrhage detection in retina with DPFE and splat segmentation in fundus images. Biomed Signal Process Control 2024;88:105692. [Crossref]
- Li AL, Feng M, Wang Z, et al. Automated Detection of Posterior Vitreous Detachment on OCT Using Computer Vision and Deep Learning Algorithms. Ophthalmol Sci 2023;3:100254. [Crossref] [PubMed]
- Alwakid G, Gouda W, Humayun M. Deep Learning-Based Prediction of Diabetic Retinopathy Using CLAHE and ESRGAN for Enhancement. Healthcare (Basel) 2023.
- Saranya P, Pranati R, Patro SS. Detection and classification of red lesions from retinal images for diabetic retinopathy detection using deep learning models. Multimed Tools Appl 2023;82:39327-47. [Crossref]
- Kiliçarslan S. A novel nonlinear hybrid HardSReLUE activation function in transfer learning architectures for hemorrhage classification. Multimed Tools Appl 2023;82:6345-65. [Crossref]
- Mondal SS, Mandal N, Singh KK, et al. EDLDR: An Ensemble Deep Learning Technique for Detection and Classification of Diabetic Retinopathy. Diagnostics (Basel) 2022;13:124. [Crossref] [PubMed]
- Xia H, Rao Z, Zhou Z. A multi-scale gated network for retinal hemorrhage detection. Applied Intelligence 2023;53:5259-73.
- Goel S, Gupta S, Panwar A, Kumar S, Verma M, Bourouis S, Ullah MA. Deep learning approach for stages of severity classification in diabetic retinopathy using color fundus retinal images. Math Probl Eng 2021;2021:7627566. [Crossref]
- Zhang X, Li F, Li D, et al. Automated detection of severe diabetic retinopathy using deep learning method. Graefes Arch Clin Exp Ophthalmol 2022;260:849-56. [Crossref] [PubMed]
- Hacisoftaoglu RE, Karakaya M, Sallam AB. Deep Learning Frameworks for Diabetic Retinopathy Detection with Smartphone-based Retinal Imaging Systems. Pattern Recognit Lett 2020;135:409-17. [Crossref] [PubMed]
- Li Z, Guo C, Nie D, et al. Development and Evaluation of a Deep Learning System for Screening Retinal Hemorrhage Based on Ultra-Widefield Fundus Images. Transl Vis Sci Technol 2020;9:3. [Crossref] [PubMed]
- Suriyal S, Druzgalski C, Gautam K. Mobile assisted diabetic retinopathy detection using deep neural network. In: 2018 Global Medical Engineering Physics Exchanges/Pan American Health Care Exchanges (GMEPE/PAHCE). IEEE; 2018.
- Guo L, Yang JJ, Peng L, Li J, Liang Q. A computer-aided healthcare system for cataract classification and grading based on fundus image analysis. Comput Ind 2015;69:72-80. [Crossref]
- BahadarKhan K. A Khaliq A, Shahid M. A Morphological Hessian Based Approach for Retinal Blood Vessels Segmentation and Denoising Using Region Based Otsu Thresholding. PLoS One 2016;11:e0158996. [Crossref] [PubMed]
- Sahu S, Singh AK, Ghrera SP, Elhoseny M. An approach for de-noising and contrast enhancement of retinal fundus image using CLAHE. Optics & Laser Technology 2019;110:87-98. [Crossref]
- Leopold HA, Orchard J, Zelek JS, et al. PixelBNN: Augmenting the PixelCNN with Batch Normalization and the Presentation of a Fast Architecture for Retinal Vessel Segmentation. J Imaging 2019;5:26. [Crossref] [PubMed]
- Mahapatra D, Bozorgtabar B, Garnavi R. Image super-resolution using progressive generative adversarial networks for medical image analysis. Comput Med Imaging Graph 2019;71:30-9. [Crossref] [PubMed]
- Wang X, Jiang X, Ren J. Blood vessel segmentation from fundus image by a cascade classification framework. Pattern Recognition 2019;88:331-41. [Crossref]
- Fan Z, Lu J, Wei C, et al. A Hierarchical Image Matting Model for Blood Vessel Segmentation in Fundus Images. IEEE Trans Image Process 2018; Epub ahead of print. [Crossref] [PubMed]
- Hossain NI, Reza S. Blood vessel detection from fundus image using Markov random field based image segmentation. In: 2017 4th International Conference on Advances in Electrical Engineering (ICAEE). IEEE; 2017:123-7.
- Feng S, Zhao H, Shi F, et al. CPFNet: Context Pyramid Fusion Network for Medical Image Segmentation. IEEE Trans Med Imaging 2020;39:3008-18. [Crossref] [PubMed]
- Xi X, Meng X, Qin Z, et al. IA-net: informative attention convolutional neural network for choroidal neovascularization segmentation in OCT images. Biomed Opt Express 2020;11:6122-36. [Crossref] [PubMed]
- Vij R, Arora S. A hybrid evolutionary weighted ensemble of deep transfer learning models for retinal vessel segmentation and diabetic retinopathy detection. Comput Electr Eng 2024;115:109107. [Crossref]
- Singh LK, Khanna M, Thawkar S, Singh R. Deep-learning based system for effective and automatic blood vessel segmentation from Retinal fundus images. Multimed Tools Appl 2024;83:6005-49. [Crossref]
- Ma Z, Li X. An improved supervised and attention mechanism-based U-Net algorithm for retinal vessel segmentation. Comput Biol Med 2024;168:107770. [Crossref] [PubMed]
- Kumar KS, Singh NP. Retinal disease prediction through blood vessel segmentation and classification using ensemble-based deep learning approaches. Neural Comput Appl 2023;35:12495-511. [Crossref]
- Bhardwaj P, Gupta P, Guhan T, et al. Early Diagnosis of Retinal Blood Vessel Damage via Deep Learning-Powered Collective Intelligence Models. Comput Math Methods Med 2022;2022:3571364. [Crossref] [PubMed]
- Sethuraman S, Palakuzhiyil Gopi V. Staircase-Net: a deep learning based architecture for retinal blood vessel segmentation. Sādhanā 2022;47:191. [Crossref]
- Sanjeewani, Yadav AK, Akbar M, Kumar M, Yadav D. Retinal blood vessel segmentation using a deep learning method based on modified U-NET model. Multimed Tools Appl 2024;83:82659-78. [Crossref]
- Li Z, Jia M, Yang X, et al. Blood Vessel Segmentation of Retinal Image Based on Dense-U-Net Network. Micromachines (Basel) 2021;12:1478. [Crossref] [PubMed]
- Gao J, Chen G, Lin W. An Effective Retinal Blood Vessel Segmentation by Using Automatic Random Walks Based on Centerline Extraction. Biomed Res Int 2020;2020:7352129. [Crossref] [PubMed]
- Chen D, Ao Y, Liu S. Semi-supervised learning method of U-net deep learning network for blood vessel segmentation in retinal images. Symmetry 2020;12:1067. [Crossref]
- Adal KM, van Etten PG, Martinez JP, et al. An Automated System for the Detection and Classification of Retinal Changes Due to Red Lesions in Longitudinal Fundus Images. IEEE Trans Biomed Eng 2018;65:1382-90. [Crossref] [PubMed]
- Singh LK, Khanna M, Thawkar S, Singh R. Deep-learning based system for effective and automatic blood vessel segmentation from Retinal fundus images. Multimed Tools Appl 2024;83:6005-49. [Crossref]
- Basha SM, Rajput DS. Survey on evaluating the performance of machine learning algorithms: Past contributions and future roadmap. In: Sangaiah AK. editor. Deep Learning and Parallel Computing Environment for Bioengineering Systems. Cambridge: Academic Press; 2019:153-64.
- Hicks SA, Strümke I, Thambawita V, et al. On evaluation metrics for medical applications of artificial intelligence. Sci Rep 2022;12:5979. [Crossref] [PubMed]
- Alwakid G, Gouda W, Humayun M. Deep Learning-Based Prediction of Diabetic Retinopathy Using CLAHE and ESRGAN for Enhancement. Healthcare (Basel) 2023;11:863. [Crossref] [PubMed]
- Zhou L, Zhao Y, Yang J, Yu Q, Xu X. Deep multiple instance learning for automatic detection of diabetic retinopathy in retinal images. IET Image Process 2018;12:563-71. [Crossref]
- Anene IL, Li Y. Segmentation of Retinal Blood Vessels Using Deep Learning. arXiv:2303.09679 [Preprint]. 2023. Available online: https://arxiv.org/abs/2303.09679
- Zhang S, Liang G, Pan S, Zheng L. A fast medical image super resolution method based on deep learning network. IEEE Access 2018;7:12319-27.
- Khunger M, Choudhury T, Satapathy SC, Ting KC. Automated detection of glaucoma using image processing techniques. In: Emerging Technologies in Data Mining and Information Security: Proceedings of IEMIS 2018, Volume 3. 2019:323-35.
- Bhardwaj P, Gupta P, Guhan T, et al. Early Diagnosis of Retinal Blood Vessel Damage via Deep Learning-Powered Collective Intelligence Models. Comput Math Methods Med 2022;2022:3571364. [Crossref] [PubMed]
- Maninis KK, Pont-Tuset J, Arbeláez P, Van Gool L. Deep retinal image understanding. In: Medical Image Computing and Computer-Assisted Intervention-MICCAI 2016: 19th International Conference, Athens, Greece, October 17-21, 2016, Proceedings, Part II 19. Springer International Publishing; 2016:140-8.
- Fu H, Cheng J, Xu Y, et al. Joint Optic Disc and Cup Segmentation Based on Multi-Label Deep Network and Polar Transformation. IEEE Trans Med Imaging 2018;37:1597-605. [Crossref] [PubMed]
- Bhat SH, Kumar P. Segmentation of optic disc by localized active contour model in retinal fundus image. In: Smart Innovations in Communication and Computational Sciences: Proceedings of ICSICCS-2018. Springer Singapore; 2019:35-44.
- Kwasigroch A, Jarzembinski B, Grochowski M. Deep CNN based decision support system for detection and assessing the stage of diabetic retinopathy. In: 2018 International Interdisciplinary PhD Workshop (IIPhDW). IEEE; 2018:111-6.
- Lalithadevi B, Krishnaveni S, Gnanadurai JSC. A Feasibility Study of Diabetic Retinopathy Detection in Type II Diabetic Patients Based on Explainable Artificial Intelligence. J Med Syst 2023;47:85. [Crossref] [PubMed]
- Hassija V, Chamola V, Mahapatra A, Singal A, Goel D, Huang K, Scardapane S, Spinelli I, Mahmud M, Hussain A. Interpreting black-box models: a review on explainable artificial intelligence. Cognitive Computation 2024;16:45-74. [Crossref]
- Rohmetra H, Raghunath N, Narang P, Chamola V, Guizani M, Lakkaniga NR. AI-enabled remote monitoring of vital signs for COVID-19: methods, prospects and challenges. Computing 2023;105:783-809.
- Chaddad A, Peng J, Xu J, et al. Survey of Explainable AI Techniques in Healthcare. Sensors (Basel) 2023;23:634. [Crossref] [PubMed]
- Quellec G, Al Hajj H, Lamard M, et al. ExplAIn: Explanatory artificial intelligence for diabetic retinopathy diagnosis. Med Image Anal 2021;72:102118. [Crossref] [PubMed]
- Gottesman O, Johansson F, Komorowski M, et al. Guidelines for reinforcement learning in healthcare. Nat Med 2019;25:16-8. [Crossref] [PubMed]
- Matheny M, Israni ST, Ahmed M, Whicher D. Artificial intelligence in health care: The hope, the hype, the promise, the peril. Washington, DC: National Academy of Medicine; 2019.
- Qureshi I, Ma J, Shaheed K. A hybrid proposed fundus image enhancement framework for diabetic retinopathy. Algorithms 2019;12:14. [Crossref]
- Welikala RA, Fraz MM, Dehmeshki J, et al. Genetic algorithm based feature selection combined with dual classification for the automated detection of proliferative diabetic retinopathy. Comput Med Imaging Graph 2015;43:64-77. [Crossref] [PubMed]
- Hwang TS, Gao SS, Liu L, et al. Automated Quantification of Capillary Nonperfusion Using Optical Coherence Tomography Angiography in Diabetic Retinopathy. JAMA Ophthalmol 2016;134:367-73. [Crossref] [PubMed]
- ElTanboly A, Ismail M, Shalaby A, et al. A computer-aided diagnostic system for detecting diabetic retinopathy in optical coherence tomography images. Med Phys 2017;44:914-23. [Crossref] [PubMed]
- Eladawi N, Elmogy M, Khalifa F, et al. Early diabetic retinopathy diagnosis based on local retinal blood vessel analysis in optical coherence tomography angiography (OCTA) images. Med Phys 2018;45:4582-99. [Crossref] [PubMed]
- Khansari MM, Zhang J, Qiao Y, et al. Automated Deformation-Based Analysis of 3D Optical Coherence Tomography in Diabetic Retinopathy. IEEE Trans Med Imaging 2020;39:236-45. [Crossref] [PubMed]
- Amato A, Nadin F, Borghesan F, et al. Widefield Optical Coherence Tomography Angiography in Diabetic Retinopathy. J Diabetes Res 2020;2020:8855709. [Crossref] [PubMed]
- Arrigo A, Teussink M, Aragona E, et al. MultiColor imaging to detect different subtypes of retinal microaneurysms in diabetic retinopathy. Eye (Lond) 2021;35:277-81. [Crossref] [PubMed]
- Hervella ÁS, Rouco J, Novo J, et al. Multimodal image encoding pre-training for diabetic retinopathy grading. Comput Biol Med 2022;143:105302. [Crossref] [PubMed]
- Rajesh AE, Davidson OQ, Lee CS, et al. Artificial Intelligence and Diabetic Retinopathy: AI Framework, Prospective Studies, Head-to-head Validation, and Cost-effectiveness. Diabetes Care 2023;46:1728-39. [Crossref] [PubMed]
- Huemer J, Heeren TF, Olvera-Barrios A, et al. Sight threatening diabetic retinopathy in patients with macular telangiectasia type 2. Int J Retina Vitreous 2024;10:28. [Crossref] [PubMed]
- Mateen M, Wen J, Hassan M, Nasrullah N, Sun S, Hayat S. Automatic detection of diabetic retinopathy: a review on datasets, methods and evaluation metrics. IEEE Access 2020;8:48784-811.
- Hasan A, Deng Z, Loo J, Mukherjee D, Duncan JL, Birch DG, Jaffe GJ, Farsiu S. Meta-learning approach to automatically register multivendor retinal images. Investigative Ophthalmology & Visual Science 2020;61:1634.
- Liu X, Dong X, Li T, et al. A difficulty-aware and task-augmentation method based on meta-learning model for few-shot diabetic retinopathy classification. Quant Imaging Med Surg 2024;14:861-76. [Crossref] [PubMed]
- Fu DJ, Faes L, Wagner SK, et al. Predicting Incremental and Future Visual Change in Neovascular Age-Related Macular Degeneration Using Deep Learning. Ophthalmol Retina 2021;5:1074-84. [Crossref] [PubMed]
- Wang X, Ju L, Zhao X, Ge Z. Retinal abnormalities recognition using regional multitask learning. In: Medical Image Computing and Computer Assisted Intervention–MICCAI 2019: 22nd International Conference, Shenzhen, China, October 13–17, 2019, Proceedings, Part I 22. Springer International Publishing; 2019:30-8.
- Mahapatra D, Ge Z. Training data independent image registration using generative adversarial networks and domain adaptation. Pattern Recognition 2020;100:107109. [Crossref]
- Meng Q, Shin'ichi S. ADINet: Attribute driven incremental network for retinal image classification. In: Proceedings of the IEEE/CVF Conference on Computer Vision and Pattern Recognition. 2020:4033-42.
- He Y, Carass A, Liu Y, Saidha S, Calabresi PA, Prince JL. Adversarial domain adaptation for multi-device retinal OCT segmentation. In: Medical Imaging 2020: Image Processing. SPIE; 2020;11313:26-31.
- Algan G, Ulusoy I, Gönül Ş, Turgut B, Bakbak B. Deep learning from small amount of medical data with noisy labels: A meta-learning approach. arXiv:2010.06939 [Preprint]. 2020. Available online: https://arxiv.org/abs/2010.06939
- Vettoruzzo A, Bouguelia MR, Rögnvaldsson T. Multimodal meta-learning through meta-learned task representations. Neural Comput Appl 2024;36:8519-29. [Crossref]
- Murugappan M, Prakash NB, Jeya R, Mohanarathinam A, Hemalakshmi GR, Mahmud M. A novel few-shot classification framework for diabetic retinopathy detection and grading. Measurement 2022;200:111485. [Crossref]
- Cao P, Hou Q, Song R, et al. Collaborative learning of weakly-supervised domain adaptation for diabetic retinopathy grading on retinal images. Comput Biol Med 2022;144:105341. [Crossref] [PubMed]
- Rahim SS, Palade V, Shuttleworth J, et al. Automatic screening and classification of diabetic retinopathy and maculopathy using fuzzy image processing. Brain Inform 2016;3:249-67. [Crossref] [PubMed]
- Vengalil SK, Krishnamurthy B, Sinha N. Simultaneous segmentation of multiple structures in fundal images using multi-tasking deep neural networks. Frontiers in Signal Processing 2023;2:936875. [Crossref]
- Pan L, Shi F, Xiang D, et al. OCTRexpert:A Feature-based 3D Registration Method for Retinal OCT Images. IEEE Trans Image Process 2020; Epub ahead of print. [Crossref] [PubMed]
- Pan L, Chen X. Retinal OCT Image Registration: Methods and Applications. IEEE Rev Biomed Eng 2023;16:307-18. [Crossref] [PubMed]
- Luo G, Chen X, Shi F, et al. Multimodal affine registration for ICGA and MCSL fundus images of high myopia. Biomed Opt Express 2020;11:4443-57. [Crossref] [PubMed]
- Finn C, Abbeel P, Levine S. Model-agnostic meta-learning for fast adaptation of deep networks. In: International Conference on Machine Learning. PMLR; 2017:1126-35.
- Liu M, Chen X, Wang B. Automatic focus method using interference fringes magnitudes in retinal optical coherence tomography. Optical Engineering 2021;60:093101. [Crossref]
- Wahab Sait AR. A Lightweight Diabetic Retinopathy Detection Model Using a Deep-Learning Technique. Diagnostics (Basel) 2023;13:3120. [Crossref] [PubMed]
- Wang M, Zhu W, Yu K, et al. Semi-Supervised Capsule cGAN for Speckle Noise Reduction in Retinal OCT Images. IEEE Trans Med Imaging 2021;40:1168-83. [Crossref] [PubMed]
- Wang M, Zhu W, Shi F, et al. MsTGANet: Automatic Drusen Segmentation From Retinal OCT Images. IEEE Trans Med Imaging 2022;41:394-406. [Crossref] [PubMed]
- Ju L, Wang X, Zhou Q, Zhu H, Harandi M, Bonnington P, Drummond T, Ge Z. Bridge the domain gap between ultra-wide-field and traditional fundus images via adversarial domain adaptation. arXiv:2003.10042 [Preprint]. Available online: https://arxiv.org/abs/2003.10042
- Peng Y, Zhu W, Chen Z, et al. Automatic Staging for Retinopathy of Prematurity With Deep Feature Fusion and Ordinal Classification Strategy. IEEE Trans Med Imaging 2021;40:1750-62. [Crossref] [PubMed]
- Barberis A, Aerts HJWL, Buffa FM. Robustness and reproducibility for AI learning in biomedical sciences: RENOIR. Sci Rep 2024;14:1933. [Crossref] [PubMed]
- Gundersen OE, Kjensmo S. State of the art: Reproducibility in artificial intelligence. In: Proceedings of the AAAI Conference on Artificial Intelligence. 2018. doi:
10.1609/aaai.v32i1.11503 .10.1609/aaai.v32i1.11503 - Goodman SN, Fanelli D, Ioannidis JP. What does research reproducibility mean? Sci Transl Med 2016;8:341ps12. [Crossref] [PubMed]
- Danese C, Kale AU, Aslam T, et al. The impact of artificial intelligence on retinal disease management: Vision Academy retinal expert consensus. Curr Opin Ophthalmol 2023;34:396-402. [Crossref] [PubMed]