Multimodal radiomics based on fluorine-18 prostate-specific membrane antigen positron emission tomography and multiparametric magnetic resonance imaging in predicting persistent prostate-specific antigen after radical prostatectomy
Introduction
For patients with localized and locally progressed prostate cancer (PCa), radical prostatectomy (RP) provides favorable long-term oncological outcomes (1-3). However, a significant number of patients may experience disease recurrence after RP. Prostate-specific antigen (PSA) measurements play a crucial role in patient follow-up. The guidelines from the European Association of Urology (EAU) recommend a PSA assessment within 3 months after RP (4). Given the short half-life of PSA, it is theoretically possible that PSA is at an undetectable low level within 4–8 weeks after surgery. However, PSA can still be detected in 5% to 30% of patients with PCa after RP due to the presence of undetected micrometastases, residual malignant lesions, and residual benign tissue at the surgical margin (5). Persistent PSA is generally defined as a PSA level ≥0.1 ng/mL within 4–8 weeks after RP (6-15). Previous studies have discovered that persistent PSA is a powerful prognostic predictor for the development of metastasis and death following RP (6,8,12,15). Therefore, accurate prediction of persistent PSA before patients experience biochemical recurrence (BCR) could help identify high-risk patients with poor tumor prognosis in clinical practice, which could further assist physicians in determining which individuals are most likely to benefit from follow-up and adjuvant therapy.
In clinical practice, multiparametric magnetic resonance imaging (mpMRI) serves as the primary imaging modality to evaluate PCa. Many retrospective studies have highlighted the unique advantages of mpMRI in assessing PCa (16-18). Recently, positron emission tomography (PET) with prostate-specific membrane antigen (PSMA) targeting has come into prominence as a novel imaging evaluation approach for PCa, due to its whole-body imaging capabilities and the advantages of specific prostate targeting (19-22). 18F-labeled PSMA-targeted radiopharmaceuticals have a higher image resolution compared to other radiopharmaceuticals (21,23). Notably, in clinical applications, 18F-PSMA-1007 has been successfully applied in diagnosing PCa and has demonstrated good performance (24). The integration of clinical, radiological, and functional imaging parameters before surgery has also been shown to be effective in predicting persistent PSA (25).
As a novel noninvasive technique, radiomics aims to extract and analyze a wide range of quantitative imaging features from radiographic images with high throughput. This facilitates a comprehensive characterization of intratumoral information and changes (26). Handcrafted radiomics features have been used in previous studies to assist with the detection and prognosis of various cancers, including breast cancer (27), pancreatic neuroendocrine tumor (28), and lung cancer (29). Recently, the application of radiomics in PCa has garnered intense extensive research attention due to its potentially significant benefits for healthcare systems (30-33). A number of studies have reported on radiomics improving PCa detection, International Society of Urological Pathology (ISUP) Grade Group prediction, and BCR in patients with primary PCa; however, there is little evidence regarding the use of multimodal radiomics in predicting persistent PSA (31).
The study aimed to determine the value of preoperative 18F -PSMA-1007 PET and mpMRI radiomics in predicting persistent PSA after RP. We hypothesize that radiomics could be used to predict persistent PSA. We present this article in accordance with the TRIPOD reporting checklist (available at https://qims.amegroups.com/article/view/10.21037/qims-24-2162/rc).
Methods
Patient population
A total of 321 patients with histopathologically proven localized PCa who underwent RP at The First Affiliated Hospital of Wenzhou Medical University between January 2019 and March 2022 were included in this retrospective analysis. The laparoscopic RP approach was applied to patients including but not limited to those whose preoperative examination showed positive lymph nodes and who underwent lymph node dissection. The exclusion criteria were as follows: (I) adjuvant therapy administered before imaging exam or operation, (II) adjuvant therapy administered within 8 weeks after surgery, (III) lack of complete clinical and pathological data, and (IV) patients without complete preoperative 18F-PSMA-1007 PET and mpMRI data. The final analysis included a cohort of 141 patients. The study was conducted in accordance with the Declaration of Helsinki (as revised in 2013) and was approved by the Ethics Committee of The First Affiliated Hospital of Wenzhou Medical University (No. KY2022-R012). Informed consent was waived due to the retrospective nature of the analysis. The patient selection process is visually represented in Figure 1.
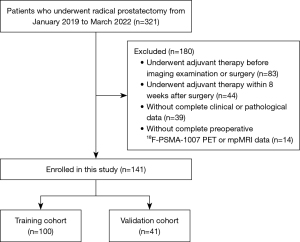
Imaging protocol
For each patient involved in the study, a hybrid PET-computed tomography (CT) scanner (Gemini TF 64, Philips Healthcare, Best, the Netherlands) was used to obtain the 18F-PSMA-1007 PET images. Additionally, 3.0-T MR scanners (Signa HDxt × t3.0 T, GE HealthCare, Chicago, IL, USA; Achieva 3.0 T, Philips Healthcare) were used conduct the mpMRI experiments. The mpMRI scans comprised a T2-weighted (T2W) sequence, a diffusion-weighted imaging (DWI) sequence, and apparent diffusion coefficient (ADC) maps. The details are provided in Appendix 1.
Image segmentation and radiomics feature extraction
All procedures were carried out in accordance with the Image Biomarker Standardization Initiative principles (34). The workflow for feature extraction is illustrated in Figure 2. To extract the radiomic features, the volumes of interest (VOIs) were first manually segmented in both 18F-PSMA-1007 PET and mpMRI images.
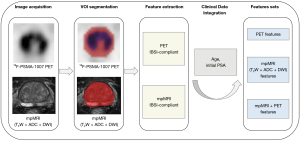
For the PET images, LIFEx software (version 6.30; https://www.lifexsoft.org) was used to segment the whole prostate. During the segmentation process, PET’ VOIs were defined based on (I) the whole prostate (VOIwhole) and (II) the threshold at 40% of standardize uptake value (SUVmax) (VOI40%). A previous study reported that at a 40% SUVmax, radiomics features based on the 18F-PSMA-1007 PET demonstrate optimal predictive performance in assessing the extracapsular extension (ECE) of PCa (35). Before feature extraction, all VOIs underwent normalization and discretization with a fixed bin width (default =0.3) and subsequently were resampled to 2×2×2 mm3. Radiomics features were extracted from the defined VOIs (VOIwhole and VOI40%) in PET images using LIFEx software. For each of the defined VOIs, conventional features (SUVmin, SUVmax, SUVmean, etc.), shape features, and texture features were extracted separately.
For the mpMRI images, we used ITK-SNAP software (version 3.6.0; http://www.itksnap.org) to manually segment the whole prostate on DWI and T2W images in a slice-by-slice approach. Subsequently, rigid registration was performed to align the VOIs delineated on DWI scans with the corresponding regions on ADC maps, via ITK-SNAP software. Since ADC maps are derived from DWI data, rigid registration was sufficient to ensure spatial consistency. Finally, radiomics features extraction from mpMRI was carried out using the “Pyradiomics” package (version 2.2.0) in Python software (Python Software Foundation, Wilmington, DE, USA). A total of 1,316 features were extracted from the VOIs of each image sequence (T2W, DWI, and ADC), which included seven categories: (I) first order, (II) shape features, and texture features derived from a (III) gray-level co-occurrence matrix (GLCM), (IV) gray-level difference method (GLDM), (V) gray-level run-length matrix (GLRLM), (VI) gray-level size zone matrix (GLSZM), and (VII) neighborhood gray-tone difference matrix (NGTDM).
The segmentations were carried out independently by two nuclear medicine physicians (with 3 and 5 years of experience interpreting PSMA hybrid imaging, respectively) and two radiologists (with 3 and 5 years of experience reading prostate mpMRl). To guarantee impartial and unbiased results, all doctors were kept blind to patient-specific information during the VOIs delineation. The intraclass correlation coefficient (ICC) with one-way analysis of variance (ANOVA) was used to assess interobserver reproducibility. High feature stability was indicated by an ICC of ≥0.75. All parameters with an ICC <0.75 were initially eliminated in order to decrease unstable features.
Prediction models
First, the preserved stable radiomics features were sorted using the minimum redundancy maximum relevance (mRMR) algorithm, with the top 15 radiomic features most related to persistent PSA being selected for each model. Subsequently, the least absolute shrinkage and selection operator (LASSO) algorithm with 10-fold cross-validation was used to select the most optimized feature subsets for building the radiomics models. To identify persistent PSA, multivariate logistic regression was used to develop prediction models using the previously selected optimized feature subsets on the training cohort. The prediction capacity of models was then computed on the validation cohort. Different prediction models were established. We first established and compared the predictive performance of two PET radiomics models based on the defined VOIs (VOIwhole and VOI40%) and chose the model with better performance as the final PET radiomics model (referred to as the P model). Additionally, a mpMRI radiomics model (referred to as the M model) based on T2W, DWI, and ADC radiomics features and a multimodal radiomics model (referred to as the PM model) integrating the radiomics features from both the PET and mpMRI were established. Age and initial PSA level were incorporated as variables in the clinical model (referred to as the C model) because these variables have long been considered to be independent predictors in PCa risk calculators (36). Finally, the clinical variables (age + initial PSA) were added to the best-performing radiomics model to further investigate whether a combined radiomics-clinical model (referred to as the combined model) might more accurately distinguish persistent PSA from nonpersistent PSA. The area under curve (AUC), sensitivity, specificity, accuracy, and F1 value were used to assess these models’ prediction ability.
As only 26 patients had persistent PSA after RP (~18%), oversampling the less common category (PSA ≥0.1) in the training set was addressed by using the synthetic minority oversampling technique (SMOTE) to achieve a 1:1 ratio to the most common category (PSA <0.1). The prediction models were trained with the augmented training data, and then the ability of the trained models to predict persistent PSA was evaluated using the unaugmented validation data.
Statistical analysis
Statistical analyses were performed using SPSS software (version 26.0; IBM Corp., Armonk, NY, USA), MedCalc software (version 19.8, MedCalc Software, Mariakerke, Belgium), and R software (The R Foundation for Statistical Computing; version 4.1.2; https://www.R-project.org). For SPSS, the t test or Mann-Whitney test was used to compare the differences between continuous variables, while the Fisher exact test or Chi-squared test was applied for categorical variables. For R software, multivariable logistic regression was performed to create the prediction models, and decision curve analysis (DCA) was conducted to evaluate the clinical applicability of the models. The DeLong test was employed to examine the accuracy of prediction between the different models, and the net reclassification improvement (NRI) method was used to assess the incremental value of the models. MedCalc software was used for the assessment of model performance via AUC values and confusion matrix-derived indices. The reproducibility of VOI segmentation was evaluated using the Dice similarity coefficient (DSC), which quantifies the pairwise overlap between repeated segmentations. The DSC values were calculated using R software, with values ranging from 0 to 1. Higher values indicated greater overlap, and a DSC value exceeding 0.70 was considered indicative of good segmentation reproducibility. All statistical tests were conducted in two-sided fashion, with statistical significance defined as a P value of 0.05 or less.
Results
Study population and baseline characteristics
The study included 141 patients who met the criteria for participation. Based on their PSA levels within 4–8 weeks after RP, they were classified into groups: a persistent PSA (26 patients) group and a nonpersistent PSA group (115 patients). Subsequently, at a 7:3 ratio, the patients were randomly separated into a training cohort (n=100) and a validation cohort (n=41). Table 1 provides an overview of the baseline characteristics of these patients. No statistically significant differences were observed in baseline characteristics, such as age and weight, between the training and validation cohorts (P>0.05).
Table 1
Characteristic | Training cohort (n=100) | Validation cohort (n=41) | P value |
---|---|---|---|
Age (years), median [IQR] | 69 [64–72] | 67 [62–73] | 0.674 |
Weight (kg), median [IQR] | 69.45 [62–75] | 65 [58.25–74] | 0.054 |
Initial PSA (ng/mL), median [IQR] | 10.66 [7.48–17.65] | 10.66 [7.83–22.80] | 0.773 |
Persistent PSA | 0.789 | ||
Yes | 19 (19%) | 7 (17.07%) | |
No | 81 (81%) | 34 (82.93%) | |
ISUP GG | 0.153 | ||
1 | 5 (5%) | 2 (4.9%) | |
2 | 36 (36%) | 11 (26.8%) | |
3 | 39 (39%) | 13 (31.7%) | |
4 | 10 (10%) | 4 (9.8%) | |
5 | 10 (10%) | 11 (26.8%) | |
pT stage | 0.885 | ||
T2 | 52 (52%) | 20 (48.8%) | |
T3a | 27 (27%) | 11 (26.8%) | |
T3b | 17 (17%) | 9 (22%) | |
T4 | 4 (4%) | 1 (2.4%) | |
pN stage | 0.822 | ||
0 | 95 (95%) | 40 (97.6%) | |
1 | 5 (5%) | 1 (2.4%) | |
ECE | 0.884 | ||
Yes | 45 (45%) | 19 (46.3%) | |
No | 55 (55%) | 22 (54.7%) | |
PSM | 0.144 | ||
Yes | 31 (31%) | 18 (44%) | |
No | 69 (69%) | 23 (56%) | |
VI | 0.629 | ||
Yes | 18 (18%) | 6 (14.6%) | |
No | 82 (82%) | 35 (85.4%) |
ECE, extracapsular extension; IQR, interquartile range; ISUP GG, International Society of Urological Pathology Group Grade; PSA, prostate-specific antigen; PSM, positive surgical margin; VI, vascular invasion.
Model evaluation and comparison
A summary of the diagnostic performance of the established models for the classification of nonpersistent PSA versus persistent PSA (PSA <0.1 vs. ≥0.1) within 4–8 weeks following RP is provided in Table 2 and Figure 3.
Table 2
Models | Training cohort | Validation cohort | |||||||||
---|---|---|---|---|---|---|---|---|---|---|---|
AUC | ACC | SEN | SPE | F1 | AUC | ACC | SEN | SPE | F1 | ||
C model | 0.776 | 0.780 | 0.632 | 0.815 | 0.522 | 0.744 | 0.756 | 0.714 | 0.765 | 0.500 | |
M model | 0.862 | 0.780 | 0.842 | 0.765 | 0.593 | 0.815 | 0.707 | 0.714 | 0.706 | 0.455 | |
P model | 0.819 | 0.810 | 0.737 | 0.827 | 0.596 | 0.794 | 0.707 | 0.714 | 0.706 | 0.455 | |
PM model | 0.893 | 0.820 | 0.842 | 0.815 | 0.640 | 0.849 | 0.756 | 0.857 | 0.735 | 0.546 | |
Combined | 0.914 | 0.830 | 0.842 | 0.827 | 0.653 | 0.903 | 0.780 | 0.857 | 0.765 | 0.571 |
AUC, area under the curve; ACC, accuracy; C model, clinical model; M model, multiparametric magnetic resonance imaging-derived model; P model, positron emission tomography-derived model; PM model, PET- and mpMRI-derived model; SEN, sensitivity; SPE, specificity.
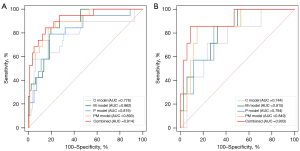
The reproducibility of manual segmentation of VOIs was good (average DSCs: 0.82 for PET, 0.78 for DWI, and 0.80 for T2WI). For the PET-derived radiomics models, the model based on VOI40% performed better than did the VOIwhole, and thus we chose the model based on the radiomics features from VOI40% as our P model (Appendix 1). Overall, The PM model, a multimodal radiomics model, was the best-performing radiomics model, with the highest AUC among other radiomics models in both the training [AUC =0.893; 95% confidence interval (CI): 0.815–0.946] and validation cohorts (AUC =0.849; 95% CI: 0.72–0.941). The P model yielded in an AUC of 0.819 (95% CI: 0.730–0.889) and 0.794 (95% CI: 0.639–0.904) in the training and validation cohorts. Meanwhile, the AUC value of the M model for predicting patients with persistent PSA was 0.862 (95% CI: 0.779–0.923) in the training cohort and 0.815 (95% CI: 0.663–0.919) in the validation cohort. The C model’s AUCs were 0.776 (95% CI: 0.681–0.853) and 0.744 (95% CI: 0.583–0.867) in the training and validation cohorts, respectively. When all radiomics models were compared to the C model alone, all demonstrated superior performance. The Delong test indicated no significant difference in AUC values between any of the radiomics models (P model, M model, and PM model) and the C model (P>0.05). However, the NRI indicated that the radiomics models, except the P model, could improve the ability to predict persistent PSA as compared to the C model (P<0.05),
The combined model constructed by combining the C model with the best-performing radiomics model was the best model, with AUCs in the training and validation cohorts of 0.914 (95% CI: 0.840–0.960) and 0.903 (95% CI: 0.770–0.973), respectively. These findings highlight the value of combining radiomics features with clinical variables to improve the accuracy of predicting persistent PSA after RP. According to the Delong test, the combined model and the C model had statistically significantly different AUC values (P<0.05). Additionally, DCA indicated that as compared to the C model or PM model and a process with all or no intervention, the combined model yielded larger net benefits within a given threshold probability (details in Figure 4). The details of the radiomic features and the parameters of the logistic regression model are shown in Appendix 2.
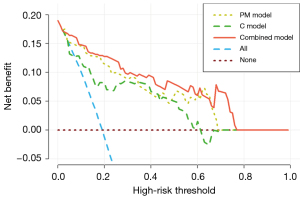
Discussion
In this study, we conducted an analysis of patients who underwent RP to assess the diagnostic accuracy of radiomics based on preoperative mpMRI and 18F-PSMA-1007 PET in the identification of persistent PSA. Overall, our analysis revealed that the combined model included both clinical variables and the best-performing radiomics model yielded a good predictive performance and outperformed all the radiomics models (P model, M model, and PM model), as well as the clinical baseline model (C model).
Identifying early persistent PSA after RP has significant clinical implications, as it is strongly correlated with poor metastasis-free survival, cancer-specific survival, and overall survival (6). Patients with persistent PSA tend to have more aggressive tumors and poorer oncology outcomes compared to those with undetectable PSA levels after RP (37). Therefore, accurately identifying those patients with persistent PSA after RP can assist in providing personalized treatment options so that patients may benefit from further treatment. Although previous research has focused on pathologic features and conventional imaging parameters for risk stratification in patients with persistent PSA (25), the value of radiomics in this context remains unclear. Our study represents the first comprehensive assessment of the potential of preoperative mpMRI and 18F-PSMA-1007 PET-based radiomics for predicting persistent PSA after RP. Consequently, our findings may offer novel insights and valuable contributions to this area of research.
The importance of hybrid PSMA PET and mpMRI in diagnosing, staging, and identifying recurrent lesions in PCa has been the subject of a thorough investigation (38-41). Its capacity to detect primary tumors and local recurrence, along with its utility in assessing capsular invasion and lymph node staging within the prostate gland, has been substantiated (42,43). Notably, some studies have suggested that this hybrid imaging technique may be more effective than MRI or PET alone in detecting PCa (44-46). Radiomics analysis has been widely employed in patients diagnosed with primary PCa (31). A significant number of these studies have primarily used MRI and have effectively demonstrated the ability of radiomics to accurately characterize and detect clinically significant PCa, as well as provide valuable insights into ECE and the prediction of BCR (32,33). Limited evidence exists regarding the clinical significance of combining PET and MRI radiomics features in predicting PCa. Basso Dias et al. conducted a study of patients with histopathologically confirmed PCa lesions with different ISUP grades to assess the diagnostic accuracy of radiomics features for identifying medium or high-risk tumors. The combined radiomics features based on MRI (including ADC and T2W) and 18F-DCFPyL (2-(3-(1-carboxy-5-[(6-18F-fluoro-pyr-idine-3-carbonyl)-amino]-pentyl)-ureido)-pentanedioic acid) PET performed better than did the baseline clinical model. However, the final radiomics model did not significantly benefit from the addition of clinical data in terms of improved predictive value (47). Solari et al. investigated the performance of radiomics based on [68Ga]Ga-PSMA-11 PET/MR to predict postoperative ISUP grade. According to their research, the radiomics model combining ADC and PET features had the highest accuracy (accuracy =0.82). Overall, the ADC exhibited promising outcomes in single-modality models, and its accuracy improved with the incorporation of T2W- or PET-derived features (48). Furthermore, Feliciani et al. developed radiomics models based on MRI-ADC and [68Ga]Ga-PSMA-11 PET images to assess their ability to differentiate low-risk patients with PCa (ISUP =1) from higher-risk patients (ISUP >1). They found that for predicting patients with low ISUP Group Grade, the ADC and [68Ga]Ga-PSMA-11 PET radiomics feature-based models were equal and complementary (49). Overall, there is limited research on combining 18F-PSMA-1007 PET and MRI radiomics in PCa. The majority of radiomics studies of combined PET and MRI are based on 68Ga-labeled PSMA tracers and may not be exactly comparable to our 18F-PSMA-1007 tracer as they have different resolutions and uptake patterns. Furthermore, most studies have focused on predicting ISUP Group Grade, and there is limited literature on early persistent PSA after RP.
As stated above, our finding may have implications for clinical practice. Our combined model outperformed both the radiomics models and the clinical baseline model in assessing early persistent PSA. This model was constructed from clinical variables (age + initial PSA) and PET- and mpMRI- (including T2W, DWI, and ADC) derived radiomics features. The radiomics features included first-order features such as “lbp-3D-m2_first-order_90Percentile” for DWI, “lbp-3D-k_first-order_Median” for T2W, and “wavelet-LLL_first-order_Minimum” for ADC. Here, “lbp” (local binary patterns) is a texture analysis method that captures local spatial variations in voxel intensity. The “m2” and “k” in “lbp-3D-m2” and “lbp-3D-k” refer to different lbp radius and neighborhood configurations, which influence the granularity of the extracted texture features. The “90Percentile” represents the 90th percentile of intensity values in the transformed image, while the “Median” denotes the median intensity value. “Wavelet-LLL” refers to low-low-low (LLL) frequency wavelet decomposition, which applies low-pass filtering along three orthogonal axes (x, y, z) to preserve coarse structural information while removing high-frequency noise. “Minimum” corresponds to the minimum voxel intensity value after wavelet transformation. These features quantitatively describe the distribution and texture characteristics of the medical images, aiding in the differentiation of tissue properties. Additionally, it was also discovered that persistent PSA could be predicted through the use of a few second-order features including “GLZLM_ZLNU” for PET, which quantifies the nonuniformity of the gray levels or the length of the homogeneous zones. Specifically, GLZLM stands for Gray Level Zone Length Matrix, a method that captures the spatial distribution of gray levels in an image. The ZLNU (Zone Length Nonuniformity) measures the variability in the length of contiguous zones with the same gray level intensity. A higher ZLNU value indicates greater heterogeneity in zone lengths, providing information about the complexity and texture of the image. Other second-order features such as GLSZM, GLRLM, and GLCM, reflect the spatial distribution of gray levels. In summary, each of these radiomics features characterized tumor heterogeneity and served a complementary role in predicting persistent PSA. It should be mentioned that while our study primarily focused on evaluating the predictive ability of preoperative 18F-PSMA-1007 PET- and mpMRI-derived radiomics features in predicting PSA persistence after RP, we acknowledge that preoperative molecular staging, such as PSMA PET findings related to lymph node involvement, could potentially enhance the model’s predictive accuracy. Due to our study population consisting of patients with localized PCa and the small number of patients with preoperative nodal involvement on imaging (n=6), the molecular stage was not included as a variable in the clinical model. In clinical practice, early PSA monitoring following RP can help identify high-risk patients with worse oncologic outcomes. Therefore, our combined model may improve clinical treatment. Our study roughly evaluated the value of radiomics features in predicting early postoperative PSA. Further prospective studies are required to assess whether using PET and mpMRI radiomics in treatment selection results in better patient outcomes.
There are several limitations in our study. First, potential selection bias could have arisen due to the study’s retrospective design. Second, persistent PSA is a relatively rare event, and we thus only examined a limited number of events in our study. This could have led to the overfitting of models, potentially constraining the models’ generalizability. The DeLong test indicated no statistically significant difference in the AUC values between the combined model and radiomics models. The small patient population and the imbalanced dataset may partly explain this. The statistical power of the findings may be limited. Additionally, although we used whole-prostate segmentations in our study to reduce complexity and achieve effective radiomics, our segmentation strategy could still be improved. Future research could be enhanced by implementing a fully automated fine-segmentation approach through the appropriate training of a deep learning-based algorithm (50,51). Finally, it is important to note that our clinical baseline model included only age and initial PSA; however, Hao et al. demonstrated that preoperative PSA, high-risk group, preoperative ISUP grades 2–5, percentage of positive cores, and other postoperative pathological indicators are independent risk factors for PSA persistence in patients with PCa following RP (52). Due to limitations in data collection and insufficient documentation, biopsy ISUP Group Grade was excluded from our baseline model analysis, which might have affected the predictive performance of the clinical model. Future studies will be focused on the gathering of more extensive and comprehensive multicenter data to address the abovementioned limitations.
Conclusions
In our study, we demonstrated the potential of radiomics based on preoperative images to predict persistent PSA after RP. We successfully developed and validated a novel, combined radiomics-clinical model that outperformed all the other radiomic models and clinical baseline model in predicting postoperative persistent PSA. This highlights the value of the radiomics features derived from 18F-PSMA-1007 PET and mpMRI in complementing clinical variables for early postoperative risk stratification in PCa. Further verification studies are needed to confirm the reproducibility and clinical utility of this method.
Acknowledgments
The authors would like to thank the Prostate Cancer research group of the First Affiliated Hospital of Wenzhou Medical University for their contribution.
Footnote
Reporting Checklist: The authors have completed the TRIPOD reporting checklist. Available at https://qims.amegroups.com/article/view/10.21037/qims-24-2162/rc
Funding: This work was supported by
Conflicts of Interest: All authors have completed the ICMJE uniform disclosure form (available at https://qims.amegroups.com/article/view/10.21037/qims-24-2162/coif). The authors have no conflicts of interest to declare.
Ethical Statement: The authors are accountable for all aspects of the work in ensuring that questions related to the accuracy or integrity of any part of the work are appropriately investigated and resolved. This study was conducted in accordance with the Declaration of Helsinki (as revised in 2013) and was approved by the Ethics Committee of The First Affiliated Hospital of Wenzhou Medical University (No. KY2022-R012). Informed consent was waived due to the retrospective nature of the analysis.
Open Access Statement: This is an Open Access article distributed in accordance with the Creative Commons Attribution-NonCommercial-NoDerivs 4.0 International License (CC BY-NC-ND 4.0), which permits the non-commercial replication and distribution of the article with the strict proviso that no changes or edits are made and the original work is properly cited (including links to both the formal publication through the relevant DOI and the license). See: https://creativecommons.org/licenses/by-nc-nd/4.0/.
References
- Beyer B, Schlomm T, Tennstedt P, Boehm K, Adam M, Schiffmann J, Sauter G, Wittmer C, Steuber T, Graefen M, Huland H, Haese A. A feasible and time-efficient adaptation of NeuroSAFE for da Vinci robot-assisted radical prostatectomy. Eur Urol 2014;66:138-44. [Crossref] [PubMed]
- Moris L, Cumberbatch MG, Van den Broeck T, Gandaglia G, Fossati N, Kelly B, et al. Benefits and Risks of Primary Treatments for High-risk Localized and Locally Advanced Prostate Cancer: An International Multidisciplinary Systematic Review. Eur Urol 2020;77:614-27. [Crossref] [PubMed]
- Joniau SG, Van Baelen AA, Hsu CY, Van Poppel HP. Complications and functional results of surgery for locally advanced prostate cancer. Adv Urol 2012;2012:706309. [Crossref] [PubMed]
- Mottet N, Bellmunt J, Bolla M, Briers E, Cumberbatch MG, De Santis M, et al. EAU-ESTRO-SIOG Guidelines on Prostate Cancer. Part 1: Screening, Diagnosis, and Local Treatment with Curative Intent. Eur Urol 2017;71:618-29. [Crossref] [PubMed]
- Mottet N, van den Bergh RCN, Briers E, Van den Broeck T, Cumberbatch MG, De Santis M, et al. EAU-EANM-ESTRO-ESUR-SIOG Guidelines on Prostate Cancer-2020 Update. Part 1: Screening, Diagnosis, and Local Treatment with Curative Intent. Eur Urol 2021;79:243-62. [Crossref] [PubMed]
- Preisser F, Chun FKH, Pompe RS, Heinze A, Salomon G, Graefen M, Huland H, Tilki D. Persistent Prostate-Specific Antigen After Radical Prostatectomy and Its Impact on Oncologic Outcomes. Eur Urol 2019;76:106-14. [Crossref] [PubMed]
- Fossati N, Karnes RJ, Colicchia M, Boorjian SA, Bossi A, Seisen T, et al. Impact of Early Salvage Radiation Therapy in Patients with Persistently Elevated or Rising Prostate-specific Antigen After Radical Prostatectomy. Eur Urol 2018;73:436-44. [Crossref] [PubMed]
- Milonas D, Venclovas Z, Sasnauskas G, Ruzgas T. The Significance of Prostate Specific Antigen Persistence in Prostate Cancer Risk Groups on Long-Term Oncological Outcomes. Cancers (Basel) 2021.
- Ploussard G, Fossati N, Wiegel T, D'Amico A, Hofman MS, Gillessen S, Mottet N, Joniau S, Spratt DE. Management of Persistently Elevated Prostate-specific Antigen After Radical Prostatectomy: A Systematic Review of the Literature. Eur Urol Oncol 2021;4:150-69. [Crossref] [PubMed]
- Spratt DE, Dai DLY, Den RB, Troncoso P, Yousefi K, Ross AE, et al. Performance of a Prostate Cancer Genomic Classifier in Predicting Metastasis in Men with Prostate-specific Antigen Persistence Postprostatectomy. Eur Urol 2018;74:107-14. [Crossref] [PubMed]
- Audenet F, Seringe E, Drouin SJ, Comperat E, Cussenot O, Bitker MO, Rouprêt M. Persistently elevated prostate-specific antigen at six weeks after radical prostatectomy helps in early identification of patients who are likely to recur. World J Urol 2012;30:239-44. [Crossref] [PubMed]
- Kumar A, Samavedi S, Mouraviev V, Bates AS, Coelho RF, Rocco B, Patel VR. Predictive factors and oncological outcomes of persistently elevated prostate-specific antigen in patients following robot-assisted radical prostatectomy. J Robot Surg 2017;11:37-45. [Crossref] [PubMed]
- Kimura S, Urabe F, Sasaki H, Kimura T, Miki K, Egawa S. Prognostic Significance of Prostate-Specific Antigen Persistence after Radical Prostatectomy: A Systematic Review and Meta-Analysis. Cancers (Basel) 2021.
- Bartkowiak D, Siegmann A, Böhmer D, Budach V, Wiegel T. The impact of prostate-specific antigen persistence after radical prostatectomy on the efficacy of salvage radiotherapy in patients with primary N0 prostate cancer. BJU Int 2019;124:785-91. [Crossref] [PubMed]
- Ploussard G, Staerman F, Pierrevelcin J, Saad R, Beauval JB, Roupret M, Audenet F, Peyromaure M, Delongchamps NB, Vincendeau S, Fardoun T, Rigaud J, Villers A, Bastide C, Soulie M, Salomon LCommittee of Cancerology of the Association of French Urology. Predictive factors of oncologic outcomes in patients who do not achieve undetectable prostate specific antigen after radical prostatectomy. J Urol 2013;190:1750-6. [Crossref] [PubMed]
- de Rooij M, Hamoen EH, Witjes JA, Barentsz JO, Rovers MM. Accuracy of Magnetic Resonance Imaging for Local Staging of Prostate Cancer: A Diagnostic Meta-analysis. Eur Urol 2016;70:233-45. [Crossref] [PubMed]
- Şahin B, Çelik S, Sözen S, Türkeri L, Aslan G, Yazıcı S, Çetin SMembers of Turkish Urooncology Association. A new parameter to increase the predictive value of multiparametric prostate magnetic resonance imaging for clinically significant prostate cancer in targeted biopsies: lesion density. Prostate Int 2024;12:145-50. [Crossref] [PubMed]
- Kasivisvanathan V, Rannikko AS, Borghi M, Panebianco V, Mynderse LA, Vaarala MH, et al. MRI-Targeted or Standard Biopsy for Prostate-Cancer Diagnosis. N Engl J Med 2018;378:1767-77. [Crossref] [PubMed]
- Czarniecki M, Mena E, Lindenberg L, Cacko M, Harmon S, Radtke JP, Giesel F, Turkbey B, Choyke PL. Keeping up with the prostate-specific membrane antigens (PSMAs): an introduction to a new class of positron emission tomography (PET) imaging agents. Transl Androl Urol 2018;7:831-43. [Crossref] [PubMed]
- Zippel C, Ronski SC, Bohnet-Joschko S, Giesel FL, Kopka K. Current Status of PSMA-Radiotracers for Prostate Cancer: Data Analysis of Prospective Trials Listed on ClinicalTrials.gov. Pharmaceuticals (Basel) 2020.
- Wester HJ, Schottelius M. PSMA-Targeted Radiopharmaceuticals for Imaging and Therapy. Semin Nucl Med 2019;49:302-12. [Crossref] [PubMed]
- Fendler WP, Eiber M, Beheshti M, Bomanji J, Ceci F, Cho S, Giesel F, Haberkorn U, Hope TA, Kopka K, Krause BJ, Mottaghy FM, Schöder H, Sunderland J, Wan S, Wester HJ, Fanti S, Herrmann K. (68)Ga-PSMA PET/CT: Joint EANM and SNMMI procedure guideline for prostate cancer imaging: version 1.0. Eur J Nucl Med Mol Imaging 2017;44:1014-24. [Crossref] [PubMed]
- Sengupta S, Asha Krishnan M, Chattopadhyay S, Chelvam V. Comparison of prostate-specific membrane antigen ligands in clinical translation research for diagnosis of prostate cancer. Cancer Rep (Hoboken) 2019;2:e1169. [Crossref] [PubMed]
- Awenat S, Piccardo A, Carvoeiras P, Signore G, Giovanella L, Prior JO, Treglia G. Diagnostic Role of 18F-PSMA-1007 PET/CT in Prostate Cancer Staging: A Systematic Review. Diagnostics (Basel) 2021;11:552. [Crossref] [PubMed]
- Mazzone E, Gandaglia G, Robesti D, Rajwa P, Gomez Rivas J, Ibáñez L, et al. Which Patients with Prostate Cancer and Lymph Node Uptake at Preoperative Prostate-specific Membrane Antigen Positron Emission Tomography/Computerized Tomography Scan Are at a Higher Risk of Prostate-specific Antigen Persistence After Radical Prostatectomy? Identifying Indicators of Systemic Disease by Integrating Clinical, Magnetic Resonance Imaging, and Functional Imaging Parameters. Eur Urol Oncol 2024;7:231-40. [Crossref] [PubMed]
- Lambin P, Rios-Velazquez E, Leijenaar R, Carvalho S, van Stiphout RG, Granton P, Zegers CM, Gillies R, Boellard R, Dekker A, Aerts HJ. Radiomics: extracting more information from medical images using advanced feature analysis. Eur J Cancer 2012;48:441-6. [Crossref] [PubMed]
- Valdora F, Houssami N, Rossi F, Calabrese M, Tagliafico AS. Rapid review: radiomics and breast cancer. Breast Cancer Res Treat 2018;169:217-29. [Crossref] [PubMed]
- Bezzi C, Mapelli P, Presotto L, Neri I, Scifo P, Savi A, Bettinardi V, Partelli S, Gianolli L, Falconi M, Picchio M. Radiomics in pancreatic neuroendocrine tumors: methodological issues and clinical significance. Eur J Nucl Med Mol Imaging 2021;48:4002-15. [Crossref] [PubMed]
- Thawani R, McLane M, Beig N, Ghose S, Prasanna P, Velcheti V, Madabhushi A. Radiomics and radiogenomics in lung cancer: A review for the clinician. Lung Cancer 2018;115:34-41. [Crossref] [PubMed]
- Yi Z, Hu S, Lin X, Zou Q, Zou M, Zhang Z, Xu L, Jiang N, Zhang Y. Machine learning-based prediction of invisible intraprostatic prostate cancer lesions on (68) Ga-PSMA-11 PET/CT in patients with primary prostate cancer. Eur J Nucl Med Mol Imaging 2022;49:1523-34. [Crossref] [PubMed]
- Spohn SKB, Bettermann AS, Bamberg F, Benndorf M, Mix M, Nicolay NH, Fechter T, Hölscher T, Grosu R, Chiti A, Grosu AL, Zamboglou C. Radiomics in prostate cancer imaging for a personalized treatment approach - current aspects of methodology and a systematic review on validated studies. Theranostics 2021;11:8027-42. [Crossref] [PubMed]
- Bourbonne V, Fournier G, Vallières M, Lucia F, Doucet L, Tissot V, Cuvelier G, Hue S, Le Penn Du H, Perdriel L, Bertrand N, Staroz F, Visvikis D, Pradier O, Hatt M, Schick U. External Validation of an MRI-Derived Radiomics Model to Predict Biochemical Recurrence after Surgery for High-Risk Prostate Cancer. Cancers (Basel) 2020.
- Shiradkar R, Ghose S, Jambor I, Taimen P, Ettala O, Purysko AS, Madabhushi A. Radiomic features from pretreatment biparametric MRI predict prostate cancer biochemical recurrence: Preliminary findings. J Magn Reson Imaging 2018;48:1626-36. [Crossref] [PubMed]
- Zwanenburg A, Vallières M, Abdalah MA, Aerts HJWL, Andrearczyk V, Apte A, et al. The Image Biomarker Standardization Initiative: Standardized Quantitative Radiomics for High-Throughput Image-based Phenotyping. Radiology 2020;295:328-38. [Crossref] [PubMed]
- Yao F, Bian S, Zhu D, Yuan Y, Pan K, Pan Z, Feng X, Tang K, Yang Y. Machine learning-based radiomics for multiple primary prostate cancer biological characteristics prediction with (18)F-PSMA-1007 PET: comparison among different volume segmentation thresholds. Radiol Med 2022;127:1170-8. [Crossref] [PubMed]
- Ankerst DP, Straubinger J, Selig K, Guerrios L, De Hoedt A, Hernandez J, Liss MA, Leach RJ, Freedland SJ, Kattan MW, Nam R, Haese A, Montorsi F, Boorjian SA, Cooperberg MR, Poyet C, Vertosick E, Vickers AJ. A Contemporary Prostate Biopsy Risk Calculator Based on Multiple Heterogeneous Cohorts. Eur Urol 2018;74:197-203. [Crossref] [PubMed]
- García-Barreras S, Rozet F, Nunes-Silva I, Srougi V, Sanchez-Salas R, Barret E, Galiano M, Cathelineau X. Predictive factors and the important role of detectable prostate-specific antigen for detection of clinical recurrence and cancer-specific mortality following robot-assisted radical prostatectomy. Clin Transl Oncol 2018;20:1004-10. [Crossref] [PubMed]
- Domachevsky L, Bernstine H, Goldberg N, Nidam M, Catalano OA, Groshar D. Comparison between pelvic PSMA-PET/MR and whole-body PSMA-PET/CT for the initial evaluation of prostate cancer: a proof of concept study. Eur Radiol 2020;30:328-36. [Crossref] [PubMed]
- Park SY, Zacharias C, Harrison C, Fan RE, Kunder C, Hatami N, Giesel F, Ghanouni P, Daniel B, Loening AM, Sonn GA, Iagaru A. Gallium 68 PSMA-11 PET/MR Imaging in Patients with Intermediate- or High-Risk Prostate Cancer. Radiology 2018;288:495-505. [Crossref] [PubMed]
- Ferraro DA, Becker AS, Kranzbühler B, Mebert I, Baltensperger A, Zeimpekis KG, Grünig H, Messerli M, Rupp NJ, Rueschoff JH, Mortezavi A, Donati OF, Sapienza MT, Eberli D, Burger IA. Diagnostic performance of (68)Ga-PSMA-11 PET/MRI-guided biopsy in patients with suspected prostate cancer: a prospective single-center study. Eur J Nucl Med Mol Imaging 2021;48:3315-24. [Crossref] [PubMed]
- Metser U, Ortega C, Perlis N, Lechtman E, Berlin A, Anconina R, Eshet Y, Chan R, Veit-Haibach P, van der Kwast TH, Liu A, Ghai S. Detection of clinically significant prostate cancer with (18)F-DCFPyL PET/multiparametric MR. Eur J Nucl Med Mol Imaging 2021;48:3702-11. [Crossref] [PubMed]
- Freitag MT, Radtke JP, Afshar-Oromieh A, Roethke MC, Hadaschik BA, Gleave M, Bonekamp D, Kopka K, Eder M, Heusser T, Kachelriess M, Wieczorek K, Sachpekidis C, Flechsig P, Giesel F, Hohenfellner M, Haberkorn U, Schlemmer HP, Dimitrakopoulou-Strauss A. Local recurrence of prostate cancer after radical prostatectomy is at risk to be missed in (68)Ga-PSMA-11-PET of PET/CT and PET/MRI: comparison with mpMRI integrated in simultaneous PET/MRI. Eur J Nucl Med Mol Imaging 2017;44:776-87. [Crossref] [PubMed]
- Grubmüller B, Baltzer P, Hartenbach S, D'Andrea D, Helbich TH, Haug AR, et al. PSMA Ligand PET/MRI for Primary Prostate Cancer: Staging Performance and Clinical Impact. Clin Cancer Res 2018;24:6300-7. [Crossref] [PubMed]
- Muehlematter UJ, Burger IA, Becker AS, Schawkat K, Hötker AM, Reiner CS, Müller J, Rupp NJ, Rüschoff JH, Eberli D, Donati OF. Diagnostic Accuracy of Multiparametric MRI versus (68)Ga-PSMA-11 PET/MRI for Extracapsular Extension and Seminal Vesicle Invasion in Patients with Prostate Cancer. Radiology 2019;293:350-8. [Crossref] [PubMed]
- Scheltema MJ, Chang JI, Stricker PD, van Leeuwen PJ, Nguyen QA, Ho B, Delprado W, Lee J, Thompson JE, Cusick T, Spriensma AS, Siriwardana AR, Yuen C, Kooner R, Hruby G, O'Neill G, Emmett L. Diagnostic accuracy of (68) Ga-prostate-specific membrane antigen (PSMA) positron-emission tomography (PET) and multiparametric (mp)MRI to detect intermediate-grade intra-prostatic prostate cancer using whole-mount pathology: impact of the addition of (68) Ga-PSMA PET to mpMRI. BJU Int 2019;124:42-9. [Crossref] [PubMed]
- Burger IA, Müller J, Donati OF, Ferraro DA, Messerli M, Kranzbühler B, Ter Voert EEGW, Muehlematter UJ, Rupp NJ, Mortezavi A, Eberli D. (68)Ga-PSMA-11 PET/MR Detects Local Recurrence Occult on mpMRI in Prostate Cancer Patients After HIFU. J Nucl Med 2019;60:1118-23. [Crossref] [PubMed]
- Basso Dias A, Mirshahvalad SA, Ortega C, Perlis N, Berlin A, van der Kwast T, Ghai S, Jhaveri K, Metser U, Haider M, Avery L, Veit-Haibach P. The role of [18F]-DCFPyL PET/MRI radiomics for pathological grade group prediction in prostate cancer. Eur J Nucl Med Mol Imaging 2023;50:2167-76.
- Solari EL, Gafita A, Schachoff S, Bogdanović B, Villagrán Asiares A, Amiel T, Hui W, Rauscher I, Visvikis D, Maurer T, Schwamborn K, Mustafa M, Weber W, Navab N, Eiber M, Hatt M, Nekolla SG. The added value of PSMA PET/MR radiomics for prostate cancer staging. Eur J Nucl Med Mol Imaging 2022;49:527-38. [Crossref] [PubMed]
- Feliciani G, Celli M, Ferroni F, Menghi E, Azzali I, Caroli P, Matteucci F, Barone D, Paganelli G, Sarnelli A. Radiomics Analysis on [68Ga]Ga-PSMA-11 PET and MRI-ADC for the Prediction of Prostate Cancer ISUP Grades: Preliminary Results of the BIOPSTAGE Trial. Cancers (Basel) 2022;14:1888.
- Xu Z, Heidari AA, Kuang F, Khalil A, Mafarja M, Zhang S, Chen H, Pan Z. Enhanced Gaussian bare-bones grasshopper optimization: Mitigating the performance concerns for feature selection. Expert Syst Appl 2023; [Crossref]
- Xu Y, Huang H, Heidari AA, Gui W, Ye X, Chen Y, Chen H, Pan Z. MFeature: Towards high performance evolutionary tools for feature selection. Expert Syst Appl 2021;186:115655.
- Hao S, Wang H, Lin S, Chen H, Xie L, Zheng X. Analysis of risk factors for persistent PSA after radical prostatectomy: results from a high-volume center in Southeast China. BMC Urol 2024;24:185. [Crossref] [PubMed]