Brain white matter microstructural alterations in patients with diabetic retinopathy: an automated fiber-tract quantification study
Introduction
Diabetic retinopathy (DR) is one of the most common microvascular complications in diabetic patients, which seriously affects patients’ quality of life. The International Diabetes Federation estimates that 642 million people worldwide will be affected by diabetes in 2040. As the prevalence of diabetes continues to increase, diabetes-related complications, such as DR, will also increase (1). The main pathological manifestations of DR include glucose metabolic disorders of retinal cells, blood-retinal barrier disruption, and increased retinal vascular permeability (2,3). Recent studies have shown that DR may be associated with white matter lesions, cognitive impairment, and dementia (4-6). However, it is unclear whether DR is an independent risk factor for these conditions or whether it can predict damage in the brain.
To date, neuroimaging studies on DR have mostly applied the functional magnetic resonance imaging (MRI) technique to study the neurophysiopathological changes in patients with DR. For example, Wang et al. (7) documented altered amplitude of low-frequency fluctuation in visual-related and cognitive-related brain regions in patients with DR. Huang et al. (8) reported widespread abnormal intranetwork functional connectivity and functional network connectivity (in 9 functional networks, such as the visual network, auditory network, ventral default mode network, and dorsal default mode network) in patients with DR using independent component analysis. Wan et al. (9) demonstrated abnormal changes in the functional connectivity of the visual- and cognitive-related functional brain networks in patients with DR by using network analysis. Nonetheless, few studies have applied the diffusion tensor imaging (DTI) technique to investigate brain white matter microstructural alterations in patients with DR. DTI is a non-invasive imaging method used to explore central nervous system alterations, which can provide detailed information about the microstructure of tissues by measuring the diffusion properties of water molecules in the brain tissue.
Many methods have been used to analyze DTI data. Traditional analysis methods include voxel-based analysis and tract-based spatial statistics, which can only analyze the abnormalities of white matter fiber tracts at the individual level, and cannot identify the specific altered segments of each fiber tract. The automated fiber-tract quantification (AFQ) technique can fill this gap by applying pointwise comparative analysis. Using whole-brain deterministic fiber tractography, AFQ can reconstruct fiber tracts by estimating 100 anatomically equivalent positions along each fiber trajectory, and estimates the point-by-point diffusion parameters for each specific fiber bundle, providing more accurate information for quantitative analysis studies. Yeatman et al. (10) proposed the concept of AFQ in 2012. In the ICBM-DTI-81 white-matter labels atlas developed at Johns Hopkins University, AFQ can automatically extract 20 major white matter fiber tracts, further segment each fiber tract into 100 equidistant nodes, and then calculate fractional anisotropy (FA), mean diffusion (MD), axial diffusion (AD), and radial diffusion (RD) in 100 nodes of each fiber tract. This technique allows the observation of alterations in brain white matter fiber tracts at the point-wise level. In recent years, the AFQ technique has been applied successfully to study many diseases, such as Alzheimer’s disease, schizophrenia, and depression (11-13).
For the first time, the present study applied the AFQ technique to analyze the damage patterns of 100 different nodes of 20 specific brain white matter fiber tracts in patients with DR, and explored the correlation between altered DTI metrics and cognitive test scores, as well as clinical indicators, so as to provide an imaging basis for understanding central nervous system alterations in patients with DR. We present this article in accordance with the STROBE reporting checklist (available at https://qims.amegroups.com/article/view/10.21037/qims-24-1440/rc).
Methods
Participants
A total of 19 patients who were diagnosed with stage IV–VI proliferative DR according to the diagnostic criteria for DR adopted by the American Academy of Ophthalmology in Taizhou People’s Hospital, Jiangsu Province from January 2022 to January 2023 were enrolled. The inclusion criteria were as follows: (I) age 25–60 years; (II) having DR caused by type 2 diabetes mellitus. Type 2 diabetes mellitus was diagnosed using the criteria established by the American Diabetes Association: fasting plasma glucose ≥7.0 mmol/L, random plasma glucose ≥11.1 mmol/L, or two hours glucose ≥11.1 mmol/L; meeting the diagnostic criteria of DR adopted by the American Academy of Ophthalmology; (III) right-handedness; and (IV) the absence of functional impairment, such as speech, vision or hearing impairment. The exclusion criteria were as follows: (I) patients with a history of psychiatric or neurological disorders or depression; (II) patients with other eye diseases, including dry eye syndrome, strabismus, amblyopia, cataract, glaucoma, optic neuritis, and ocular pain; (III) T1, T2, fluid-attenuated inversion recovery (FLAIR), and diffusion-weighted imaging (DWI) sequences were used to exclude cases with abnormal MRI changes (such as cerebral small vessel disease, brain tumor, and brain injury); and (IV) patients with MRI contraindications, such as pacemakers and stents.
A total of 20 age-, sex-, and education-matched healthy controls (HCs) were recruited from the hospital or local community. The inclusion criteria were as follows: (I) age 25–60 years; (II) right-handedness; (III) the absence of brain computed tomography (CT) or MRI abnormalities; and (IV) being able to cooperate with MRI and cognitive tests. The exclusion criteria for HCs were the same as those for patients with DR.
Two trained neurologists performed the cognitive tests on all patients. The data of all participants, including age and years of education, fasting blood glucose (FBG), and glycated hemoglobin A1c (HbA1c) levels within 1 day before MRI scan were collected and recorded. HbA1c levels were detected by using the fully automated glycated hemoglobin analyzer (HLC-723G8, Tosoh Bioscience, Tokyo, Japan). The cognitive function status of all patients was assessed using the Montreal Cognitive Assessment (MoCA) scale and Mini-Mental State Examination (MMSE) before the MRI scan.
The study was conducted in accordance with the Declaration of Helsinki (as revised in 2013). The study was approved by the Ethics Committee of Taizhou People’s Hospital (No. KY2022-079-01) and informed consent was provided by all individual participants.
Data acquisition and processing
Data acquisition
All MR scans were performed on a Siemens Skyra 3.0T MR scanner (Siemens, Erlangen, Germany) with 16-channel head coil. High-resolution structural images were acquired using 3-dimensional (3D)-T1-weighted sequences with the following parameters: repetition time (TR) =2,300 ms, echo time (TE) =2.98 ms, flip angle =9°, slices =176, slice thickness =1 mm, field of view (FOV) =256×256 mm, and voxel size =1 mm × 1 mm × 1 mm. The acquisition parameters for DTI data were as follows: TR =14,100 ms, TE =113 ms, slices =70, slice thickness =2 mm, 30 gradient diffusion directions, FOV =256×256 mm, voxel size =2 mm × 2 mm × 2 mm, and b-value =1,000 s/mm2. MRI scans of all participants were performed on the same MRI scanner.
Data processing
DTI data were preprocessed using FSL software (Oxford Centre for Functional MRI of the Brain, University of Oxford, Oxford, UK; http://www.fmrib.ox.ac.uk/fsl/) (14), with the following steps: (I) conversion of the raw Digital Imaging and Communications in Medicine (DICOM) format into Neuroimaging Informatics Technology Initiative (NIFTI) format; (II) removal of non-brain structures using the Brain Extraction Tool (BET) (15); (III) eddy-current and head-motion correction; (IV) fitting the diffusion tensor model to each image voxel using the DTIFit program (16) to generate FA, MD, AD, and RD values. Subsequently, the diffusion tensor maps were calculated for each participant.
The 3D T1WI data and the pre-processed DTI data were processed using the AFQ software package (https://github.com/jyeatman/AFQ). Quantitative analysis was performed on 100 equidistant nodes on each fiber tract, according to the following steps: (I) whole-brain fiber tracking; (II) fiber tract segmentation; (III) fiber tract refinement; (IV) fiber tract cleaning; (V) determination of the core of the fiber tract; and (VI) fiber tract quantification. The 20 tracts are as follows: the bilateral thalamus radiation, corticospinal tract, cingulum cingulate (CGC), cingulum hippocampus, inferior fronto-occipital fasciculus, inferior longitudinal fasciculus, superior longitudinal fasciculus (SLF), uncinated fasciculus, arcuate fasciculus, callosum forceps major, and callosum forceps minor.
Statistical analysis
Demographic data between the two groups were analyzed by SPSS 26.0 (IBM Corp., Armonk, NY, USA). Normally distributed data were expressed as mean ± standard deviation (SD), and analyzed using independent samples t-test. Non-normally distributed data were expressed as the median and 25th, 75th quartiles, and analyzed by using Mann-Whitney U test. A level of P<0.05 was considered statistically significant.
MATLAB software (MathWorks, Natick, MA, USA) was used to analyze the differences in FA, MD, AD, and RD values in 100 nodes of 20 fiber tracts between the two groups. False discovery rate (FDR) was used to correct for multiple comparisons. Partial correlation analysis was performed to analyze the correlation between altered DTI metrics in segments of each fiber tract and cognitive test scores, as well as clinical biochemical indicators in patients with DR. To control for possible confounding effects, we included age, gender, and years of education as covariates in our analyses. A P value less than 0.05 was considered significant.
Results
Demographic characteristics and cognitive test scores
The demographics and clinical information of patients with DR and HCs are shown in Table 1. A total of 39 participants (19 patients with DR and 20 HCs) were included. No significant differences in age, gender, education years, disease duration, MMSE score, FBG, and HbA1c levels were found between the two groups (P>0.05), whereas the MoCA score in the DR group was significantly lower than in the HC group (t=−4.878, P<0.001). Clinical signs of retinal edema (such as diabetic macular edema, cotton-wool spots, retinal thickness) were not noted in patients with DR.
Table 1
Variables | DR group (n=19) | HC group (n=20) | χ2/Z/t value | P value |
---|---|---|---|---|
Age (years) | 51.95±6.01 | 44.50±5.22 | −1.890 | 0.059a |
Education years | 12 [4, 16] | 10.36±3.54 | −1.471 | 0.141b |
Sex (male/female) | 7/12 | 12/9 | – | 0.113c |
Disease duration (years) | 9.47±3.76 | – | – | – |
HbA1c level (%) | 9 [6.9, 13.9] | – | – | – |
FBG level (mmol/L) | 13.09±4.69 | – | – | – |
MoCA scores | 23.47±2.20 | 28.5 [25, 30] | −4.878 | <0.001b |
MMSE scores | 28 [26, 29] | 29 [26, 30] | −1.767 | 0.77b |
Data are presented as number, median [25th percentile, 75th percentile] or mean ± standard deviation. a, two-sample t-test; b, Mann-Whitney U test; c, Chi-squared test. DR, diabetic retinopathy; HC, healthy control; FBG, fasting blood glucose; HbA1c, hemoglobin A1c; MoCA, Montreal Cognitive Assessment; MMSE, Mini-Mental State Examination.
Results of AFQ analysis
Of the 20-brain white matter fiber tracts, 18 were successfully tracked, including bilateral thalamic radiation, corticospinal tract, CGC, inferior fronto-occipital fasciculus, inferior longitudinal fasciculus, SLF, uncinate fasciculus, arcuate fasciculus, and callosum forceps major and minor. The segments of each fiber tract with significant differences between the two groups are shown in Table 2. Compared with the HC group, the DR group showed significantly reduced FA values in nodes 81–100 of the left CGC, increased MD values in nodes 39–50 of the left CGC, and reduced AD values in nodes 91–100 of left CGC (P<0.05, FDR corrected). The DR group also showed significantly increased AD values in the left SLF (nodes 1–23, 37–50, and 66–99) and the right SLF (nodes 1–36 and 79–100) compared with HCs (P<0.05, FDR corrected, Figures 1,2).
Table 2
DTI parameters | Tracts | HC group | DR group | Segments | t | P value (FDR) |
---|---|---|---|---|---|---|
FA | CGC_L | 0.468±0.066 | 0.412±0.069 | 81–100 | −2.801 | 0.008 |
MD | CGC_L | 0.743±0.071 | 0.802±0.090 | 39–50 | 2.748 | 0.009 |
AD | CGC_L | 1.151±0.057 | 1.068±0.072 | 91–100 | −3.129 | 0.006 |
SLF_L | 1.020±0.073 | 1.089±0.097 | 1–23, 37–50, 66–99 | 2.195 | 0.03 | |
SLF_R | 1.034±0.070 | 1.120±0.094 | 1–36, 79–100 | 2.423 | 0.02 |
Data are presented as mean ± standard deviation. DR, diabetic retinopathy; FDR, false discovery rate; HC, healthy control; CGC, cingulum cingulate; SLF, superior longitudinal fasciculus; L, left; R, right; FA, fractional anisotropy; MD, mean diffusion; AD, axial diffusion; DTI, diffusion tensor imaging.
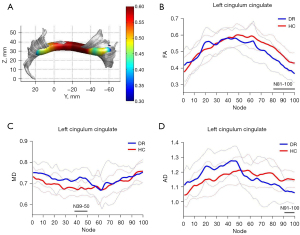

Results of correlation analysis
Correlation analysis of the altered DTI metrics in the segments of the left CGC and bilateral SLF and cognitive test scores, as well as clinical biochemical indicators in patients with DR revealed a positive correlation between FA values in nodes 82–98 of the left CGC and MoCA scores (r=0.760, P<0.05, Figure 3), and a positive correlation between the AD values in nodes 37–41 in the left SLF and HbA1c levels (r=0.559, P<0.05, Figure 4). No significant correlation was found between altered DTI metrics and FBG levels, as well as MMSE scores (P>0.05).
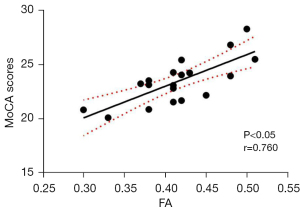
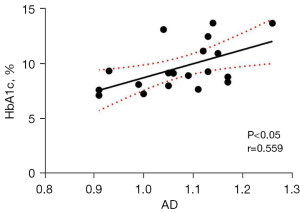
Discussion
In the present study, we applied the AFQ technique to study the alterations in the integrity of white matter fiber tracts in patients with DR and explored their correlation with cognitive impairment. The results showed that compared with the HC group, patients with DR showed significantly reduced FA and AD values, and increased MD values in nodes 81–100, 91–100, and 39–50 of the left CGC, respectively. They also showed increased AD values in left (nodes 1–23, 37–50, and 66–99) and right SLF (nodes 1–36 and 79–100). Correlation analysis showed that decreased FA values in the damaged segments of the left CGC were positively correlated with MoCA scores (r=0.760, P<0.05), and increased AD values in the damaged segments of the left SLF were negatively correlated with HbA1c levels. The use of AFQ technique allows accurate segmentation of the CGC and SLF into multiple nodes, and provides more precise localization of fiber tract damage, which has good prospects for application in central nervous system diseases. The FA value represents the compactness of white matter fibers and the integrity of myelin sheaths. Decreased FA values in the left CGC may reflect abnormal integrity of the fiber tract connecting the frontal lobe to the occipital, parietal, and temporal lobes. MD value reflects the degree of diffusion of water molecules in all directions, namely, the ability of water molecules to diffuse within tissues, which is sensitive to cell proliferation, edema, and necrosis (17). An increase in AD value indicates an increase in diffusion parallel to the fiber tract orientation, which can result in a decrease in axonal density and an increase in intra-axonal space, followed by disorganization of the axonal structure, suggesting axonal loss or loss of orientation coherence of axons in the fiber tract (18). A previous study demonstrated that decreased AD values indicated acute axonal damage, whereas increased AD values indicated axonal hyperplasia, reflecting chronic damage (19).
The CGC is the core part of the limbic system and a key structure of the Papez circuit (20). It is a main intrahemispheric pathway connecting the anterior cingulate cortex to the posterior cingulate cortex, which plays an important role in the processes of executive function, sensory function, emotion, memory, and spatial processing/integration (21). Numerous studies have shown that damage to the CGC was associated with symptoms of depression and cognitive impairment (22,23). Therefore, we hypothesized that altered FA, MD, and AD values in the left CGC may lead to a decrease in the connections between the anterior and posterior cingulate cortex, which interfere with the sensory, cognitive, or emotional regulation, thereby causing corresponding dysfunction in patients. The results of the present study showed that the damaged segments were mainly located in the middle and posterior parts of the left CGC in patients with DR. A previous study showed that decreased FA in the posterior part of the CGC was associated with functional impairment in several cognitive domains, including executive function, language, memory, and visuospatial processing (24). A voxel-based morphometric study demonstrated reduced gray matter volume in the left middle cingulate gyrus in patients with DR (25), and a resting-state functional MRI study reported deceased regional homogeneity value in the right anterior cingulate gyrus in patients with DR (26). These findings suggest that alterations of white matter integrity in the cingulate gyrus may lead to abnormalities in neuronal information transmission by the CGC, which in turn cause abnormal neural activity in the cingulate cortex, and ultimately result in corresponding functional impairment in patients with DR. Additionally, Shi et al. (27) reported that DR patients showed increased amplitude of low-frequency fluctuation in the anterior cingulate cortex, indicating that spontaneous neural activity in this brain region is enhanced. Our results are consistent with these findings, suggesting that if the capacity of the posterior part of the left CGC for information transmission is impaired, the brain compensates for this impaired information transmission pathway by increasing spontaneous neural activity in the anterior cingulate cortex. In the present study, correlation analysis of the FA values in the damaged segments of the left CGC and cognitive test scores in the DR group showed that the FA values in nodes 82–98 of the left CGC were positively correlated with the MoCA scores. However, no significant correlation was found between altered DTI metrics and MMSE scores. The reason may be due to the fact that MoCA is one of the most commonly used scales with high sensitivity and specificity for detecting mild cognitive impairment (28), whereas MMSE has high specificity and low sensitivity to identify mild cognitive disorders. In addition, the MoCA covers the cognitive domains of visuospatial and executive function, namely, memory, attention, language, abstraction, delayed recall, and orientation. Patients with DR generally had impaired visuospatial and executive function due to continuous impairment of visual function (29-31). Kantarci et al. (32) documented that FA values in the CGC of patients with Alzheimer’s disease were closely correlated with disease severity. So, these findings further corroborate our speculation that structural alterations in the CGC may underlie cognitive impairment in patients with DR, which may be potential imaging markers reflecting changes in cognitive function in these patients.
The SLF is an important white matter fiber tract that connects the frontal cortex to the occipital, parietal, and temporal cortices. It is mainly involved in regulating motor behavior, vision spatial attention, visuomotor function, transfer of somatosensory information between parietal and motor cortices, and language function, and plays an important role in the default mode network (33,34). In the present study, we found that when compared to HCs, patients with DR exhibited increased AD values in many segments of the bilateral SLF, and the damaged segments were mainly located in the anterior and posterior parts of the SLF. Since the white matter fiber tracts of the brain are carriers for information transmission during functional brain activity, abnormal changes in brain white matter structure can affect the transmission of neural information, which further affects the function of the corresponding damaged brain regions in patients. Several neuroimaging studies have demonstrated that patients with DR exhibited abnormal spontaneous neural activity, cerebral blood flow, and gray matter volume in brain regions connected by the SLF, such as the orbital part of middle frontal gyrus, middle occipital gyrus, superior temporal gyrus, and inferior parietal lobule (35-37). Chronic axonal injury in the anterior and posterior parts of the SLF can lead to abnormalities of neural information transmission, which in turn causes widespread functional and structural brain alterations from the frontal lobe to the parieto-temporo-occipital lobes. So, SLF may be a key target fiber tract for the occurrence of cognitive impairment and visual processing impairment in patients with DR. Huang et al. (38) documented decreased FA and increased RD values in nodes 16–21 of the right SLF in diabetic patients. Our results showed significantly increased AD values in nodes 1–36 and 79–100 of the right SLF, and nodes 1–23, 37–50, and 66–99 of the left SLF in patients with DR when compared with HCs. DR is considered the severe stage of diabetes. Long-term exposure to a hyperglycemic environment can lead to reduced transmission of related fiber bundle nutrient factors and abnormal neurometabolism, which eventually results in neuronal death. Therefore, during the transition from diabetes to DR, the location of damaged segments of the right SLF and their damage patterns would change accordingly, specifically, transition from unilateral SLF damage to bilateral SLF damage, and transition from decreased FA values and increased RD values in the unilateral SLF to increased AD values in the bilateral SLF. Since the white matter pathway of the SLF connects several important brain regions related to cognitive function, the above-mentioned changes in damage patterns of the SLF may also occur in brain regions connected by the SLF during progression from diabetes to DR. In future studies, these changes in the damage patterns of the SLF could be used to discriminate between patients with diabetes and DR, thus providing an important reference for the differential diagnosis and treatment of DR. Correlation analysis revealed a negative correlation between the AD values in nodes 37–41 of the left SLF and HbA1c levels, suggesting that the abnormal alterations in the segments of the SLF may be influenced by clinical indicators of long-term glycemic control, such as HbA1c levels, and HbA1c levels may also reflect the degree of damage to the SLF. HbA1c is an indicator for monitoring long-term glycemic control and has been considered correlated with cognitive function (7,39). The hyperglycemic environment tends to exacerbate cognitive impairment. These findings suggest that glycemic control is beneficial in delaying axonal injury in the SLF and improving neuronal information transmission, thus alleviating cognitive impairment in patients with DR.
There were some limitations to this study. First, due to the AFQ’s strict criteria for fiber tract identification, the left and right cingulum hippocampus were not identified in this study. Second, the selection of fiber tracking parameters (such fiber turning angle and termination criteria) during AFQ may influence the tracking results, because the fiber tracts may not follow the true information transmission pathway of the brain. Third, this was a cross-sectional study with a small sample size. Further large-sample longitudinal studies are needed to confirm the patterns of longitudinal changes in white matter fiber tract microstructure in patients with DR. Due to the limited number of cases, further clinical staging was not performed. Stratified studies with a larger sample size are warranted to further explore the correlation between the severity of DR and DTI parameters.
Conclusions
This study found alterations in the white matter fiber tracts at the point-wise level in patients with DR using AFQ analysis. Altered DTI metrics in the segments of white matter fiber tracts may be related to cognitive impairment in DR.
Acknowledgments
None.
Footnote
Reporting Checklist: The authors have completed the STROBE reporting checklist. Available at https://qims.amegroups.com/article/view/10.21037/qims-24-1440/rc
Funding: The study was supported by
Conflicts of Interest: All authors have completed the ICMJE uniform disclosure form (available at https://qims.amegroups.com/article/view/10.21037/qims-24-1440/coif). The authors have no conflicts of interest to declare.
Ethical Statement: The authors are accountable for all aspects of the work in ensuring that questions related to the accuracy or integrity of any part of the work are appropriately investigated and resolved. The study was conducted in accordance with the Declaration of Helsinki (as revised in 2013). The study was approved by the Ethics Committee of Taizhou People’s Hospital (No. KY2022-079-01) and informed consent was provided by all individual participants.
Open Access Statement: This is an Open Access article distributed in accordance with the Creative Commons Attribution-NonCommercial-NoDerivs 4.0 International License (CC BY-NC-ND 4.0), which permits the non-commercial replication and distribution of the article with the strict proviso that no changes or edits are made and the original work is properly cited (including links to both the formal publication through the relevant DOI and the license). See: https://creativecommons.org/licenses/by-nc-nd/4.0/.
References
- Ogurtsova K, da Rocha Fernandes JD, Huang Y, Linnenkamp U, Guariguata L, Cho NH, Cavan D, Shaw JE, Makaroff LE. IDF Diabetes Atlas: Global estimates for the prevalence of diabetes for 2015 and 2040. Diabetes Res Clin Pract 2017;128:40-50. [PubMed]
- Kollias AN, Ulbig MW. Diabetic retinopathy: Early diagnosis and effective treatment. Dtsch Arztebl Int 2010;107:75-83; quiz 84. [PubMed]
- Pearce I, Simó R, Lövestam-Adrian M, Wong DT, Evans M. Association between diabetic eye disease and other complications of diabetes: Implications for care. A systematic review. Diabetes Obes Metab 2019;21:467-78. [Crossref] [PubMed]
- Wong KH, Hu K, Peterson C, Sheibani N, Tsivgoulis G, Majersik JJ, de Havenon AH. Diabetic Retinopathy and Risk of Stroke: A Secondary Analysis of the ACCORD Eye Study. Stroke 2020;51:3733-6. [PubMed]
- Sundstrom JM, Hernández C, Weber SR, Zhao Y, Dunklebarger M, Tiberti N, Laremore T, Simó-Servat O, Garcia-Ramirez M, Barber AJ, Gardner TW, Simó R. Proteomic Analysis of Early Diabetic Retinopathy Reveals Mediators of Neurodegenerative Brain Diseases. Invest Ophthalmol Vis Sci 2018;59:2264-74. [PubMed]
- Ryan CM, Geckle MO, Orchard TJ. Cognitive efficiency declines over time in adults with Type 1 diabetes: effects of micro- and macrovascular complications. Diabetologia 2003;46:940-8. [Crossref] [PubMed]
- Wang ZL, Zou L, Lu ZW, Xie XQ, Jia ZZ, Pan CJ, Zhang GX, Ge XM. Abnormal spontaneous brain activity in type 2 diabetic retinopathy revealed by amplitude of low-frequency fluctuations: a resting-state fMRI study. Clin Radiol 2017;72:340.e1-7. [Crossref] [PubMed]
- Huang X, Tong Y, Qi CX, Dan HD, Deng QQ, Shen Y. Large-Scale Neuronal Network Dysfunction in Diabetic Retinopathy. Neural Plast 2020;2020:6872508. [Crossref] [PubMed]
- Wan S, Xia WQ, Zhong YL. Aberrant Interhemispheric Functional Connectivity in Diabetic Retinopathy Patients. Front Neurosci 2021;15:792264. [PubMed]
- Yeatman JD, Dougherty RF, Myall NJ, Wandell BA, Feldman HM. Tract profiles of white matter properties: automating fiber-tract quantification. PLoS One 2012;7:e49790. [PubMed]
- Chen H, Sheng X, Qin R, Luo C, Li M, Liu R, Zhang B, Xu Y, Zhao H, Bai F. Aberrant White Matter Microstructure as a Potential Diagnostic Marker in Alzheimer's Disease by Automated Fiber Quantification. Front Neurosci 2020;14:570123. [PubMed]
- Long X, Zhou Y, Zhang F, Li F, Wang X, Meng Y, Roberts N, Cheng B, Jia Z. Altered MRI Diffusion Properties of the White Matter Tracts Connecting Frontal and Thalamic Brain Regions in First-Episode, Drug-Naïve Patients With Postpartum Depression. J Magn Reson Imaging 2023;57:899-906. [PubMed]
- Xu F, Jin C, Zuo T, Wang R, Yang Y, Wang K. Segmental abnormalities of superior longitudinal fasciculus microstructure in patients with schizophrenia, bipolar disorder, and attention-deficit/hyperactivity disorder: An automated fiber quantification tractography study. Front Psychiatry 2022;13:999384. [PubMed]
- Jenkinson M, Beckmann CF, Behrens TE, Woolrich MW, Smith SM. FSL. Neuroimage 2012;62:782-90. [PubMed]
- Smith SM. Fast robust automated brain extraction. Hum Brain Mapp 2002;17:143-55. [Crossref] [PubMed]
- Jenkinson M, Pechaud M, Smith S. ET2: MR-based estimation of brain, skull and scalp surfaces. 2005.
- Zhou M, Hu Y, Huang R, Zhou Y, Xie X, Zhang S, Jia S, Zhang Y, Xue T, Dong F, Lu X, Yuan K, Yu D. Right arcuate fasciculus and left uncinate fasciculus abnormalities in young smoker. Addict Biol 2022;27:e13132. [PubMed]
- Douaud G, Jbabdi S, Behrens TE, Menke RA, Gass A, Monsch AU, Rao A, Whitcher B, Kindlmann G, Matthews PM, Smith S. DTI measures in crossing-fibre areas: increased diffusion anisotropy reveals early white matter alteration in MCI and mild Alzheimer's disease. Neuroimage 2011;55:880-90. [PubMed]
- Song SK, Sun SW, Ramsbottom MJ, Chang C, Russell J, Cross AH. Dysmyelination revealed through MRI as increased radial (but unchanged axial) diffusion of water. Neuroimage 2002;17:1429-36. [PubMed]
- Dillon DG, Gonenc A, Belleau E, Pizzagalli DA. Depression is associated with dimensional and categorical effects on white matter pathways. Depress Anxiety 2018;35:440-7. [PubMed]
- Bubb EJ, Metzler-Baddeley C, Aggleton JP. The cingulum bundle: Anatomy, function, and dysfunction. Neurosci Biobehav Rev 2018;92:104-27. [PubMed]
- Jiang Y, Liu Y, Gao B, Che Y, Lin L, Jiang J, Chang P, Song Q, Wang N, Wang W, Miao Y. Segmental Abnormalities of White Matter Microstructure in End-Stage Renal Disease Patients: An Automated Fiber Quantification Tractography Study. Front Neurosci 2021;15:765677. [PubMed]
- Zhang H, Li H, Yin L, Chen Z, Wu B, Huang X, Jia Z, Gong Q. Aberrant White Matter Microstructure in Depressed Patients with Suicidality. J Magn Reson Imaging 2022;55:1141-50. [Crossref] [PubMed]
- Bettcher BM, Mungas D, Patel N, Elofson J, Dutt S, Wynn M, Watson CL, Stephens M, Walsh CM, Kramer JH. Neuroanatomical substrates of executive functions: Beyond prefrontal structures. Neuropsychologia 2016;85:100-9. [Crossref] [PubMed]
- Xiao A, Ge QM, Zhong HF, Zhang LJ, Shu HY, Liang RB, Shao Y, Zhou Q. White Matter Hyperintensities of Bilateral Lenticular Putamen in Patients with Proliferative Diabetic Retinopathy: A Voxel-based Morphometric Study. Diabetes Metab Syndr Obes 2021;14:3653-65. [Crossref] [PubMed]
- Liao XL, Yuan Q, Shi WQ, Li B, Su T, Lin Q, Min YL, Zhu PW, Ye L, Shao Y. Altered brain actisvity in patients with diabetic retinopathy using regional homogeneity: a resting-state fMRI study. Endocr Pract 2019;25:320-7. [Crossref] [PubMed]
- Shi WQ, Zhang MX, Tang LY, Ye L, Zhang YQ, Lin Q, Li B, Shao Y, Yu Y. Altered spontaneous brain activity patterns in patients with diabetic retinopathy using amplitude of low-frequency fluctuation. World J Diabetes 2022;13:97-109. [PubMed]
- Hobson J. The Montreal Cognitive Assessment (MoCA). Occup Med (Lond) 2015;65:764-5. [PubMed]
- Li YM, Zhou HM, Xu XY, Shi HS. Research Progress in MRI of the Visual Pathway in Diabetic Retinopathy. Curr Med Sci 2018;38:968-75. [Crossref] [PubMed]
- Huang X, Wen Z, Qi CX, Tong Y, Shen Y. Dynamic Changes of Amplitude of Low-Frequency Fluctuations in Patients With Diabetic Retinopathy. Front Neurol 2021;12:611702. [Crossref] [PubMed]
- Huang X, Xie BJ, Qi CX, Tong Y, Shen Y. Abnormal intrinsic functional network hubs in diabetic retinopathy patients. Neuroreport 2021;32:498-506. [Crossref] [PubMed]
- Kantarci K, Murray ME, Schwarz CG, Reid RI, Przybelski SA, Lesnick T, Zuk SM, Raman MR, Senjem ML, Gunter JL, Boeve BF, Knopman DS, Parisi JE, Petersen RC, Jack CR Jr, Dickson DW. White-matter integrity on DTI and the pathologic staging of Alzheimer's disease. Neurobiol Aging 2017;56:172-9. [PubMed]
- Nakajima R, Kinoshita M, Shinohara H, Nakada M. The superior longitudinal fascicle: reconsidering the fronto-parietal neural network based on anatomy and function. Brain Imaging Behav 2020;14:2817-30. [Crossref] [PubMed]
- Joo SW, Kim H, Jo YT, Ahn S, Choi YJ, Park S, Kang Y, Lee J. White matter impairments in patients with schizophrenia: A multisite diffusion MRI study. Prog Neuropsychopharmacol Biol Psychiatry 2021;111:110381. [Crossref] [PubMed]
- Huang X, Wen Z, Tong Y, Qi CX, Shen Y. Altered resting cerebral blood flow specific to patients with diabetic retinopathy revealed by arterial spin labeling perfusion magnetic resonance imaging. Acta Radiol 2021;62:524-32. [Crossref] [PubMed]
- Qi CX, Huang X, Tong Y, Shen Y. Altered Functional Connectivity Strength of Primary Visual Cortex in Subjects with Diabetic Retinopathy. Diabetes Metab Syndr Obes 2021;14:3209-19. [Crossref] [PubMed]
- Lu X, Gong W, Wen Z, Hu L, Peng Z, Zha Y. Correlation Between Diabetic Cognitive Impairment and Diabetic Retinopathy in Patients With T2DM by (1)H-MRS. Front Neurol 2019;10:1068. [PubMed]
- Huang H, Ma X, Yue X, Kang S, Li Y, Rao Y, Feng Y, Wu J, Long W, Chen Y, Lyu W, Tan X, Qiu S. White Matter Characteristics of Damage Along Fiber Tracts in Patients with Type 2 Diabetes Mellitus. Clin Neuroradiol 2023;33:327-41. [Crossref] [PubMed]
- Xia W, Wang S, Sun Z, Bai F, Zhou Y, Yang Y, Wang P, Huang Y, Yuan Y. Altered baseline brain activity in type 2 diabetes: a resting-state fMRI study. Psychoneuroendocrinology 2013;38:2493-501. [Crossref] [PubMed]