Association of glymphatic system disturbance with neural dysfunction in amyotrophic lateral sclerosis
Introduction
Amyotrophic lateral sclerosis (ALS), the most common motor neuron disease affecting adults, is characterized by progressive degeneration of both the upper and lower motor neurons (1). Motor neuron degeneration destabilizes axonal projections and leads to axonal retraction and denervation of the target cell, resulting in motor function impairment (1). Spinal-onset ALS presents most commonly with unilateral distal muscle weakness and atrophy of the upper or lower limb muscles, whereas bulbar-onset ALS usually presents with dysarthria or dysphagia (2). ALS progresses rapidly, with a median survival time of 3 years after the first appearance of symptoms (3), and respiratory failure is the most common cause of death for patients with ALS (1). Unfortunately, disease-modifying treatments that are designed to slow ALS progression, such as riluzole and edaravone, have highly limited efficacy (1). Importantly, the etiopathogenesis of ALS remains poorly understood, which introduces serious challenges for the development of effective ALS therapeutics.
It is now recognized that the formation and accumulation of pathological inclusions composed of abnormal aggregated proteins in the brain is a key factor in multiple neurodegenerative diseases, including ALS (4). Transactive response DNA-binding protein 43 (TDP-43) is one such pathological protein; the ubiquitination and phosphorylation of TDP-43 and cytosolic TDP-43 mis-localization are pathological hallmarks of ALS (5,6). Studies have identified an increased concentration of TDP-43 in the cerebrospinal fluid (CSF) of patients with ALS, which serves as an indirect measure of TDP-43 levels in the interstitial and extracellular compartments of the central nervous system (7,8). In addition, cytosolic TDP-43 may enter the extracellular space via exosome-membrane fusion, passive diffusion, or release after cell death, all processes which are thought to be part of the cell-to-cell propagation mechanisms of TDP-43 (9). Given that the abundance of TDP-43 is closely correlated with the severity and progression of ALS (10-12), enhancing its clearance within the extracellular environment might be a potential therapeutic target. Notably, the clearance of neurotoxic proteins such as TDP-43 is associated with the flow of central glymphatic system (13).
It is commonly understood that since the brain lacks lymphatic vessels, the mechanisms that drive the clearance of neurotoxic proteins such as TDP-43 from the brain are predominantly associated with the ubiquitin-proteasome system and autophagy (13). In a recent study, the glymphatic system was found to also be involved in this process and may serve as a novel pathway for waste clearance in the brain (14). The glymphatic system is a recently discovered perivascular pathway of the brain involved in the exchange of CSF and interstitial fluid (ISF), facilitating the efficient clearance of solutes and waste from the brain (14). In addition to the elimination of waste such as lactate and neurotoxic proteins, the glymphatic system is also essential for the distribution of nonwaste compounds, including glucose, lipids, amino acids, growth factors, and neuromodulators, and is therefore critical for maintaining cerebral homeostasis (15).
Preliminary investigations of human brain glymphatic drainage employed dynamic contrast-enhanced magnetic resonance imaging (MRI) with intrathecal or intravenous administration of gadolinium-based contrast agents (16-18). However, it is worth noting that both routes are relatively invasive, and repeated administration of gadolinium-based contrast agents is linked with the deposition of gadolinium in the brain (19). The use of alternative MRI-based techniques have been proposed, particularly diffusion tensor imaging (DTI) analysis along the perivascular space (ALPS) (20), which may be a promising approach to the noninvasive evaluation of the central glymphatic system in humans. DTI-ALPS enables the indirect assessment of perivascular clearance activity in the human brain by calculating the diffusivity along the deep medullary vein at the level of the lateral ventricle body (20). Furthermore, the glymphatic function indirectly evaluated by DTI-ALPS has demonstrated a significant correlation with that measured by direct intrathecal tracer-based administration in humans (21). The ALPS index has been observed to decrease in various neurodegenerative disorders, such as multiple sclerosis (22), Alzheimer disease (23), frontal lobe epilepsy (24), and Parkinson disease (25). Altered glymphatic system function in ALS has been similarly demonstrated: one study based on dynamic contrast-enhanced MRI reported disrupted glymphatic clearance in a TDP-43 mouse model of ALS (26), and another study using DTI-ALPS reported glymphatic clearance dysfunction in early-stage patients with ALS (27).
Neural dysfunction, especially in the primary motor function and sensory function areas, is a key feature of ALS. For example, a resting-state electroencephalography (EEG) study revealed decreased spectral power and β-band synchrony in the sensorimotor region among patients with ALS, suggesting sensorimotor dysfunction (28). A task-based functional MRI study found significant hypoactivation in the primary sensorimotor cortex of patients with ALS when performing a simple handgrip motor task (29). In addition, resting-state functional MRI (rs-fMRI) studies have revealed abnormal neural function in patients with ALS. For example, several studies have used the amplitude of low-frequency fluctuations (ALFFs) algorithm to examine aberrant neural functional patterns in patients with ALS and found ALS to be characterized by reduced intensity of spontaneous neural activity in the primary sensorimotor cortex (30-32). Although studies in this area have identified neural functional abnormalities in ALS, the underlying mechanisms of the dysfunction remain unclear.
Within this context, the aims of this study were follows: (I) evaluate the severity of glymphatic system dysfunction in patients with ALS using DTI-ALPS analysis; (II) depict the pattern of neural dysfunction in sensorimotor-related areas in patients with ALS using the ALFF algorithm; (III) determine the association between glymphatic system dysfunction and neural functional impairment in patients with ALS; and (IV) analyze the diagnostic value of the ALPS index and ALFF for ALS. We present this article in accordance with the STROBE reporting checklist (available at https://qims.amegroups.com/article/view/10.21037/qims-24-1297/rc).
Methods
Participants
This cross-sectional study was conducted in accordance with the Declaration of Helsinki (as revised in 2013) and was approved by the Ethics Committee of Fujian Medical University Union Hospital (No. 2022WSJK022). All participants provided written informed consent. A total of 38 healthy controls (HCs) and 30 patients with sporadic ALS, matched by age, sex, and education level, were enrolled. ALS was diagnosed using the El Escorial criteria (33), and patients were required to have a definite or probable diagnosis. Disease severity was evaluated according to the revised ALS Functional Rating Scale (ALSFRS-R) (34). In addition, the disease duration was recorded, and the disease progression rate was calculated as follows: disease progression rate = (48 − ALSFRS-R score)/disease duration. Participant demographic and clinical characteristics are provided in Table 1. The exclusion criteria for this study were as follows: (I) codiagnosis of other neuropsychiatric disorders (e.g., multiple sclerosis, Parkinson disease, Alzheimer disease, schizophrenia, or bipolar disorder); (II) currently receiving treatment with psychoactive drugs (e.g., antipsychotics, antidepressants, or sedatives); (III) presence of other serious comorbidities (e.g., respiratory failure, heart failure, or malignancy); and (IV) any contraindications to MRI.
Table 1
Clinical and demographic characteristics | HC group (n=38) | ALS group (n=30) | P value |
---|---|---|---|
Age (years) | 52.4±8.2 | 51.2±11.4 | 0.621 |
Sex (female/male) | 10/28 | 10/20 | 0.531 |
Education (years) | 8.5±4.2 | 7.3±4.3 | 0.191 |
Diagnostic category (definite/probable) | – | 7/23 | – |
ALSFRS-R score | – | 38.3±7.0 | – |
Disease duration (months) | – | 10.2±6.2 | – |
Disease progression rate | – | 1.23±1.18 | – |
The disease progression rate was calculated as follows: disease progression rate = (48 − ALSFRS-R score)/disease duration. “–” denotes no data available. Continuous variables are presented as the mean ± standard deviation, and categorical variables are presented as counts. ALS, amyotrophic lateral sclerosis; ALSFRS-R, revised ALS Functional Rating Scale; HC, healthy control.
MRI data acquisition
All MR images were acquired using 3-T MRI scanner (MAGNETOM Prisma, Siemens Healthineers, Erlangen, Germany) with a 64-channel head coil. T1-weighted, rs-fMRI, and DTI images were acquired as follows: (I) the three-dimensional magnetization-prepared rapid gradient echo (MPRAGE) sequence was used for sagittal T1-weighted image acquisition [repetition time (TR) =1,610 ms, echo time (TE) =2.25 ms, field of view (FOV) =224 mm × 224 mm, matrix =224×224, flip angle =8°, slice thickness =1.0 mm (no interslice gap), slice number =176]. (II) The echo-planar imaging (EPI) sequence was used for axial rs-fMRI image acquisition [TR =700 ms, TE =30 ms, FOV =228 mm × 228 mm, matrix =76×76, flip angle =50°, slice thickness =3.0 mm (no interslice gap), slice number =48, multiband factor =4, volume number =600]. (III) The spin-echo echo-planar imaging (SE-EPI) sequence was used for axial DTI image acquisition [TR =2,500 ms, TE =81 ms, FOV =260 mm × 260 mm, matrix =130×130, flip angle =90°, slice thickness =2.0 mm (no interslice gap), slice number =72, multiband factor =4], with b values of 1,000, 2,000, and 3,000 s/mm2 and with 64, 64, and 64 gradient directions, respectively. Ten images with a b value of 0 were also acquired.
Processing of rs-fMRI data
Preprocessing of the rs-fMRI data was performed using Statistical Parametric Mapping software package version 12 (SPM12; https://www.fil.ion.ucl.ac.uk/spm/) and the Data Processing & Analysis of Brain Imaging toolbox (DPABI; https://rfmri.org/DPABI). Data were processed as follows: (I) removal of the first 30 volumes of rs-fMRI data from each participant; (II) head-motion correction [participants whose head motion exceeded 2° of rotation or 2 mm of displacement in any direction were excluded (35)]; (III) spatial normalization for motion-corrected functional images to the Montreal Neurological Institute (MNI) space via the diffeomorphic anatomical registration through exponentiated lie algebra (DARTEL) algorithm (36); (IV) spatial smoothing using a Gaussian filter with full-width at half-maximum (FWHM) kernel size of 4 mm; (V) linear detrending and regression of nuisance covariance (including white matter, CSF, global signals, and head motion parameters calculated using the Friston 24 model); and (VI) band-pass filtering (0.01–0.08 Hz).
After rs-fMRI data preprocessing, the ALFF values of each participant were calculated according to the method established by a previous study (37). For a given voxel, the filtered time course was converted to a frequency domain using a fast Fourier transform to acquire the power spectrum. Subsequently, each frequency of the power spectrum was square root-transformed at each voxel. The average square root of the frequency range of 0.01–0.08 Hz was taken as the ALFF value. Finally, all ALFF values were standardized by mean division.
According to a previous study (38), we defined the sensorimotor-related brain areas using the Human Brainnetome Atlas (39), including the left primary motor area (L_M1) and right primary motor area (R_M1), the left premotor cortex (L_PMC) and right premotor cortex (R_PMC), the left primary somatosensory area (L_S1) and right primary somatosensory area (R_S1), and the left supplementary motor area (L_SMA) and the right supplementary motor area (R_SMA). The averaged ALFF value within each sensorimotor-related area was computed and used in the subsequent statistical analysis.
Processing of diffusion MRI data and ALPS analysis
The DTI data were preprocessed with the FMRIB’s Software Library (FSL) (www.jiscmail.ac.uk/lists/fsl.html) (40). The head motion and distortion caused by eddy currents were corrected first. Subsequently, an open-source library diffusion in Python (DIPY) (41) was used for DTI image reconstruction. The diffusivity along the direction of the x- (Dxx), y- (Dyy), and z-axis (Dzz) was calculated. Additionally, fractional anisotropy color (FA-color) maps were derived.
The method for DTI-ALPS calculation, which was obtained from a previous study (20), is summarized in Figure 1. On the FA-color maps, the spherical regions of interest (ROIs) with a 6-mm diameter were manually drawn in the areas of the projection fibers and association fibers at the level of the lateral ventricle body in the left hemisphere, respectively. This process was conducted with blinding in respect to the clinical data and other images to maintain objectivity and prevent potential bias. Since the perivascular space direction is perpendicular to both the projection and association fibers, we could indirectly quantify the activity of the glymphatic system through the function of perivascular interstitial flows. The diffusivity within the projection fibers (Dxx_projection and Dyy_projection) and association fibers (Dxx_association and Dzz_association) were automatically derived for each individual to calculate the ALPS index according to the following equation (20):
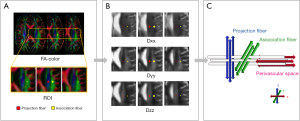
Statistical analysis
A two-sample t test and the Mann-Whitney test were used to compare data with normal and skewed distributions, respectively. The statistical significance of the between-group difference in ALPS, diffusivities, and ALFF was set as P<0.05 after false discovery rate (FDR) correction. Spearman correlation analysis was used to determine the association between ALPS, diffusivities, ALFF, and clinical assessments; results with a P value <0.05 were considered statistically significant. According to a standardized pipeline (42), the diagnostic performance of ALPS, diffusivities, and ALFF was evaluated via receiver operating characteristic (ROC) curve analysis using R software version 3.5.3 (The R Foundation for Statistical Computing), and the area under the curve (AUC) was calculated. Furthermore, the sensitivity and specificity determined according to the optimal cutoff values were calculated.
Results
The between-group differences in the ALPS and diffusivities indices are displayed in Figure 2 and Table 2. The ALPS index was lower in the patients with ALS than in HCs. Patients with ALS showed increased Dyy_association and Dyy_projection in comparison with HCs. There was no significant between-group difference for the other diffusivities, including Dxx_association, Dxx_projection, Dzz_association, and Dzz_projection.
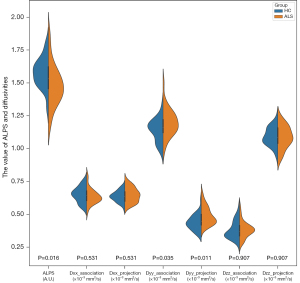
Table 2
ALPS and diffusivities indices | HC group, mean ± SD | ALS group, mean ± SD | P value (FDR-corrected) |
---|---|---|---|
ALPS (A.U.) | 1.579±0.113 | 1.483±0.156 | 0.016 |
Dxx_association (×10−3 mm2/s) | 0.650±0.059 | 0.636±0.058 | 0.531 |
Dxx_projection (×10−3 mm2/s) | 0.637±0.041 | 0.647±0.055 | 0.531 |
Dyy_association (×10−3 mm2/s) | 1.143±0.086 | 1.200±0.103 | 0.035 |
Dyy_projection (×10−3 mm2/s) | 0.438±0.058 | 0.489±0.068 | 0.011 |
Dzz_association (×10−3 mm2/s) | 0.381±0.068 | 0.383±0.061 | 0.907 |
Dzz_projection (×10−3 mm2/s) | 1.093±0.069 | 1.088±0.085 | 0.907 |
Dxx_association, Dyy_association, and Dzz_association represent the diffusivities of the x-axis, y-axis, and z-axis, respectively, within the ROI of association fiber bundles. Dxx_projection, Dyy_projection, and Dzz_projection represent the diffusivities of the x-axis, y-axis, and z-axis, respectively, within the ROI of projection fiber bundles. Diffusivities are presented as the apparent diffusion coefficients. ALPS, along the perivascular space; ALS, amyotrophic lateral sclerosis; A.U., arbitrary unit; FDR, false discovery rate; HC, healthy control; ROI, region of interest; SD, standard deviation; sec, second.
The between-group differences in the ALFF of sensorimotor-related brain areas are shown in Figure 3 and Table 3. Compared with HCs, patients with ALS showed decreased ALFF in the L_M1, R_M1, L_S1, R_S1, and L_SMA. No significant between-group difference was detected in the ALFF of the L_PMC, R_PMC, and R_SMA regions.
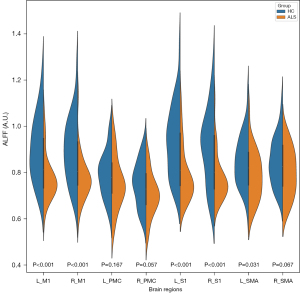
Table 3
ALFF | HC group, mean ± SD | ALS group, mean ± SD | P value (FDR-corrected) |
---|---|---|---|
L_M1 (A.U.) | 0.903±0.141 | 0.777±0.097 | <0.001 |
R_M1 (A.U.) | 0.899±0.140 | 0.776±0.097 | <0.001 |
L_PMC (A.U.) | 0.792±0.098 | 0.756±0.112 | 0.167 |
R_PMC (A.U.) | 0.752±0.096 | 0.704±0.092 | 0.057 |
L_S1 (A.U.) | 0.933±0.150 | 0.791±0.116 | <0.001 |
R_S1 (A.U.) | 0.911±0.158 | 0.777±0.100 | <0.001 |
L_SMA (A.U.) | 0.850±0.109 | 0.787±0.106 | 0.031 |
R_SMA (A.U.) | 0.849±0.103 | 0.800±0.104 | 0.067 |
ALFF, amplitude of low-frequency fluctuation; ALS, amyotrophic lateral sclerosis; AU, arbitrary unit; FDR, false discovery rate; HC, healthy control; L_M1, left primary motor area; L_PMC, left premotor cortex; L_S1, left primary somatosensory area; L_SMA, left supplementary motor area; R_M1, right primary motor area; R_PMC, right premotor cortex; R_S1, right primary somatosensory area; R_SMA, right supplementary motor area; SD, standard deviation.
The results of the correlation analysis are displayed in Figure 4. The ALPS index was positively correlated with ALFF in the L_M1 (r=0.402; P=0.028) and L_S1 (r=0.468; P=0.009). Dyy_association was negatively correlated with ALFF in the L_S1 (r=−0.397; P=0.030) and R_S1 (r=−0.406; P=0.026), and Dyy_projection was negatively correlated with ALFF in the L_M1 (r=−0.592; P=0.001), R_M1 (r=−0.392; P=0.032), and L_S1 (r=−0.398; P=0.029). In addition, ALPS was positively correlated with ALSFRS-R (r=0.367; P=0.046). ALFF in the L_M1 (r=0.365; P=0.048), R_M1 (r=0.435; P=0.016), L_S1 (r=0.431; P=0.017), and R_S1 (r=0.490; P=0.006) was positively correlated with ALSFRS-R. Moreover, ALFF in the L_SMA (r=0.496; P=0.005) was positively correlated with ALS disease duration.
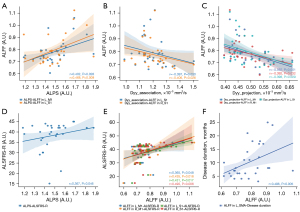
The results of ROC curve analysis are presented in Figure 5. The factors that demonstrated a moderate diagnostic value for ALS were the ALPS index (AUC =0.721; sensitivity =0.895; specificity =0.567; P=0.002) and Dyy_projection (AUC =0.710, sensitivity =0.553; specificity =0.800; P=0.003). Meanwhile, factors that demonstrated moderate diagnostic value for ALS included ALFF in the L_M1 (AUC =0.773; sensitivity =0.816; specificity =0.767; P<0.001), R_M1 (AUC =0.773, sensitivity =0.763, specificity =0.800; P<0.001), L_S1 (AUC =0.796, sensitivity =0.816, specificity =0.767; P<0.001), and R_S1 (AUC =0.747, sensitivity =0.711, specificity =0.833; P<0.001).
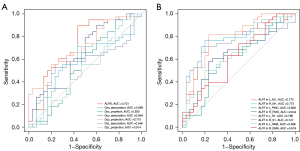
Discussion
This study investigated glymphatic system function in ALS and its association with neural dysfunction in sensorimotor-related brain areas. The principal findings are as follows: (I) the ALPS index of patients with ALS was lower, indirectly suggesting glymphatic system dysfunction. (II) Patients with ALS exhibited reduced ALFF in the bilateral M1 and S1 and L_SMA, indicating disease-related neural function impairment in sensorimotor-related areas. (III) ALPS and diffusivity indices were associated with ALFF in sensorimotor-motor regions, suggesting glymphatic system dysfunction and white matter integrity impairment are related to sensorimotor deficit. Moreover, ALPS and ALFF correlated with clinical assessments (i.e., ALSFRS-R and disease duration), which indicates that glymphatic system dysfunction and impairment of neural function are associated with disease severity and development. (IV) ROC analysis demonstrated that ALPS, diffusivities, and ALFF had a moderate ability to diagnose ALS, suggesting these indices could be used as potential biomarkers for ALS.
Additionally, we found glymphatic system dysfunction in patients with ALS as demonstrated by their reduced ALPS indices, which agrees with the findings of previous studies (26,27). A reduced ALPS index reflects decreased water diffusivity in the perivenous spaces, which could be an indication of altered glymphatic flow in general (including inflow) (43). The aquaporin-4 (AQP-4) water channel in astrocytic perivascular endfeet is crucial for the glymphatic system, aiding CSF-ISF exchange and interstitial solute clearance (14). One study reported the presence of AQP-4 dysfunction in ALS models, with increased expression in the brainstem and cortex, along with higher immunoreactivity in the facial and trigeminal nuclei and in the motor cortex (44). Additionally, in a mouse model, AQP-4 deficiency was found to lead to stagnant ISF flow and delayed waste clearance, suggesting that AQP-4 dysfunction may impair glymphatic function and contribute to ALS pathology (45).
The glymphatic system plays a crucial role in maintaining homeostasis within the central nervous system by incorporating vital functions such as waste clearance and nutrient delivery (15). A series of studies confirmed that after AQP-4 knockout or after inhibition of AQP-4 with drugs, the clearance of brain amyloid-β and tau proteins is significantly impaired due to the destruction of glymphatic function (14,46). Impairment in glymphatic flow may impede the efflux of neurotoxic proteins, leading to misfolded and aggregated TDP-43 inclusions in the brain, spinal cord, and motor neurons in patients with ALS (13). The aberrant accumulation of neurotoxic proteins results in cellular dysfunction, loss of synaptic connections, and brain damage (4). In addition, the glymphatic system is involved in the delivery of glucose in the brain, which is essential for maintaining the normal physiological function of neurons (47). Indeed, lowered cerebral glucose utilization in patients with ALS has been well documented (48,49). Defects in central nervous system glucose transport and metabolism are believed to result in reduced mitochondrial energy generation and increased oxidative stress, which is associated with the selective death of motor neurons in ALS (50). Therefore, it is not surprising that glymphatic system dysfunction could result in neural impairment in patients with ALS.
Previous rs-fMRI studies on patients with ALS have reported abnormal spontaneous neural function in sensorimotor-related brain areas, including decreased ALFF in the primary sensorimotor area and postcentral gyrus (30,32), in addition to regional homogeneity reduction in the precentral gyrus, postcentral gyrus, and supplementary motor cortex (51), which is consistent with our findings. Other research has found that glymphatic influx correlates positively with cortical delta power and negatively with cortical beta power in electroencephalogram recordings (52), suggesting that glymphatic system activity is related to neural activity. Thus, it is reasonable to conclude that glymphatic system function (as reflected by ALPS index) is associated with neural activity (as reflected by ALFF) in sensorimotor-related brain areas.
This study involved several noteworthy limitations which should be mentioned. First, we did not measure the concentration of TDP-43 in the CSF, restricting our examination of the association between central TDP-43 accumulation and glymphatic system function. Second, the sample size of this study was relatively small, which not only limited the statistical power, but also prevented us from investigating the effect of ALS heterogeneity on glymphatic function. In future studies, larger sample sizes would help capture the variability in glymphatic function between different ALS subgroups (e.g., based on disease stage and severity, site of onset, disease progression rate, and genotype). Additionally, multivariate analysis could be employed to eliminate the influence of these confounding factors on the research results. Third, the experimental design of this study was cross-sectional. A longitudinal design should be implemented in future studies to enable observations of the impact of disease progression on the glymphatic system. Fourth, sleep is one of the influencing factors of the glymphatic system, and sleep disturbance is a common event in patients with ALS (15,53), yet participants sleep status was not recorded in our study. Sleep assessment via the Pittsburgh Sleep Quality Index, Epworth Sleepiness Scale, and polysomnography should be carried out in future research to account for the effect of sleep as a potential confounding factor on glymphatic assessment (27). Finally, the ALPS index indirectly measures the glymphatic clearance function by calculating the diffusivity along the deep medullary vein at the level of the lateral ventricle body (20), which can only be achieved through a specific ROI and restricts the characterization of glymphatic activity on the whole-brain level. In future studies, fractional volume of free water in white matter, which calculates the fractional volume of free water in the brain parenchyma (i.e., brain ISF) from a bitensor DTI model (54), could be used to assess glymphatic CSF-ISF flow on the whole-brain level.
Conclusions
This study found impaired glymphatic system function in ALS, which may contribute to spontaneous neural activity disturbance and could constitute the underlying mechanism for the sensorimotor deficits frequently observed in patients with ALS. Glymphatic system function, as assessed by ALPS index, may serve as a potential diagnostic biomarker for ALS.
Acknowledgments
None.
Footnote
Reporting Checklist: The authors have completed the STROBE reporting checklist. Available at https://qims.amegroups.com/article/view/10.21037/qims-24-1297/rc
Funding: This study was supported by grants from
Conflicts of Interest: All authors have completed the ICMJE uniform disclosure form (available at https://qims.amegroups.com/article/view/10.21037/qims-24-1297/coif). The authors have no conflicts of interest to declare.
Ethical Statement: The authors are accountable for all aspects of the work in ensuring that questions related to the accuracy or integrity of any part of the work are appropriately investigated and resolved. This study was conducted in accordance with the Declaration of Helsinki (as revised in 2013) and was approved by the Ethics Committee of Fujian Medical University Union Hospital (No. 2022WSJK022). Written informed consent was obtained from each participant.
Open Access Statement: This is an Open Access article distributed in accordance with the Creative Commons Attribution-NonCommercial-NoDerivs 4.0 International License (CC BY-NC-ND 4.0), which permits the non-commercial replication and distribution of the article with the strict proviso that no changes or edits are made and the original work is properly cited (including links to both the formal publication through the relevant DOI and the license). See: https://creativecommons.org/licenses/by-nc-nd/4.0/.
References
- Hardiman O, Al-Chalabi A, Chio A, Corr EM, Logroscino G, Robberecht W, Shaw PJ, Simmons Z, van den Berg LH. Amyotrophic lateral sclerosis. Nat Rev Dis Primers 2017;3:17071. [Crossref] [PubMed]
- Masrori P, Van Damme P. Amyotrophic lateral sclerosis: a clinical review. Eur J Neurol 2020;27:1918-29. [Crossref] [PubMed]
- Couratier P, Corcia P, Lautrette G, Nicol M, Preux PM, Marin B. Epidemiology of amyotrophic lateral sclerosis: A review of literature. Rev Neurol (Paris) 2016;172:37-45. [Crossref] [PubMed]
- Soto C, Pritzkow S. Protein misfolding, aggregation, and conformational strains in neurodegenerative diseases. Nat Neurosci 2018;21:1332-40. [Crossref] [PubMed]
- Neumann M, Sampathu DM, Kwong LK, Truax AC, Micsenyi MC, Chou TT, Bruce J, Schuck T, Grossman M, Clark CM, McCluskey LF, Miller BL, Masliah E, Mackenzie IR, Feldman H, Feiden W, Kretzschmar HA, Trojanowski JQ, Lee VM. Ubiquitinated TDP-43 in frontotemporal lobar degeneration and amyotrophic lateral sclerosis. Science 2006;314:130-3. [Crossref] [PubMed]
- Ziff OJ, Neeves J, Mitchell J, Tyzack G, Martinez-Ruiz C, Luisier R, Chakrabarti AM, McGranahan N, Litchfield K, Boulton SJ, Al-Chalabi A, Kelly G, Humphrey J, Patani R. Integrated transcriptome landscape of ALS identifies genome instability linked to TDP-43 pathology. Nat Commun 2023;14:2176. [Crossref] [PubMed]
- Kasai T, Tokuda T, Ishigami N, Sasayama H, Foulds P, Mitchell DJ, Mann DM, Allsop D, Nakagawa M. Increased TDP-43 protein in cerebrospinal fluid of patients with amyotrophic lateral sclerosis. Acta Neuropathol 2009;117:55-62. [Crossref] [PubMed]
- Kasai T, Kojima Y, Ohmichi T, Tatebe H, Tsuji Y, Noto YI, Kitani-Morii F, Shinomoto M, Allsop D, Mizuno T, Tokuda T. Combined use of CSF NfL and CSF TDP-43 improves diagnostic performance in ALS. Ann Clin Transl Neurol 2019;6:2489-502. [Crossref] [PubMed]
- Smethurst P, Sidle KC, Hardy J. Review: Prion-like mechanisms of transactive response DNA binding protein of 43 kDa (TDP-43) in amyotrophic lateral sclerosis (ALS). Neuropathol Appl Neurobiol 2015;41:578-97. [Crossref] [PubMed]
- Brettschneider J, Del Tredici K, Toledo JB, Robinson JL, Irwin DJ, Grossman M, Suh E, Van Deerlin VM, Wood EM, Baek Y, Kwong L, Lee EB, Elman L, McCluskey L, Fang L, Feldengut S, Ludolph AC, Lee VM, Braak H, Trojanowski JQ. Stages of pTDP-43 pathology in amyotrophic lateral sclerosis. Ann Neurol 2013;74:20-38. [Crossref] [PubMed]
- Brettschneider J, Arai K, Del Tredici K, Toledo JB, Robinson JL, Lee EB, Kuwabara S, Shibuya K, Irwin DJ, Fang L, Van Deerlin VM, Elman L, McCluskey L, Ludolph AC, Lee VM, Braak H, Trojanowski JQ. TDP-43 pathology and neuronal loss in amyotrophic lateral sclerosis spinal cord. Acta Neuropathol 2014;128:423-37. [Crossref] [PubMed]
- Cykowski MD, Powell SZ, Peterson LE, Appel JW, Rivera AL, Takei H, Chang E, Appel SH. Clinical Significance of TDP-43 Neuropathology in Amyotrophic Lateral Sclerosis. J Neuropathol Exp Neurol 2017;76:402-13. [Crossref] [PubMed]
- Boland B, Yu WH, Corti O, Mollereau B, Henriques A, Bezard E, Pastores GM, Rubinsztein DC, Nixon RA, Duchen MR, Mallucci GR, Kroemer G, Levine B, Eskelinen EL, Mochel F, Spedding M, Louis C, Martin OR, Millan MJ. Promoting the clearance of neurotoxic proteins in neurodegenerative disorders of ageing. Nat Rev Drug Discov 2018;17:660-88. [Crossref] [PubMed]
- Iliff JJ, Wang M, Liao Y, Plogg BA, Peng W, Gundersen GA, Benveniste H, Vates GE, Deane R, Goldman SA, Nagelhus EA, Nedergaard M. A paravascular pathway facilitates CSF flow through the brain parenchyma and the clearance of interstitial solutes, including amyloid β. Sci Transl Med 2012;4:147ra111. [Crossref] [PubMed]
- Jessen NA, Munk AS, Lundgaard I, Nedergaard M. The Glymphatic System: A Beginner's Guide. Neurochem Res 2015;40:2583-99. [Crossref] [PubMed]
- Eide PK, Ringstad G. MRI with intrathecal MRI gadolinium contrast medium administration: a possible method to assess glymphatic function in human brain. Acta Radiol Open 2015;4:2058460115609635. [Crossref] [PubMed]
- Ringstad G, Vatnehol SAS, Eide PK. Glymphatic MRI in idiopathic normal pressure hydrocephalus. Brain 2017;140:2691-705. [Crossref] [PubMed]
- Naganawa S, Ito R, Kawai H, Taoka T, Yoshida T, Sone M. Confirmation of Age-dependence in the Leakage of Contrast Medium around the Cortical Veins into Cerebrospinal Fluid after Intravenous Administration of Gadolinium-based Contrast Agent. Magn Reson Med Sci 2020;19:375-81. [Crossref] [PubMed]
- Gulani V, Calamante F, Shellock FG, Kanal E, Reeder SBInternational Society for Magnetic Resonance in Medicine. Gadolinium deposition in the brain: summary of evidence and recommendations. Lancet Neurol 2017;16:564-70. [Crossref] [PubMed]
- Taoka T, Masutani Y, Kawai H, Nakane T, Matsuoka K, Yasuno F, Kishimoto T, Naganawa S. Evaluation of glymphatic system activity with the diffusion MR technique: diffusion tensor image analysis along the perivascular space (DTI-ALPS) in Alzheimer's disease cases. Jpn J Radiol 2017;35:172-8. [Crossref] [PubMed]
- Zhang W, Zhou Y, Wang J, Gong X, Chen Z, Zhang X, Cai J, Chen S, Fang L, Sun J, Lou M. Glymphatic clearance function in patients with cerebral small vessel disease. Neuroimage 2021;238:118257. [Crossref] [PubMed]
- Carotenuto A, Cacciaguerra L, Pagani E, Preziosa P, Filippi M, Rocca MA. Glymphatic system impairment in multiple sclerosis: relation with brain damage and disability. Brain 2022;145:2785-95. [Crossref] [PubMed]
- Kamagata K, Andica C, Takabayashi K, Saito Y, Taoka T, Nozaki H, Kikuta J, Fujita S, Hagiwara A, Kamiya K, Wada A, Akashi T, Sano K, Nishizawa M, Hori M, Naganawa S, Aoki SAlzheimer's Disease Neuroimaging Initiative. Association of MRI Indices of Glymphatic System With Amyloid Deposition and Cognition in Mild Cognitive Impairment and Alzheimer Disease. Neurology 2022;99:e2648-60. [Crossref] [PubMed]
- Zhou K, Peng S, Yao G, Luo Y, Li Q, Huang Y, Zhang Q, Deng L, Song Z, Wang W, Liu D, Liu Y. Association between glymphatic dysfunction and neurocognitive decline in patients with frontal lobe epilepsy. Quant Imaging Med Surg 2024;14:6745-55. [Crossref] [PubMed]
- Tian S, Hong H, Luo X, Zeng Q, Huang P, Zhang M. Association between body mass index and glymphatic function using diffusion tensor image-along the perivascular space (DTI-ALPS) in patients with Parkinson's disease. Quant Imaging Med Surg 2024;14:2296-308. [Crossref] [PubMed]
- Zamani A, Walker AK, Rollo B, Ayers KL, Farah R, O'Brien TJ, Wright DK. Impaired glymphatic function in the early stages of disease in a TDP-43 mouse model of amyotrophic lateral sclerosis. Transl Neurodegener 2022;11:17. [Crossref] [PubMed]
- Liu S, Sun X, Ren Q, Chen Y, Dai T, Yang Y, Gong G, Li W, Zhao Y, Meng X, Lin P, Yan C. Glymphatic dysfunction in patients with early-stage amyotrophic lateral sclerosis. Brain 2024;147:100-8. [Crossref] [PubMed]
- Dukic S, McMackin R, Buxo T, Fasano A, Chipika R, Pinto-Grau M, et al. Patterned functional network disruption in amyotrophic lateral sclerosis. Hum Brain Mapp 2019;40:4827-42. [Crossref] [PubMed]
- Cosottini M, Pesaresi I, Piazza S, Diciotti S, Cecchi P, Fabbri S, Carlesi C, Mascalchi M, Siciliano G. Structural and functional evaluation of cortical motor areas in Amyotrophic Lateral Sclerosis. Exp Neurol 2012;234:169-80. [Crossref] [PubMed]
- Li Q, Zhu W, Wen X, Zang Z, Da Y, Lu J. Different sensorimotor mechanism in fast and slow progression amyotrophic lateral sclerosis. Hum Brain Mapp 2022;43:1710-9. [Crossref] [PubMed]
- Sako W, Abe T, Izumi Y, Yamazaki H, Matsui N, Harada M, Kaji R. Spontaneous brain activity in the sensorimotor cortex in amyotrophic lateral sclerosis can be negatively regulated by corticospinal fiber integrity. Neurol Sci 2017;38:755-60. [Crossref] [PubMed]
- Luo C, Chen Q, Huang R, Chen X, Chen K, Huang X, Tang H, Gong Q, Shang HF. Patterns of spontaneous brain activity in amyotrophic lateral sclerosis: a resting-state FMRI study. PLoS One 2012;7:e45470. [Crossref] [PubMed]
- Brooks BR, Miller RG, Swash M, Munsat TLWorld Federation of Neurology Research Group on Motor Neuron Diseases. El Escorial revisited: revised criteria for the diagnosis of amyotrophic lateral sclerosis. Amyotroph Lateral Scler Other Motor Neuron Disord 2000;1:293-9. [Crossref] [PubMed]
- Cedarbaum JM, Stambler N, Malta E, Fuller C, Hilt D, Thurmond B, Nakanishi A. The ALSFRS-R: a revised ALS functional rating scale that incorporates assessments of respiratory function. BDNF ALS Study Group (Phase III). J Neurol Sci 1999;169:13-21. [Crossref] [PubMed]
- Chen HJ, Zou ZY, Zhang XH, Shi JY, Huang NX, Lin YJ. Dynamic Changes in Functional Network Connectivity Involving Amyotrophic Lateral Sclerosis and Its Correlation With Disease Severity. J Magn Reson Imaging 2021;54:239-48. [Crossref] [PubMed]
- Ashburner J. A fast diffeomorphic image registration algorithm. Neuroimage 2007;38:95-113. [Crossref] [PubMed]
- Zang YF, He Y, Zhu CZ, Cao QJ, Sui MQ, Liang M, Tian LX, Jiang TZ, Wang YF. Altered baseline brain activity in children with ADHD revealed by resting-state functional MRI. Brain Dev 2007;29:83-91. [Crossref] [PubMed]
- Liu J, Wang C, Qin W, Ding H, Guo J, Han T, Cheng J, Yu C. Corticospinal Fibers With Different Origins Impact Motor Outcome and Brain After Subcortical Stroke. Stroke 2020;51:2170-8. [Crossref] [PubMed]
- Fan L, Li H, Zhuo J, Zhang Y, Wang J, Chen L, Yang Z, Chu C, Xie S, Laird AR, Fox PT, Eickhoff SB, Yu C, Jiang T. The Human Brainnetome Atlas: A New Brain Atlas Based on Connectional Architecture. Cereb Cortex 2016;26:3508-26. [Crossref] [PubMed]
- Jenkinson M, Beckmann CF, Behrens TE, Woolrich MW, Smith SM. FSL. Neuroimage 2012;62:782-90. [Crossref] [PubMed]
- Garyfallidis E, Brett M, Amirbekian B, Rokem A, van der Walt S, Descoteaux M, Nimmo-Smith I. Dipy Contributors. Dipy, a library for the analysis of diffusion MRI data. Front Neuroinform 2014;8:8. [Crossref] [PubMed]
- Team RC. R: a language and environment for statistical computing, version 3.5. 5. Viennes: R Foundation for Statistical Computing. 2021. Available online: https://www.r-project.org/
- Gomolka RS, Hablitz LM, Mestre H, Giannetto M, Du T, Hauglund NL, Xie L, Peng W, Martinez PM, Nedergaard M, Mori Y. Loss of aquaporin-4 results in glymphatic system dysfunction via brain-wide interstitial fluid stagnation. Elife 2023;12:e82232. [Crossref] [PubMed]
- Bataveljić D, Nikolić L, Milosević M, Todorović N, Andjus PR. Changes in the astrocytic aquaporin-4 and inwardly rectifying potassium channel expression in the brain of the amyotrophic lateral sclerosis SOD1(G93A) rat model. Glia 2012;60:1991-2003. [Crossref] [PubMed]
- Hirose M, Asano M, Watanabe-Matsumoto S, Yamanaka K, Abe Y, Yasui M, Tokuda E, Furukawa Y, Misawa H. Stagnation of glymphatic interstitial fluid flow and delay in waste clearance in the SOD1-G93A mouse model of ALS. Neurosci Res 2021;171:74-82. [Crossref] [PubMed]
- Harrison IF, Ismail O, Machhada A, Colgan N, Ohene Y, Nahavandi P, Ahmed Z, Fisher A, Meftah S, Murray TK, Ottersen OP, Nagelhus EA, O'Neill MJ, Wells JA, Lythgoe MF. Impaired glymphatic function and clearance of tau in an Alzheimer's disease model. Brain 2020;143:2576-93. [Crossref] [PubMed]
- Lundgaard I, Li B, Xie L, Kang H, Sanggaard S, Haswell JD, Sun W, Goldman S, Blekot S, Nielsen M, Takano T, Deane R, Nedergaard M. Direct neuronal glucose uptake heralds activity-dependent increases in cerebral metabolism. Nat Commun 2015;6:6807. [Crossref] [PubMed]
- Van Laere K, Vanhee A, Verschueren J, De Coster L, Driesen A, Dupont P, Robberecht W, Van Damme P. Value of 18fluorodeoxyglucose-positron-emission tomography in amyotrophic lateral sclerosis: a prospective study. JAMA Neurol 2014;71:553-61. [Crossref] [PubMed]
- Matías-Guiu JA, Pytel V, Cabrera-Martín MN, Galán L, Valles-Salgado M, Guerrero A, Moreno-Ramos T, Matías-Guiu J, Carreras JL. Amyloid- and FDG-PET imaging in amyotrophic lateral sclerosis. Eur J Nucl Med Mol Imaging 2016;43:2050-60. [Crossref] [PubMed]
- Tefera TW, Steyn FJ, Ngo ST, Borges K. CNS glucose metabolism in Amyotrophic Lateral Sclerosis: a therapeutic target? Cell Biosci 2021;11:14. [Crossref] [PubMed]
- Bueno APA, Pinaya WHL, Rebello K, de Souza LC, Hornberger M, Sato JR. Regional Dynamics of the Resting Brain in Amyotrophic Lateral Sclerosis Using Fractional Amplitude of Low-Frequency Fluctuations and Regional Homogeneity Analyses. Brain Connect 2019;9:356-64. [Crossref] [PubMed]
- Hablitz LM, Vinitsky HS, Sun Q, Stæger FF, Sigurdsson B, Mortensen KN, Lilius TO, Nedergaard M. Increased glymphatic influx is correlated with high EEG delta power and low heart rate in mice under anesthesia. Sci Adv 2019;5:eaav5447. [Crossref] [PubMed]
- Boentert M. Sleep disturbances in patients with amyotrophic lateral sclerosis: current perspectives. Nat Sci Sleep 2019;11:97-111. [Crossref] [PubMed]
- Pasternak O, Sochen N, Gur Y, Intrator N, Assaf Y. Free water elimination and mapping from diffusion MRI. Magn Reson Med 2009;62:717-30. [Crossref] [PubMed]