Quantitative susceptibility mapping for Alzheimer’s disease, mild cognitive impairment, and normal aging: evaluation of corpus callosum
Introduction
Alzheimer’s disease (AD) is the most common neurological disorder leading to dementia, characterized by selective memory impairment and loss of executive function and judgment in its early stages (1). The pathology of AD includes diffuse extracellular amyloid-β (Aβ) plaques and intracellular neurofibrillary tangles, leading to progressive neuronal and synaptic loss and ultimately brain atrophy. Current histochemical and histopathological research has revealed altered iron metabolism and accumulation in AD brain tissues, with iron colocalizing with Aβ aggregates in senile plaques and intracellular hyperphosphorylated tau aggregates in neurofibrillary tangles (2).
Quantitative susceptibility mapping (QSM) is a non-invasive imaging technique that can detect magnetic susceptibility changes related to iron deposits (3). Many QSM studies have examined both gray matter nuclei detect iron accumulation in the deep gray matter in AD (4-6) and the cortex (7) in AD. It has shown promise as an early diagnostic tool for AD, with studies reporting increased cerebral iron in subcortical nuclei and cortical regions among individuals with mild cognitive impairment (MCI) and AD, particularly in the amygdala, caudate nucleus, and putamen of Alzheimer’s patients. Furthermore, this heightened susceptibility can help distinguish Alzheimer’s patients from healthy individuals in various cortical areas such as the precuneus and hippocampus (8-10). White matter primarily consists of myelin, which exhibits diamagnetic properties (negative susceptibility). An increase in white matter may result from increased iron, decreased myelin (demyelination), or both (11). In AD, the absolute diamagnetic susceptibility in gray matter is influenced by protein aggregation and demyelination. White matter in AD shows lower absolute diamagnetic susceptibility compared to controls, primarily due to demyelination (12).
The corpus callosum (CC) is a vital white matter structure in the brain that connects the two hemispheres and facilitates their communication. Its volume and microstructural changes have been reported in dementia patients, including those with AD. Specific regional atrophy of the CC may be associated with cognitive impairment and dementia severity in Alzheimer’s patients, particularly in the genu and splenium regions (13). Reduced white matter density and fractional anisotropy (FA), and increased mean diffusivity (MD) at the level of isthmus/splenium of the CC, were also significantly related to increased global cognitive deterioration during the early course of AD (14).
Overall, QSM has provided valuable insights into the role of iron accumulation in AD and has the potential to be used as a biomarker for monitoring disease progression and evaluating new therapies. However, further research is needed to validate QSM as a reliable and sensitive tool for measuring iron in the brain and to better understand the relationship between iron and the pathophysiology of AD.
An animal study has demonstrated significant susceptibility changes in the posterior parts of the CC compared to normal controls in primate model (15). In contrast, another study revealed differences in magnetic susceptibility in the rostral CC of a mouse model of tauopathy compared to a normal mouse (16). However, there have been no reported QSM studies in humans to validate these findings yet, particularly those that segment the CC into several regions.
The aim of this study was to measure susceptibility on QSM in AD, MCI, and normal aging in each part of the CC and assess their associations with neurocognitive scores and the change in susceptibility in follow-up imaging. We present this article in accordance with the STROBE reporting checklist (available at https://qims.amegroups.com/article/view/10.21037/qims-24-319/rc).
Methods
Patients
This retrospective study, approved by the Siriraj Institutional Review Board (SIRB) (COA: Si009/2022) and conducted in accordance with the Declaration of Helsinki (as revised in 2013), included three participant groups: patients who had been visited Siriraj Hospital diagnosed with AD or MCI, and healthy control (HC) from March 2015 to August 2021. Informed consent was provided by all participants. National Institute of Neurological and Communicative Disorders and Stroke and the Alzheimer’s Disease and Related Disorders Association (NINCDS-ADRDA) criteria, the guideline was used to classify AD as well as clinical scores were used to evaluate subjects including Thai Mental State Examination (TMSE) score (17) and Clinical Dementia Rating (CDR) scores (18). The TMSE, a Thai version of the Mini-Mental State Examination (MMSE), quantifies cognitive impairment and evaluates functions including memory, attention, language, and spatial orientation based on scale of 0–30, while CDR score based on a scale of 0–3: ranking no dementia to severe cognitive impairment. The AD group consisted of 34 patients diagnosed with probable AD according to the NINCDS-ADRDA criteria, with a TMSE score of less than 26 and a CDR score of 0.5 or greater. Patients with psychiatric or other neurological illnesses were excluded. 32 clinically-diagnosed AD patients have amyloid PET with the results of 25 positive scan. The MCI group included 32 patients with a TMSE score between 24 and 30, a CDR score of 0.5, subjective memory complaints with preserved activities of daily living (ADLs), and no dementia according to the NINCDS-ADRDA criteria. The HC group comprised 29 subjects with TMSE scores between 24 and 30, a CDR score of 0, no neurological or psychiatric illnesses, and normal ADLs. A subset of participants underwent follow-up magnetic resonance imaging (MRI), including 13 AD patients, 14 MCI patients, and 14 HC cases.
Clinical features
Retrospective review of electronically stored clinical notes for all participants was conducted to collect information on sex, age, educational years, and neurocognitive scores (TMSE and CDR, including CDR-global and CDR-sum of box).
Image acquisition
All imaging data were obtained using a 3-T MR system (Ingenia, Philips Medical System, Best, the Netherlands) with a 32-channel head coil. T1-weighted imaging was acquired using three-dimensional (3D)-turbo field echo (TFE) with parameters such as an echo time (TE) of 4.8 ms, a repetition time (TR) of 9.8 ms, a voxel size of 0.65×0.65×0.65 mm3, and a field-of-view (FOV) of 230×230×265 mm3. The total acquisition time was six minutes and four seconds. SWI/Phase images were performed using FFE with TEs of 8, 16, 24, and 32 ms; a TR of 36 ms; a voxel size of 0.4×0.4×1 mm3; bandwidth =181 Hz/pixel; flip angle =24°; readout direction = LR and a field-of-view (FOV) of 230×230×140 mm3. The FOV was angulated 3–5° to B0 field.
Image processing
Structural MRI data (3D T1 images) were processed using the recon-all command from the FreeSurfer software version 6.0, which automatically segmented the CC into five parts: anterior, mid-anterior, central, mid-posterior, and posterior, as demonstrated in Figure 1.
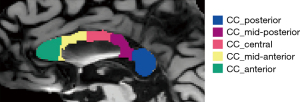
QSM processing was performed using the STI Suite, (http://people.eecs.berkeley.edu/~chunlei.liu/software.html), a software package that includes brain extraction, phase unwrapping, background field removal, and dipole inversion with the improved sparse linear equation and least squares (iLSQR) algorithm. QSM images were generated from 3D multi-echo gradient echo sequence with short TE for detecting magnetic susceptibility with strong signal and long TEs for weak susceptibilities (Figure 2). There are four steps to create QSM data from magnitude and phase images, including brain extraction using BET (in FSL), phase unwrapping, background field removal, and dipole inversion. These steps were performed in STISuite 3.0 by running them in a matlab script (MATLAB version R2021a, The Math Works, Natick, MA, USA). As shown in Figure 3, a binary brain mask is needed first to determine the area of interest which had a proper signal-to-noise ratio (SNR). Then, 3D unwrapped phase using Laplacian phase unwrapping was performed in the STISuite toolbox (19). For phase processing between −π and π, the algorithm can remove the discontinuities (20). The next step, background field removal, aimed to eliminate the background filed originating outside a region of interest such as air-tissue interface. A 3D varying spherical kernel of the sophisticated harmonic artifact reduction for phase data (V-SHARP) method was chosen to deliver accurate background field corrections and harmonic background phase removal using Laplacian, namely the iHARPERELLA method (21,22). This study used the spherical mean value (SMV) size with a default value of 12 and the iteration number of 40 for all computations. Finally, iLSQR algorithms were employed to generate QSM image of the whole brain. A range of magnetic susceptibility value and voxel-based QSM would be observed between −0.20 and 0.20 ppm for assessing intrinsic magnetic tissue properties (22).
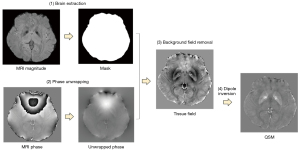
Subsequently, the QSM images were registered to 3D T1-weighted images using FSL (FMRIB Software Library, version 5.0.7), and their susceptibility was calculated within each segment of the CC using FSL’s Linear Image Registration Tool (FLIRT) and fslstats commands, as shown in Figure 4.
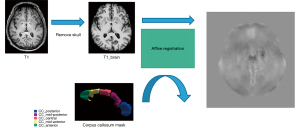
Statistics
Data were prepared and analyzed using IBM SPSS Statistics for Windows, version 26.0 (IBM Corp, Armonk, NY, USA). Demographic data, including sex, age, education years, TMSE, CDR, and QSM data from each part of the CC, were tested for normal distribution using the Kolmogorov-Smirnov test. Data with a normal distribution including demographic data, including sex, age, education years, TMSE, and CDR are reported as mean ± standard deviation (SD). Susceptibility in each region which has non-normal distribution was assessed using the Kruskal-Wallis test, with median values reported alongside minimum and maximum values. The correlation between susceptibility and neurocognitive scores was evaluated using Spearman’s rank correlation coefficient after performing a semi-partial correlation analysis on these scores and age to minimize the effect of aging. Mixed-analysis of variance (ANOVA) was also performed with susceptibility value as dependent variable, Group (HC, MCI, AD) as within subject effect and clinical scores (TMSE, CDR-global, CDR-sum of box) to clarify the effect of each variable. Susceptibility differences after follow-up were assessed using paired samples t-test and one-way ANOVA. All results were predetermined to be statistically significant if the P value was less than 0.05. Corrections for multiple comparisons used a false discovery rate (FDR) threshold of 0.05.
Results
Demographic data
Baseline demographic data for patients with AD and controls and clinical scores are presented in Table 1. AD patients had significantly lower TMSE scores, higher CDR-global, and higher CDR-sum of box. There was a trend towards higher age (P<0.001) in the AD patients compared to the other two groups, but no significant difference with regard to gender distribution and educational years (P=0.626 and 0.058, respectively).
Table 1
Participant characteristics | AD (n=34) | MCI (n=32) | HC (n=29) | P |
---|---|---|---|---|
Sex (M/F) | 16/18 | 16/16 | 11/18 | 0.626 |
Age (years) | 71.71±6.16 | 68.59±5.45 | 64.51±8.20 | <0.001 |
Education years | 11.32±4.26 | 13.41±3.89 | 12.53±4.30 | 0.058 |
TMSE | 22.59±4.3 | 26.53±2.61 | 28.07±1.69 | <0.001 |
CDR | <0.001 | |||
0 | 0 | 2 | 29 | |
0.5 | 20 | 29 | 0 | |
1 | 12 | 1 | 0 | |
1.5 | 0 | 0 | 0 | |
2 | 1 | 0 | 0 | |
CDR-sum of box | 1.19±1.96 | 1.44±2.14 | 1.46±1.57 | <0.001 |
Data are presented as number or mean ± SD. AD, Alzheimer’s disease; CDR, Clinical Dementia Rating; F, female; HC, healthy control; M, male; MCI, mild cognitive impairment; SD, standard deviation; TMSE, Thai Mental State Examination.
Susceptibility in each part of CC among the groups
The magnetic susceptibility varied across different regions of the CC in each group, as demonstrated in Table 2. The intercomparisons of susceptibility among the groups in each part of the CC were performed, revealing significantly increased susceptibility in the mid-anterior and central parts of the CC for Alzheimer’s patients [0.051 (−0.386, 0.170) and 0.103 (−0.374, 0.291) ppm, respectively] compared to those of normal controls [−0.014 (−0.246, 0.136) and 0.003 (−0.157, 0.206) ppm, respectively].
Table 2
Corpus callosal parts | Susceptibility (ppm) | P | |||||
---|---|---|---|---|---|---|---|
HC (n=34) | MCI (n=32) | AD (n=29) | HC vs. MCI | MCI vs. AD | HC vs. AD | ||
Anterior | −0.051 (−0.318, 0.208) | −0.069 (−0.273, 0.290) | −0.027 (−0.296, 0.123) | 0.851 | 0.516 | 0.389 | |
Mid-anterior | −0.014 (−0.246, 0.136) | 0.024 (−0.211, 0.300) | 0.051 (−0.386, 0.170) | 0.121 | 0.361 | 0.014* | |
Central | 0.003 (−0.157, 0.206) | 0.065 (−0.119, 0.286) | 0.103 (−0.374, 0.291) | 0.035 | 0.636 | 0.009* | |
Mid-posterior | 0.039 (−0.073, 0.135) | 0.045 (−0.091, 0.227) | 0.062 (−0.394, 0.194) | 0.204 | 0.789 | 0.131 | |
Posterior | 0.015 (−0.067, 0.085) | 0.013 (−0.031, 0.222) | −0.001 (−0.461, 0.125) | 0.188 | 0.375 | 0.023 |
Susceptibility is assessed using Kruskal-Wallis test. Data are presented as median (minimal, maximal) values. *, significance following correction for multiple comparisons (FDR threshold of 0.05). Adjusted P values are reported in the table. AD, Alzheimer’s disease; CC, corpus callosum; FDR, false discovery rate; HC, healthy control; MCI, mild cognitive impairment.
No other regions showed significant differences in susceptibility among the control, AD, and MCI groups. However, there was a slight increase in susceptibility in the central part of the CC in the MCI group compared to the HC group, with susceptibility of 0.003 (−0.157, 0.206) and 0.065 (−0.119, 0.286) ppm, respectively, and a P value of 0.035. This difference did not survive the multiple comparison analysis, which is also the case for the differences in susceptibility in the posterior CC between the HC and AD groups. The susceptibilities were 0.015 (−0.067, 0.085) ppm for HC and −0.001 (−0.461, 0.125) ppm for AD in the posterior part with P values =0.023 (Table 2).
Change in susceptibility in each part of CC during follow-up imaging
Following a 2-year follow-up, the susceptibility exhibited significant increases that varied across each group, as described in Table 3 and Figure 5. In the HC group, significant increases were observed in the mid-anterior region (mean difference =0.074 ppm; P value =0.021). In contrast, the MCI group displayed significant increases in susceptibility in the mid-posterior region (mean difference =0.081 ppm; P value =0.039). For the AD group, significant increases in susceptibility were found in the mid-posterior region and the posterior CC (mean difference =0.021 and 0.086 ppm; P value =0.013 and 0.005, respectively).
Table 3
CC part | HC (n=14) | MCI (n=14) | AD (n=13) | |||||||||||
---|---|---|---|---|---|---|---|---|---|---|---|---|---|---|
Baseline | Follow-up | Mean diff. | P | Baseline | Follow-up | Mean diff. | P | Baseline | Follow-up | Mean diff. | P | |||
Anterior | −0.066 | −0.038 | 0.027 | 0.317 | −0.028 | −0.081 | −0.053 | 0.279 | −0.02 | −0.038 | 0.036 | 0.435 | ||
Mid-anterior | −0.053 | 0.021 | 0.074 | 0.021* | 0.014 | 0.089 | 0.075 | 0.081 | 0.011 | 0.045 | −0.018 | 0.369 | ||
Central | −0.017 | 0.051 | 0.068 | 0.069 | 0.052 | 0.161 | 0.109 | 0.09 | 0.039 | 0.06 | 0.034 | 0.642 | ||
Mid-posterior | −0.003 | 0.049 | 0.052 | 0.095 | 0.034 | 0.116 | 0.081 | 0.039* | −0.012 | 0.075 | 0.021 | 0.013* | ||
Posterior | 0.010 | 0.03 | 0.02 | 0.242 | 0.029 | 0.045 | 0.016 | 0.394 | −0.045 | 0.042 | 0.086 | 0.005* |
*, significance following correction for multiple comparisons (FDR threshold of 0.05). Adjusted P values are reported in the table. AD, Alzheimer’s disease; CC, corpus callosum; diff., difference; FDR, false discovery rate; HC, healthy control; MCI, mild cognitive impairment.
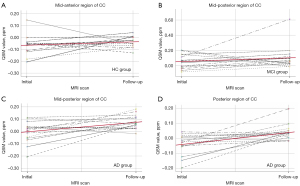
Correlations between susceptibility of CC and neurocognitive score
As depicted in Table 4 and Figure 6, our findings reveal that the susceptibility in the mid-anterior and central regions of the CC displayed a significant weakly positive correlation with the CDR-global (r=0.296, P=0.006 and r=0.287, P=0.005). No significant correlation between susceptibility of the CC in each region and TMSE or CDR SB was observed. The mixed-ANOVA test was also performed to confirm the main effect and between-subject effect of susceptibility value. There was a difference of susceptibility value in each region [F(4,248) =14.033, P<0.001, µp2 =0.185] without significant effect of susceptibility group (P>0.05). The between-subject effect analysis shows CDR-global score significantly associated with susceptibility value [F(1,62) =4.176, P=0.045, µp2 =0.063], as shown in Tables S1,S2.
Table 4
CC part | Total TMSE | CDR-global | CDR-sum of box | |||||
---|---|---|---|---|---|---|---|---|
Correlation coefficient (r) | P | Correlation coefficient (r) | P | Correlation coefficient (r) | P | |||
Anterior | −0.127 | 0.220 | 0.042 | 0.696 | −0.112 | 0.365 | ||
Mid-anterior | −0.099 | 0.341 | 0.296 | 0.006* | 0.071 | 0.564 | ||
Central | −0.124 | 0.233 | 0.287 | 0.005* | 0.068 | 0.581 | ||
Mid-posterior | 0.044 | 0.675 | 0.185 | 0.081 | 0.076 | 0.540 | ||
Posterior | 0.106 | 0.308 | 0.072 | 0.497 | −0.077 | 0.531 |
The correlation coefficients presented in this table are based on the analysis of Spearman’s rank correlation after performing a semi-partial correlation analysis on these scores and age to minimize the effect of aging. Statistically significant correlation is denoted by an asterisk (*) with significance level set at 0.05. CC, corpus callosum; CDR, Clinical Dementia Rating; TMSE, Thai Mental State Examination.
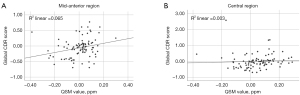
Discussion
Our study provides a detailed analysis of the CC’s susceptibility in AD, MCI, and healthy aging. We found that the findings specific to certain subregions of the CC correlate with diagnosis, cognitive function, and disease progression, using cross-sectional and longitudinal data.
Susceptibility in each part of CC among the groups
QSM is believed to provide novel ultrastructural data on white matter alterations. A previous QSM study has shown that myelinated white matter tracts exhibit low susceptibility values due to the diamagnetic property of myelin, while demyelination is associated with increased susceptibility (23). This is similar to reported microstructural alterations in diffusion tensor imaging (DTI) studies that reflect pathological changes such as axon loss, damage, or demyelination (24). To some extent, QSM and DTI could provide similar information regarding certain white matter changes. For instance, a study in Parkinson’s disease showed only some areas of overlap between QSM and DTI changes, such as an increase in mean susceptibility and radial diffusion (25).
Microstructural changes in the CC have been detected in many DTI studies in AD, with decreased FA and increased MD, in addition to other white matter regions, particularly in the cingulum bundles (26-29). For subsegmental regions of the CC, predominant atrophy and reduced microstructural integrity in the posterior part, especially the posterior body, isthmus, and splenium, have been noted in patients with AD (14). However, QSM studies have observed increased magnetic susceptibilities in the medial temporal lobes’ gray matter and the genu, body, and splenium of the CC in the white matter (30). Findings from animal models of sporadic AD have shown increased susceptibility in the posterior parts of the CC (15).
Despite prior findings tending to specify the posterior part, our study revealed significantly increased susceptibility in the mid-anterior and central parts of the CC in Alzheimer’s patients compared to normal controls, which could demonstrate the demyelination process. However, from a tau mouse model, susceptibility differences were found in the rostral CC, which had low to intermediate neurofibrillary tangle content and active inflammation during the intermediate stages of the disease. No significant differences in susceptibilities were found in the advanced stages where extensive myelin breakdown occurs in the posterior part of the CC (16).
Our study revealed significantly increased susceptibility in the mid-anterior and central parts of the CC specifically in Alzheimer’s patients compared to normal controls. The significantly increased susceptibilities in these regions may represent active areas of neurodegeneration with some level of neurofibrillary tangle content, and the lack of differences in susceptibilities in the posterior part could be due to the advanced stage of the disease, like findings in the tau mouse study. Further investigation is needed to validate these theories, such as assessing volumetric or microstructural changes or correlating the findings with histopathologic results.
Change of susceptibility in each part of CC among the groups during follow-up imaging
Longitudinal changes in the magnetic susceptibility (QSM value) of the CC have not been well studied in AD, MCI, and HC. While a previous study has observed increases in magnetic susceptibility over time in an amyloid mouse model of AD compared to controls (31), the specific changes in the CC have not been investigated.
In our study, we performed a 2-year follow-up of participants in each group (HC, MCI, and AD) and found significant increases in susceptibilities that varied by region. HCs exhibited significant increases in the mid-anterior region, while the MCI group showed significant increases in the mid-posterior region. The AD group displayed significant increases in the mid-posterior region and the posterior CC. These changes could represent an anteroposterior progression of the disease, with changes in the anterior regions potentially occurring in the early stages of amnestic MCI/early AD and then in the posterior part in the latter stage of the disease.
In normal aging, the most predominant finding is a decline in size and microstructural integrity in the anterior parts of the CC (32-36), which has been substantiated by postmortem findings (37,38). Additionally, an anterior-to-posterior gradient in fiber integrity has been observed in the CC, where anterior bundles are more vulnerable to age-related degeneration than posterior ones. This has been shown through various analytical methods, including histological examination and high-gradient diffusion MRI (39-48).
In microstructural studies using DTI for MCI and AD patients, increased axial diffusivity in the temporal subregion and increased radial diffusivity in the frontal and premotor/supplementary motor area subregions of the CC were observed. As the disease advances to AD dementia, both axonal damage and myelin degradation spread throughout the CC (47-51). Aligned with volume studies in MCI and early AD, atrophy is more pronounced than in normal aging and tends to affect the anterior region (genu) of the CC to a greater extent (52,53). In more severe or later stages of AD, the atrophy progresses to the posterior part of the CC. It is also thought that atrophy in these regions may serve as an early indicator of the progression from MCI to AD (54,55).
These results suggest that longitudinal QSM studies may be useful in differentiating between AD, MCI, and healthy aging subjects by examining the pattern involvement of the CC. Furthermore, the QSM changes observed in this study may provide valuable information on the progression of cognitive decline and offer potential biomarkers for disease diagnosis and monitoring.
Correlations between susceptibility of CC and neurocognitive score
Previous studies have indicated a connection between elevated brain iron levels, particularly in the presence of beta-amyloid, and lower cognitive performance in individuals with MCI or dementia (56,57). However, a study revealed elevated cerebral iron load found to be associated with lower cognitive performance, independent of beta-amyloid (57). A negative correlation was observed between brain iron levels in the hippocampus, frontal cortex, and globus pallidus, and global cognitive performance (57). However, there has been no reported information about the susceptibility of the CC in this context.
In our study, a weak correlation was observed between the susceptibility in specific regions of the CC in the mid-anterior and central parts, and the CDR-global scores. In addition, there was a correlation between CDR-global scores and Susceptibility across the entire CC. These results suggest that the CC plays a crucial role in cognitive abilities, as measured by changes in susceptibility within the CC may contribute to dementia and age-related cognitive decline.
Considering the projections of the anterior and mid-anterior CC regions to the frontal lobes, the increased susceptibility in these regions may indicate pathological changes that could lead to cognitive impairments, as seen in results resembling those from microstructural change and DTI tractography studies. These studies demonstrated connectivity of the anterior callosal regions with frontal cortical areas and posterior callosal regions with parietal and occipital cortical areas, along with associated brain-behavioral links to cognitive, motor, and sensory functions (58,59).
This hypothesis is also supported by findings from volumetric study that anterior-predominant CC atrophy is associated with cognitive dysfunction in normal aging (52) and in diseases characterized by cognitive deficits, such as AD, amnestic MCI, vascular dementia, and multiple sclerosis (48,52,59,60). These deficits include worse MMSE scores (60), verbal fluency (52), attention, executive function, processing speed (59,60), working memory (61), and global cognition (52).
Several limitations in this study are needed to be addressed. One limitation of our study is our small sample size with a small number of patients at follow-up imaging, requiring further validation in future studies. Additionally, not all participants received biomarker confirmation, a limitation given the reliance on the NINCDS-ADRDA criteria for diagnosing AD. The absence of biomarkers like amyloid and tau proteins could impact the accuracy of our diagnoses, potentially confounding distinctions between Alzheimer’s and other dementias. Future research should incorporate these biomarkers to enhance diagnostic precision.
Moreover, QSM shows promise as a potential imaging biomarker in AD. Based on prior studies the ATN system (62), magnetic susceptibility in deep gray matter may be a biomarker for AD pathogenesis and has some evidence of correlation to amyloid pathology, Tau pathology, and neurodegeneration, particularly detecting early tau pathological changes (10). Several clinicoradiological studies have investigated controversial relationship between iron and Aβ deposition as detected using QSM and amyloid PET (63,64). However, lack of ATN biomarker evaluation in this study makes it difficult to confirm those expected correlations.
Another limitation is that while QSM has demonstrated sensitivity to iron content changes in AD, it may not be specific to AD pathology as well as it does not directly indicate either neurodegeneration or tau accumulation. Other factors, such as inflammation, demyelination, and microbleeds, can also affect susceptibility. Therefore, lack of other information from structural MRI including white matter lesions, infarcts, microbleeds, or other structural abnormalities in this study could influence QSM measurements and be significant limitation. Even though patients with psychiatric or other neurological illnesses were excluded. Lack of data of other systemic diseases that might cause brain iron accumulation is also another significant limitation in this study and may confound the results. Another notable limitation is the significant difference in chronological age across participant groups, where age is a critical factor that can influence cognitive performance and susceptibility effects independently of the disease status. Future analyses will incorporate age as well as other diseases and structural changes that might cause brain iron accumulation should be helpful.
Additionally, QSM processing methods and acquisition protocols can vary across research centers, leading to potential discrepancies in reported findings. Standardization of QSM methods and the establishment of normative databases could improve the reliability and comparability of results across studies.
Conclusions
This study found increased susceptibility in the mid-anterior and central parts of the CC in Alzheimer’s patients compared to HCs. The observed weak correlation between susceptibility in these subregions of CC and CDR-global scores implies that susceptibility changes within the CC could contribute to dementia and cognitive decline. A 2-year follow-up revealed significant increases in susceptibility across different regions of the CC, depending on the group, aligning with patterns of atrophy observed in previous studies, which represent anteroposterior gradient as disease progress. Despite QSM’s potential as an imaging biomarker for AD, limitations include its sensitivity to factors other than AD pathology and the need for standardized protocols across research centers.
Acknowledgments
The abstract of this work had been presented in 47th European Society of Neuroradiology (ESNR) Annual Meeting, Paris, France, from 18th to 22nd September 2024.
Footnote
Reporting Checklist: The authors have completed the STROBE reporting checklist. Available at https://qims.amegroups.com/article/view/10.21037/qims-24-319/rc
Funding: None.
Conflicts of Interest: All authors have completed the ICMJE uniform disclosure form (available at https://qims.amegroups.com/article/view/10.21037/qims-24-319/coif). The authors have no conflicts of interest to declare.
Ethical Statement: The authors are accountable for all aspects of the work in ensuring that questions related to the accuracy or integrity of any part of the work are appropriately investigated and resolved. This retrospective study was conducted in accordance with the Declaration of Helsinki (as revised in 2013). The study was approved by the Siriraj Institutional Review Board (SIRB) (COA: Si009/2022). Informed consent was provided by all participants.
Open Access Statement: This is an Open Access article distributed in accordance with the Creative Commons Attribution-NonCommercial-NoDerivs 4.0 International License (CC BY-NC-ND 4.0), which permits the non-commercial replication and distribution of the article with the strict proviso that no changes or edits are made and the original work is properly cited (including links to both the formal publication through the relevant DOI and the license). See: https://creativecommons.org/licenses/by-nc-nd/4.0/.
References
- Ballard C, Gauthier S, Corbett A, Brayne C, Aarsland D, Jones E. Alzheimer’s disease. Lancet 2011;377:1019-31. [Crossref] [PubMed]
- Aillaud I, Funke SA. Tau Aggregation Inhibiting Peptides as Potential Therapeutics for Alzheimer Disease. Cell Mol Neurobiol 2023;43:951-61. [Crossref] [PubMed]
- Uchida Y, Kan H, Sakurai K, Oishi K, Matsukawa N. Quantitative susceptibility mapping as an imaging biomarker for Alzheimer’s disease: The expectations and limitations. Front Neurosci 2022;16:938092. [Crossref] [PubMed]
- Ghaderi S, Mohammadi S, Nezhad NJ, Karami S, Sayehmiri F. Iron quantification in basal ganglia: quantitative susceptibility mapping as a potential biomarker for Alzheimer’s disease - a systematic review and meta-analysis. Front Neurosci 2024;18:1338891. [Crossref] [PubMed]
- Huang C, Li J, Liu C, Zhang Y, Tang Q, Lv X, Ruan M, Deng K. Investigation of brain iron levels in Chinese patients with Alzheimer’s disease. Front Aging Neurosci 2023;15:1168845. [Crossref] [PubMed]
- Cogswell PM, Wiste HJ, Senjem ML, Gunter JL, Weigand SD, Schwarz CG, Arani A, Therneau TM, Lowe VJ, Knopman DS, Botha H, Graff-Radford J, Jones DT, Kantarci K, Vemuri P, Boeve BF, Mielke MM, Petersen RC, Jack CR Jr. Associations of quantitative susceptibility mapping with Alzheimer’s disease clinical and imaging markers. Neuroimage 2021;224:117433. [Crossref] [PubMed]
- Merenstein JL, Zhao J, Overson DK, Truong TK, Johnson KG, Song AW, Madden DJ. Depth- and curvature-based quantitative susceptibility mapping analyses of cortical iron in Alzheimer’s disease. Cereb Cortex 2024;34:bhad525. [Crossref] [PubMed]
- Moon Y, Han SH, Moon WJ. Patterns of Brain Iron Accumulation in Vascular Dementia and Alzheimer’s Dementia Using Quantitative Susceptibility Mapping Imaging. J Alzheimers Dis 2016;51:737-45. [Crossref] [PubMed]
- Acosta-Cabronero J, Williams GB, Cardenas-Blanco A, Arnold RJ, Lupson V, Nestor PJ. In vivo quantitative susceptibility mapping (QSM) in Alzheimer’s disease. PLoS One 2013;8:e81093. [Crossref] [PubMed]
- Au CKF, Abrigo J, Liu C, Liu W, Lee J, Au LWC, Chan Q, Chen S, Leung EYL, Ho CL, Ko H, Mok VCT, Chen W. Quantitative Susceptibility Mapping of the Hippocampal Fimbria in Alzheimer’s Disease. J Magn Reson Imaging 2021;53:1823-32. [Crossref] [PubMed]
- Hametner S, Endmayr V, Deistung A, Palmrich P, Prihoda M, Haimburger E, Menard C, Feng X, Haider T, Leisser M, Köck U, Kaider A, Höftberger R, Robinson S, Reichenbach JR, Lassmann H, Traxler H, Trattnig S, Grabner G. The influence of brain iron and myelin on magnetic susceptibility and effective transverse relaxation - A biochemical and histological validation study. Neuroimage 2018;179:117-33. [Crossref] [PubMed]
- Ahmed M, Chen J, Arani A, Senjem ML, Cogswell PM, Jack CR Jr, Liu C. The diamagnetic component map from quantitative susceptibility mapping (QSM) source separation reveals pathological alteration in Alzheimer’s disease-driven neurodegeneration. Neuroimage 2023;280:120357. [Crossref] [PubMed]
- Teipel SJ, Bayer W, Alexander GE, Bokde AL, Zebuhr Y, Teichberg D, Müller-Spahn F, Schapiro MB, Möller HJ, Rapoport SI, Hampel H. Regional pattern of hippocampus and corpus callosum atrophy in Alzheimer’s disease in relation to dementia severity: evidence for early neocortical degeneration. Neurobiol Aging 2003;24:85-94. [Crossref] [PubMed]
- Di Paola M, Phillips O, Orfei MD, Piras F, Cacciari C, Caltagirone C, Spalletta G. Corpus Callosum Structure is Topographically Correlated with the Early Course of Cognition and Depression in Alzheimer’s Disease. J Alzheimers Dis 2015;45:1097-108. [Crossref] [PubMed]
- Kim S, Lee Y, Jeon CY, Kim K, Jeon Y, Jin YB, Oh S, Lee C. Quantitative magnetic susceptibility assessed by 7T magnetic resonance imaging in Alzheimer’s disease caused by streptozotocin administration. Quant Imaging Med Surg 2020;10:789-97. [Crossref] [PubMed]
- O’Callaghan J, Holmes H, Powell N, Wells JA, Ismail O, Harrison IF, Siow B, Johnson R, Ahmed Z, Fisher A, Meftah S, O’Neill MJ, Murray TK, Collins EC, Shmueli K, Lythgoe MF. Tissue magnetic susceptibility mapping as a marker of tau pathology in Alzheimer’s disease. Neuroimage 2017;159:334-45. [Crossref] [PubMed]
- Train the Brain Forum Committee. Thai mental state examination (TMSE). Siriraj Hosp Gaz 1993;45:359-74.
- Morris JC. The Clinical Dementia Rating (CDR): current version and scoring rules. Neurology 1993;43:2412-4. [Crossref] [PubMed]
- Wang Y, Liu T. Quantitative susceptibility mapping (QSM): Decoding MRI data for a tissue magnetic biomarker. Magn Reson Med 2015;73:82-101. [Crossref] [PubMed]
- Li W, Wu B, Liu C. Quantitative susceptibility mapping of human brain reflects spatial variation in tissue composition. Neuroimage 2011;55:1645-56. [Crossref] [PubMed]
- Liu T, Khalidov I, de Rochefort L, Spincemaille P, Liu J, Tsiouris AJ, Wang Y. A novel background field removal method for MRI using projection onto dipole fields (PDF). NMR Biomed 2011;24:1129-36. [Crossref] [PubMed]
- Feng X, Deistung A, Reichenbach JR. Quantitative susceptibility mapping (QSM) and R(2)(*) in the human brain at 3T: Evaluation of intra-scanner repeatability. Z Med Phys 2018;28:36-48. [Crossref] [PubMed]
- Liu C, Li W, Tong KA, Yeom KW, Kuzminski S. Susceptibility-weighted imaging and quantitative susceptibility mapping in the brain. J Magn Reson Imaging 2015;42:23-41. [Crossref] [PubMed]
- Le Bihan D. Looking into the functional architecture of the brain with diffusion MRI. Nat Rev Neurosci 2003;4:469-80. [Crossref] [PubMed]
- Guan X, Huang P, Zeng Q, Liu C, Wei H, Xuan M, Gu Q, Xu X, Wang N, Yu X, Luo X, Zhang M. Quantitative susceptibility mapping as a biomarker for evaluating white matter alterations in Parkinson’s disease. Brain Imaging Behav 2019;13:220-31. [Crossref] [PubMed]
- Daianu M, Jahanshad N, Nir TM, Jack CR Jr, Weiner MW, Bernstein MA, Thompson PMAlzheimer’s Disease Neuroimaging Initiative. Rich club analysis in the Alzheimer’s disease connectome reveals a relatively undisturbed structural core network. Hum Brain Mapp 2015;36:3087-103. [Crossref] [PubMed]
- Lo CY, Wang PN, Chou KH, Wang J, He Y, Lin CP. Diffusion tensor tractography reveals abnormal topological organization in structural cortical networks in Alzheimer’s disease. J Neurosci 2010;30:16876-85. [Crossref] [PubMed]
- Wang XN, Zeng Y, Chen GQ, Zhang YH, Li XY, Hao XY, Yu Y, Zhang M, Sheng C, Li YX, Sun Y, Li HY, Song Y, Li KC, Yan TY, Tang XY, Han Y. Abnormal organization of white matter networks in patients with subjective cognitive decline and mild cognitive impairment. Oncotarget 2016;7:48953-62. [Crossref] [PubMed]
- Zhang Y, Schuff N, Du AT, Rosen HJ, Kramer JH, Gorno-Tempini ML, Miller BL, Weiner MW. White matter damage in frontotemporal dementia and Alzheimer’s disease measured by diffusion MRI. Brain 2009;132:2579-92. [Crossref] [PubMed]
- Kan H, Uchida Y, Arai N, Ueki Y, Aoki T, Kasai H, Kunitomo H, Hirose Y, Matsukawa N, Shibamoto Y. Simultaneous voxel-based magnetic susceptibility and morphometry analysis using magnetization-prepared spoiled turbo multiple gradient echo. NMR Biomed 2020;33:e4272. [Crossref] [PubMed]
- Ota M, Obata T, Akine Y, Ito H, Ikehira H, Asada T, Suhara T. Age-related degeneration of corpus callosum measured with diffusion tensor imaging. Neuroimage 2006;31:1445-52. [Crossref] [PubMed]
- McLaughlin NC, Paul RH, Grieve SM, Williams LM, Laidlaw D, DiCarlo M, Clark CR, Whelihan W, Cohen RA, Whitford TJ, Gordon E. Diffusion tensor imaging of the corpus callosum: a cross-sectional study across the lifespan. Int J Dev Neurosci 2007;25:215-21. [Crossref] [PubMed]
- Michielse S, Coupland N, Camicioli R, Carter R, Seres P, Sabino J, Malykhin N. Selective effects of aging on brain white matter microstructure: a diffusion tensor imaging tractography study. Neuroimage 2010;52:1190-201. [Crossref] [PubMed]
- Davis SW, Dennis NA, Buchler NG, White LE, Madden DJ, Cabeza R. Assessing the effects of age on long white matter tracts using diffusion tensor tractography. Neuroimage 2009;46:530-41. [Crossref] [PubMed]
- Sullivan EV, Rohlfing T, Pfefferbaum A. Longitudinal study of callosal microstructure in the normal adult aging brain using quantitative DTI fiber tracking. Dev Neuropsychol 2010;35:233-56. [Crossref] [PubMed]
- Aboitiz F, Rodríguez E, Olivares R, Zaidel E. Age-related changes in fibre composition of the human corpus callosum: sex differences. Neuroreport 1996;7:1761-4. [Crossref] [PubMed]
- Aboitiz F, Scheibel AB, Zaidel E. Morphometry of the Sylvian fissure and the corpus callosum, with emphasis on sex differences. Brain 1992;115:1521-41. [Crossref] [PubMed]
- Bender AR, Völkle MC, Raz N. Differential aging of cerebral white matter in middle-aged and older adults: A seven-year follow-up. Neuroimage 2016;125:74-83. [Crossref] [PubMed]
- Madden DJ, Bennett IJ, Burzynska A, Potter GG, Chen NK, Song AW. Diffusion tensor imaging of cerebral white matter integrity in cognitive aging. Biochim Biophys Acta 2012;1822:386-400. [Crossref] [PubMed]
- Marner L, Nyengaard JR, Tang Y, Pakkenberg B. Marked loss of myelinated nerve fibers in the human brain with age. J Comp Neurol 2003;462:144-52. [Crossref] [PubMed]
- O’Sullivan M, Jones DK, Summers PE, Morris RG, Williams SC, Markus HS. Evidence for cortical “disconnection” as a mechanism of age-related cognitive decline. Neurology 2001;57:632-8. [Crossref] [PubMed]
- Pfefferbaum A, Adalsteinsson E, Sullivan EV. Frontal circuitry degradation marks healthy adult aging: Evidence from diffusion tensor imaging. Neuroimage 2005;26:891-9. [Crossref] [PubMed]
- Salat DH, Tuch DS, Hevelone ND, Fischl B, Corkin S, Rosas HD, Dale AM. Age-related changes in prefrontal white matter measured by diffusion tensor imaging. Ann N Y Acad Sci 2005;1064:37-49. [Crossref] [PubMed]
- Sexton CE, Walhovd KB, Storsve AB, Tamnes CK, Westlye LT, Johansen-Berg H, Fjell AM. Accelerated changes in white matter microstructure during aging: a longitudinal diffusion tensor imaging study. J Neurosci 2014;34:15425-36. [Crossref] [PubMed]
- Zhang Y, Du AT, Hayasaka S, Jahng GH, Hlavin J, Zhan W, Weiner MW, Schuff N. Patterns of age-related water diffusion changes in human brain by concordance and discordance analysis. Neurobiol Aging 2010;31:1991-2001. [Crossref] [PubMed]
- Fan Q, Tian Q, Ohringer NA, Nummenmaa A, Witzel T, Tobyne SM, Klawiter EC, Mekkaoui C, Rosen BR, Wald LL, Salat DH, Huang SY. Age-related alterations in axonal microstructure in the corpus callosum measured by high-gradient diffusion MRI. Neuroimage 2019;191:325-36. [Crossref] [PubMed]
- Hou J, Pakkenberg B. Age-related degeneration of corpus callosum in the 90+ years measured with stereology. Neurobiol Aging 2012;33:1009.e1-9. [Crossref] [PubMed]
- Fling BW, Chapekis M, Reuter-Lorenz PA, Anguera J, Bo J, Langan J, Welsh RC, Seidler RD. Age differences in callosal contributions to cognitive processes. Neuropsychologia 2011;49:2564-9. [Crossref] [PubMed]
- Wang PN, Chou KH, Chang NJ, Lin KN, Chen WT, Lan GY, Lin CP, Lirng JF. Callosal degeneration topographically correlated with cognitive function in amnestic mild cognitive impairment and Alzheimer’s disease dementia. Hum Brain Mapp 2014;35:1529-43. [Crossref] [PubMed]
- Di Paola M, Luders E, Di Iulio F, Cherubini A, Passafiume D, Thompson PM, Caltagirone C, Toga AW, Spalletta G. Callosal atrophy in mild cognitive impairment and Alzheimer’s disease: different effects in different stages. Neuroimage 2010;49:141-9. [Crossref] [PubMed]
- Ukmar M, Makuc E, Onor ML, Garbin G, Trevisiol M, Cova MA. Evaluation of white matter damage in patients with Alzheimer’s disease and in patients with mild cognitive impairment by using diffusion tensor imaging. Radiol Med 2008;113:915-22. [Crossref] [PubMed]
- Di Paola M, Di Iulio F, Cherubini A, Blundo C, Casini AR, Sancesario G, Passafiume D, Caltagirone C, Spalletta G. When, where, and how the corpus callosum changes in MCI and AD: a multimodal MRI study. Neurology 2010;74:1136-42. [Crossref] [PubMed]
- Frederiksen KS. Corpus callosum in aging and dementia. Dan Med J 2013;60:B4721.
- van Bergen JM, Li X, Hua J, Schreiner SJ, Steininger SC, Quevenco FC, Wyss M, Gietl AF, Treyer V, Leh SE, Buck F, Nitsch RM, Pruessmann KP, van Zijl PC, Hock C, Unschuld PG. Colocalization of cerebral iron with Amyloid beta in Mild Cognitive Impairment. Sci Rep 2016;6:35514. [Crossref] [PubMed]
- Ayton S, Fazlollahi A, Bourgeat P, Raniga P, Ng A, Lim YY, Diouf I, Farquharson S, Fripp J, Ames D, Doecke J, Desmond P, Ordidge R, Masters CL, Rowe CC, Maruff P, Villemagne VLAustralian Imaging Biomarkers and Lifestyle (AIBL) Research Group. Salvado O, Bush AI. Cerebral quantitative susceptibility mapping predicts amyloid-β-related cognitive decline. Brain 2017;140:2112-9. [Crossref] [PubMed]
- Chen L, Soldan A, Oishi K, Faria A, Zhu Y, Albert M, van Zijl PCM, Li X. Quantitative Susceptibility Mapping of Brain Iron and β-Amyloid in MRI and PET Relating to Cognitive Performance in Cognitively Normal Older Adults. Radiology 2021;298:353-62. [Crossref] [PubMed]
- Jarbo K, Verstynen T, Schneider W. In vivo quantification of global connectivity in the human corpus callosum. Neuroimage 2012;59:1988-96. [Crossref] [PubMed]
- Hofer S, Frahm J. Topography of the human corpus callosum revisited--comprehensive fiber tractography using diffusion tensor magnetic resonance imaging. Neuroimage 2006;32:989-94. [Crossref] [PubMed]
- Jokinen H, Frederiksen KS, Garde E, Skimminge A, Siebner H, Waldemar G, Ylikoski R, Madureira S, Verdelho A, van Straaten EC, Barkhof F, Fazekas F, Schmidt R, Pantoni L, Inzitari D, Erkinjuntti T. Callosal tissue loss parallels subtle decline in psychomotor speed. a longitudinal quantitative MRI study. The LADIS Study. Neuropsychologia 2012;50:1650-5. [Crossref] [PubMed]
- Jokinen H, Ryberg C, Kalska H, Ylikoski R, Rostrup E, Stegmann MB, Waldemar G, Madureira S, Ferro JM, van Straaten EC, Scheltens P, Barkhof F, Fazekas F, Schmidt R, Carlucci G, Pantoni L, Inzitari D, Erkinjuntti T. Corpus callosum atrophy is associated with mental slowing and executive deficits in subjects with age-related white matter hyperintensities: the LADIS Study. J Neurol Neurosurg Psychiatry 2007;78:491-6. [Crossref] [PubMed]
- Chaim TM, Duran FL, Uchida RR, Périco CA, de Castro CC, Busatto GF. Volumetric reduction of the corpus callosum in Alzheimer’s disease in vivo as assessed with voxel-based morphometry. Psychiatry Res 2007;154:59-68. [Crossref] [PubMed]
- Jack CR Jr, Bennett DA, Blennow K, Carrillo MC, Dunn B, Haeberlein SB, et al. NIA-AA Research Framework: Toward a biological definition of Alzheimer’s disease. Alzheimers Dement 2018;14:535-62. [Crossref] [PubMed]
- van Bergen JM, Hua J, Unschuld PG, Lim IA, Jones CK, Margolis RL, Ross CA, van Zijl PC, Li X. Quantitative Susceptibility Mapping Suggests Altered Brain Iron in Premanifest Huntington Disease. AJNR Am J Neuroradiol 2016;37:789-96. [Crossref] [PubMed]
- Tiepolt S, Schäfer A, Rullmann M, Roggenhofer E, Gertz HJ, Schroeter ML, Patt M, Bazin PL, Jochimsen TH, Turner R, Sabri O, Barthel H. Quantitative Susceptibility Mapping of Amyloid-β Aggregates in Alzheimer’s Disease with 7T MR. J Alzheimers Dis 2018;64:393-404. [Crossref] [PubMed]