Integrated radiomics and immune infiltration analysis to decipher immunotherapy efficacy in lung adenocarcinoma
Introduction
With over one million fatalities each year globally, lung adenocarcinoma (LUAD), the most common subtype of non-small-cell lung cancer (NSCLC), accounts for over 40% of all cases of lung cancer (1,2). LUAD exhibits elevated expression of programmed death-ligand 1 (PD-L1), tumor mutation burden (TMB), and potent immunogenicity, hence immunotherapy is an especially optimum therapeutic modality among the available therapeutic options and has demonstrated efficacy targeting tumor cells by manipulating the immune system (3,4). The most often used immunotherapy for treating advanced LUAD is immune checkpoint blockade (ICB), which uses inhibitors that target immunological checkpoints such programmed cell death protein 1 (PD-1) and PD-L1 (5). However, the immunotherapy outcomes are not optimistic for most patients, and multiple initial responders will ultimately become resistant to the therapy (6). Therefore, there is an urgent need to predict the immunotherapy response and clinical outcomes of LUAD patients, which will serve as a guidance to stratify patients for treatment with optimal therapeutic modalities.
Currently, variable immune checkpoint genes (ICGs)-related classification methods on the basis of gene signatures or immune components in transcriptome investigations have shown predictive value in LUAD immunotherapy (7,8). Although there has been some progress with ICGs-related classifications, existing approaches carry considerable limitations, due largely to the heterogeneity and complexity of tumor microenvironment (TME) components (8). Indeed, rather than growing alone, the tumor expands and invades through engaging in dynamic interactions with the TME that surrounds it (9). Recently, increasing publications have demonstrated that the TME of lung cancer patients can define the immune subtypes of tumors and is potently correlated with the prognosis of immunotherapy (10-13). Besides, it should be noted that the sex factor may also affect immunotherapy response (14). A significant sex-based heterogeneity of response to various immunotherapy strategies, such as ICGs, in patients with advanced LUAD may be partially explained by the research that previously showed that the TME of LUAD between females and males was characterized by different abundance of innate and adaptive immune cell types (15). Therefore, further studies, which take sex factor and immune infiltration into account in LUAD immunotherapy response, will need to be performed. Nevertheless, the detection of underlying biomarkers and identification of immune subtypes require an invasive surgical lung biopsy as well as time-consuming and labor-intensive laboratory work, after which the mortality can be even compared to the lobectomy for lung cancer (16,17).
Radiomics, which is known as quantitative evaluation of radiological images, has been demonstrated to have high performance in predicting clinical response and survival outcomes in the cancer treatment (18,19). Currently, noninvasive radiological imaging enables extensive low-cost repetitions, reflecting the entire picture of tumor features and the surrounding ecosystem (20). High anticipation has been placed that a noninvasive and holistic radiomics approach may offer a unique avenue to depict the molecular landscape of TME, which has been an intriguing topic for the prediction of LUAD immunotherapy. Combined with advanced technology and deep learning approaches, radiomics for the classification of immune subtypes is promising to pave the way for clinical translation in the guidance of precision cancer therapy.
Here, we intended to develop a noninvasive radiomics method to disclose the immune infiltration for the prediction of immunotherapy response and prognosis of LUAD patients. By integrating 535 LUAD samples from The Cancer Genome Atlas (TCGA) LUAD dataset, we carried out an extensive investigation of the immune infiltration landscape. Herein, two immune subtypes, A and B, were identified by the significant differences in immune infiltration. Then, a risk score model was created on differentially expressed genes (DEGs) between A and B subtypes, after which LUAD samples with immunotherapy outcomes were chosen to verify the prognosis utility. An algorithm for radiomics was utilized for collecting the image signatures, and radiomics prediction models were developed afterwards to determine the immunosubtype categorization. In summary, our study suggested the crucial function of noninvasive radiomics in the classification of LUAD subtypes based on immune infiltration, which may serve as guidance to precision immunotherapy and predict survival outcomes for LUAD patients. We present this article in accordance with the TRIPOD + AI reporting checklist (available at https://qims.amegroups.com/article/view/10.21037/qims-24-130/rc).
Methods
LUAD dataset source and processing
The clinical details of LUAD samples as well as the public transcriptome expression data were obtained from TCGA database (https://tcga-data, nci.nih.gov/tcga) (21). Our analysis involved 535 LUAD patients in total. All analyses were performed excluding patients without complete clinical data. RNA sequencing data [fragments per kilobase of transcript per million mapped reads (FPKM) values] extracted from Genomic Data Commons (GDC, https://portal.gdc.cancer.gov/) (22) were transformed into transcripts per kilobase million (TPM) values for the combined analysis. From the Tumor and Immune System Interaction Database (TISIDB) (http://cis.hku.hk/TISIDB) (23), 116 genes connected to immune systems including MHC, immunoinhibitory, immunostimulatory, chemokine, and receptors were collected. The somatic mutations, copy number variations (CNVs), and DNA methylation data were extracted from TCGA database and the University of California, Santa Cruz (UCSC) Xena database (http://xena.ucsc.edu/) (24), and CNVs, somatic mutations, and DNA methylation data of LUAD were taken, respectively. TMB was determined by multiplying the number of somatic mutations by 106 and dividing the total exonic bases with sufficient coverage. We processed the somatic mutation data via the VarScan software (https://varscan.sourceforge.net/). Publicly available data with clinical drug response information on LUAD were downloaded from Cancer Treatment Response Gene Signature Database (ctr-db, http://ctrdb.cloudna.cn/home). The Cancer Imaging Archive database (TCIA, https://wiki.cancerimagingarchive.net/) (25) was applied to gather the successful clinical immunotherapy performance and radiomics data of LUAD patients.
Collection of clinical data
From December 2021 to September 2022, researchers gathered clinical information on patients with LUAD at the Third Xiangya Hospital. The study was conducted in accordance with the Declaration of Helsinki (as revised in 2013). The study was approved by Ethics Board of the Third Xiangya Hospital (No. Express 23466) and the requirement for individual consent for this retrospective analysis was waived. With clinical diagnoses with LUAD, all patients admitted to the Respiratory Department of The Third Xiangya Hospital were enrolled. All individuals who failed to attend follow-ups were not included. The presence of tumor, node, and metastasis (TNM) classification and stage was also considered as exclusion criteria for LUAD patients. Follow-up time: 28 October 2022. Overall survival (OS) was taken as the amount of time between the hospital discharge date and the death or follow-up date. Table 1 summarizes the clinical data from LUAD patients.
Table 1
Characteristic | Levels | N (%) |
---|---|---|
Sex | Female | 25 (31.25) |
Male | 55 (68.75) | |
Age (years) | ≤65 | 49 (61.25) |
>65 | 31 (38.75) | |
T stage† | T1 | 6 (7.79) |
T2 | 19 (24.68) | |
T3 | 9 (11.69) | |
T4 | 43 (55.84) | |
N stage† | N0 | 13 (16.46) |
N1 | 5 (6.33) | |
N2 | 20 (25.31) | |
N3 | 41 (51.90) | |
M stage | M0 | 11 (13.75) |
M1 | 69 (86.25) | |
Stage | Stage I | 1 (1.25) |
Stage II | 1 (1.25) | |
Stage III | 9 (11.25) | |
Stage IV | 69 (86.25) | |
OS event | Alive | 39 (48.75) |
Dead | 41 (51.25) |
†, ambiguous data were deleted. LUAD, lung adenocarcinoma; OS, overall survival.
Immune infiltration and genetic variation estimation
Single-sample gene set enrichment analysis (ssGSEA) examines a single sample that cannot be processed using regular gene set enrichment analysis (GSEA) (26). We used the ssGSEA algorithm and the R packages [gene set variation analysis (GSVA), GSEABase, and limma] to fully assess the immunological characteristics of each sample used in our investigation on the basis of 29 immune gene sets (27). Based on the levels of ICGs expression, ssGSEA was employed to determine the relative number of immune cells in LUAD samples (28). Heatmaps have been produced using the results to show how immune infiltration and ICGs expression are related. Through the UCSC Genome Browser (http://genome.ucsc.edu/), we carried out the analysis of differentially methylated regions (DMR) between the two immunosubtypes. The “limma” R package was further implemented to perform the methylation difference analysis. Additionally, to prioritize ICGs that displayed a higher mutation frequency than the background, we employed the “MutSigCV” algorithm. The mutation landscapes of ICGs across high- and low-immune infiltration subtypes in the LUAD patients were analyzed and visualized using the “maftools” R package.
Identification of LUAD subtypes based on immune infiltration
Using an unsupervised clustering methodology, we categorized LUAD patients into distinct immune subtypes depending on the infiltration levels of 23 immune cells. Therefore, 50 iterations (each utilizing 80% of the data) of k-means clustering was applied. By analyzing factors including cluster stability, cophenetic, dispersion, and silhouette, the optimal number of clusters was determined. After 1,000 repetitions, consensus clustering was accomplished facilitated by the “ConsensusClusterPlus” R package. Comparative analyses of the area under the cumulative distribution function (CDF) curve were performed. To ensure the consensus clustering reliability and verify the independence between the two subtypes, principal component analysis (PCA) was utilized for dimensionality reduction. Afterwards, the GSVA R package was employed to assess differences in biological pathways among the two immune subtypes (29). We obtained the gene set “c2.cp.kegg.v7.2.symbols.” via the MsigDB database (http://www.gsea-misgdb.org/gsea/downloads.jsp). The top 20 biological pathways were chosen for inclusion after adjusting the P value. Normalized to an expression range of 0–1, the enrichment scores were computed for each sample. The Kyoto Encyclopedia of Genes and Genomes (KEGG) database served to disclose the biological characteristics. The deconvolution algorithm “cibersort” was used to further assess the relative abundance of 23 immune cells. Additionally, the stromal and immune abundance were calculated on the basis of the RNS-seq data through the “ESTIMATE” algorithm, which depicted the overview of the components of the TME. Survival outcomes of LUAD patients were analyzed by Kaplan-Meier curves for each dataset. LUAD patients had their OS and disease-specific survival (DSS) evaluated. The significance threshold for the log-rank test was set at P<0.05.
Construction and validation of the risk score model based on DEGs
DEGs between A and B immune subtypes were selected by means of the empirical Bayesian function of the “limma” R package, with adjusted P value as 0.001. In order to isolate the genes associated with prognosis, univariate Cox regression was adopted. Afterwards, the genes with prognostic significance (P<0.05) in OS, DSS, and disease free internal (DFI) were input into a least absolute shrinkage and selection operator (LASSO) Cox regression model with 10-fold cross-validation. A risk score model on the basis of the genes associated with prognosis was established using the “glmnet” R package. Following the formula, we computed the risk score for each sample across all of the datasets. Additionally, a multivariate Cox regression model was utilized to optimize the selected genes and to achieve the regression coefficients for these genes. Using this technique, genes associated with the prognosis of LUAD were identified. For each patient, a risk score was created by multiplying the normalized gene expression of each important gene by the appropriate regression coefficient. Patients were divided into high- and low-risk groups according to their median risk scores. To increase the prognostic model’s accuracy, the 535 patients with LUAD were randomly split into a test set (n=267) and a train set (n=268) in a ratio of 1:1. TNM stage, sex, anatomy, cancer stage, residual tumor, survival status, age, smoking years, and survival time were among the important clinical factors taken into account to guarantee balanced sampling of the train and test sets. A prognosis risk score for LUAD was established utilizing the train set, and the prognostic validity was validated using the test set and all sets.
Quantitative real-time polymerase chain reaction
Total messenger RNA (mRNA) from BEAS-2B and A549 cells were extracted using RNA simple Total RNA Kit (TIANGEN BIOTECH, Beijing, China). The complementary DNA (cDNA) was synthesized from RNA using All-in-One First-Strand Synthesis MasterMix (Yugong Biolabs, Jiangsu, China). Subsequently, gene expression was quantified by quantitative real-time polymerase chain reaction (qPCR) using SuperReal PreMix Plus (SYBR Green) and the LightCycler480 System (TIANGEN BIOTECH) for the housekeeping gene GAPDH and for the genes of interest: LINC01117, MSLNL, CDC42EP2, FAM207A, E2F7, TENT5C, HSDL1, and TIMP1. The 2−ΔΔCt method [ΔCt = Ct (target gene − internal reference gene); ΔΔΔCt = ΔCt – Δctmin] was used to compute the relative expression levels of mRNA, and the unpaired t-test was employed to evaluate the variations in gene expression between tumor tissues and normal tissues. Table 2 contains a list of the primers utilized for the study.
Table 2
Gene | Sequences (5'-3') |
---|---|
LINC01117 | Forward: ATCCTTCGGCGGGGCGATAC |
Reverse: GCTTTCAGGGTCAGGAGGTACAATG | |
MSLNL | Forward: ACGGCGAGTCCAGAGTCACG |
Reverse: GGTTGTAGAAGAGCAGCAGGTTGG | |
CDC42EP2 | Forward: CCAAGGTGCCCATCTATCTGAAGC |
Reverse: CCGCCGCCACTGCCAATATG | |
FAM207A | Forward: GGAAGGACTGGGCGTTCATCAAC |
Reverse: ACCTCTCCTGACGGAAGTGACAC | |
E2F7 | Forward: CGTCAGGGTCAGGGTCAGAGAG |
Reverse: TTAGTGGCTGGCTCATCCTCCTC | |
TENT5C | Forward: AGTCACCTACAGCCAGCCTTACC |
Reverse: CTACCTGAGAGCCCTAGCCCTATTG | |
HSDL1 | Forward: TTTAGACCACTTCAGCAGAGCCTTG |
Reverse: GCACCAACCACGAGCACCTG | |
TIMP1 | Forward: ATCCTGTTGTTGCTGTGGCTGATAG |
Reverse: CGCTGGTATAAGGTGGTCTGGTTG |
Tumor Immune Estimation Resource (TIMER2.0)
TIMER2.0 (http://timer.comp-genomics.org/) (30) is a tumor immunity related database. “Immune estimation module” refers to analyzing immune infiltration estimations for users-provided expression profiles by TIMER, Cell-type Identification by Estimating Relative Subsets of RNA Transcripts (CIBERSORT), quanTIseq, xCell, Microenvironment Cell Populations (MCP)-counter, and Estimating the Proportion of Immune and Cancer cells (EPIC) algorithms. We studied whether the expression of the DEGs was related to the level of immune infiltration in LUAD.
Construction and evaluation of the nomogram
In order to better correctly forecast the prognosis of LUAD patients based on the TCGA-LUAD cohort, we constructed a nomogram incorporating the risk score as well as other clinical parameters. Then, to identify independent predictive factors for the OS of LUAD patients, variables comprising age, sex, TNM stages, and risk score were incorporated in a multivariate Cox-regression analysis. Moreover, the constructed nomogram, immune-genes model, and clinical model based on clinical characteristics were compared by the 1-, 3-, and 5-year decision curve analysis (DCA) curves to evaluate their prediction efficiency. The discriminatory power and calibration performance of the constructed nomogram were evaluated utilizing calibration plots. To examine the probability between the nomogram-predicted survival and the observed survival, calibration curves were used.
Radiomics features selection and radiomics signature construction
We used the immune subtypes as a classifier to capture imaging characteristics from these digital computed tomography (CT) scans and constructed radiogenomic prediction models. From the TCIA dataset, 103 CT imaging samples matching TCGA-LUAD samples were collected. Feature extraction and definition were conducted via the “PyRadiomics” embedded in python, which enables radiomics data to be extracted from CT images and processed. Raw image, three-dimensional (3D) binary model, a Laplacian of Gaussian filter (Σ=2, 3, 4, 5), logarithm, and wavelet transforms were utilized to facilitate the feature extraction. Samples that surpassed three standard deviations were regarded as outliers and excluded from the analysis. The extracted radiogenomic features comprised but were not restricted to the first order statistics, 3D form, gray-level co-occurrence matrix, gray-level dependence matrix, gray-level run length matrix, gray-level size zone matrix, and neighboring gray tone difference matrix. To eliminate the unit constraints, radiomics characteristics were subjected to further processing for the reduction of dimension. We utilized the median value to substitute when a parameter had aberrant values. We randomly chose 80% of the total cases as a train set for the construction of the radiomics model; the other 20% served as an independent test set. The feature matrix was then normalized. Features were selected employing LASSO logistic regression algorithm. A series of machine learning techniques were applied to build radiogenomic models. The best area under the curve (AUC) value was employed as a criterion to validate the final model. The Z-score and min-max normalization were employed to standardize the information. Receiver operating characteristic (ROC) curves were drawn to assess the model’s capacity for prediction. Quantification of AUC, accuracy, sensitivity, specificity, positive predictive value, and negative predictive value were all performed at the highest Youden index value among the cut-off values. The above procedures were implemented using seeitV8.0 for Python programming language (3.7.6).
Statistical analysis
The samples were separated into two groups for survival analysis via the R package “survival”. To investigate the prognostic significance of the immune-related 9-gene signature, the survival curves of patients with LUAD were plotted using Kaplan-Meier analysis. Multivariate survival analyses were assessed utilizing multivariate Cox regression. The R package “pROC” was implemented to calculate AUC in order to assess the efficacy of the created models. The ROC curve of 1-, 3-, and 5-year survival was studied in the train, test, and all sets. We located the ICGs and their circular sequences on the chromosomes through “RCircos”. R (version 4.0.2) was used to create visuals using the “ggplot2”, “VennDiagram”, and “cowplot” packages. A P value less than 0.05 was used to determine statistical significance.
Results
Identification of 23 immune cells related to immune checkpoints and molecules
The design of our study was mapped on a flow chart (Figure 1). We utilized the ssGSEA to conduct a comprehensive investigation of the quantity of immune cells that infiltrated the tumor and showed a strong positive connection with PDCD1 and CD274. According to the heatmap, the immune cells including type 2 T helper cell, type 17 T helper cell, type 1 T helper cell, T follicular helper cell, regulatory T cell, plasmacytoid dendritic cell, neutrophil, natural killer cell, monocyte, myeloid-derived suppressor cell (MDSC), mast cell, macrophage, immature dendritic cell, immature B cell, gamma delta T cell, eosinophil, CD56dim natural killer cell, CD56bright natural killer cell, activated dendritic cell, activated CD8 T cell, activated CD4 T cell, and activated B cell all demonstrated a strong correlation with PDCD1 and CD274 expression (Figure 2A). Figure 2B illustrates the correlation between the expression of IFNG, IFNGR1, and IFNGR2 and the infiltration degree of aforementioned immune cells. Furthermore, we carried out a correlation analysis between the infiltration degree of immune cells and the expression of 108 ICGs, and also observed a relatively strong correlation (Figure 2C). The scatter plots from Figure 2D-2K further indicated the correlation of PDCD1 and IFNG expression with the infiltration degree of four major immune cells including activated CD8 T cell, type 1 T cell, activated B cell, and activated CD4 T cell. The expression level of immune checkpoint inhibitors (ICIs) in correlation with the infiltration degree of 23 immune cells implied that immune infiltration was a potential criterion for the classification of immune subtypes in LUAD.
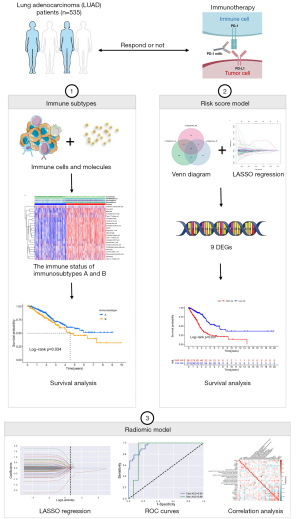

Identification of two LUAD immune subtypes based on 23 immune cells
Based on the immune infiltration level, we subdivided patients into variable subtypes via unsupervised consensus clustering. The results of the clustering identified two distinct immune subtypes, including 299 samples in immune subtype A and 236 samples in immune subtype B. The empirical CDF plot was utilized to identify the optimum cluster number (k) from 2–9 for the standard deviation to attain a suitable maximum, which indicated the highest stability. With the highest consensus and the clearest cluster division at k=2, the consensus matrix highlighted that LUAD patients could be separated into two non-overlapping categories (Figure 3A,3B). The delta area plot of relative change in area under CDF curve also increased in cluster stability when k=2 (Figure 3C). In addition, PCA verified the reliability of the two immunosubtypes (Figure 3D). We utilized GSVA to explore the biological process alteration in the two immune subtypes. The results indicated significant distinction between the two immune subtypes in KEGG pathways analyses, in which immune subtype A, enriching in B cell receptor signaling pathway, T cell receptor signaling pathway, and toll-like receptor signaling pathway, involved mainly in immune activation pathways (Figure 3E). Additionally, three cross-platform data sets revealed that immunosubtype A had a greater level of immune infiltration, which was in line with our ssGSEA clustering analysis (Figure 3F). To further disclose the relationships between the findings of clustering and survival outcomes, we compared the OS and DSS between the two immunological subgroups. As exhibited in the Kaplan-Meier curves, immunosubtype A exerted a superior prognosis compared with the immunosubtype B (P=0.037, Figure 3G; P=0.034, Figure 3H), highlighting that the immune infiltration was significantly linked with the prognosis of LUAD patients. Further, multivariate Cox regression analysis of OS in the LUAD patients including immunological subtypes and major clinicopathological parameters, including stage, age, and grade, revealed immunological subtypes [hazard ratio (HR) 1.468, 95% confidence interval (CI): 1.087–1.982, P=0.012] as independent prognostic factors (Table 3). The TME scores of the two immunological subtypes were compared using three distinct cross-platform data sets, and immunosubtype A showed a higher TME score, suggesting potential relevance in the prognosis of LUAD (Figure 3I). Moreover, we carried out systematic investigation of the gene expression of 108 ICGs in the two diverse immune subtypes, and obvious differences in the gene expression were observed (Figure 3J). Therefore, two immune subtypes were identified, which were implicated in diverse biological processes, levels of immune infiltration, survival outcomes, and gene expression.
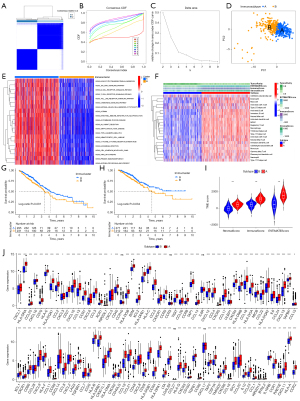
Table 3
Characteristics | Multivariate analysis | |
---|---|---|
HR (95% CI) | P value | |
Immucluster | ||
A | Reference | |
B | 1.468 (1.087–1.982) | 0.012 |
T | ||
T2 | Reference | |
T3 | 1.059 (0.615–1.823) | 0.837 |
T1 | 1.144 (0.822–1.592) | 0.424 |
T4 | 1.662 (0.806–3.428) | 0.169 |
Tx | 0.000 (0.000–Inf) | 0.997 |
N | ||
N2 | Reference | |
N0 | 1.620 (0.971–2.705) | 0.065 |
N1 | 1.662 (0.909–3.036) | 0.099 |
N3 | 2.649 (0.585–12.003) | 0.206 |
Nx | 1.440 (0.405–5.113) | 0.573 |
Unknown | 90,714,567.6392 (0.000–Inf) | 0.991 |
M | ||
M1 | Reference | |
M0 | 1.000 (0.504–1.984) | 0.999 |
Unknown | 0.000 (0.000–Inf) | 0.992 |
MX | 0.969 (0.465–2.019) | 0.932 |
Gender | ||
Male | Reference | |
Female | 1.033 (0.760–1.404) | 0.836 |
Age | 0.994 (0.980–1.009) | 0.452 |
CI, confidence interval; HR, hazard ratio; OS, overall survival; TCGA, The Cancer Genome Atlas.
Clinical characteristics of the two immune subtypes
We analyzed the TNM stage, sex, anatomy, residual tumor, age, and smoking years in the TCGA cohort to look at the association between the A and B immunological subtypes of LUAD and common clinical characteristics. There were no obvious differences in clinical characteristics including T stage (P=0.606), N stage (P=0.052), M stage (P=0.833), anatomy (P=0.449), stage (P=0.602), residual tumor (P=0.139), age (P=0.295), and smoking years (P=0.521) between A and B immune subtypes on the basis of the P value (P>0.05). However, the P value of sex was statistically significant (P=0.048), which exerted no impact on our classification of the two immune subtypes based on the immune infiltration (Table 4). Consequently, outcomes of correlations between the two immune subtypes and clinical traits consolidated the reliability to classify LUAD samples in accordance with the infiltration of 23 selected immune cells.
Table 4
Characteristic | A (n=299) | B (n=236) | P |
---|---|---|---|
T | 0.606 | ||
T1 | 93 (17.4) | 82 (15.3) | |
T2 | 170 (31.8) | 119 (22.2) | |
T3 | 25 (4.7) | 24 (4.5) | |
T4 | 10 (1.9) | 9 (1.7) | |
Tx | 1 (0.2) | 2 (0.4) | |
N | 0.052 | ||
N0 | 194 (36.3) | 154 (28.8) | |
N1 | 63 (11.8) | 32 (6) | |
N2 | 31 (5.8) | 43 (8) | |
N3 | 1 (0.2) | 1 (0.2) | |
Nx | 9 (1.7) | 6 (1.1) | |
Unknown | 1 (0.2) | 0 (0) | |
M | 0.833 | ||
M0 | 204 (38.1) | 157 (29.3) | |
M1 | 15 (2.8) | 10 (1.9) | |
Mx | 76 (14.2) | 67 (12.5) | |
Unknown | 4 (0.7) | 2 (0.4) | |
Gender | 0.048 | ||
Female | 148 (27.7) | 138 (25.8) | |
Male | 151 (28.2) | 98 (18.3) | |
Anatomy | 0.449 | ||
Discrepancy | 4 (0.7) | 4 (0.7) | |
L-lower | 38 (7.1) | 41 (7.7) | |
L-upper | 70 (13.1) | 56 (10.5) | |
Not available | 2 (0.4) | 1 (0.2) | |
Other | 1 (0.2) | 3 (0.6) |
Data are presented as n (%). L, left; TCGA, The Cancer Genome Atlas.
The landscape of genetic variation of ICGs in LUAD
To decipher the genomic and epigenomic features indicating the heterogeneity of the two immune subtypes, multiomics data were analyzed, including CNVs frequency, somatic mutation profiles, DNA methylation status, and TMB. Based on TCGA database and UCSC Xena database, we initially explored the incidence of CNVs of 116 ICGs in LUAD and then summarized the somatic mutations, methylation differences, and TMB in immune subtypes A and B. CNVs alteration analysis suggested that CNVs frequency was common with amplifications in the copy number observed in most ICGs including IFNG. In comparison, a few genes (e.g., PDCD1, CD274, B2M, LAG3, CD40) exhibited significant CNVs deletions (Figure 4A). The waterfall plot depicted that among 286 TCGA-LUAD samples of the immunosubtype A, 45 cases harbored TMB at a frequency of 15.73%, mainly for missense mutation; and among 223 TCGA-LUAD samples of immunosubtype B, 32 cases harbored TMB, with a frequency of 14.35%. In immunosubtype A, KIR2DL1 ranked first in mutation frequency followed by TGFBR1, CSF1R, CX3CR1, and CCR6, whereas PDCD1, CD28, and CD40L did not exhibit any known mutation. Contrarily, in immunosubtype B, CD274 and CD40L did not exhibit any known mutations, whereas CSF1R showed the highest rate of mutation, followed by CD86, PDCD1, and CX3CR1 (Figure 4B). The circular diagram in Figure 4C depicts how various ICGs’ CNVs changes were distributed throughout human chromosomes. The volcano plot with adjusted results showed differentially methylated CpG sites in immune subtypes A and B (Figure 4D). The top 10 major genes regulated by differential methylation sites in the two immune subtypes were exhibited in Figure 4E. Additionally, immunosubtype B also presented a higher TMB frequency compared with immunosubtype A (Figure 4F). The aforementioned investigations indicated a potent distinction in expression alternations and genomic mutations between the two immune subtypes, which was initially validated for the construction of immune classification.
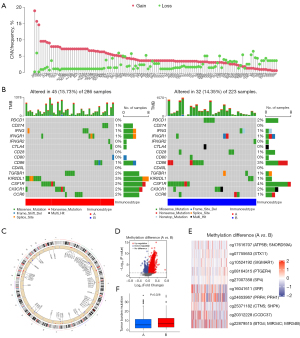
The prognostic risk score model: development and validation
Selected DEGs between A and B immune subtypes were first submitted to univariate Cox regression to develop risk score model for prognosis. We cross-analyzed the selected DEGs which were associated with OS, DSS, and DFI (P<0.05), respectively. An interactive Venn diagram, which searched out 138 DEGs, was used to show the selection process (Figure 5A). After that, we conducted LASSO Cox regression to identify highly relevant elements among 138 DEGs. According to the best value of λ, we discovered nine genes using multiple Cox regression analysis and derived the risk score formula as (Figure 5B,5C). Then, we tried to find evidence for the predictive effect of nine genes on the efficacy of specific immunotherapy. With the help of the Tumor Immune Dysfunction and Exclusion (TIDE) website, by comparing DEGs with standardized biomarkers, we evaluated the predictive efficacy AUC in different immunotherapy cohort studies. Our finding revealed that in the PD-1-NSCLC sub-cohort, utilizing the combination of the nine genes was more reliable than the presented well-known biomarkers including TIDE, microsatellite instability (MSI) score, and IFNG (Figure S1). As we further investigated the relationships between the immunological subtypes, risk score groups, and survival outcomes, an alluvial diagram was utilized to depict the modelling process (Figure 5D). Using Kaplan-Meier curves to evaluate the prognostic risk score model, it was discovered that high-risk patients had significantly worse OS across the three sets of patients (Figure 5E-5G). In the two groups, the patients’ risk score values were displayed in the TCGA databases. Low-risk patients generally had longer survival times and lower mortality rates than patients in the high-risk category. In addition, we displayed the expression levels of nine genes in high- and low-risk groups in the train, test, and all sets using a heat map. The genes with higher expression levels in the high-risk group included TIMP1, AL606489.1, LINC01117, MSLNL, E2F7, CDC42EP2, and FAM207A, whereas the other two genes in the low-risk group had higher expression levels (Figure S2).
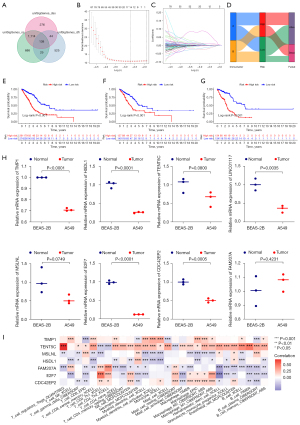
We first employed qPCR to compare the mRNA expression of 8 DEGs between tumor and normal cells in order to analyze the expression of the chosen genes. The results showed that the expression levels of five selected genes (TIMP1, E2F7, HSDL1, CDC42EP2, LINC01117) were significantly lower in tumor tissues compared with matched normal tissues (P<0.05), whereas no significant difference was seen in other three genes (P>0.05) for lack of more repetitions (Figure 5H). Further, qPCR experiments were performed to reflect the interaction between tumor cells and immune cells in the TME. We therefore used six immune cell quantification algorithms to clarify the association between DEGs expression and immune infiltration in the TME of LUAD, and the results demonstrated that DEGs maintained a close relationship with the infiltration degree of immune cells in LUAD. Particularly, five DEGs including MSLNL, HSDL1, FAM207A, E2F7, and CDC42EP2 mostly maintained a significant negative correlation with the infiltration degree of anti-tumor immune cells infiltration such as CD8+ T cell, B cell, neutrophil, and hematopoietic stem cell except CD4+ T cell in LUAD, whereas a positive correlation with the infiltration degree of tumor promoting immune cells infiltration including mast cell resting. The other two DEGs almost demonstrated the opposite correlation (Figure 5I). A distinct difference in nine genes expression levels between A and B immune subtypes was displayed in Table 5 (P<0.05). To validate the random distribution of the train test sets between immunotyping and usual clinical characteristics, we analyzed TNM stage, sex, anatomy, stage, residual tumor, survival status, age, smoke years, and survival time in the TCGA cohort. No clinical traits differed significantly between the train and test sets (Table 6). Thus, we established a risk score model consisting of nine crucial DEGs and validated its prognosis efficacy.
Table 5
Gene | LogFC | AveExpr | t | P value | Adj. P value | B |
---|---|---|---|---|---|---|
TIMP1 | −362.133 | 998.8155 | −6.08793 | 2.19E−09 | 7.87E−08 | 10.7604 |
HSDL1 | 5.147401 | 23.56571 | 5.03247 | 6.63E−07 | 1.16E−05 | 5.248516 |
TENT5C | −10.8373 | 36.05905 | −4.87621 | 1.43E−06 | 2.2E−05 | 4.512693 |
AL606489.1 | 1.30427 | 3.15352 | 3.767113 | 0.000184 | 0.001043 | −0.08934 |
LINC01117 | 0.665515 | 0.951358 | 3.216176 | 0.001378 | 0.00481 | −1.95919 |
MSLNL | 3.587529 | 4.770566 | 3.089796 | 0.002107 | 0.006781 | −2.34817 |
E2F7 | 0.926456 | 2.500659 | 2.986638 | 0.00295 | 0.008942 | −2.65453 |
CDC42EP2 | −3.29097 | 18.93849 | −2.87031 | 0.004263 | 0.012092 | −2.98794 |
FAM207A | 2.486412 | 24.57889 | 2.407581 | 0.016397 | 0.036868 | −4.18649 |
Table 6
Characteristic | Train (n=268) | Test (n=267) | P value |
---|---|---|---|
T, n (%) | 0.634 | ||
Tl | 94 (17.6) | 81 (15.1) | |
T2 | 140 (26.2) | 149 (27.9) | |
T3 | 22 (4.1) | 27 (5) | |
T4 | 11 (2.1) | 8 (1.5) | |
Tx | 1 (0.2) | 2 (0.4) | |
N, n (%) | 0.262 | ||
N0 | 185 (34.6) | 163 (30.5) | |
Nl | 42 (7.9) | 53 (9.9) | |
N2 | 35 (6.5) | 39 (7.3) | |
N3 | 0 (0) | 2 (0.4) | |
Nx | 6 (1.1) | 9 (1.7) | |
Unknown | 0 (0) | 1 (0.2) | |
M, n (%) | 0.240 | ||
M0 | 178 (33.3) | 183 (34.2) | |
Ml | 11 (2.1) | 14 (2.6) | |
Mx | 78 (14.6) | 65 (12.1) | |
Unknown | 1 (0.2) | 5 (0.9) | |
Sex, n (%) | 0.364 | ||
Female | 149 (27.9) | 137 (25.6) | |
Male | 119 (22.2) | 130 (24.3) | |
Anatomy, n (%) | 0.098 | ||
Discrepancy | 3 (0.6) | 5 (0.9) | |
L-lower | 34 (6.4) | 45 (8.4) | |
L-upper | 62 (11.6) | 64 (12) | |
Not available | 2 (0.4) | 1 (0.2) | |
Other | 3 (0.6) | 1 (0.2) | |
R-lower | 48 (9) | 48 (9) | |
R-middle | 5 (0.9) | 16 (3) | |
R-upper | 111 (20.7) | 87 (16.3) | |
Stage, n (%) | 0.395 | ||
Discrepancy | 5 (0.9) | 2 (0.4) | |
I | 2 (0.4) | 3 (0.6) | |
IA | 76 (14.2) | 61 (11.4) | |
IB | 79 (14.8) | 73 (13.6) | |
II | 94 (17.6) | 113 (21.1) | |
IV | 12 (2.2) | 14 (2.6) | |
Unknown | 0 (0) | 1 (0.2) | |
Residual tumor, n (%) | 0.491 | ||
Not evaluated | 15 (2.8) | 7 (1.3) | |
R0 | 172 (32.1) | 183 (34.2) | |
Rl | 5 (0.9) | 8 (1.5) | |
R2 | 2 (0.4) | 2 (0.4) | |
Rx | 15 (2.8) | 12 (2.2) | |
Unknown | 59 (11.0) | 55 (10.3) | |
Fustat, n (%) | 0.954 | ||
Alive | 171 (32.0) | 172 (32.1) | |
Dead | 97 (18.1) | 95 (17.8) | |
Age (years), median [IQR] | 67 [59, 72] | 65 [59, 72] | 0.499 |
Smoking years, median [IQR] | 35 [22, 52] | 40 [20, 50] | 0.967 |
Follow-up time, years, median [IQR] | 1.89 [1.1, 2.88] | 1.71 [1.14, 3.26] | 0.965 |
IQR, interquartile range; L, left; R, right.
Sex characteristics and clinical utility of immune subtype classification
The immune subtypes classification model performed better at prognostic prediction for females than for males, as seen by the Kaplan-Meier curves, which showed a significant difference in OS in different subtypes for female but not for male from the TCGA dataset (Figure 6A,6B). According to the risk score model, the low-risk group exerted a better prognosis than the high-risk group for both female and male patients from TCGA dataset (P<0.001, Figure 6C,6D). TCGA cohort and clinical cohort from the Third Xiangya Hospital were utilized to demonstrate no significant differences of survival probability between sexes (Figure 6E,6F). Through Kaplan-Meier survival analysis, this model appears to show better fit for females than for males.
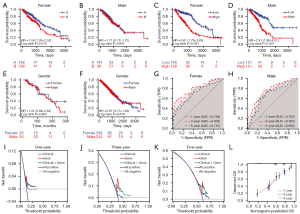
We established a nomogram combining the risk score and the clinicopathologic risk indicators including TNM stage, sex, and age for the prediction of survival probability for LUAD patients (Figure S3). Using time-dependent ROC analysis, the nomogram’s sensitivity and specificity were evaluated. For females with LUAD, the AUCs were 0.738, 0.800, and 0.715 at 1-, 3-, and 5-year survival; for males, they were 0.702, 0.763, and 0.682 at the same respective times (Figure 6G,6H). Later, decision curve tests were conducted to evaluate the nomogram’s clinical efficacy. The nomogram had superior net advantages than the other alternatives for 1-, 3-, and 5-year survival likelihood, according to DCA, but the gene model offered the greatest results for particular thresholds (Figure 6I-6K). The calibration plot showed that the nomogram, which calculated probability of 1-, 3-, and 5-year survival, worked properly and that the anticipated OS was consistent with the actual OS (Figure 6L). Consequently, these studies collectively verified that the nine immune-related genes signature, equivalent to immunosubtypes, could work as a standalone prognostic factor for LUAD patients, especially for females.
Immune characteristics of LUAD subtypes based on immune infiltration for clinical cohort
We conducted consensus clustering to categorize 43 LUAD patients from ctr-db in order to examine the clinical applicability of the risk score model based on immune infiltration. The objective of the empirical CDF plot was to figure out the optimal cluster number (k) from two to nine for the sample distribution of the data to roughly reach a maximum signifying the highest stability. According to the consensus matrix, LUAD patients can be divided into two subtypes with the highest consensus and the most distinct cluster division at k=2 (Figure 7A,7B). As a consequence, subtype A presented a longer life expectancy, which supported the findings of our study of the TCGA dataset (Figure 7C). The “GSVA” enrichment effectively supported our hypothesis that the two subtypes were biological function distinct as well as immune infiltration distinct since the two subtypes exhibited diverse prognoses under the same treatment regimen (Figure 7D,7E). In addition, we discovered that immunotypes differed significantly in terms of immunotherapy response characteristics (Table 7).
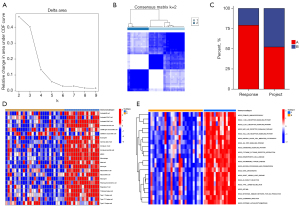
Table 7
Characteristic | A (n=299) | B (n=236) | P value |
---|---|---|---|
Responder, n (%) | <0.001 | ||
False | 95 (17.8) | 10 (1.9) | |
True | 204 (38.1) | 226 (42.2) | |
TAM M2, n (%) | <0.001 | ||
−0.05 | 1 (0.2) | 0 (0) | |
−0.04 | 4 (0.7) | 2 (0.4) | |
−0.03 | 12 (2.2) | 11 (2.1) | |
−0.02 | 50 (9.3) | 17 (3.2) | |
−0.01 | 131 (24.5) | 70 (13.1) | |
0 | 87 (16.3) | 96 (17.9) | |
0.01 | 14 (2.6) | 33 (6.2) | |
0.02 | 0 (0) | 7 (1.3) | |
TIDE, median (IQR) | −0.07 (−0.18, 0.02) | −0.26 (−0.44, −0.14) | <0.001 |
IFNG, median (IQR) | 849.39 (597.81, 1,220.18) | 317.72 (176.56, 508.96) | <0.001 |
MSI Expr Sig, median (IQR) | 0 (0, 0) | 0 (0, 0.02) | <0.001 |
Merck18, median (IQR) | 258.78 (192.38, 354.02) | 109.36 (71.73, 174.39) | <0.001 |
CD274, median (IQR) | 9.82 (6.23, 17.73) | 4.05 (2.52, 9.07) | <0.001 |
CD8, median (IQR) | 11.4 (6.51, 21.2) | 4.75 (2.71, 8.06) | <0.001 |
Dysfunction, median (IQR) | −0.07 (−0.18, 0.02) | −0.26 (−0.44, −0.14) | <0.001 |
Exclusion, median (IQR) | 0.47 (0.27, 0.74) | 0.68 (0.45, 1) | <0.001 |
MDSC, median (IQR) | 0.01 (0, 0.01) | 0.02 (0.01, 0.03) | <0.001 |
CAF, median (IQR) | 0.04 (0.03, 0.06) | 0.04 (0.03, 0.06) | 0.124 |
CAF, cancer-associated fibroblast; IQR, interquartile range; MSI, microsatellite instability; MDSC, myeloid-derived suppressor cell; TCGA, The Cancer Genome Atlas; TIDE, Tumor Immune Dysfunction and Exclusion.
The immunosubtype was demonstrated to be an excellent tool for predicting immunotherapy efficacy using the data from TCGA and ctr-db. Considering that the differences in immune traits were the essence of immune subtypes, we further analyzed immunotherapy response, biomarkers (TIDE, IFNG, MSI Expr Sig, Merck 18, CD274, CD8), immune infiltration, including tumor-associated macrophage (TAM), MDSC, and cancer-associated fibroblast (CAF) in the TCGA cohort between A and B subtypes. The outcome revealed that immune features differed significantly among the two immunotypes, which were consistent with the immunotherapy outcomes (Table 8).
Table 8
Characteristic | A (n=16) | B (n=27) | P value |
---|---|---|---|
Response, n (%) | 0.007 | ||
No | 7 (16.3) | 23 (53.5) | |
Yes | 9 (20.9) | 4 (9.3) | |
Project, n (%) | 0.658 | ||
CTR-13 | 2 (4.7) | 7 (16.3) | |
CTR-197 | 11 (25.6) | 16 (37.2) | |
CTR-381 | 3 (7.0) | 4 (9.3) |
LUAD, lung adenocarcinoma.
Construction of optimal radiomics signatures
More than a thousand different radiomics models have been created in search of the most accurate one for predicting immune subtypes A and B. The classifier AUC values of the test set were used as the criterion of choice for the most effective model. A strategy based on machine learning built with the Z-score method for data normalization and use of the LASSO-constrained logistic regression method enabled the best predictive efficacy to be achieved. The ideal number of features for radiomics was determined to be 12. Based on varying levels of screening for the model’s attributes, the model with the best accuracy for prediction was determined among all the constructed models (Figure 8A). We comprehensively evaluated the radiogenomic model for accuracy by estimating how well the estimated value matched the true value in every test set (Figure 8B). Following that, we used LASSO Cox regression to choose from the 1,595 features the ones that were most important. Using the optimal value, the model selected 12 characteristics from which the prognostic index was derived (Figure 8C). We compared the expression of 12 radiomics characteristics across 103 samples (Figure S4). We discovered that the model achieved the maximum AUC value for the validation set, with an AUC degree of 0.95 and an AUC degree of 0.89 for the train and test sets, respectively (Figure 8D). To evaluate the association between the immune subtypes and radiomics characteristics in LUAD, we incorporated the radiomics characteristics, selected DEGs, risk score, and clinical features for the comprehensive analysis (Figure S5). Consequently, our constructed radiogenomic model incorporating 12 radiomics features displayed satisfactory potential for predicting the immune subtypes.
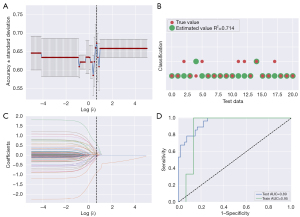
Discussion
LUAD is one of the representative cancers benefiting from the immunotherapy period of cancer with the application of ICB (31). However, only approximately 15% of patients achieved dramatic and durable benefits from targeted immunotherapy (32). Intensive efforts have gone into developing accurate predictive immune biomarkers, including PD-1 and PD-L1 expression, TMB, MSI, and tumor-infiltrating immune cells (33). Zhang et al. developed ICG score to subdivide LUAD patients into subgroups with low and high ICG score (8). The finding confirmed that patients with higher ICG score were characterized by elevated expression of ICGs and favorable survival outcomes. In addition, Yi et al. developed a predictive immune signature incorporating 17 immune-related genes and unraveled its potential predictive relevance with ICIs therapy (4). The publication by Wu et al. reported that a risk model constructed on TME could serve as prediction to predict the OS of patients with LUAD (34). Additionally, a recent paper by Xu et al. has illustrated that specific components in the TME may identify pathways to facilitate the efficiency of ICB (35). Despite the abundance of literature focusing on immune-related biomarkers, no literature has fully elucidated the immune infiltration landscape combined with radiomics in the prediction of the efficacy of immunotherapy, which impelled us to exploit online databases to portray immune subtypes of LUAD and further develop a noninvasive radiomics approach to predict the immune subtype classification.
Based on the aforementioned studies, we first investigated the landscape of tumor-related immune infiltration in LUAD and initially identified immune cells from TME of LUAD patients. Given that PD-1 expression is a pivotal predictive biomarker for ICB and that IFNG is a significant driver of the expression level of PD-1, we conducted a correlation analysis between 23 immune cells and PD-1, its ligand PD-L1, and IFNG expression, which identified the above 23 immune cells for our immune classification (36,37). We next revealed two distinct immune subtypes on the basis of the identified immune infiltration, which exhibited robust correlation with prognosis and immune subtypes in different patients. Immunosubtype A, which is characterized by extensive immune infiltration in the TME, mainly participated in the pathways related to immune activation, which exerted better prognosis, underlying the potential advantages and better efficacy of immunotherapy. Further, the genetic aberrations and epigenetic mechanisms were investigated underlying the heterogeneity of the two subtypes. TMB, measured as the total number of unique mutations per coding area of tumor genome, has served as a standard to characterize tumor antigenicity and to predict response or resistance to ICIs. TMB has been identified in melanoma, lung, and bladder cancer to be related to a relatively potent response to ICB and is used for the guidance of PD-1 inhibitor therapy (38,39). Notably, the immunosubtype A, with a relatively high frequency of TMB, exhibited better OS and DSS, probably attributing to the novel antigens and termed neoantigens (39). Epigenetic aberrations have also been noted to be related to ICB therapeutic response beyond genomic and transcriptomic alterations. It has long been demonstrated that DNA methylation contributes to the growth and development of NSCLC and other malignancies (40,41). Therefore, the differences in CNVs frequency, TMB, and DNA methylation between the two immune subtypes demonstrate the rationality of the immune subtypes.
Additionally, a risk score model was established utilizing univariate regression, LASSO regression, and multivariate regression to identify the association between immune subtypes and prognosis. In this study, the risk score model was based on nine genes, including TIMP1, HSDL1, TENT5C, AL606489.1, LINC01117, MSLNL, E2F7, CDC42EP2, and FAM207A. We noted that the qPCR finding seemingly contradicted our previous result which showed that increased DEGs expression was substantially related with a better prognosis in LUAD patients. We speculated that the infiltrating immune cells had considerably high expression of DEGs. So, we looked into the relationship between immune infiltration and DEGs in more detail. Our findings further demonstrated that the higher the expression of most DEGs, the lower the degree of immune cell infiltration, and the worse the prognosis of patients. It clarified that the key to immunosubtype classification is the impact of gene expression differentials on immune cell infiltration.
Due to the inhibitory effects on matrix metalloproteinases, TIMP1 is a crucial regulator of extracellular matrix turnover and has been historically thought to have tumor suppressive properties (42). However, TIMP-1 has been recently revealed as a signaling molecule and was a potential biomarker for unfavorable prognosis via activation of the PI3K/Akt pathway (43). As for E2F7 and AL606489.1, E2F7 is a CD8 T cell-related gene and AL606489.1 is a pyroptosis-related lncRNA, both of which have been reported as reliable and effective indicators for predicting LUAD prognosis (44,45). However, other genes have been poorly studied in LUAD, which may point to a direction for further research.
Considering LUAD a sexually dimorphic disorder, we performed survival analyzes for females and males with subtype classification and risk score model. We concluded that subtype classification appears to show better fit for females than for males, whereas a more essential and optimized risk score model has superior predictive effect for both males and females. In general, women mount an immunological response against LUAD that is more robust and organized. As evidenced by the increased expression of various immune checkpoint markers and the larger abundance of immune suppressive cells in the TME, LUAD in women develops more complicated and redundant mechanisms of resistance to avoid effective initial immune identification and response (46-48). In other words, immune infiltration exerts a key role in female LUAD patients, which explains why this model is more suitable for females. Collectively, these findings may pave the way for specific LUAD therapeutic strategies for women only, which may achieve response and curative effect.
Moreover, we assessed the risk score model’s capacity to predict outcomes in order to assess the prognostic significance of the immune subtypes. According to the DCA curve and nomogram, a considerable prognostic value was obtained by the risk score in comparison with some clinical features.
Apart from the data from TCGA, we selected LUAD patients from ctr-db with immunotherapy outcomes to test the practical function of the immunosubtypes. The results also verified the previous conclusion.
Nevertheless, prediction models established based on immune infiltration are invasive and complicated, which are not conducive to promoting the evaluation of specialized immunotherapy. Emerging studies have revealed that features derived from radiomics are inextricably linked to clinical prognosis and response to immunotherapy (16,49). Within comparison to tissue-based immune studies, radiological imaging offers a comprehensive view of tumor features and tumor immune contexture (18). In recent research, Yang et al. constructed radiomics nomogram models incorporating CT image-based radiomics and clinicopathological factors, which exhibited utility in the guidance of ICB efficacy to NSCLC patients (50). Ligero et al. also developed a radiomics signature to classify patients who will benefit from ICB more in advanced solid tumors including bladder and lung cancers (51). Based on these studies, we further demonstrated that the CT-based radiomics model can successfully stratify most patients into A and B immunosubtypes with a high AUC value, sensitivity, and specificity. Based on these studies, we went on to show that the CT-based radiomics model is capable of accurately classifying the majority of patients into the A and B immunosubtypes while maintaining a high AUC value, sensitivity, and specificity. By integrating radiological imaging with immune subtypes of the tumor, our established radiomics model can serve as a more precise noninvasive prediction tool to predict the efficacy of ICB and prognosis with significant application prospects. Nevertheless, limitations still exist in our study. The majority of the data we examined came from internet databases, and we predicted that additional studies using larger samples would confirm our findings. In order to expand the range of potential therapeutic treatments, we also need to further clarify the mechanism by which immune infiltration impacts the prognosis of LUAD patients. The predictive importance of our radiomics signature should be confirmed in the future through the use of large samples and multimodal research.
Conclusions
We distinguished two subtypes of LUAD patients on the basis of the immune infiltration and innovatively combined them with radiomics signatures to provide a noninvasive prediction of the ICIs based immunotherapy response and survival outcomes with taking into account sex difference. More technical and machine learning advances are anticipated to unravel reliable imaging biomarkers integrating the radiomics field, which will open the door for the clinical translation in precise cancer immunotherapy guidance.
Acknowledgments
We are grateful to Yiyou Creative Space for their support of this study.
Footnote
Reporting Checklist: The authors have completed the TRIPOD + AI reporting checklist. Available at https://qims.amegroups.com/article/view/10.21037/qims-24-130/rc
Funding: This work was supported by
Conflicts of Interest: All authors have completed the ICMJE uniform disclosure form (available at https://qims.amegroups.com/article/view/10.21037/qims-24-130/coif). The authors have no conflicts of interest to declare.
Ethical Statement: The authors are accountable for all aspects of the work in ensuring that questions related to the accuracy or integrity of any part of the work are appropriately investigated and resolved. The study was conducted in accordance with the Declaration of Helsinki (as revised in 2013). The study was approved by the institutional ethics board of the Third Xiangya Hospital (approval No. Express 23466) and the requirement for individual consent for this retrospective analysis was waived.
Open Access Statement: This is an Open Access article distributed in accordance with the Creative Commons Attribution-NonCommercial-NoDerivs 4.0 International License (CC BY-NC-ND 4.0), which permits the non-commercial replication and distribution of the article with the strict proviso that no changes or edits are made and the original work is properly cited (including links to both the formal publication through the relevant DOI and the license). See: https://creativecommons.org/licenses/by-nc-nd/4.0/.
References
- Denisenko TV, Budkevich IN, Zhivotovsky B. Cell death-based treatment of lung adenocarcinoma. Cell Death Dis 2018;9:117. [Crossref] [PubMed]
- Chen J, Yang H, Teo ASM, Amer LB, Sherbaf FG, Tan CQ, et al. Genomic landscape of lung adenocarcinoma in East Asians. Nat Genet 2020;52:177-86. [Crossref] [PubMed]
- Yi M, Qin S, Zhao W, Yu S, Chu Q, Wu K. The role of neoantigen in immune checkpoint blockade therapy. Exp Hematol Oncol 2018;7:28. [Crossref] [PubMed]
- Yi M, Li A, Zhou L, Chu Q, Luo S, Wu K. Immune signature-based risk stratification and prediction of immune checkpoint inhibitor's efficacy for lung adenocarcinoma. Cancer Immunol Immunother 2021;70:1705-19. [Crossref] [PubMed]
- Santarpia M, Aguilar A, Chaib I, Cardona AF, Fancelli S, Laguia F, Bracht JWP, Cao P, Molina-Vila MA, Karachaliou N, Rosell R. Non-Small-Cell Lung Cancer Signaling Pathways, Metabolism, and PD-1/PD-L1 Antibodies. Cancers (Basel) 2020.
- Gettinger SN, Horn L, Gandhi L, Spigel DR, Antonia SJ, Rizvi NA, et al. Overall Survival and Long-Term Safety of Nivolumab (Anti-Programmed Death 1 Antibody, BMS-936558, ONO-4538) in Patients With Previously Treated Advanced Non-Small-Cell Lung Cancer. J Clin Oncol 2015;33:2004-12. [Crossref] [PubMed]
- Liao Y, He D, Wen F. Analyzing the characteristics of immune cell infiltration in lung adenocarcinoma via bioinformatics to predict the effect of immunotherapy. Immunogenetics 2021;73:369-80. [Crossref] [PubMed]
- Zhang J, Han X, Lin L, Chen J, Wang F, Ding Q, Hao L, Wang L, Wei J, Wang Y, Pan Y. Unraveling the Expression Patterns of Immune Checkpoints Identifies New Subtypes and Emerging Therapeutic Indicators in Lung Adenocarcinoma. Oxid Med Cell Longev 2022;2022:3583985. [Crossref] [PubMed]
- Li Y, Mao AS, Seo BR, Zhao X, Gupta SK, Chen M, Han YL, Shih TY, Mooney DJ, Guo M. Compression-induced dedifferentiation of adipocytes promotes tumor progression. Sci Adv 2020;6:eaax5611. [Crossref] [PubMed]
- Giraldo NA, Becht E, Vano Y, Petitprez F, Lacroix L, Validire P, Sanchez-Salas R, Ingels A, Oudard S, Moatti A, Buttard B, Bourass S, Germain C, Cathelineau X, Fridman WH, Sautès-Fridman C. Tumor-Infiltrating and Peripheral Blood T-cell Immunophenotypes Predict Early Relapse in Localized Clear Cell Renal Cell Carcinoma. Clin Cancer Res 2017;23:4416-28. [Crossref] [PubMed]
- Smida T, Bruno TC, Stabile LP. Influence of Estrogen on the NSCLC Microenvironment: A Comprehensive Picture and Clinical Implications. Front Oncol 2020;10:137. [Crossref] [PubMed]
- Zhang J, Zhang Z, Song W, Liu J. EPHA5 mutation impairs natural killer cell-mediated cytotoxicity against non-small lung cancer cells and promotes cancer cell migration and invasion. Mol Cell Probes 2020;52:101566. [Crossref] [PubMed]
- Zhang Y, Yang M, Ng DM, Haleem M, Yi T, Hu S, Zhu H, Zhao G, Liao Q. Multi-omics Data Analyses Construct TME and Identify the Immune-Related Prognosis Signatures in Human LUAD. Mol Ther Nucleic Acids 2020;21:860-73. [Crossref] [PubMed]
- Liang J, Hong J, Tang X, Qiu X, Zhu K, Zhou L, Guo D. Sex difference in response to non-small cell lung cancer immunotherapy: an updated meta-analysis. Ann Med 2022;54:2606-16. [Crossref] [PubMed]
- Conforti F, Pala L, Pagan E, Bagnardi V, De Pas T, Queirolo P, et al. Sex-Based Dimorphism of Anticancer Immune Response and Molecular Mechanisms of Immune Evasion. Clin Cancer Res 2021;27:4311-24. [Crossref] [PubMed]
- He B, Dong D, She Y, Zhou C, Fang M, Zhu Y, Zhang H, Huang Z, Jiang T, Tian J, Chen C. Predicting response to immunotherapy in advanced non-small-cell lung cancer using tumor mutational burden radiomic biomarker. J Immunother Cancer 2020;8:e000550. [Crossref] [PubMed]
- Cottin V. Lung biopsy in interstitial lung disease: balancing the risk of surgery and diagnostic uncertainty. Eur Respir J 2016;48:1274-7. [Crossref] [PubMed]
- Wu J, Mayer AT, Li R. Integrated imaging and molecular analysis to decipher tumor microenvironment in the era of immunotherapy. Semin Cancer Biol 2022;84:310-28. [Crossref] [PubMed]
- Gillies RJ, Kinahan PE, Hricak H. Radiomics: Images Are More than Pictures, They Are Data. Radiology 2016;278:563-77. [Crossref] [PubMed]
- Wu J, Cui Y, Sun X, Cao G, Li B, Ikeda DM, Kurian AW, Li R. Unsupervised Clustering of Quantitative Image Phenotypes Reveals Breast Cancer Subtypes with Distinct Prognoses and Molecular Pathways. Clin Cancer Res 2017;23:3334-42. [Crossref] [PubMed]
- Tomczak K, Czerwińska P, Wiznerowicz M. The Cancer Genome Atlas (TCGA): an immeasurable source of knowledge. Contemp Oncol (Pozn) 2015;19:A68-77. [Crossref] [PubMed]
- Grossman RL, Heath AP, Ferretti V, Varmus HE, Lowy DR, Kibbe WA, Staudt LM. Toward a Shared Vision for Cancer Genomic Data. N Engl J Med 2016;375:1109-12. [Crossref] [PubMed]
- Ru B, Wong CN, Tong Y, Zhong JY, Zhong SSW, Wu WC, Chu KC, Wong CY, Lau CY, Chen I, Chan NW, Zhang J. TISIDB: an integrated repository portal for tumor-immune system interactions. Bioinformatics 2019;35:4200-2. [Crossref] [PubMed]
- Goldman MJ, Craft B, Hastie M, Repečka K, McDade F, Kamath A, Banerjee A, Luo Y, Rogers D, Brooks AN, Zhu J, Haussler D. Visualizing and interpreting cancer genomics data via the Xena platform. Nat Biotechnol 2020;38:675-8. [Crossref] [PubMed]
- Clark K, Vendt B, Smith K, Freymann J, Kirby J, Koppel P, Moore S, Phillips S, Maffitt D, Pringle M, Tarbox L, Prior F. The Cancer Imaging Archive (TCIA): maintaining and operating a public information repository. J Digit Imaging 2013;26:1045-57. [Crossref] [PubMed]
- Barbie DA, Tamayo P, Boehm JS, Kim SY, Moody SE, Dunn IF, et al. Systematic RNA interference reveals that oncogenic KRAS-driven cancers require TBK1. Nature 2009;462:108-12. [Crossref] [PubMed]
- Jin Y, Wang Z, He D, Zhu Y, Chen X, Cao K. Identification of novel subtypes based on ssGSEA in immune-related prognostic signature for tongue squamous cell carcinoma. Cancer Med 2021;10:8693-707. [Crossref] [PubMed]
- Xue Y, Tong L. LiuAnwei Liu F, Liu A, Zeng S, Xiong Q, Yang Z, He X, Sun Y, Xu C. Tumor-infiltrating M2 macrophages driven by specific genomic alterations are associated with prognosis in bladder cancer. Oncol Rep 2019;42:581-94. [Crossref] [PubMed]
- Hänzelmann S, Castelo R, Guinney J. GSVA: gene set variation analysis for microarray and RNA-seq data. BMC Bioinformatics 2013;14:7. [Crossref] [PubMed]
- Li T, Fu J, Zeng Z, Cohen D, Li J, Chen Q, Li B, Liu XS. TIMER2.0 for analysis of tumor-infiltrating immune cells. Nucleic Acids Res 2020;48:W509-14. [Crossref] [PubMed]
- Park C, Na KJ, Choi H, Ock CY, Ha S, Kim M, Park S, Keam B, Kim TM, Paeng JC, Park IK, Kang CH, Kim DW, Cheon GJ, Kang KW, Kim YT, Heo DS. Tumor immune profiles noninvasively estimated by FDG PET with deep learning correlate with immunotherapy response in lung adenocarcinoma. Theranostics 2020;10:10838-48. [Crossref] [PubMed]
- Negrao MV, Lam VK, Reuben A, Rubin ML, Landry LL, Roarty EB, Rinsurongkawong W, Lewis J, Roth JA, Swisher SG, Gibbons DL, Wistuba II, Papadimitrakopoulou V, Glisson BS, Blumenschein GR Jr, Lee JJ, Heymach JV, Zhang J. PD-L1 Expression, Tumor Mutational Burden, and Cancer Gene Mutations Are Stronger Predictors of Benefit from Immune Checkpoint Blockade than HLA Class I Genotype in Non-Small Cell Lung Cancer. J Thorac Oncol 2019;14:1021-31. [Crossref] [PubMed]
- Rizvi H, Sanchez-Vega F, La K, Chatila W, Jonsson P, Halpenny D, et al. Molecular Determinants of Response to Anti-Programmed Cell Death (PD)-1 and Anti-Programmed Death-Ligand 1 (PD-L1) Blockade in Patients With Non-Small-Cell Lung Cancer Profiled With Targeted Next-Generation Sequencing. J Clin Oncol 2018;36:633-41. [Crossref] [PubMed]
- Wu J, Li L, Zhang H, Zhao Y, Zhang H, Wu S, Xu B. A risk model developed based on tumor microenvironment predicts overall survival and associates with tumor immunity of patients with lung adenocarcinoma. Oncogene 2021;40:4413-24. [Crossref] [PubMed]
- Xu L, Zou C, Zhang S, Chu TSM, Zhang Y, Chen W, Zhao C, Yang L, Xu Z, Dong S, Yu H, Li B, Guan X, Hou Y, Kong FM. Reshaping the systemic tumor immune environment (STIE) and tumor immune microenvironment (TIME) to enhance immunotherapy efficacy in solid tumors. J Hematol Oncol 2022;15:87. [Crossref] [PubMed]
- Ayers M, Lunceford J, Nebozhyn M, Murphy E, Loboda A, Kaufman DR, Albright A, Cheng JD, Kang SP, Shankaran V, Piha-Paul SA, Yearley J, Seiwert TY, Ribas A, McClanahan TK. IFN-γ-related mRNA profile predicts clinical response to PD-1 blockade. J Clin Invest 2017;127:2930-40. [Crossref] [PubMed]
- Schoenfeld AJ, Rizvi H, Bandlamudi C, Sauter JL, Travis WD, Rekhtman N, Plodkowski AJ, Perez-Johnston R, Sawan P, Beras A, Egger JV, Ladanyi M, Arbour KC, Rudin CM, Riely GJ, Taylor BS, Donoghue MTA, Hellmann MD. Clinical and molecular correlates of PD-L1 expression in patients with lung adenocarcinomas. Ann Oncol 2020;31:599-608. [Crossref] [PubMed]
- Huang RSP, Haberberger J, Severson E, Duncan DL, Hemmerich A, Edgerly C, et al. A pan-cancer analysis of PD-L1 immunohistochemistry and gene amplification, tumor mutation burden and microsatellite instability in 48,782 cases. Mod Pathol 2021;34:252-63. [Crossref] [PubMed]
- Morad G, Helmink BA, Sharma P, Wargo JA. Hallmarks of response, resistance, and toxicity to immune checkpoint blockade. Cell 2021;184:5309-37. [Crossref] [PubMed]
- Loo Yau H, Ettayebi I, De Carvalho DD. The Cancer Epigenome: Exploiting Its Vulnerabilities for Immunotherapy. Trends Cell Biol 2019;29:31-43. [Crossref] [PubMed]
- Dawson MA, Kouzarides T. Cancer epigenetics: from mechanism to therapy. Cell 2012;150:12-27. [Crossref] [PubMed]
- Duch P, Díaz-Valdivia N, Ikemori R, Gabasa M, Radisky ES, Arshakyan M, Gea-Sorlí S, Mateu-Bosch A, Bragado P, Carrasco JL, Mori H, Ramírez J, Teixidó C, Reguart N, Fillat C, Radisky DC, Alcaraz J. Aberrant TIMP-1 overexpression in tumor-associated fibroblasts drives tumor progression through CD63 in lung adenocarcinoma. Matrix Biol 2022;111:207-25. [Crossref] [PubMed]
- Wang Z, Li Z, Zhou K, Wang C, Jiang L, Zhang L, Yang Y, Luo W, Qiao W, Wang G, Ni Y, Dai S, Guo T, Ji G, Xu M, Liu Y, Su Z, Che G, Li W. Deciphering cell lineage specification of human lung adenocarcinoma with single-cell RNA sequencing. Nat Commun 2021;12:6500. [Crossref] [PubMed]
- Ren J, Yang Y, Li C, Xie L, Hu R, Qin X, Zhang M. A Novel Prognostic Model of Early-Stage Lung Adenocarcinoma Integrating Methylation and Immune Biomarkers. Front Genet 2020;11:634634. [Crossref] [PubMed]
- Song J, Sun Y, Cao H, Liu Z, Xi L, Dong C, Yang R, Shi Y. A novel pyroptosis-related lncRNA signature for prognostic prediction in patients with lung adenocarcinoma. Bioengineered 2021;12:5932-49. [Crossref] [PubMed]
- Fares CM, Van Allen EM, Drake CG, Allison JP, Hu-Lieskovan S. Mechanisms of Resistance to Immune Checkpoint Blockade: Why Does Checkpoint Inhibitor Immunotherapy Not Work for All Patients? Am Soc Clin Oncol Educ Book 2019;39:147-64. [Crossref] [PubMed]
- Hegde PS, Chen DS. Top 10 Challenges in Cancer Immunotherapy. Immunity 2020;52:17-35. [Crossref] [PubMed]
- Chen DS, Mellman I. Elements of cancer immunity and the cancer-immune set point. Nature 2017;541:321-30. [Crossref] [PubMed]
- Tong H, Sun J, Fang J, Zhang M, Liu H, Xia R, Zhou W, Liu K, Chen X. A Machine Learning Model Based on PET/CT Radiomics and Clinical Characteristics Predicts Tumor Immune Profiles in Non-Small Cell Lung Cancer: A Retrospective Multicohort Study. Front Immunol 2022;13:859323. [Crossref] [PubMed]
- Yang B, Zhou L, Zhong J, Lv T, Li A, Ma L, Zhong J, Yin S, Huang L, Zhou C, Li X, Ge YQ, Tao X, Zhang L, Son Y, Lu G. Combination of computed tomography imaging-based radiomics and clinicopathological characteristics for predicting the clinical benefits of immune checkpoint inhibitors in lung cancer. Respir Res 2021;22:189. [Crossref] [PubMed]
- Ligero M, Garcia-Ruiz A, Viaplana C, Villacampa G, Raciti MV, Landa J, et al. A CT-based Radiomics Signature Is Associated with Response to Immune Checkpoint Inhibitors in Advanced Solid Tumors. Radiology 2021;299:109-19. [Crossref] [PubMed]