Diagnostic accuracy of deep learning for the invasiveness assessment of ground-glass nodules with fine segmentation: a systematic review and meta-analysis
Introduction
Lung cancer remains the most commonly diagnosed malignancy and the leading cause of cancer-related deaths globally (1,2). The recent decrease in lung cancer mortality can be partly attributed to the widespread application of low-dose computed tomography (CT) for lung cancer screening, as this has contributed to the increased detection of early-stage lung adenocarcinomas manifesting as ground-glass nodules (GGNs) (3-5). GGNs, which contain haziness without obscuring bronchi or vessels on CT, may represent a range of lung adenocarcinoma lesions, including adenomatous atypical hyperplasia (AAH), adenocarcinoma in situ (AIS), microinvasive adenocarcinoma (MIA), and invasive lung adenocarcinoma (IAC) (6-8).
Currently, for patients with IAC, the standard surgical treatment is lobectomy with lymph node dissection (9,10). However, AAH, AIS, or MIA lesions typically exhibit slow growth characteristics, with a nearly 100% recurrence-free survival rate after sublobar resection and a low likelihood of lymph node metastasis (11). Therefore, for these lesions, sublobar resection or close follow-up is considered an appropriate option given better postoperative lung function without compromising surgical outcomes (12). Hence, the ability to accurately identify IAC appearing as GGNs can inform clinical decisions and help determine the surgical extent.
Advancements in artificial intelligence (AI) have given rise to deep learning (DL), a new direction in machine learning (13). DL technology directly receives raw data and gradually extracts distinctive features through multiple layers, automatically learning imperceptible underlying patterns for feature recognition and model construction (13,14). Over the past decade, DL algorithms have achieved significant success in medical image analysis, with their superior performance in image segmentation and feature extraction providing robust support for the invasiveness assessment of lung adenocarcinoma (15).
In most DL models, GGNs are delineated using bounding boxes without fine segmentation (16-18), and due to the uncertainty and randomness inherent in DL training, elimination of the interference from the surrounding structures cannot be guaranteed. Nodule segmentation can assist in classifying pulmonary nodules, and the segmented nodule mask can directly discriminate the peritumoral region from the nodule entity of the original CT image. The output of the segmentation model can be viewed as an “attention” weight map applied to the data, representing the importance of different regions for the classification task (19,20).
For the invasiveness assessment of lung adenocarcinoma, precise image segmentation techniques can effectively extract boundaries of the lesion area, providing an accurate foundation for further evaluation. Since nodule segmentation is a prerequisite for quantitative analysis and simple measurement of nodules, DL models with nodule fine segmentation also have the potential to be extended to other applications. However, systematic reviews on the accuracy of DL methods incorporating nodule fine segmentation for the invasiveness assessment of lung adenocarcinoma are lacking.
Therefore, we conducted a systematic review aimed at comprehensively evaluating the accuracy of DL models with fine nodule segmentation in assessing the invasiveness of lung adenocarcinoma. We present this article in accordance with the PRISMA-DTA reporting checklist (21) (available at https://qims.amegroups.com/article/view/10.21037/qims-24-1839/rc).
Methods
Before initiation, this study was registered in the PROSPERO (International Prospective Register of Systematic Reviews; No. CRD42024535661).
Search strategy
A thorough search was comprehensively executed up to April 4, 2024, across four databases (PubMed, Embase, Cochrane Library, and Web of Science databases) for published and unpublished studies according to the Cochrane Handbook for Systematic Evaluation of the Accuracy of Diagnostic Tests (22). A modified complete search strategy was employed to retrieve eligible studies. The following search terms were used: “isolated lung nodule”, “multiple lung nodules”, “lung nodule”, “lung cancer”, “lung adenocarcinoma”, “artificial intelligence”, “deep learning”, “convolutional neural network”, “invasiveness assessment”, “risk assessment”, and “computed tomography”. The full search strategy for keywords and subject headings for each database is provided in Appendix 1.
Study selection
Studies were included if they assessed the ability of the DL method, combined with nodule segmentation, to detect lung adenocarcinoma invasiveness via interpretation of medical images. Literature screening and management were performed to remove duplicates. Two reviewers (Chuan Gao and W.W.) independently screened the titles and abstracts of all retrieved studies to determine eligibility. Full texts of potentially relevant studies were evaluated. During the selection process, disagreements were resolved by consulting a third reviewer (Chen Gao).
The inclusion criteria were as follows: (I) the target condition was an IAC diagnosis; (II) at least one DL method was used for diagnosis; (III) nodule fine segmentation was used to aid in classification; (IV) an assessment of diagnostic accuracy with two-by-two data [true negative (TN), true positive (TP), false negative (FN), and false positive (FP)], or sufficient information to construct a 2×2 outcome table was available. Fine segmentation was considered to be the precise delineation of the edges of the nodule at each slice as the annotation mask instead of a cubic boundary box containing the nodule for model input.
The exclusion criteria were as follows: (I) studies on the benign and malignant diagnosis of lung cancer whose purpose was not the assessment of lung adenocarcinoma invasiveness; (II) studies on diseases other than lung adenocarcinoma; (III) studies on non-radiographic imaging modalities such as pathology or genetics; (IV) no data or insufficient data to construct a 2×2 outcome table; (V) reviews, editorials, letters, review articles, case reports, conference abstracts, phantom models, or animal studies; and (VI) non-English language publications.
Data collection process
Two radiologists (J.L. and W.W.) independently selected articles, extracted data, and determined the reliability of each study with regard to the total evidence. For studies that did not report diagnostic accuracy estimates directly but did provide sufficient information, calculations were performed to derive these estimates. The systematic review included results obtained from validation and testing datasets for the meta-analysis and meta-regression. Some studies did not specify the training and testing datasets; in this case, all samples were extracted (one study). If a study only provided data from the test set, the result was recorded and used for the analyses (three studies).
Extracted items included study characteristics (authors, year of publication, country, type of study, number of institutions, classification task and design, reference standard, etc.), participant characteristics (age, sex, number of patients or nodules, nodule type, location, diameter, proportion of lung adenocarcinoma subtypes, etc.), scanning parameters [modality, number of devices, kilovoltage peak (kVp), slice thickness, reconstruction kernel], and diagnostic modeling methodology (model, classification task, imaging modality, data source, sample size of training set, preprocessing methods, use of data augmentation, external validation, comparison with expert assessments, etc.), nodule segmentation-related features (automatic or manual, segmentation method, segmentation performance, comparison with classification model with no fine segmentation), classification task-related results [TP, FP, TN, FN, area under the curve (AUC), sensitivity, specificity, and accuracy].
Risk of bias and applicability
The included articles’ risk of bias and applicability were assessed using the Quality Assessment of Diagnostic Accuracy Studies 2 (QUADAS-2) tool (23) revised with topics from the Checklist for Artificial Intelligence in Medical Imaging (CLAIM) (24). Individual domains were deemed to have a low risk of bias if all requirements were assessed as yes and at high risk if one requirement was assessed as no. The remaining cases were assessed as “unclear”. The detailed evaluation criteria are provided in Appendix 2. Two reviewers (Z.S. and H.Z.) independently assess the risk of bias. Any disagreements were resolved through joint discussion and consensus with a third independent reviewer (L.W.).
Diagnostic accuracy indicators
Diagnostic accuracy metrics, including sensitivity and specificity, were used to assess the performance of the DL method, which was calculated as the percentage of positive test results for those with the target condition and the percentage of negative test results for those without the target condition, respectively (25,26). Optimal diagnostic tests have high sensitivity and specificity (27).
Data synthesis and analysis
Data were analyzed using Stata 17.0 (StataCorp., College Station, TX, USA) and RevMan 5.3 software (Cochrane Collaboration, London, UK). Meta-analysis was performed using a hierarchical model including the hierarchical summary receiver operating characteristic (HSROC) (28,29). The robustness of the pooled results was evaluated by a leave-one-out sensitivity analysis. Subgroup analysis and meta-regression was applied to explore the potential source of heterogeneity across the studies.
Certainty of evidence
The certainty of evidence was assessed using the Grading of Recommendations, Assessment, Development, and Evaluation (GRADE) criteria, with factors such as risk of bias, indirectness, inconsistency, imprecision, and publication bias being considered (30,31). The certainty of evidence was downgraded when decisions in each area were well justified.
Results
Study selection
Figure 1 illustrates the study selection process. In total, 3,141 records were identified and imported from four database searches. Subsequently, 1,173 duplicate records were removed, and 1,881 were excluded after screening for titles and abstracts. The full text of the remaining 87 records was examined and evaluated. Among these, 79 records were excluded, mainly for the reasons stated in Appendix 3. This systematic evaluation and meta-analysis included eight studies that met the inclusion criteria (32-40). Figure 2 present the architecture of application of DL with precise nodule segmentation in lung adenocarcinoma imaging. It is worth noting how the segmentation annotation input into the models varies across studies. In addition to the method shown in Figure 2, some other architecture types were examined. For model input, Wang et al. (40) preprocessed the volume of interest (VOI) covering the lesion area. Qi et al. (35) used nodule features and cropped nodule VOI as the input data into the classification pipeline. Xu et al. (38) constructed a single-DL model using gross region-of-interest (ROI) patches containing only tumor region as inputs. Wang et al. (36) multiplied the soft attention map of the segmentation branch to the input feature map of the classification branch.
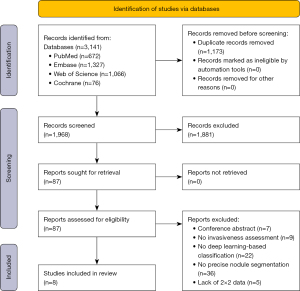
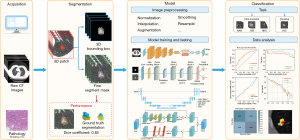
Study features
The main features of the eight selected articles are shown in Table 1, and the DL-based model features are summarized in Table 2. The patients and scan parameter features are in Tables S1,S2. The methods and results regarding segmentation are shown in Table S3. A total of 5,281 nodes and 4,676 patients were included. The studies reported the demographic characteristics of their study populations, with mean ages ranging from 46.8 to 59.5 years and the proportion of patients with IAC ranging from 22.5% to 70.8%. Geographically, seven studies were conducted in China, and one was from Korea. Of the selected articles, seven used pathologic biopsies as the gold standard, and one did not mention the type used. All studies employed CT for imaging, with only one used enhanced CT scanning.
Table 1
Author (year) | Country | Study design | Institution | Per nodule/per patient | Reference standard |
---|---|---|---|---|---|
Chunlong Fu, 2023 (37) | C | R | 3 | Per nodule | P (biopsy or surgery) |
Jun Wang, 2021 (36) | C | R | 2 | Per nodule | P (biopsy or surgery) |
Kang Qi, 2024 (35) | C | R | 1 | Per nodule | P (surgery) |
Sohee Park, 2021 (32) | S | R | 1 | Per nodule | P (surgery) |
Tianle Shen, 2021 (34) | C | R | 1 | Per patient | P (surgery) |
Xiang Wang, 2021 (40) | C | R | 1 | Per nodule | P |
Yanqiu Wang, 2021 (33) | C | R | 1 | Per nodule | NR |
Yao Xu, 2021 (38) | C | R | 1 | Per nodule | P (biopsy or surgery) |
C, China; S, South Korea; R, retrospective cohort; P, pathology; NR, not reported.
Table 2
Author (year) | Classification task | Model | Use of public database^ | Sample size of training set | Type of preprocessing | Data augmentation | External validation | AI vs. clinician | Performance of AI AUC (95% CI) | |||
---|---|---|---|---|---|---|---|---|---|---|---|---|
Training | Validation | Internal test | External test | |||||||||
Chunlong Fu, 2023 (37) | MIA/IAC | MedicalNet | No | 431 | RS; LI; ZN | Yes | Yes | No | 0.95 (0.93–0.97) | – | 0.92 (0.87–0.97) | 0.89 (0.85–0.92); 0.82 (0.76–0.88)* |
Jun Wang, 2021 (36) | AAH + AIS + MIA/IAC | IMAL-Net | LIDC-IDRI& | 1,440 | 5-F CV | Yes | Yes | No | – | 93.8±1.1$ | – | 91.8±2.2$ |
Kang Qi, 2024 (35) | AAH + AIS + MIA/IAC | Lung-PNet | LUNA 2016; MSD | 327 | RS; DTL; HOM; SWN; 5FCV | No | Yes | Yes | – | 0.885 (0.845-0.917) | 0.925 (0.845–0.971) | 0.911 (0.776–0.978)# |
Sohee Park, 2021 (32) | AAH + AIS + MIA/IAC | 3D fully CNN | No | 370 | RS; CSI; resample | Yes | Yes | Yes | 0.914£ | 0.956£ | – | 0.833 (0.744–0.923) |
Tianle Shen, 2021 (34) | AAH + AIS + MIA/IAC | AdaDense_M | No | 1,592 | RS; LI; MMN | Yes | No | No | – | – | 0.908 (0.877–0.939) | – |
Xiang Wang, 2021 (40) | AAH + AIS + MIA/IAC | 3D DenseNet | ImageNet | 687 | 5-F CV | No | No | No | – | 0.921 (0.896–0.937) | – | – |
Yanqiu Wang, 2021 (33) | AAH + AIS + MIA/IAC | Two-channel integrated network based on DenseNet | No | 282 | 4FCV | Yes | No | No | – | – | 0.9715£ | – |
Yao Xu, 2021 (38) | AAH + AIS/MIA + IAC | ResNeXt34-based CNN merged with an RNN; LSTM | TCGA-LUAD, LUSC; CPTAC-LUAD, LSCC | 123 | RS; TL; resample | Yes | No | No | – | – | 0.831 (0.690–0.926) | – |
^, training model; &, training segmentation; *, AUC of two external validation cohorts; $, mean ± standard deviation; £, 95% CI not reported; #, hold-out test set: ROC AUC =0.911; PR AUC =0.842. CI, confidence interval; AUC, area under the curve; AAH, atypical adenomatous hyperplasia; AIS, adenocarcinoma in situ; MIA, minimally invasive adenocarcinoma; IAC, invasive adenocarcinoma; IMAL, interpretable multitask attention learning; CNN, convolutional neural network; AdaDense, adaptive DenseNet; RNN, recurrent neural network; LSTM, long short-term memory; LIDC-IDRI, The Lung Image Database Consortium; LUAD, lung adenocarcinoma; LUSC/LSCC, lung squamous cell carcinoma; LUNA, lung nodule analysis; TCGA, The Cancer Genome Atlas; CPTAC, Clinical Proteomic Tumor Analysis Consortium. ROC, receiver operating characteristic; PR, precision-recall; F CV, fold cross-validation; RS, random split; MMN, Min-Max normalization; ZN, Z-score normalization; SWN, stochastic window normalization; DTL, deep transfer learning; LI, linear interpolation; CSI, cubic spline interpolation; HOM, hold-out method; MSD, Medical Segmentation Decathlon.
All studies obtained images from local hospitals. Four of the studies (n=4) used images from public databases for model training, including Lung Nodule Analysis (LUNA) 2016, The Cancer Genome Atlas-Lung Adenocarcinoma (TCGA-LUAD), Clinical Proteomic Tumor Analysis Consortium-Lung Adenocarcinoma (CPTACLUAD), The Cancer Genome Atlas-Lung Squamous Cell Carcinoma (TCGA-LUSC), and Clinical Proteomic Tumor Analysis Consortium-Lung Squamous Cell Carcinoma (CPTAC-LSCC), ImageNet, and the Lung Image Database Consortium and Image Database Resource Initiative (LIDC-IDRI).
Two studies were multicenter, and four included external validation. The classification tasks addressed in the studies varied: seven studies focused on distinguishing noninvasive adenocarcinomas (AAH, AIS, MIA) from IAC, and one study compared MIA to IAC. Six studies used data augmentation methods for data preprocessing.
Risk of bias and applicability
In the domain of patient selection, two studies were at high risk of bias owing to unclear inclusion/exclusion criteria or unclear procedures regarding patient enrollment. For the index test, five were at high risk of bias. Unclear risk of bias regarding the reference standards and the flow and timing were present in two studies and four studies, respectively.
Evidence’s applicability to patients needed to be clarified or improved in three studies, the evidence applicability to patient selection could have been clearer or improved in three studies, and the applicability of index tests was of concern for one study. The related results are shown in Figure 3 and Table S4. The Deeks’ funnel plot showed no significant publication bias in the included literature (P=0.94), as shown in Figure 4.
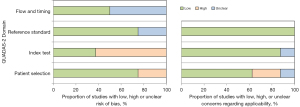
Results of the studies and synthesis
Figures 5,6 show the paired forest plots of the sensitivity and specificity estimates and corresponding 95% confidence intervals (CIs) for each independent study. The sensitivity of the eight DL images on the assessment of lung cancer invasiveness ranging from 55% to 89%, and the specificity varied between 60% and 98%.
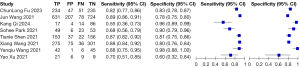
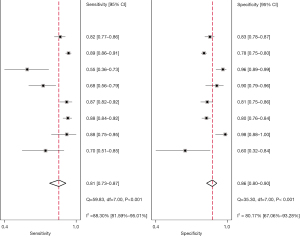
The eligible studies were further combined using the HSROC model. The summary receiver operating characteristics (SROC) curves are shown in Figure 7, including the 95% prediction region and 95% confidence region. The overall pooled estimates of DL images for lung cancer invasiveness assessment using the HSROC model were as follows: sensitivity, 0.81 (95% CI: 0.73–0.87); specificity, 0.86 (95% CI: 0.80–0.90); positive likelihood ratio (PLR), 5.6 (95% CI: 4.3–7.5); negative likelihood ratio (NLR), 0.22 (95% CI: 0.16–0.30); and diagnostic odds ratio (DOR), 25 (95% CI: 19–34). The SROC plot with the scatter of the study points in the receiving operating characteristic space revealed considerable variability in sensitivity and specificity for each study. Based on the SROC model, the AUC was 0.9 (95% CI: 0.88–0.93), indicating high diagnostic efficacy.
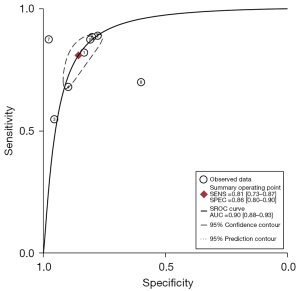
To evaluate the potential clinical application of DL-based assessment, a Fagan’s nomogram was employed. Under a pretest probability of 20%, the posttest probability of IAC increased to 59% following a positive test and decreased to 5% following a negative test, as shown in Figure 8.
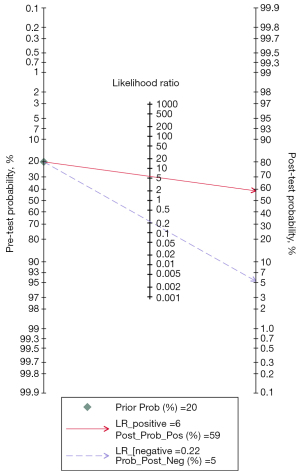
GGN segmentation and annotation
In the included studies, there were seven targeting subsolid nodules (part-solid and pure GGNs) and one targeting pure GGNs. As for the segmentation approach, if the annotation mask covering the entire range of each tumor labeled by clinicians on software (e.g., ITK-SNAP) was used as the input for the classification task, we considered the model to involve manual segmentation techniques. If the built-in subnetwork of the DL model could automatically and precisely segment lung nodule boundaries to serve as the auxiliary in the classification task, we considered the model to involve an automatic segmentation technique. Table S3 shows that half of the studies (n=4) used automatic segmentation, while the other half used manual segmentation. Four studies drew comparisons with classification models with no fine segmentation.
Sensitivity analysis, subgroup analyses, and meta-regression
In the sensitivity analysis, removing any one study did not significantly affect the pooled results, indicating the reliability and robustness of this meta-analysis (Figure 9). Table 3 shows the detailed results of meta-regression analyses regarding the possible causes and sources of interstudy heterogeneity. The results showed that the application of automated or manual segmentation techniques was not associated with heterogeneity. In terms of data source, whether a public database or external validation was used had no statistical effect on the heterogeneity. Regarding the sample size of the validation or test set, the P value of the group with a sample size ≥150 and <150 was 0.01, indicating that a sample size less than 150 was a possible source of heterogeneity. The subgroup analyses produced similar results, as shown in Figure 10.
Table 3
Subgroup variable | Number of eligible studies | Sensitivity, % (95% CI) | Specificity, % (95 % CI) | P value |
---|---|---|---|---|
Segment method | 0.27 | |||
Automatic | 4 | 0.77 (0.67–0.86) | 0.89 (0.83–0.95) | |
Manual | 4 | 0.85 (0.78–0.92) | 0.82 (0.75–0.89) | |
Use of public data | 0.89 | |||
Yes | 4 | 0.81 (0.71–0.91) | 0.85 (0.78–0.92) | |
No | 4 | 0.81 (0.71–0.91) | 0.86 (0.80–0.92) | |
Sample size* | 0.01 | |||
≥150 | 4 | 0.87 (0.84–0.90) | 0.80 (0.77–0.83) | |
<150 | 4 | 0.71 (0.63–0.80) | 0.92 (0.88–0.96) | |
External validation | 0.39 | |||
Yes | 4 | 0.77 (0.67–0.87) | 0.88 (0.82–0.93) | |
No | 4 | 0.85 (0.78–0.93) | 0.84 (0.77–0.91) |
*, sample size in the validation or testing set. CI, confidence interval.
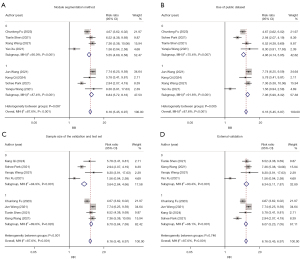
Comparison of DL and healthcare professional classification performance
Two of the studies compared the diagnostic performance between DL-based methods and healthcare professionals. In one study conducted by Qi et al. (35), several evaluation metrics were calculated to compare the independent diagnosis of GGN pathological subtypes by several observers with that of a DL model. In study conducted by Park et al. (32), several clinicians measured the longest diameter of the solid component of each nodule and calculated the predictive performance for differentiating IAC from non-IAC under multiple thresholds. The detailed results are provided in the Table S5.
Certainty of evidence
Each reviewer independently assessed the overall certainty of the evidence. In multiple studies, the risk of bias, indirectness, and consistency domains was downgraded, with a high risk of bias for the index test and patient selection and large differences in specificity and sensitivity. Based on the GRADE for diagnostic tests, the certainty of evidence for the studies on the accuracy of DL image tests was rated as very low for both sensitivity and specificity estimates (Table S6).
Discussion
To the best of our knowledge, this is the first meta-analysis evaluating the accuracy of diagnosis of DL models with precise nodule segmentation in diagnosing lung adenocarcinoma invasiveness. Meta-analysis results showed a pooled sensitivity of 0.81 and a specificity of 0.86, the area under the SROC curve was 0.90, indicating high diagnostic efficacy. However, despite the high discriminative power of the DL algorithm evaluated in this meta-analysis, the GRADE score was very low due to a high degree of bias in patient selection and index test, indirectness, and the low inconsistency for the sensitivity and specificity. This means that we have limited confidence in the diagnostic performance, and the results should be interpreted with caution. The main sources of risk factors were from inconsistent inclusion/exclusion criteria and lack of external validation, limiting the generalizability of the model. Furthermore, it was difficult to identify the specific methodology used to develop the DL classification model, potentially diminishing the repeatability and quality of these models. Although the result of sensitivity analysis and meta-regression indicate the stability of the results, the results are not completely reliable; therefore, additional external validation using updated multicenter data and larger sample size is necessary to ensure the applicability of the data and reduce uncertainty. Overall, the available evidence needs to be further validated and strengthened before it can reliably inform clinical practice (41).
Previous meta-analysis results indicate that DL can be valuable in lung cancer screening, detection, and staging (42-46). It has also been demonstrated that AI techniques can more accurately determine the histopathological subtypes of lung nodules and the degree of adenocarcinoma invasiveness (20), thus assisting in planning lung nodule diagnosis and treatment. However, many existing DL models for lung nodule classification are trained with nodule-centered bounding boxes as inputs. Consequently, the effectiveness of fine segmentation of nodule boundaries on lung nodule classification has yet to be extensively explored (47).
In nodule annotation methodologies, clinicians manually trace the boundary of nodule slice by slice with the lung and/or mediastinal window via software and then directly input the annotation as the segmentation mask into the model. In models using automatic segmentation technology, the clinician’s annotation will first be used as the ground truth to train the model subnetwork to automatically segment nodules and to construct a model with both automatic segmentation and classification capabilities. Our analysis showed that in the subgroup analysis and meta-regression of various nodule segmentation annotation techniques, no significant impact on the pooled results of diagnostic performance was found between the application of automatic and manual segmentation. In addition, three studies (35,37,40) excluded the large blood vessels and/or bronchi within nodules during the labeling process. Kou et al. (48) found that CT characteristics such as bronchial inflation signs can be used for the differentiation of IAC from AAH, AIS, or MIA. Zhao et al. (49) found that that the number and volume of intranodular vessels on GGN were associated with tumor invasiveness. Therefore, we can speculate that the removal of large vessels and bronchi labeling may result in a negative impact on the predictive performance of the classification model. In clinical practice, nodule-related parameters obtained from automatic segmentation can also help analyze nodules (50,51).
Accurate annotation of pulmonary nodule boundaries can provide attention weights to help network focus on the area within the nodule for the analysis and classification of nodules (20,47). Several deep models have been used to automatically segment lung nodules and have achieved remarkable accuracy (52,53). Wu et al. (54) noted that there were differences between IAC and MIA/AIS in terms of radiomic features of cluster prominence and the gray-level run-length matrix in the surrounding area of the tumor. It was also observed that the lesions had interclass similarity and intraclass variation, which is one of the main challenges in IAC screening. However, as most of the subsolid nodules’ sizes were small, noise and artifacts were present in the background in the cropped image patches (34). Indeed, automatic segmentation has higher consistency than does the laborious and time-consuming manual segmentation. This suggests that it is feasible for DL methods to combine segmentation and classification and use automatic segmentation to assist in the diagnosis of adenocarcinoma invasiveness. Nonetheless, further studies are needed to analyze the value of peritumoral information for classification performance (40).
Although an abundance of research has demonstrated the efficacy and potential of DL in medical imaging, certain challenges limit its application to real-world situations. First, the “black box” nature of DL models renders their decision-making processes difficult to interpret (55). Exploring explicable DL methods such as attentional mechanisms and visualization techniques can provide an intuitive understanding of how algorithms arrive at decisions and increase physician confidence (56). Second, the research community has yet to reach a consensus regarding the specific datasets that are used in terms of extensibility and reproducibility, although the volume of publicly available medical data is encouraging advance (57). Implementing DL imaging tools requires the appropriate resources, including high-performance computing and data storage capabilities, such as graphic processing units (GPUs), servers, and cloud platforms (58). Third, integrating DL tools into the workflows requires the development of tools compatible with existing healthcare information systems and the design of user-friendly interfaces that allow clinicians to easily integrate DL tools into daily work (59). This requires education and training for clinicians to help them understand how DL tools work, their strengths and limitations, and how to integrate the outputs of these tools into clinical decision-making. Fourth, many models have been developed and constructed for specific scenarios (e.g., for diagnosing a single disease), yet in clinical practice, GGNs can appear in many other diseases with varying prognoses, such as coronavirus disease 2019 (COVID-19) (60,61). Therefore, future work should investigate the implications of incorporating DL-based computer-aided detection schemes in real-world clinical practice, and reader studies should be conducted to examine clinician performance (62).
Some limitations to this study should be addressed. First, our study only considered the DL method for invasive classification of lung adenocarcinoma using nodule fine segmentation, and many published studies without precise nodule segmentation need to be further analyzed. Second, differences between studies, including study design, data source and scanner type, and imaging acquisition standards, potentially reduced the accuracy of the integration and inference of results. Third, due to the limited study types and geographic restriction to the Asian region, the generalizability of the results may be limited. Additional large-scale, multicenter studies are needed to assess the ability of DL to evaluate lung adenocarcinoma invasiveness.
Conclusions
Our findings support the application of DL models that integrate automatic segmentation and classification of local data to evaluate the degree of lung adenocarcinoma invasiveness. Overall, DL models enhanced by nodule fine segmentation possess high accuracy in the assessment of lung adenocarcinoma invasiveness. Future studies should address bias and uncertainty and explore the applicability these models to different patient populations and clinical contexts.
Acknowledgments
None.
Footnote
Reporting Checklist: The authors have completed the PRISMA-DTA reporting checklist. Available at https://qims.amegroups.com/article/view/10.21037/qims-24-1839/rc
Funding: This work was supported by
Conflicts of Interest: All authors have completed the ICMJE uniform disclosure form (available at https://qims.amegroups.com/article/view/10.21037/qims-24-1839/coif). The authors have no conflicts of interest to declare.
Ethical Statement: The authors are accountable for all aspects of the work in ensuring that questions related to the accuracy or integrity of any part of the work are appropriately investigated and resolved.
Open Access Statement: This is an Open Access article distributed in accordance with the Creative Commons Attribution-NonCommercial-NoDerivs 4.0 International License (CC BY-NC-ND 4.0), which permits the non-commercial replication and distribution of the article with the strict proviso that no changes or edits are made and the original work is properly cited (including links to both the formal publication through the relevant DOI and the license). See: https://creativecommons.org/licenses/by-nc-nd/4.0/.
References
- Siegel RL, Giaquinto AN, Jemal A. Cancer statistics, 2024. CA Cancer J Clin 2024;74:12-49. [Crossref] [PubMed]
- Sung H, Ferlay J, Siegel RL, Laversanne M, Soerjomataram I, Jemal A, Bray F. Global Cancer Statistics 2020: GLOBOCAN Estimates of Incidence and Mortality Worldwide for 36 Cancers in 185 Countries. CA Cancer J Clin 2021;71:209-49. [Crossref] [PubMed]
- de Koning HJ, van der Aalst CM, de Jong PA, Scholten ET, Nackaerts K, Heuvelmans MA, et al. Reduced Lung-Cancer Mortality with Volume CT Screening in a Randomized Trial. N Engl J Med 2020;382:503-13. [Crossref] [PubMed]
- Church TR, Black WC, Aberle DR, Berg CD, Clingan KL, Duan F, Fagerstrom RM, Gareen IF, Gierada DS, Jones GC, Mahon I, Marcus PM, Sicks JD, Jain A, Baum S. Results of initial low-dose computed tomographic screening for lung cancer. N Engl J Med 2013;368:1980-91. [Crossref] [PubMed]
- Goo JM, Park CM, Lee HJ. Ground-glass nodules on chest CT as imaging biomarkers in the management of lung adenocarcinoma. AJR Am J Roentgenol 2011;196:533-43. [Crossref] [PubMed]
- Travis WD, Brambilla E, Noguchi M, Nicholson AG, Geisinger KR, Yatabe Y, et al. International association for the study of lung cancer/american thoracic society/european respiratory society international multidisciplinary classification of lung adenocarcinoma. J Thorac Oncol 2011;6:244-85. [Crossref] [PubMed]
- Aoki T. Growth of pure ground-glass lung nodule detected at computed tomography. J Thorac Dis 2015;7:E326-8. [Crossref] [PubMed]
- MacMahon H, Naidich DP, Goo JM, Lee KS, Leung ANC, Mayo JR, Mehta AC, Ohno Y, Powell CA, Prokop M, Rubin GD, Schaefer-Prokop CM, Travis WD, Van Schil PE, Bankier AA. Guidelines for Management of Incidental Pulmonary Nodules Detected on CT Images: From the Fleischner Society 2017. Radiology 2017;284:228-43. [Crossref] [PubMed]
- Yotsukura M, Muraoka Y, Yoshida Y, Nakagawa K, Shiraishi K, Kohno T, Yatabe Y, Watanabe SI. Long-Term Prognosis and Prognostic Indicators of Stage IA Lung Adenocarcinoma. Ann Surg Oncol 2023;30:851-8. [Crossref] [PubMed]
- Saji H, Okada M, Tsuboi M, Nakajima R, Suzuki K, Aokage K, et al. Segmentectomy versus lobectomy in small-sized peripheral non-small-cell lung cancer (JCOG0802/WJOG4607L): a multicentre, open-label, phase 3, randomised, controlled, non-inferiority trial. Lancet 2022;399:1607-17. [Crossref] [PubMed]
- Jia M, Yu S, Cao L, Sun PL, Gao H. Clinicopathologic Features and Genetic Alterations in Adenocarcinoma In Situ and Minimally Invasive Adenocarcinoma of the Lung: Long-Term Follow-Up Study of 121 Asian Patients. Ann Surg Oncol 2020;27:3052-63. [Crossref] [PubMed]
- Yotsukura M, Asamura H, Motoi N, Kashima J, Yoshida Y, Nakagawa K, Shiraishi K, Kohno T, Yatabe Y, Watanabe SI. Long-Term Prognosis of Patients With Resected Adenocarcinoma In Situ and Minimally Invasive Adenocarcinoma of the Lung. J Thorac Oncol 2021;16:1312-20. [Crossref] [PubMed]
- LeCun Y, Bengio Y, Hinton G. Deep learning. Nature 2015;521:436-44. [Crossref] [PubMed]
- Esteva A, Robicquet A, Ramsundar B, Kuleshov V, DePristo M, Chou K, Cui C, Corrado G, Thrun S, Dean J. A guide to deep learning in healthcare. Nat Med 2019;25:24-9. [Crossref] [PubMed]
- Ashraf SF, Yin K, Meng CX, Wang Q, Wang Q, Pu J, Dhupar R. Predicting benign, preinvasive, and invasive lung nodules on computed tomography scans using machine learning. J Thorac Cardiovasc Surg 2022;163:1496-1505.e10. [Crossref] [PubMed]
- Lv Y, Wei Y, Xu K, Zhang X, Hua R, Huang J, et al. 3D deep learning versus the current methods for predicting tumor invasiveness of lung adenocarcinoma based on high-resolution computed tomography images. Front Oncol 2022;12:995870. [Crossref] [PubMed]
- Zhou J, Hu B, Feng W, Zhang Z, Fu X, Shao H, Wang H, Jin L, Ai S, Ji Y. An ensemble deep learning model for risk stratification of invasive lung adenocarcinoma using thin-slice CT. NPJ Digit Med 2023;6:119. [Crossref] [PubMed]
- Kim H, Lee D, Cho WS, Lee JC, Goo JM, Kim HC, Park CM. CT-based deep learning model to differentiate invasive pulmonary adenocarcinomas appearing as subsolid nodules among surgical candidates: comparison of the diagnostic performance with a size-based logistic model and radiologists. Eur Radiol 2020;30:3295-305. [Crossref] [PubMed]
- Wu W, He Z, Xu J, Wen W, Wang J, Zhu Q, Chen L. Anatomical Pulmonary Sublobar Resection Based on Subsegment. Ann Thorac Surg 2021;111:e447-50. [Crossref] [PubMed]
- Zhao W, Yang J, Sun Y, Li C, Wu W, Jin L, Yang Z, Ni B, Gao P, Wang P, Hua Y, Li M. 3D Deep Learning from CT Scans Predicts Tumor Invasiveness of Subcentimeter Pulmonary Adenocarcinomas. Cancer Res 2018;78:6881-9. [Crossref] [PubMed]
- McInnes MDF, Moher D, Thombs BD, McGrath TA, Bossuyt PM, et al. Preferred Reporting Items for a Systematic Review and Meta-analysis of Diagnostic Test Accuracy Studies: The PRISMA-DTA Statement. JAMA 2018;319:388-96. [Crossref] [PubMed]
- Spijker R, Dinnes J, Glanville J, Eisinga A. Searching for and selecting studies. Cochrane Handbook for Systematic Reviews of Diagnostic Test Accuracy. John Wiley & Sons, Ltd., Chichester, UK; 2023:97-129.
- Whiting PF, Rutjes AW, Westwood ME, Mallett S, Deeks JJ, Reitsma JB, Leeflang MM, Sterne JA, Bossuyt PM. QUADAS-2 Group. QUADAS-2: a revised tool for the quality assessment of diagnostic accuracy studies. Ann Intern Med 2011;155:529-36. [Crossref] [PubMed]
- Tejani AS, Klontzas ME, Gatti AA, Mongan JT, Moy L, Park SH, Kahn CE JrCLAIM 2024 Update Panel. Checklist for Artificial Intelligence in Medical Imaging (CLAIM): 2024 Update. Radiol Artif Intell 2024;6:e240300. [Crossref] [PubMed]
- Campbell JM, Klugar M, Ding S, Carmody DP, Hakonsen SJ, Jadotte YT, White S, Munn Z. Diagnostic test accuracy: methods for systematic review and meta-analysis. Int J Evid Based Healthc 2015;13:154-62. [Crossref] [PubMed]
- Mandrekar JN. Simple statistical measures for diagnostic accuracy assessment. J Thorac Oncol 2010;5:763-4. [Crossref] [PubMed]
- Leeflang MMG, Allerberger F. How to: evaluate a diagnostic test. Clin Microbiol Infect 2019;25:54-9. [Crossref] [PubMed]
- Takwoingi Y, Dendukuri N, Schiller I, Rücker G, Jones HE, Partlett C, Macaskill P. Undertaking meta-analysis. Cochrane Handbook for Systematic Reviews of Diagnostic Test Accuracy. John Wiley & Sons, Ltd., Chichester, UK; 2023:249-325.
- Lee YH. Overview of the Process of Conducting Meta-analyses of the Diagnostic Test Accuracy. Journal of Rheumatic Diseases 2018;25:3.
- Schünemann HJ, Mustafa RA, Brozek J, Steingart KR, Leeflang M, Murad MH, et al. GRADE guidelines: 21 part 1. Study design, risk of bias, and indirectness in rating the certainty across a body of evidence for test accuracy. J Clin Epidemiol 2020;122:129-41. [Crossref] [PubMed]
- Schünemann HJ, Mustafa RA, Brozek J, Steingart KR, Leeflang M, Murad MH, et al. GRADE guidelines: 21 part 2. Test accuracy: inconsistency, imprecision, publication bias, and other domains for rating the certainty of evidence and presenting it in evidence profiles and summary of findings tables. J Clin Epidemiol 2020;122:142-52. [Crossref] [PubMed]
- Park S, Park G, Lee SM, Kim W, Park H, Jung K, Seo JB. Deep learning-based differentiation of invasive adenocarcinomas from preinvasive or minimally invasive lesions among pulmonary subsolid nodules. Eur Radiol 2021;31:6239-47. [Crossref] [PubMed]
- Wang Y, Yue S, Chen J, Li Q. Segmentation and classification of ground glass nodule on CT images. Int J Imaging Syst Technol 2021;31:2204-13.
- Shen T, Hou R, Ye X, Li X, Xiong J, Zhang Q, Zhang C, Cai X, Yu W, Zhao J, Fu X. Predicting Malignancy and Invasiveness of Pulmonary Subsolid Nodules on CT Images Using Deep Learning. Front Oncol 2021;11:700158. [Crossref] [PubMed]
- Qi K, Wang K, Wang X, Zhang YD, Lin G, Zhang X, Liu H, Huang W, Wu J, Zhao K, Liu J, Li J, Zhang X. Lung-PNet: An Automated Deep Learning Model for the Diagnosis of Invasive Adenocarcinoma in Pure Ground-Glass Nodules on Chest CT. AJR Am J Roentgenol 2024;222:e2329674. [Crossref] [PubMed]
- Wang J, Yuan C, Han C, Wen Y, Lu H, Liu C, She Y, Deng J, Li B, Qian D, Chen C. IMAL-Net: Interpretable multi-task attention learning network for invasive lung adenocarcinoma screening in CT images. Med Phys 2021;48:7913-29. [Crossref] [PubMed]
- Fu CL, Yang ZB, Li P, Shan KF, Wu MK, Xu JP, Ma CJ, Luo FH, Zhou L, Sun JH, Zhao FH. Discrimination of ground-glass nodular lung adenocarcinoma pathological subtypes via transfer learning: A multicenter study. Cancer Med 2023;12:18460-9. [Crossref] [PubMed]
- Xu Y, Li Y, Yin H, Tang W, Fan G. Consecutive Serial Non-Contrast CT Scan-Based Deep Learning Model Facilitates the Prediction of Tumor Invasiveness of Ground-Glass Nodules. Front Oncol 2021;11:725599. [Crossref] [PubMed]
- Xia X, Gong J, Hao W, Yang T, Lin Y, Wang S, Peng W. Comparison and Fusion of Deep Learning and Radiomics Features of Ground-Glass Nodules to Predict the Invasiveness Risk of Stage-I Lung Adenocarcinomas in CT Scan. Front Oncol 2020;10:418. [Crossref] [PubMed]
- Wang X, Chen K, Wang W, Li Q, Liu K, Li Q, Cui X, Tu W, Sun H, Xu S, Zhang R, Xiao Y, Fan L, Liu S. Can peritumoral regions increase the efficiency of machine-learning prediction of pathological invasiveness in lung adenocarcinoma manifesting as ground-glass nodules? J Thorac Dis 2021;13:1327-37. [Crossref] [PubMed]
- Ather S, Kadir T, Gleeson F. Artificial intelligence and radiomics in pulmonary nodule management: current status and future applications. Clin Radiol 2020;75:13-9. [Crossref] [PubMed]
- Liu X, Faes L, Kale AU, Wagner SK, Fu DJ, Bruynseels A, Mahendiran T, Moraes G, Shamdas M, Kern C, Ledsam JR, Schmid MK, Balaskas K, Topol EJ, Bachmann LM, Keane PA, Denniston AK. A comparison of deep learning performance against health-care professionals in detecting diseases from medical imaging: a systematic review and meta-analysis. Lancet Digit Health 2019;1:e271-97. [Crossref] [PubMed]
- Thong LT, Chou HS, Chew HSJ, Lau Y. Diagnostic test accuracy of artificial intelligence-based imaging for lung cancer screening: A systematic review and meta-analysis. Lung Cancer 2023;176:4-13. [Crossref] [PubMed]
- Aggarwal R, Sounderajah V, Martin G, Ting DSW, Karthikesalingam A, King D, Ashrafian H, Darzi A. Diagnostic accuracy of deep learning in medical imaging: a systematic review and meta-analysis. NPJ Digit Med 2021;4:65. [Crossref] [PubMed]
- Liu M, Wu J, Wang N, Zhang X, Bai Y, Guo J, Zhang L, Liu S, Tao K. The value of artificial intelligence in the diagnosis of lung cancer: A systematic review and meta-analysis. PLoS One 2023;18:e0273445. [Crossref] [PubMed]
- Zheng X, He B, Hu Y, Ren M, Chen Z, Zhang Z, Ma J, Ouyang L, Chu H, Gao H, He W, Liu T, Li G. Diagnostic Accuracy of Deep Learning and Radiomics in Lung Cancer Staging: A Systematic Review and Meta-Analysis. Front Public Health 2022;10:938113. [Crossref] [PubMed]
- Wang D, Zhang T, Li M, Bueno R, Jayender J. 3D deep learning based classification of pulmonary ground glass opacity nodules with automatic segmentation. Comput Med Imaging Graph 2021;88:101814. [Crossref] [PubMed]
- Kou J, Gu X, Kang L. Correlation Analysis of Computed Tomography Features and Pathological Types of Multifocal Ground-Glass Nodular Lung Adenocarcinoma. Comput Math Methods Med 2022;2022:7267036. [Crossref] [PubMed]
- Zhao B, Wang X, Sun K, Kang H, Zhang K, Yin H, Liu K, Xiao Y, Liu S. Correlation Between Intranodular Vessels and Tumor Invasiveness of Lung Adenocarcinoma Presenting as Ground-glass Nodules: A Deep Learning 3-Dimensional Reconstruction Algorithm-based Quantitative Analysis on Noncontrast Computed Tomography Images. J Thorac Imaging 2023;38:297-303. [Crossref] [PubMed]
- Ahn Y, Lee SM, Noh HN, Kim W, Choe J, Do KH, Seo JB. Use of a Commercially Available Deep Learning Algorithm to Measure the Solid Portions of Lung Cancer Manifesting as Subsolid Lesions at CT: Comparisons with Radiologists and Invasive Component Size at Pathologic Examination. Radiology 2021;299:202-10. [Crossref] [PubMed]
- Qi LL, Wang JW, Yang L, Huang Y, Zhao SJ, Tang W, Jin YJ, Zhang ZW, Zhou Z, Yu YZ, Wang YZ, Wu N. Natural history of pathologically confirmed pulmonary subsolid nodules with deep learning-assisted nodule segmentation. Eur Radiol 2021;31:3884-97. [Crossref] [PubMed]
- Lu D, Chu J, Zhao R, Zhang Y, Tian G. A Novel Deep Learning Network and Its Application for Pulmonary Nodule Segmentation. Comput Intell Neurosci 2022;2022:7124902. [Crossref] [PubMed]
- Bhattacharyya D, Thirupathi Rao N, Joshua ESN, Hu YC. A bi-directional deep learning architecture for lung nodule semantic segmentation. Vis Comput 2022; Epub ahead of print. [Crossref]
- Wu L, Gao C, Xiang P, Zheng S, Pang P, Xu M. CT-Imaging Based Analysis of Invasive Lung Adenocarcinoma Presenting as Ground Glass Nodules Using Peri- and Intra-nodular Radiomic Features. Front Oncol 2020;10:838. [Crossref] [PubMed]
- Peeters D, Alves N, Venkadesh KV, Dinnessen R, Saghir Z, Scholten ET, Schaefer-Prokop C, Vliegenthart R, Prokop M, Jacobs C. Enhancing a deep learning model for pulmonary nodule malignancy risk estimation in chest CT with uncertainty estimation. Eur Radiol 2024;34:6639-51. [Crossref] [PubMed]
- Borys K, Schmitt YA, Nauta M, Seifert C, Krämer N, Friedrich CM, Nensa F. Explainable AI in medical imaging: An overview for clinical practitioners - Beyond saliency-based XAI approaches. Eur J Radiol 2023;162:110786. [Crossref] [PubMed]
- Bi WL, Hosny A, Schabath MB, Giger ML, Birkbak NJ, Mehrtash A, Allison T, Arnaout O, Abbosh C, Dunn IF, Mak RH, Tamimi RM, Tempany CM, Swanton C, Hoffmann U, Schwartz LH, Gillies RJ, Huang RY, Aerts HJWL. Artificial intelligence in cancer imaging: Clinical challenges and applications. CA Cancer J Clin 2019;69:127-57. [Crossref] [PubMed]
- Rahman A, Debnath T, Kundu D, Khan MSI, Aishi AA, Sazzad S, Sayduzzaman M, Band SS. Machine learning and deep learning-based approach in smart healthcare: Recent advances, applications, challenges and opportunities. AIMS Public Health 2024;11:58-109. [Crossref] [PubMed]
- Umamaheswari T, Babu YMM. ViT-MAENB7: An innovative breast cancer diagnosis model from 3D mammograms using advanced segmentation and classification process. Comput Methods Programs Biomed 2024;257:108373. [Crossref] [PubMed]
- Roig-Marín N. Chapter 21 - Ground-glass nodules in the lungs of COVID-19 patients. In: Rajendram R, Preedy VR, Patel VB, Martin CR, editors. Management, Body Systems, and Case Studies in COVID-19. Academic Press; 2024:237-44.
- Roig-Marín N, Roig-Rico P. Ground-glass opacity on emergency department chest X-ray: a risk factor for in-hospital mortality and organ failure in elderly admitted for COVID-19. Postgrad Med 2023;135:265-72. [Crossref] [PubMed]
- Roberts M, Driggs D, Thorpe M, Gilbey J, Yeung M, Ursprung S, Aviles-Rivero AI, Etmann C, McCague C, Beer L, Weir-McCall JR, Teng Z, Gkrania-Klotsas E, Rudd JHF, Sala E, Schönlieb CB. Common pitfalls and recommendations for using machine learning to detect and prognosticate for COVID-19 using chest radiographs and CT scans. Nature Machine Intelligence 2021;3:199-217.