Assessing pulmonary vascular remodeling in connective tissue disease-associated interstitial lung disease: a CT-based quantitative analysis
Introduction
Connective tissue diseases (CTDs) are a series of heterogeneous diseases with immune-mediated multiorgan dysfunction, mainly including rheumatoid arthritis (RA), systemic lupus erythematosus (SLE), systemic sclerosis (SSc) and Sjögren’s syndrome (SS) (1-3). The common pathophysiology is typified by autoimmune abnormalities that cause damage to connective tissues and blood vessels. The lungs, with their high collagen protein content and vascular structure, are one of the most vulnerable organs to invasion. Interstitial lung disease (ILD) is a common pulmonary manifestation in patients with CTD and significantly affects patients’ prognosis (4,5), and it has been reported that CTD-ILD accounts for 31.76% of the mortality rate of CTD (6). ILD affects the alveoli, basement membranes, and vasculature, and the damage to endothelial cells and basement membranes is accompanied by inflammatory cells and proinflammatory cytokine infiltration, which triggers pulmonary vascular remodeling (endothelial dysfunction, endothelial fibrosis, and mild medial proliferation) (7,8), decreased pulmonary capillary permeability, and reduced capillary beds, leading to impaired pulmonary function (decreased diffusion function) and the development of pulmonary hypertension (PH) (9).
Previous studies have shown that computed tomography (CT)-based pulmonary vascular parameters, such as pulmonary vessel volume (PVV), vessel-related structures (VRS), and the ratio of the main pulmonary artery to the adjacent ascending aorta (rPA), can predict the prognosis of patients with idiopathic pulmonary fibrosis (IPF) and CTD-ILD (10-13). Quantitative CT-derived vessel metrics offer a noninvasive approach for the evaluation of pulmonary vasculature.
The speedy development of artificial intelligence technology has made it feasible to automatically segment and quantitatively analyze pulmonary veins and arteries in three dimensions using CT scans (14-19). These novel approaches offer more precise ways to investigate pathological, morphological, and functional alterations in the blood vessels in the pulmonary. Our previous study using the same technique examined the characteristics of pulmonary vascular remodeling in a population with chronic obstructive pulmonary disease (COPD). That study revealed that quantitative CT analysis of the pulmonary vasculature was found to be a potential imaging marker for COPD severity evaluation and early diagnosis (20). Further investigation is needed to determine the prognostic significance of pulmonary vascular remodeling in CTD-ILD patients, especially regarding arteriovenous separation, due to the limited research in this area. In this study, pulmonary arteries and pulmonary veins were automatically separated and quantitatively analyzed based on artificial intelligence technology to investigate the imaging characteristics and dynamic patterns of pulmonary vascular remodeling in CTD-ILD patients. We present this article in accordance with the STROBE reporting checklist (available at https://qims.amegroups.com/article/view/10.21037/qims-24-1770/rc).
Methods
This retrospective study was approved by the institutional review board of Shanxi Bethune Hospital (IRB No. YXLL-2022-094), and individual consent for this retrospective analysis was waived. The study was conducted in accordance with the Declaration of Helsinki (as revised in 2013).
Subjects
Subjects from Shanxi Bethune Hospital were collected from January 2018 to July 2022. The inclusion criteria were as follows: (I) patients diagnosed with CTD-ILD in our hospital and (II) patients who have undergone a nonenhanced chest CT scan and complete pulmonary function testing (PFT), with a maximum of one month separating the two tests. The exclusion criteria were as follows: (I) failure to cooperate with PFT; (II) incomplete clinical information or unsatisfactory CT image quality due to severe artifacts; (III) CTD-ILD combined with pulmonary emphysema, infection, tumor, heart disease, drug toxicity, allergy, and other conditions affecting the pulmonary vasculature; and (IV) previous history of thoracic surgery, pleural effusion or thoracic deformity. An overview of the case selection process is shown in Figure 1.
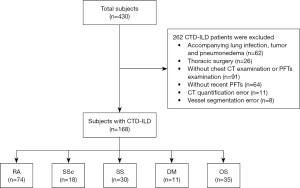
Clinical and pulmonary function parameters
The digital medical record system for patients with CTD-ILD provided the clinical data, which included smoking history, treatment records, and demographic data. Routine lung ventilation and diffusion function tests were conducted using the Master Screen Diffusion pulmonary function instrument (Eric Jaeger, Germany). The following indicators were measured: forced vital capacity (FVC), forced expiratory volume in 1.0 s (FEV1), diffusing capacity for carbon monoxide (DLCO), alveolar ventilation (VA), and total lung capacity (TLC). The results of the pulmonary function tests were expressed as a percentage of predicted performance. The operation was completed, and the results were evaluated based on the standards established by the American Thoracic Society/European Respiratory Society (21). All enrolled patients were staged using the Gender-Age-Physiology (GAP) system (22).
Image acquisition
All recruited patients received respiratory training before the scan. At the end of deep inspiration, the patient was positioned supine for the chest CT scan from the lung apex to base. Every CT scan was reconstructed using “B70f” lung convolution kernels and a 1-mm slice thickness. Scans were acquired on the one of two multidetector CT systems (SOMATOM Definition Flash and SOMATOM Definition AS, Siemens Medical Solutions, Forchheim, Germany). The relevant parameters are shown in Table 1.
Table 1
Model | Tube voltage (kVp) | Detector collimation (mm) | Care dose | Ref tube current-time product (mAs) | Rotation time (s) | Matrix size | Pitch |
---|---|---|---|---|---|---|---|
SOMATOM Definition Flash | 120 | 0.6×128 | Open | 150 | 0.5 | 512×512 | 1 |
SOMATOM Definition AS | 120 | 0.6×64 | Open | 150 | 0.5 | 512×512 | 1 |
CT, computed tomography.
Image processing and quantification of pulmonary vascular parameters
The CT images in Medicine (DICOM) format were transferred to the FACT digital lung workstation (Shaanxi Weinan Shenzhou Dexin Medical Imaging Technology Co., Ltd., China) in DICOM format. Pulmonary arterial and venous trees reconstructions were generated using a deep learning-based automated algorithm, and the volume and tortuosity of vessels of varying cross-sectional area,as well as total lung volume (TLV) were calculated (23). The FACT workstation integrates convolutional neural network (CNN) and computational differential geometry techniques to automatically identify and differentiate pulmonary arteries and veins on chest CT without iodine contrast agents. A CNN-based approach was used to identify and differentiate central extrapulmonary arterioles and veins, while a computational differential geometry approach was used to segment the intrapulmonary vasculature without distinguishing between arterioles and veins. A skeleton of pulmonary vasculature was extracted from its 3D representation for the arteries and veins, respectively. Additionally, the points along the centerlines were arranged into a binary tree structure, with each branching point in the vessel structure dividing the parent node into two child nodes. Parameters like the volume and tortuosity of artery/vein branches, etc. were computed from the skeleton. The percentage of interstitial lung abnormalities (ILA) was calculated with high attenuation areas (%HAA: the percentage of voxels of −600 to −250 Hounsfield units) (24).
The relevant parameters and calculation formulas are as follows:
where BV5 denotes volume of pulmonary blood vessels with the cross-sectional area less than 5 mm2, BV5a (v) denotes volume of pulmonary arteries or veins with a CSA less than 5 mm2, BV5+ denotes volume of the pulmonary blood vessels with a CSA greater than 5 mm2, PAV denotes the pulmonary arterial volume and PVV denotes pulmonary venous volume.
Statistical analysis
Normality of all variables was tested using the Shapiro-Wilk test and histograms. Continuous variables were expressed as means ± standards for parametric data and medians with interquartile ranges (IQRs) for nonparametric data. Levene’s test was used to test the homogeneity of variance. To assess the association between the parameters and groups of CTD-ILD patients, one-way analysis of variance (ANOVA) and nonparametric test (Kruskal-Wallis test) were used according to the results of normal distribution and homoscedasticity test results. Pairwise comparisons were performed using Bonferroni corrections post hoc tests. Categorical data were presented as numbers of patients and percentages, and the Chi-squared test and Fisher’s exact test were used for categorical variables. Spearman’s correlation coefficient (γ) was used to correlate pulmonary vascular density with PFT. For all statistical analyses, P<0.05 was considered statistically significant. All statistical analyses were performed using SPSS software version 23.0 (SPSS Inc., Chicago, IL, USA).
Results
Study participant characteristics
We enrolled 168 CTD-ILD patients ultimately with 180 sets of CT images for the analysis, of whom 12 represented follow-up evaluations. We also enrolled 74 patients with RA (44.0%), 18 patients with SSc (10.7%), 30 patients with SS (17.9%), 11 patients with dermatomyositis (DM) (6.5%), 35 patients with overlap syndrome (OS) (20.8%). Among these patients, 106, 50, and 12 were allocated to GAP 1, 2, and 3, respectively, and the pulmonary arterial and pulmonary venous vascular trees generation is shown in Figure 2. The baseline characteristics and PFT results are summarized in Table 2. The differences in age, gender, %HAA, and PFTs between the groups were statistically significant (all P<0.05), but no statistically significant difference was observed in smoking history or TLV (P>0.05).
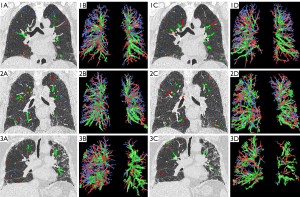
Table 2
Characteristic | GAP | P | ||
---|---|---|---|---|
1 (n=106) | 2 (n=50) | 3 (n=12) | ||
Age, years, mean [SD] | 59 [10] | 67 [8] | 72 [6] | <0.001 |
Gender, n (%) | <0.001 | |||
Female | 73 (43.5) | 11 (6.5) | 4 (2.4) | |
Male | 33 (19.6) | 39 (23.2) | 8 (4.8) | |
Smoking history, n (%) | 20 (11.9) | 13 (7.7) | 3 (1.8) | 0.625 |
BMI, kg/m2, mean [SD] | 24.61 [3.80] | 26.21 [19.48] | 22.41 [2.88] | 0.503 |
TLV, L, mean [SD] | 3.42 [1.08] | 3.57 [1.19] | 2.98 [0.61] | 0.234 |
%HAA, mean [SD] | 9.66 [4.19] | 11.69 [5.51] | 12.57 [3.43] | 0.01 |
Disease subset, n (%) | ||||
RA | 44 (26.2) | 23 (13.7) | 7 (4.2) | |
SSc | 15 (8.9) | 2 (1.2) | 1 (0.6) | |
SS | 14 (8.3) | 14 (8.3) | 2 (1.2) | |
DM | 9 (5.4) | 1 (0.6) | 1 (0.6) | |
OS | 24 (14.3) | 10 (6.0) | 1 (0.6) | |
PFTs | ||||
FVC%, mean [SD] | 86.91 [17.77] | 74.42 [18.67] | 55.68 [9.84] | <0.001 |
DLCO%, median (IQR) | 56.60 (23.90) | 36.70 (19.30) | 28.15 (12.65) | <0.001 |
FEV1%, mean [SD] | 86.18 [17.83] | 73.89 [19.62] | 60.92 [10.48] | <0.001 |
TLC%, mean [SD] | 79.27 [13.43] | 67.32 [12.44] | 59.13 [8.59] | <0.001 |
DLCO/VA%, median [IQR] | 72.7 [26] | 55.9 [28] | 34.15 [39] | <0.001 |
BMI, body mass index; DM, dermatomyositis; DLCO, diffusion capacity of carbon monoxide; FVC, forced vital capacity; FEV1, forced expiratory volume in 1 s; GAP, Gender-Age-Physiology; HAA, high attenuation areas; IQR, interquartile range; OS, overlap syndrome; PFT, pulmonary function testing; RA, rheumatoid arthritis; SD, standard deviation; SSc, systemic sclerosis; SS, Sjögren’s syndrome; TLV, total lung volume; TLC, total lung capacity; VA, alveolar ventilation.
Quantitative analysis of pulmonary blood vessels
Significant intergroup differences were observed in PAV, PVV, BV5a/v density, BV5a/v fraction, and pulmonary vascular tortuosity for different cross-sectional areas (all P<0.05) (Table 3). In the GAP stage 2, PAV, PVV, BV5+a, and BV5+v were higher than those in the GAP stage 1. However, the aforementioned indexes were smaller in the GAP stage 3 than in the GAP stages 1 and 2 (Table 3 and Figures 3,4). Additionally, the pulmonary arterial and venous tortuosity displayed an increase with more advanced CTD-ILD (Table 3 and Figure 5). Conversely, patients with a higher GAP stage demonstrated smaller values for measured arterial and venous BV5 volume, density, and fraction (Table 3 and Figures 3,4).
Table 3
Variable | GAP | P | ||
---|---|---|---|---|
1 (n=106) | 2 (n=50) | 3 (n=12) | ||
Arteries | ||||
PAV, mL, mean (SD) | 98.35 (28.73)† | 99.71 (29.56)† | 75.46 (16.74) | 0.024 |
BV5a, mL, median (IQR) | 24.67 (11.16)† | 22.84 (18.62)† | 14.07 (3.92) | <0.001 |
BV5+a, mL, median (IQR) | 67.45 (34.47) | 79.27 (35.19)† | 57.11 (20.52) | 0.047 |
BV5a density, mean (SD) | 7.56 (2.20)*† | 6.34 (2.32) | 4.96 (1.28) | <0.001 |
BV5a fraction, %, mean (SD) | 25.9 (6.7)*† | 22.3 (7.5) | 19.3 (4.0) | <0.001 |
Tortuosity-PA, median (IQR) | 1.155 (0.069) | 1.175 (0.056) | 1.225 (0.115) | 0.041 |
Veins | ||||
PVV, mL, mean (SD) | 91.78 (27.51)† | 92.07 (27.65)† | 62.20 (16.70) | 0.002 |
BV5v, mL, mean (SD) | 20.88 (7.99)† | 18.64 (10.19)† | 9.38 (4.17) | <0.001 |
BV5+v, mL, median (IQR) | 68.61 (35.14)† | 74.71 (26.67)† | 49.47 (19.60) | 0.012 |
BV5v density, mean (SD) | 6.27 (2.02)*† | 5.07 (1.80)† | 3.22 (1.27) | <0.001 |
BV5v fraction, %, mean (SD) | 22.9 (6.3)*† | 19.5 (7.1) | 14.7 (3.3) | <0.001 |
Tortuosity-PV, median (IQR) | 1.160 (0.075)*† | 1.171 (0.066) | 1.198 (0.095) | 0.003 |
†, compared with GAP3, the difference was significant (P<0.05). *, compared with GAP2, the difference was significant (P<0.05). BV5a/v, volume of pulmonary arteries/veins with a CSA less than 5 mm2; BV5+a/v,volume of pulmonary arteries/veins with a CSA greater than 5 mm2; CTD-ILD, connective tissue disease-associated interstitial lung disease; GAP, Gender-Age-Physiology; IQR, interquartile range; PAV, pulmonary arterial volume; PA, pulmonary artery; PVV, pulmonary venous volume; PV, pulmonary vein; SD, standard deviation.
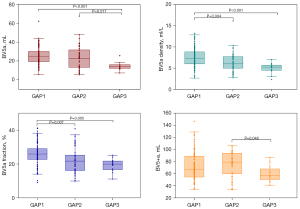
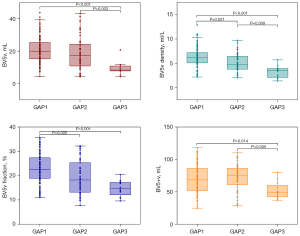
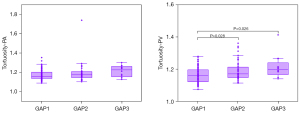
Longitudinal evaluation
Twelve patients had a second PFT examination and CT scan suitable for artery and vein separation, as presented in Table 4. After a mean follow-up of 20 months, GAP staging numerically increased in 4/12 cases (33.3%), decreased in 1/12 cases (8.3%) and remained stable in 7/12 cases (58.3%), of which 5 were GAP grade 1, 1 was GAP grade 2, and 1 was GAP grade 3. The average decrease in BV5a (BV5a density; BV5a fraction) was 5.83 mL (0.60, 1.65), and BV5v (BV5v density; BV5v fraction) was 5.92 mL (1.0, 3.73) in 4 patients with progressive GAP stage. Seven patients with stable GAP stage had an average decrease of 0.14 mL (0.06, 0.37) in BV5a (BV5a density; BV5a fraction) and 1.45 mL (0.49, 1.14) in BV5v (BV5v density; BV5v fraction). The changes in the arterial and venous volumes showed essentially the same trend as the PFT results (FVC%, Dlco%).
Table 4
Variable | Patient No. | |||||||||||
---|---|---|---|---|---|---|---|---|---|---|---|---|
1 | 2 | 3 | 4 | 5 | 6 | 7 | 8 | 9 | 10 | 11 | 12 | |
Age (years) | 72 | 78 | 66 | 56 | 61 | 67 | 66 | 64 | 62 | 60 | 54 | 52 |
Sex | F | M | F | F | M | F | M | M | M | M | F | F |
Disease subset | OS | SS | RA | SS | OS | OS | RA | OS | SSc | RA | SSc | RA |
Follow-up time (months) | 18 | 7 | 27 | 36 | 3 | 16 | 43 | 3 | 19 | 18 | 11 | 33 |
FVC% | ||||||||||||
BL | 73.5 | 58.3 | 70.9 | 62.7 | 81.3 | 89.3 | 81.6 | 85 | 83.7 | 117 | 90.2 | 62 |
FUP | 85 | 51.2 | 44.5 | 42.8 | 74.7 | 86.9 | 58.3 | 79.9 | 73.9 | 114.9 | 99.1 | 62.1 |
Difference | +11.5 | −7.1 | −26.4 | −19.9 | −6.6 | −2.4 | −23.3 | −5.1 | −9.8 | −2.1 | +8.9 | +0.1 |
DLCO% | ||||||||||||
BL | 32 | 26.1 | 33.6 | 42.5 | 40.9 | 49.3 | 75.5 | 53 | 56.2 | 62.8 | 35.7 | 32 |
FUP | 36 | 18.9 | 27.6 | 39.6 | 30.6 | 51.9 | 59.2 | 39.7 | 55.1 | 58.8 | 42 | 36 |
Difference | +4 | −7.2 | −6 | −2.9 | −10.3 | +2.6 | −16.3 | −13.3 | −1.1 | −4 | +6.3 | +4 |
GAP | 3→2 | 3→3 | 2→3 | 1→2 | 1→2 | 2→2 | 1→2 | 1→1 | 1→1 | 1→1 | 1→1 | 1→1 |
Trend | ↓ | – | ↑ | ↑ | ↑ | – | ↑ | – | – | – | – | – |
BV5a (mL) | ||||||||||||
BL | 7.21 | 12.49 | 13.77 | 13.26 | 21.96 | 14.03 | 25.13 | 26.02 | 26.71 | 44.03 | 28.39 | 20.57 |
FUP | 10.77 | 15.60 | 14.13 | 7.71 | 15.71 | 15.78 | 13.25 | 19.25 | 29.89 | 38.23 | 33.86 | 18.67 |
Difference | +3.56 | +3.11 | +0.36 | −5.55 | −6.25 | +1.75 | −11.88 | −6.77 | +3.18 | −5.8 | +5.47 | −1.9 |
BV5a density | ||||||||||||
BL | 2.34 | 4.37 | 5.10 | 7.42 | 5.33 | 3.21 | 8.57 | 6.02 | 8.29 | 10.85 | 5.94 | 7.79 |
FUP | 3.26 | 5.33 | 5.89 | 5.37 | 4.81 | 6.46 | 7.94 | 4.49 | 8.48 | 5.88 | 7.83 | 7.34 |
Difference | +0.92 | +0.96 | +0.79 | −2.05 | −0.52 | +3.52 | −0.63 | −1.53 | +0.19 | −4.97 | +1.89 | −0.45 |
BV5a | ||||||||||||
BL | 11.1 | 11.9 | 20.9 | 19.6 | 19.5 | 12.8 | 23.2 | 18.5 | 22.7 | 35.9 | 25.0 | 36.0 |
FUP | 14.1 | 14.8 | 24.9 | 15.1 | 14.2 | 22.5 | 22.4 | 14.4 | 27.1 | 29.7 | 23.2 | 33.7 |
Difference | +3.0 | +2.9 | +4.0 | −4.5 | −5.3 | +9.7 | −0.8 | −4.1 | +4.4 | −6.2 | −1.8 | −2.3 |
BV5v (mL) | ||||||||||||
BL | 4.19 | 10.78 | 14.11 | 15.50 | 17.73 | 18.95 | 18.75 | 15.29 | 23.09 | 62.8 | 21.88 | 16.15 |
FUP | 7.14 | 7.22 | 10.77 | 6.18 | 14.81 | 21.52 | 10.65 | 14.61 | 21.57 | 58.9 | 22.99 | 11.99 |
Difference | +2.95 | −3.56 | −3.34 | −9.32 | −2.92 | +2.57 | −8.1 | −0.68 | −1.52 | −3.9 | +1.11 | −4.16 |
BV5v density | ||||||||||||
BL | 1.36 | 3.77 | 5.22 | 6.99 | 4.53 | 2.97 | 6.32 | 3.54 | 7.17 | 9.68 | 5.00 | 6.12 |
FUP | 2.16 | 1.78 | 4.49 | 4.30 | 4.30 | 5.98 | 5.95 | 3.41 | 6.12 | 6.75 | 6.03 | 4.71 |
Difference | +0.8 | −1.99 | −0.73 | −2.69 | −0.23 | +3.01 | −0.37 | −0.13 | −1.05 | −2.93 | +1.03 | −1.41 |
BV5v fraction% | ||||||||||||
BL | 9.6 | 13.2 | 21.8 | 16.7 | 15.6 | 14.8 | 20.6 | 12.8 | 22.5 | 32.5 | 18.6 | 33.8 |
FUP | 13.5 | 11.0 | 17.4 | 11.2 | 13.0 | 18.9 | 18.2 | 11.9 | 21.9 | 27.4 | 18.9 | 30.2 |
Difference | +3.9 | −2.2 | −4.4 | −5.5 | −2.6 | +4.1 | −2.4 | −0.9 | −0.6 | −5.1 | +0.3 | −3.6 |
–, compared with GAP staging in the baseline group, there was no change in the follow-up group. ↑, compared with GAP staging in the baseline group, the follow-up group showed progression. ↓, compared with GAP staging in the baseline group, the follow-up group showed remission. BL, baseline; BV5a/v, volume of pulmonary arteries/veins with a cross-sectional area less than 5 mm2; DLCO, diffusion capacity of carbon monoxide; F, female; FVC, forced vital capacity; FUP, follow-up; GAP, Gender-Age-Physiology; M, male; OS, overall survival; RA, rheumatoid arthritis; SS, Sjögren’s syndrome; SSc, systemic sclerosis.
Correlations of arterial/venous BV5 parameters with PFT results
The volume, density and fraction of BV5a were positively correlated with PFT results (FVC%, DLCO%, FEV1%, TLC%) (γ=0.179–0.512, P<0.05) as shown in Table 5. Among these parameters, the correlation between BV5a and TLC% was the most obvious (γ=0.512, P<0.001). Likewise, the volume, density, and fraction of BV5v were positively correlated with PFT results (γ=0.222–0.608, P<0.05), with the most obvious correlation observed between BV5v and TLC% (γ=0.608, P<0.001).
Table 5
Variable | FVC% | DLCO% | FEV1% | TLC% | DLCO/VA% | |||||||||
---|---|---|---|---|---|---|---|---|---|---|---|---|---|---|
γ | P | γ | P | γ | P | γ | P | γ | P | |||||
BV5a | 0.478 | <0.001 | 0.289 | <0.001 | 0.390 | <0.001 | 0.512 | <0.001 | 0.141 | 0.069 | ||||
BV5a density | 0.245 | 0.001 | 0.262 | 0.001 | 0.290 | 0.014 | 0.362 | <0.001 | 0.179 | 0.020 | ||||
BV5a fraction% | 0.372 | <0.001 | 0.298 | <0.001 | 0.278 | <0.001 | 0.503 | <0.001 | 0.135 | 0.082 | ||||
BV5v | 0.566 | <0.001 | 0.414 | <0.001 | 0.430 | <0.001 | 0.608 | <0.001 | 0.224 | 0.004 | ||||
BV5v density | 0.378 | <0.001 | 0.420 | <0.001 | 0.266 | <0.001 | 0.494 | <0.001 | 0.286 | <0.001 | ||||
BV5v fraction% | 0.405 | <0.001 | 0.380 | <0.001 | 0.255 | 0.001 | 0.554 | <0.001 | 0.222 | 0.004 |
γ values larger than 0 indicate positive connection, γ values less than 0 indicate negative correlation, and γ values equal to 0 indicate no correlation. γ values less than 0.3 indicate a mild correlation, γ values between 0.3 and 0.8 suggest a moderate correlation, and γ values greater than 0.8 indicate a strong connection. BV5a/v, volume of pulmonary arteries/veins with a cross-sectional area less than 5 mm2; DLCO, diffusion capacity of carbon monoxide; FVC, forced vital capacity; FEV1, forced expiratory volume in 1 s; PFT, pulmonary function testing; TLC, total lung capacity; VA, alveolar ventilation.
Discussion
Based on nonenhanced chest CT images, this study quantitatively analyzed the pulmonary vasculature of CTD-ILD patients with varying GAP stages. Our data show that BV5 parameters, other pulmonary vascular volumes (PAV, PVV, BV5+), and tortuosity could effectively reflect the severity of CTD-ILD. Pulmonary functional impairment is associated with remodeling of the small pulmonary vessels.
Remodeling and loss of the small pulmonary vessels are referred to as pulmonary vascular pruning (25). Histopathological evaluations of pulmonary vasculature with CTD-ILD show endothelial hyperplasia, fibromyxoid intimal thickening and mild medial proliferation (26), which lead to thickening, narrowing, occlusion, and loss of the small pulmonary vessels (27-29). In this study, BV5a volume showed lower values with more advanced CTD-ILD, and BV5a volume that was normalized by pulmonary volume (BV5a density), which eliminates individual pulmonary volume differences, also showed the same result. It has been suggested that BV5a fraction is an important parameter reflecting pulmonary arterial pruning (15,30). Synn et al. (14) confirmed that the severity of pulmonary vascular pruning is associated with the progression of ILA. Our findings suggest that BV5a fraction decreased gradually with increasing GAP stage in CTD-ILD patients, which reflects the degree of pulmonary vascular pruning. Moreover, the observed pattern of BV5v volume change in this study exhibited a notable congruence with BV5a. One potential explanation for this finding is that remodeling occurs in the small pulmonary vein itself, and the other is a decrease in the downstream pulmonary venous perfusion brought on by a decline in the small pulmonary artery volume and a reduction in the pulmonary vascular bed reserve as a result of fibrosis of the pulmonary mesenchyme (31). The follow-up results of four of the twelve patients in this study’s longitudinal observation revealed that the pulmonary small vessel volume decreased when increasing GAP stage. This decline was more obvious than that in the GAP stabilization group, further confirming the existence of the phenomenon of pulmonary vascular pruning with more advanced CTD-ILD. Consequently, We concluded that BV5, BV5 density, and BV5 fraction serve as important parameters in the assessment of pulmonary vascular remodeling in patients with CTD-ILD. However, it was determined that the BV5 density and BV5 fraction are more objective and accurate indicators than BV5. This is attributed to their ability to account for the influence of differences in pulmonary volume and total lung vascular volume among individuals.
In this study, we demonstrated that whereas BV5+a was significantly lower in GAP stage 3 than in GAP stages 1 and 2, it was higher in GAP stage 2 CTD-ILD patients than in GAP stage 1. The PAV alterations were in line with BV5+a. We think that is attributed to enlargement of the proximal arteries lumen caused by remodeling of the small distal pulmonary arteries, increased pulmonary vascular resistance and lower arterial compliance (31). Furthermore, PVV and BV5+v demonstrated a similar pattern of change as the arteries. It was hypothesized that this phenomenon might be caused by an increase in vascular pressure in fibrotic areas of the lungs in GAP stage 2 CTD-ILD patients and that this would lead to a compensatory increase in the distribution of blood flow to the nonfibrotic area (32).
Jacob et al. (32) concluded that higher pulmonary vascular volumes were associated with loss of pulmonary function, worse outcomes and higher mortality. However, Synn et al. (14) and Kanski et al. (33) demonstrated that reductions in pulmonary vascular volumes on CT were linked to the advancement of ILD. Our finding support Synn and Kanski’s conclusion that both PAVs and PVVs were significantly lower in CTD-ILD patients with GAP3 stage compared to those with GAP1 and GAP2. This is because the pulmonary vascular pruning in patients with CTD-ILD is caused by the pulmonary vasculature itself as well as numerous factors associated with ILD. In the early stage of ILD, spasm of the pulmonary vasculature caused by Raynaud’s phenomenon (RP) of CTD and pulmonary vasculitis are the dominant factors in pulmonary vascular remodeling (34,35), whereas in the late stage of ILD, extensive damage and destruction of lung tissue structure leads to a significant reduction in the pulmonary vascularity in the fibrotic regions (19).
Our data show a statistically significant difference in arterial and venous tortuosity between the CTD-ILD groups, indicating an increase in pulmonary vascular tortuosity with advanced CTD-ILD. This result contrasts with those of previous studies (14,18) that showed no statistically significant difference in pulmonary vascular tortuosity between ILD patients and controls. Moreover, Helmberger et al. (36) conducted a study involving 18 patients with PH and concluded that pulmonary vascular tortuosity serves as a noninvasive quantifier of PH. Additionally, it has been suggested that pulmonary vascular tortuosity may function as a biomarker for lesions in the pulmonary vasculature itself rather than the changes caused by pulmonary fibrosis involving the lung parenchyma. As both the pulmonary arteries and veins undergo vascular remodeling in patients with CTD-ILD, further research is necessary to investigate the underlying mechanism behind changes in pulmonary vascular tortuosity.
This investigation demonstrates a relationship between PFT abnormalities and pulmonary vascular remodeling, as evidenced by the positive correlations of varying degrees between BV5, BV5 density, and BV5 fraction and PFT. Notably, BV5a/v exhibited the strongest correlation with TLC%. ILD is primarily characterized by restrictive ventilation dysfunction and diffusion disorder, with TIC serving as a sensitive qualitative marker for diagnosing restrictive ventilation disorders. The current study marginally correlated BV5, BV5density, and BV5 fraction with DLCO. Decreased DLCO is linked to the progression of PH and increased mortality, and it is attributed to a reduced alveolar volume and/or thickening of alveolar capillary membranes. An isolated DLCO impairment has been attributed to lung vasculopathy but lacks specificity in ILD. Nevertheless, available evidence suggests that TLC and DLCO should not yet be discounted as potential surrogate markers for ILD progression (37,38).
There are limitations in this study. First, this was a single-center, retrospective study. The sample sizes for the GAP stages 1–3 groups are unbalanced, particularly with respect to the small sample size of the GAP stage 3 group. Therefore, further studies with large samples and balanced data are needed. Second, this study was conducted as a cross-sectional study, which only allowed for longitudinal change data to be collected from 12 patients. To address this limitation, future follow-up must be improved to increase the sample size of the longitudinal data. Last, a range of CTD cases were gathered for this study, therefore, when the sample size is increased, a more refined analysis of a specific CTD disease can be carried out.
Conclusions
Patients with CTD-ILD exhibiting pulmonary vascular remodelling, and BV5 density/fraction is an important indicator of pulmonary vascular remodelling and may represent a candidate biomarker of ILD progression. Future longitudinal multicentre studies on larger populations are warranted to elucidate the role of imaging biomarkers in predicting early CTD-ILD and its progression.
Acknowledgments
None.
Footnote
Reporting Checklist: The authors have completed the STROBE reporting checklist. Available at https://qims.amegroups.com/article/view/10.21037/qims-24-1770/rc
Funding: This work was supported by
Conflicts of Interest: All authors have completed the ICMJE uniform disclosure form (available at https://qims.amegroups.com/article/view/10.21037/qims-24-1770/coif). The authors have no conflicts of interest to declare.
Ethical Statement: The authors are accountable for all aspects of the work in ensuring that questions related to the accuracy or integrity of any part of the work are appropriately investigated and resolved. This retrospective study was approved by the institutional review board of Shanxi Bethune Hospital (IRB No. YXLL-2022-094), and individual consent for this retrospective analysis was waived. The study was conducted in accordance with the Declaration of Helsinki (as revised in 2013).
Open Access Statement: This is an Open Access article distributed in accordance with the Creative Commons Attribution-NonCommercial-NoDerivs 4.0 International License (CC BY-NC-ND 4.0), which permits the non-commercial replication and distribution of the article with the strict proviso that no changes or edits are made and the original work is properly cited (including links to both the formal publication through the relevant DOI and the license). See: https://creativecommons.org/licenses/by-nc-nd/4.0/.
References
- Ruaro B, Baratella E, Confalonieri P, Confalonieri M, Vassallo FG, Wade B, Geri P, Pozzan R, Caforio G, Marrocchio C, Cova MA, Salton F. High-Resolution Computed Tomography and Lung Ultrasound in Patients with Systemic Sclerosis: Which One to Choose? Diagnostics (Basel) 2021;11:2293. [Crossref] [PubMed]
- Jaeger VK, Tikly M, Xu D, Siegert E, Hachulla E, Airò P, et al. Racial differences in systemic sclerosis disease presentation: a European Scleroderma Trials and Research group study. Rheumatology (Oxford) 2020;59:1684-94. [Crossref] [PubMed]
- Jeganathan N, Sathananthan M. Connective Tissue Disease-Related Interstitial Lung Disease: Prevalence, Patterns, Predictors, Prognosis, and Treatment. Lung 2020;198:735-59. [Crossref] [PubMed]
- Kondoh Y, Makino S, Ogura T, Suda T, Tomioka H, Amano H, et al. 2020 guide for the diagnosis and treatment of interstitial lung disease associated with connective tissue disease. Respir Investig 2021;59:709-40. [Crossref] [PubMed]
- Oliveira RP, Ribeiro R, Melo L, Grima B, Oliveira S, Alves JD. Connective tissue disease-associated interstitial lung disease. Pulmonology 2022;28:113-8. [Crossref] [PubMed]
- Li L, Zuo X, Luo H, Li Y, You Y, Duan L, Zhang W, Zhao H, Li T, Ning W, Xie Y, Liu S, Xie X, Jiang Y, Wu S, Zhu H, Zhou Y. Mortality trend of inpatients with connective tissue diseases: 2005-2014. Zhong Nan Da Xue Xue Bao Yi Xue Ban 2017;42:927-33. [Crossref] [PubMed]
- Wells AU, Denton CP. Interstitial lung disease in connective tissue disease--mechanisms and management. Nat Rev Rheumatol 2014;10:728-39. [Crossref] [PubMed]
- Colombat M, Mal H, Groussard O, Capron F, Thabut G, Jebrak G, Brugière O, Dauriat G, Castier Y, Lesèche G, Fournier M. Pulmonary vascular lesions in end-stage idiopathic pulmonary fibrosis: Histopathologic study on lung explant specimens and correlations with pulmonary hemodynamics. Hum Pathol 2007;38:60-5. [Crossref] [PubMed]
- Nathan SD, Barbera JA, Gaine SP, Harari S, Martinez FJ, Olschewski H, Olsson KM, Peacock AJ, Pepke-Zaba J, Provencher S, Weissmann N, Seeger W. Pulmonary hypertension in chronic lung disease and hypoxia. Eur Respir J 2019;53:1801914. [Crossref] [PubMed]
- Price LC, Devaraj A, Wort SJ. Central pulmonary arteries in idiopathic pulmonary fibrosis: size really matters. Eur Respir J 2016;47:1318-20. [Crossref] [PubMed]
- Wu X, Kim GH, Salisbury ML, Barber D, Bartholmai BJ, Brown KK, et al. Computed Tomographic Biomarkers in Idiopathic Pulmonary Fibrosis. The Future of Quantitative Analysis. Am J Respir Crit Care Med 2019;199:12-21. [Crossref] [PubMed]
- Jacob J, Bartholmai BJ, Rajagopalan S, Kokosi M, Egashira R, Brun AL, Nair A, Walsh SLF, Karwoski R, Wells AU. Serial automated quantitative CT analysis in idiopathic pulmonary fibrosis: functional correlations and comparison with changes in visual CT scores. Eur Radiol 2018;28:1318-27. [Crossref] [PubMed]
- Jacob J, Hirani N, van Moorsel CHM, Rajagopalan S, Murchison JT, van Es HW, et al. Predicting outcomes in rheumatoid arthritis related interstitial lung disease. Eur Respir J 2019;53:1800869. [Crossref] [PubMed]
- Synn AJ, Li W, Hunninghake GM, Washko GR, San José Estépar R, O'Connor GT, Kholdani CA, Hallowell RW, Bankier AA, Mittleman MA, Rice MB. Vascular Pruning on CT and Interstitial Lung Abnormalities in the Framingham Heart Study. Chest 2021;159:663-72. [Crossref] [PubMed]
- Pistenmaa CL, Nardelli P, Ash SY, Come CE, Diaz AA, Rahaghi FN, Barr RG, Young KA, Kinney GL, Simmons JP, Wade RC, Wells JM, Hokanson JE, Washko GR, San José Estépar R. COPDGene Investigators. Pulmonary Arterial Pruning and Longitudinal Change in Percent Emphysema and Lung Function: The Genetic Epidemiology of COPD Study. Chest 2021;160:470-80. [Crossref] [PubMed]
- Rahaghi FN, Argemí G, Nardelli P, Domínguez-Fandos D, Arguis P, Peinado VI, Ross JC, Ash SY, de La Bruere I, Come CE, Diaz AA, Sánchez M, Washko GR, Barberà JA, San José Estépar R. Pulmonary vascular density: comparison of findings on computed tomography imaging with histology. Eur Respir J 2019;54:1900370. [Crossref] [PubMed]
- Nardelli P, Jimenez-Carretero D, Bermejo-Pelaez D, Washko GR, Rahaghi FN, Ledesma-Carbayo MJ, San Jose Estepar R. Pulmonary Artery-Vein Classification in CT Images Using Deep Learning. IEEE Trans Med Imaging 2018;37:2428-40. [Crossref] [PubMed]
- Bruni C, Occhipinti M, Pienn M, Camiciottoli G, Bartolucci M, Bosello SL, et al. Lung vascular changes as biomarkers of severity in systemic sclerosis-associated interstitial lung disease. Rheumatology (Oxford) 2023;62:696-706. [Crossref] [PubMed]
- Jacob J, Pienn M, Payer C, Urschler M, Kokosi M, Devaraj A, Wells AU, Olschewski H. Quantitative CT-derived vessel metrics in idiopathic pulmonary fibrosis: A structure-function study. Respirology 2019;24:445-52. [Crossref] [PubMed]
- Li R, Song M, Wang R, Su N. E L. Can CT-Based Arterial and Venous Morphological Markers of Chronic Obstructive Pulmonary Disease Explain Pulmonary Vascular Remodeling? Acad Radiol 2024;31:22-34. [Crossref] [PubMed]
- Raghu G, Remy-Jardin M, Myers JL, Richeldi L, Ryerson CJ, Lederer DJ, et al. Diagnosis of Idiopathic Pulmonary Fibrosis. An Official ATS/ERS/JRS/ALAT Clinical Practice Guideline. Am J Respir Crit Care Med 2018;198:e44-68. [Crossref] [PubMed]
- Ley B, Ryerson CJ, Vittinghoff E, Ryu JH, Tomassetti S, Lee JS, Poletti V, Buccioli M, Elicker BM, Jones KD, King TE Jr, Collard HR. A multidimensional index and staging system for idiopathic pulmonary fibrosis. Ann Intern Med 2012;156:684-91. [Crossref] [PubMed]
- Pu J, Leader JK, Sechrist J, Beeche CA, Singh JP, Ocak IK, Risbano MG. Automated identification of pulmonary arteries and veins depicted in non-contrast chest CT scans. Med Image Anal 2022;77:102367. [Crossref] [PubMed]
- Alevizos MK, Danoff SK, Pappas DA, Lederer DJ, Johnson C, Hoffman EA, Bernstein EJ, Bathon JM, Giles JT. Assessing predictors of rheumatoid arthritis-associated interstitial lung disease using quantitative lung densitometry. Rheumatology (Oxford) 2022;61:2792-804. [Crossref] [PubMed]
- Synn AJ, Li W, San José Estépar R, Washko GR, O'Connor GT, Tsao CW, Mittleman MA, Rice MB. Pulmonary Vascular Pruning on Computed Tomography and Risk of Death in the Framingham Heart Study. Am J Respir Crit Care Med 2021;203:251-4. [Crossref] [PubMed]
- Kwon KY. Pathological interpretation of connective tissue disease-associated lung diseases. Yeungnam Univ J Med 2019;36:8-15. [Crossref] [PubMed]
- Farkas L, Gauldie J, Voelkel NF, Kolb M. Pulmonary hypertension and idiopathic pulmonary fibrosis: a tale of angiogenesis, apoptosis, and growth factors. Am J Respir Cell Mol Biol 2011;45:1-15. [Crossref] [PubMed]
- Ruggiero RM, Bartolome S, Torres F. Pulmonary hypertension in parenchymal lung disease. Heart Fail Clin 2012;8:461-74. [Crossref] [PubMed]
- Jiao L, Shen R, Li M, Liang Y, Guo Y, Shen C. Determination of pulmonary vessel alteration in Chinese male smokers by quantitative computed tomography measurements: a retrospective study. Quant Imaging Med Surg 2024;14:3289-301. [Crossref] [PubMed]
- Synn AJ, Margerie-Mellon C, Jeong SY, Rahaghi FN, Jhun I, Washko GR, Estépar RSJ, Bankier AA, Mittleman MA, VanderLaan PA, Rice MB. Vascular remodeling of the small pulmonary arteries and measures of vascular pruning on computed tomography. Pulm Circ 2021;11:20458940211061284. [Crossref] [PubMed]
- Rahaghi FN, Nardelli P, Harder E, Singh I, Sánchez-Ferrero GV, Ross JC, San José Estépar R, Ash SY, Hunsaker AR, Maron BA, Leopold JA, Waxman AB, San José Estépar R, Washko GR. Quantification of Arterial and Venous Morphologic Markers in Pulmonary Arterial Hypertension Using CT Imaging. Chest 2021;160:2220-31. [Crossref] [PubMed]
- Jacob J, Bartholmai BJ, Rajagopalan S, Brun AL, Egashira R, Karwoski R, Kokosi M, Wells AU, Hansell DM. Evaluation of computer-based computer tomography stratification against outcome models in connective tissue disease-related interstitial lung disease: a patient outcome study. BMC Med 2016;14:190. [Crossref] [PubMed]
- Kanski M, Arheden H, Wuttge DM, Bozovic G, Hesselstrand R, Ugander M. Pulmonary blood volume indexed to lung volume is reduced in newly diagnosed systemic sclerosis compared to normals--a prospective clinical cardiovascular magnetic resonance study addressing pulmonary vascular changes. J Cardiovasc Magn Reson 2013;15:86. [Crossref] [PubMed]
- Castellani JW, O'Brien C, Stulz DA, Blanchard LA, DeGroot DW, Bovill ME, Francis TJ, Young AJ. Physiological responses to cold exposure in men: a disabled submarine study. Undersea Hyperb Med 2002;29:189-203.
- Overbeek MJ, Boonstra A, Voskuyl AE, Vonk MC, Vonk-Noordegraaf A, van Berkel MP, Mooi WJ, Dijkmans BA, Hondema LS, Smit EF, Grünberg K. Platelet-derived growth factor receptor-β and epidermal growth factor receptor in pulmonary vasculature of systemic sclerosis-associated pulmonary arterial hypertension versus idiopathic pulmonary arterial hypertension and pulmonary veno-occlusive disease: a case-control study. Arthritis Res Ther 2011;13:R61. [Crossref] [PubMed]
- Helmberger M, Pienn M, Urschler M, Kullnig P, Stollberger R, Kovacs G, Olschewski A, Olschewski H, Bálint Z. Quantification of tortuosity and fractal dimension of the lung vessels in pulmonary hypertension patients. PLoS One 2014;9:e87515. [Crossref] [PubMed]
- Rajagopal S. Visualizing Pulmonary Vascular Disease With CT Scanning. Chest 2021;160:1998-9. [Crossref] [PubMed]
- Ruaro B, Confalonieri M, Salton F, Wade B, Baratella E, Geri P, Confalonieri P, Kodric M, Biolo M, Bruni C. The Relationship between Pulmonary Damage and Peripheral Vascular Manifestations in Systemic Sclerosis Patients. Pharmaceuticals (Basel) 2021;14:403. [Crossref] [PubMed]