A preliminary study of steady-state visually-evoked potential-based non-invasive brain-computer interface technology as a communication aid for patients with amyotrophic lateral sclerosis
Introduction
Motor neuron disease (MND) is a neurological degenerative disease with progressive deterioration of upper and lower motor neurons (1). Currently, the exact cause of the disease is unknown, and no effective treatment has been established. Amyotrophic lateral sclerosis (ALS) is the most common type of MND (2). Some people with ALS develop dementia, but many remain conscious. The disease impairs movement, speech, eating, and breathing, can lead to a locked-in feeling and a lower quality of life, and cause stress to caregivers (3). Therefore, maintaining the ability of ALS patients to communicate with the others could improve clinical decisions and patients’ quality of life (4).
Brain-computer interfaces (BCIs) (5,6) enable direct communication between the brain and electronic devices, bypassing traditional neural and muscular pathways, which in turn enables the user to control devices based on their intentions (7). BCI technology can restore the communication and control functions of patients with neuromuscular disabilities (8). In BCIs, the patterns or components of electroencephalogram (EEG) signals obtained by different experimental tasks, which serve as the conveyors of the user’s will, are varied, and the will of the user can be correspondingly encoded according to the characteristics of these EEG signals (9). Currently, the brain signal components used as input in BCI systems mainly include: P300, slow cortical potentials, sensorimotor rhythms, motion-onset visual evolved potentials, and steady-state visual evoked potentials (SSVEPs) (10). SSVEPs have a high signal-to-noise ratio (SNR) and a low response time (11). The SNR of SSVEPs is an objective index that indicates the strength of the signal response of different patients (11), and is widely used in BCIs.
To date, treatments have failed to restore motor function; however, using their brain activity, ALS patients, including those in a “locked-in state”, can use BCIs to control devices. Hosni et al. used near-infrared spectroscopy-based BCIs for motor imagery (12). Guy et al. showed that P300-based BCI technology can help patients with spelling communication (13). SSVEP-based BCI systems offer non-calibration use, and have a high information transmission rate (ITR), and thus are more user friendly and efficient than other BCI systems
This study sought to explore whether SSVEP-based non-invasive BCI technology could aid the communication of patients with ALS (14). It also sought to examine the effect of SSVEP-based BCI technology on the communication of ALS patients and identify the possible influencing factors. The findings not only advance our understanding of BCI applications in ALS but also pave the way for the development of more effective communication tools that could enhance the quality of life of patients with severe motor impairments. We present this article in accordance with the STROBE reporting checklist (available at https://qims.amegroups.com/article/view/10.21037/qims-24-1643/rc).
Methods
Study participants
In total, 13 ALS patients who were admitted to the Neurology Department of the Peking University Third Hospital were enrolled in the study. All of the patients met the revised El Escorial criteria [1998] for the diagnosis of ALS. The study was conducted in accordance with the Declaration of Helsinki (as revised in 2013). The study was approved by the Ethics Committee of Peking University Third Hospital (approval No. M2017319), and informed consent was obtained from all individual participants. Of the 13 patients, 1 (male) was aged 30–39 years, 2 (male) were aged 40–49 years, 3 (male) were aged 50–59 years, 6 (5 male, and 1 female) were aged 60–69 years, and 1 (male) was aged 70–79 years. Among them, 1 male patient (S6) also had frontotemporal lobar dementia. The EEG data of all 13 patients were obtained. However, in the case of one patient (S13), the acquired signal was abnormal as the Pz lead could not correctly acquire the signal. Thus, the experimental data of this patient were excluded from the study, and only the data of the remaining 12 patients were used for the analysis. The patients participated in the SSVEP stimuli experiment with the shortest 3-second moving window, and their information is shown in Table 1.
Table 1
Patient number | Gender | Age (years) | Course of disease (months) | Onset site | Enrolment ALSFRS-R | Accuracy (%) | ITR (bits/min) | Mean identification time (s) | Total number of trials |
---|---|---|---|---|---|---|---|---|---|
S1 | Male | 63 | 20 | Cervical spinal cord | 38 | 75.00 | 73.1 | 2.62 | 80 |
S2 | Female | 67 | 20 | Medulla oblongata | 40 | 42.50 | 27.1 | 2.88 | 120 |
S3 | Male | 68 | 6 | Cervical spinal cord | 44 | 63.80 | 60.3 | 2.45 | 80 |
S4 | Male | 60 | 9 | Lumbar spinal cord | 41 | 36.30 | 20.4 | 2.96 | 80 |
S5 | Male | 55 | 59 | Lumbar spinal cord | 28 | 7.50 | 0.9 | 3.11 | 40 |
S6† | Male | 63 | 51 | Lumbar spinal cord | 8 | 10.00 | 2.1 | 2.72 | 80 |
S7 | Male | 63 | 45 | Lumbar spinal cord | 5 | 25.00 | 11.4 | 2.88 | 160 |
S8 | Male | 55 | 17 | Cervical spinal cord | 45 | 2.50 | 0 | 3.13 | 40 |
S9 | Male | 38 | 88 | Lumbar spinal cord | 36 | 50.00 | 37.3 | 2.70 | 160 |
S10 | Male | 72 | 41 | Thoracic spinal cord | 3 | 45.80 | 37.3 | 2.35 | 120 |
S11 | Male | 48 | 31 | Lumbar spinal cord | 31 | 5.00 | 0.3 | 2.97 | 80 |
S12 | Male | 51 | 24 | Cervical spinal cord | 33 | 10.80 | 2.2 | 3.12 | 120 |
Average | – | 58.58 | 34.25 | – | 35,229.33 | 31.18 | 22.7 | 2.82 | 96.67 |
†, patient 06 had ALS with frontotemporal dementia. ALS, amyotrophic lateral sclerosis; ALSFRS-R, Revised Amyotrophic Lateral Sclerosis Functional Rating Scale; ITR, information transmission rate.
Experimental design
The stimuli schedule consisted of 40 targets with stimuli frequencies ranging from 8 to 15.8 Hz, and intervals of 0.2 Hz (Figure 1). The corresponding initial phases of the target were [0, 0.5 π, 1 π, and 1.5 π], cycling sequentially with increasing frequency. For example, the “Number 1” stimulus frequency was 8 Hz and the initial phase was 0, while the “Letter Q” stimulus frequency was 8.2 Hz and the initial phase was 0.5 π, and the “Letter A” stimulus frequency was 8.4 Hz, and the initial phase was π.
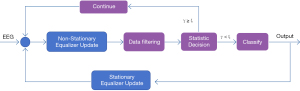
The brightness of each target was expressed as follows:
where f indicates the stimuli frequency, n indicates the display frame sequence number, and φ indicates the initial phase (15,16).
The experiment consisted of multiple blocks, each containing 40 trials. The patients participated in 1–4 blocks of experiments depending on their individual physical condition. In each block, each target was prompted once in a random order. A single trial lasted 5.5–6.5 seconds, and included the cue, stimuli, feedback, and rest stages (Figure 2). The first 0.5 seconds of the trial comprised the cue stage. During this stage, a red block appeared on the screen for 0.5 seconds, indicating the target position. The patient had to quickly shift their gaze to the prompted target. Next, came the stimulus stage, which lasted randomly for 3–4 seconds. In this stage, 40 targets flashed simultaneously, and the brightness of each target changed sinusoidally according to its predetermined frequency. The patient had to pay strict attention to the prompted target. At the same time, to facilitate the localization of the target, an empty red frame appeared around the prompted target during the stimulus process. After the stimulus stage ended, the system provided feedback for 2 seconds, and the cue time for the next trial started. The feedback stage results were returned in the form of a blue box and displayed on the interface.
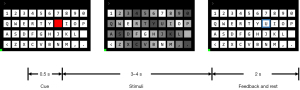
Experimental equipment
The BCI system used in the study consisted of stimuli equipment, EEG acquisition equipment, and a processing computer. The stimuli device was an ASUS MG279Q 27-inch display with 2,560×1,440 resolution (ASUSTek Computer Inc.), and a 60 Hz refresh rate. The acquisition device was a NeuSen W 64 64-lead EEG collector (Neuracle Inc., Shanghai, China). The processing device was a computer with an intel i9-9900K@3.6 GHz CPU and DDR4 32 GB of memory. The stimuli and processing programs were developed using Matlab 2015a (MathWorks, Inc., Natick, MA, USA) and Psychophysics Toolbox version 3. These stimuli and processing programs were also used in the 2018 World Robot Congress BCI Competition.
Implementation of a non-invasive BCI
In this study, the sampled sinusoidal stimuli were used to provide visual flickering stimuli encoded by the joint frequency-phase method on a 60-Hz liquid crystal display. The SSVEP signal was triggered by staring at the blinking 40-target virtual keyboard developed using the Psychtoolbox and MATLAB 2018b. The signal was then acquired using a wireless EEG amplifier at a sampling rate of 1,000 Hz, and pre-processed with a 5- to 100-Hz band-pass filter prior to classification. The electrode layout followed the standard 10 to 10 protocols, and only signals from nine channels (Pz, PO5, PO3, POz, PO4, PO6, O1, Oz, and O2) in the occipital region were recorded for the recognition experiment.
Data processing
The spatiotemporal equalization dynamic window (STE-DW) identification method (16) was used for the data processing to analyze and identify the EEG data. The algorithm was used to directly analyze and identify the SSVEP data. The STE-DW algorithm, a no-training method for real-time EEG signal analysis, used steady-state and non-steady-state equalizers for space-time filtering, with the filter order determined by an artificial intelligence classifier. It calculated a decision statistic γ; if γ was below a threshold “ϵ”, a recognition result was provided. The data were recorded with a 64-lead EEG cap, sampled at 1,000 Hz and downsampled to 250 Hz, focusing on nine occipital leads (Figure 3). The system started the data interception 140 ms after the trial trigger, analyzed the data in blocks of 40 ms, and stopped when the criteria were met or after 3 seconds. In the STE-DW algorithm, the cost coefficients were set as ϵ =[100, 10−2, 10−4, 10−6, 10−8, 10−10, 10−12, 10−14, 10−16], the adaptive range of the order of the spatiotemporal equalizer was set as 20≤ P ≤40, and the maximum adjacent trial of 20≤P≤40 noise estimation was set as M=10. Based on previous research (16), we selected ϵ =10−16 as the optimization cost function, and calculated the average trial duration of different patients.
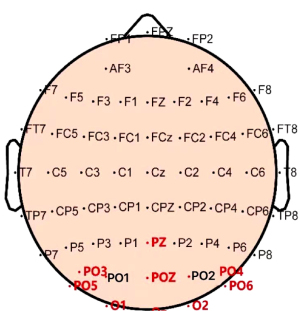
Results
SNRs of the SSVEP response of ALS patients
The patients with stronger signals had higher SNRs, which indicated that their SSVEP signals were more easily and correctly identified (Figure 4). The STE-DW method dynamically adjusted the stimuli time according to the SSVEP SNR of the patients to ensure high recognition efficiency. According to the characteristics of the SSVEP signaling, the evoked EEG response mainly contained fundamental frequency and multi-frequency sinusoidal components. Such components can be considered useful signals for SSVEPs while the other frequency components can be considered noise. The estimation method of the EEG signal SNR in the SSVEP-BCI was expressed as follows:
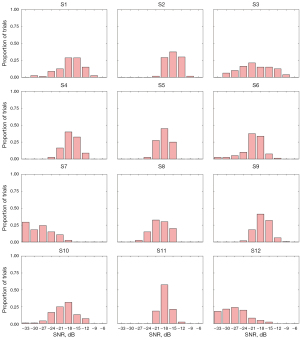
where denote L-channels, N-points denote the EEG data, denote the l-dimensional, and N-points denote the sinusoidal template signal, which is the semi-orthogonal matrix. The i-th row represents the i-th complex frequency component in the template signal. Based on the existing algorithm, a template dimension of l=10 was used in this study.
The SNRs of the different patients with ALS varied significantly, and were less than –18 dB in most trials. The SNRs of the ALS patients were much lower than those of healthy individuals. ALS patients usually require a longer stimuli time for effective recognition. This may be due to the age of the patients [see reference (17) for further information on the relationship between age and the SNRs of healthy individuals]. It is generally believed that older patients have relatively weak EEG signal responses. Moreover, due to the physical constraints related to the ALS disease state, it is difficult for ALS patients to maintain sustained targeted attention for a long time, which affects the quality of the acquired data.
The identification accuracy rate and ITR of SSVEPs of ALS patients
The correct response rate is a basic indicator for measuring the recognition accuracy of a system, and indicates the percentage of trials correctly classified by a recognition system across all trials (i.e., the number of the correct classification trials divided by the total number of trials) (Figure 5).
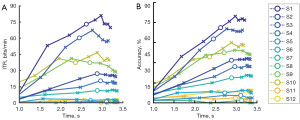
The ITR is often considered an ideal standard for measuring the efficiency of BCI systems (17). The optimization of this index is an important aspect of the system design of all kinds of BCI systems. The ITR was expressed as follows:
where Q indicates the number of targets, P indicates the recognition accuracy, and T indicates the average trial duration.
To simulate the online experimental results, the trial duration in the offline data ITR estimate was defined as the average stimuli duration plus a 0.5-second rest time. The identification time estimated by the online experiment ITR was defined as the length of time from the beginning of one stimulus to the beginning of the next stimulus (including the 0.5-second prompt time).
This study conducted a statistical analysis of the recognition results of all the trials participated in by each patient. If the feedback result of the STE-DW algorithm was consistent with the target annotated by the patient, it was classified as correct recognition; otherwise, it was classified as incorrect recognition. The number of all trials with correct recognition divided by the total number of trials completed by the patient was used to determine the detection accuracy of the STE-DW algorithm applied to the patient’s EEG signal. Similarly, the total duration of all trial recognitions divided by the total number of trials completed by the patient was used to determine the trial detection duration required for each patient. Using these two results, which were combined with Q=40 in the experimental paradigm, the ITR of each patient was determined using the ITR calculation formula.
As Figure 4 shows, the recognition efficiency of the patients differed, and the recognition rate was no more than 75% under the condition of 3 seconds at the most. In relation to the overall trend, the accuracy rate increased with time, indicating that the 3-second data did not meet the system recognition needs; thus, a longer stimuli time could further improve the recognition accuracy rate. Notably, stimuli with longer durations may be required to ensure correct recognition by ALS patients. Among the patients, patient S1 achieved a 75% recognition accuracy rate under the average condition of 2.62 s, which is similar to the recognition accuracy rate of healthy individuals who perform poorly.
The ITRs and accuracy rates of all patients were averaged. The average recognition accuracy rate of all the patients was about 31.2% under the ϵ =10−16 condition (Figure 6), which was significantly lower than the recognition accuracy rate of 91.5% reported in a study of healthy individuals with a 5-second maximum. While the mean ITR of all the patients was 22.7 bit/min, which was significantly lower than the ITR of 113 bit/min reported in a study of healthy individuals with a 5-second maximum.
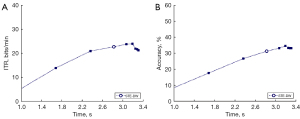
Time distribution of the SSVEP trial in ALS patients (Figure 7)
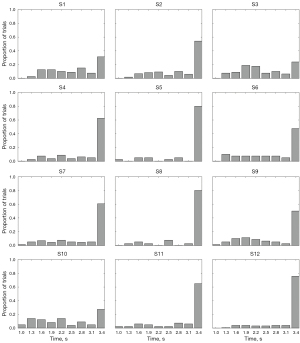
The average recognition time of healthy individuals is about 2.52 seconds (17). Conversely, the average recognition time of most ALS patients was more than 3 seconds. Thus, the signal quality of most trials was insufficient for ALS patients to make the decision within 3 seconds (see Figure 8).
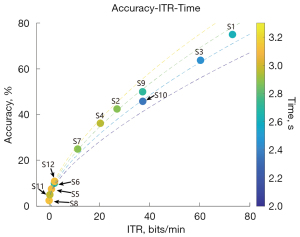
Discussion
This study showed that the algorithm results in this data set were relatively poor compared with those of healthy populations. The recognition accuracy rate of the ALS patients was poor; for example, the recognition accuracy rates of patients S5, S8, S11, and S12 were all less than 10%, indicating that the stimuli time window of up to 3 seconds was not adequate to meet the recognition needs of the ALS patients. Thus, ALS patients may require longer duration stimuli. Thus, the current BCI system’s performance was significantly limited for ALS patients, particularly those with severe motor impairments, and adjustments to the stimulation protocol need to be made to improve recognition accuracy.
The limited number of experimental trials failed to reflect the real process of SSVEP recognition. In a group of 40 trials, each target was prompted and appeared only once. If a patient lost concentration, no valid data could be acquired for the target. During the experiment, some of the patients did not focus sufficiently, which affected recognition. This might be because the experiment was not carried out in a special laboratory (but in clinical settings, conference rooms, and home environments), and the noise of the external environments might have affected the patients’ concentration. Conversely, it might be that the subjective cooperation of some patients in the trial was insufficient. In visual evoked potential (VEP) experiments, it is usually necessary for respondents to pay attention to active coordination; however, due to physical reasons, it was difficult for some of the ALS patients to complete all the experiments; thus, it was difficult to acquire complete data.
ALS patients show great heterogeneity in using BCIs. In this study, the most efficient patient had an accuracy rate of 75% and an ITR of 73.1 bits/min, which suggests that the BCI could be used to aid communication in ALS patients. However, the least efficient patient had an accuracy rate of only 2.5% and an ITR of 0 bits/min, which suggests that some patients are blind to SSVEPs; however, these patients might also have had impaired occipital lobe function. The total number of trials completed by the two least efficient patients was 40, which was significantly lower than that of the other patients, which suggests that the use of the BCI performance may improve with learning and adaptation. Thus, there were significant individual differences in the ability of ALS patients to use the BCI technology, and the effectiveness of the BCI was not consistent among these patients. Some patients showed relatively high communication efficiency using the BCI, while others barely benefited from it, possibly due to co-occurring impairments in their occipital lobe function. Further, the progression of ALS suggests that BCI technology has potential in terms of learnability and adaptability, which suggests that the ability of patients to use BCIs could potentially be improved through learning and adaptation.
The current experimental paradigm was designed based on the stimuli time and the total number of trials of healthy individuals. The efficiency values of the ALS patients were significantly lower than the published efficiency values of healthy individuals. Given their limited mental status and physical status, ALS patients often cannot fully cooperate to complete all the trials. Thus, in the future, we recommend that specific experimental setups be designed to suit the actual condition of ALS patients. For example, a code-modulated VEP paradigm could be used that incorporates more encoding strategies to implement different types of stimulus patterns (18), thereby establishing a VEP paradigm better suited for ALS patients.
The results of this study showed that the longer the course of the disease, the lower the ALSFRS-R (Revised Amyotrophic Lateral Sclerosis Functional Rating Scale) score, and the lower the usage efficiency. Additionally, the usage efficiency of the patients with lumbar myelopathy was generally lower than that of patients whose disease onset occurred in other regions. Patients with lumbar myelopathy have obvious lower limb involvement but their speech function and upper-limb activity are relatively preserved; thus, the degree of coordination in the BCI experiment were not high. However, further research with larger sample sizes and controlled studies needs to be conducted to examine the relationship between the various clinical indicators of ALS patients and the usage efficiency of BCIs to enable more accurate conclusions to be drawn.
This study had a number of limitations. The results of this data set indicate that the experimental paradigm for healthy individuals may need to be modified if good signal acquisition is to be achieved for patients with ALS. Additionally, if further experimental data are to be collected in follow-up studies, the experimental design should be modified to suit the actual condition of patients with ALS.
Conclusions
SSVEP-based non-invasive BCI technology shows promise as a communication aid for ALS patients; however, further algorithm optimization and larger sample sized controlled studies are necessary to improve its performance and usage efficiency, and to identify the disease-related influencing factors.
Acknowledgments
None.
Footnote
Reporting Checklist: The authors have completed the STROBE reporting checklist. Available at https://qims.amegroups.com/article/view/10.21037/qims-24-1643/rc
Funding: This study was supported by
Conflicts of Interest: All authors have completed the ICMJE uniform disclosure form (available at https://qims.amegroups.com/article/view/10.21037/qims-24-1643/coif). The authors have no conflicts of interest to declare.
Ethical Statement: The authors are accountable for all aspects of the work in ensuring that questions related to the accuracy or integrity of any part of the work are appropriately investigated and resolved. The study was conducted in accordance with the Declaration of Helsinki (as revised in 2013). The study was approved by the Ethics Committee of Peking University Third Hospital (approval No. M2017319), and informed consent was obtained from all individual participants.
Open Access Statement: This is an Open Access article distributed in accordance with the Creative Commons Attribution-NonCommercial-NoDerivs 4.0 International License (CC BY-NC-ND 4.0), which permits the non-commercial replication and distribution of the article with the strict proviso that no changes or edits are made and the original work is properly cited (including links to both the formal publication through the relevant DOI and the license). See: https://creativecommons.org/licenses/by-nc-nd/4.0/.
References
- Pinto WBVR, Debona R, Nunes PP, Assis ACD, Lopes CG, Bortholin T, Dias RB, Naylor FGM, Chieia MAT, Souza PVS, Oliveira ASB. Atypical Motor Neuron Disease variants: Still a diagnostic challenge in Neurology. Rev Neurol (Paris) 2019;175:221-32. [Crossref] [PubMed]
- Talbott EO, Malek AM, Lacomis D. The epidemiology of amyotrophic lateral sclerosis. Handb Clin Neurol 2016;138:225-38. [Crossref] [PubMed]
- Brown RH, Al-Chalabi A. Amyotrophic Lateral Sclerosis. N Engl J Med 2017;377:162-72. [Crossref] [PubMed]
- Rosa Silva JP, Santiago Júnior JB, Dos Santos EL, de Carvalho FO, de França Costa IMP, Mendonça DMF. Quality of life and functional independence in amyotrophic lateral sclerosis: A systematic review. Neurosci Biobehav Rev 2020;111:1-11. [Crossref] [PubMed]
- Salari E, Freudenburg ZV, Vansteensel MJ, Ramsey NF. Classification of Facial Expressions for Intended Display of Emotions Using Brain-Computer Interfaces. Ann Neurol 2020;88:631-6. [Crossref] [PubMed]
- Wolpaw JR, Wolpaw EW. Brain-computer interfaces: something new under the sun. In: Wolpaw JR, Wolpaw EW, editors. Brain-Computer Interfaces: Principles and Practice. 1st edition. Oxford: Oxford University Press; 2012:3-12.
- Wood H. Motor neuron disease: Brain-computer interface unlocks the mind of a patient with ALS. Nat Rev Neurol 2017;13:6. [Crossref] [PubMed]
- Vansteensel MJ, Pels EGM, Bleichner MG, Branco MP, Denison T, Freudenburg ZV, Gosselaar P, Leinders S, Ottens TH, Van Den Boom MA, Van Rijen PC, Aarnoutse EJ, Ramsey NF. Fully Implanted Brain-Computer Interface in a Locked-In Patient with ALS. N Engl J Med 2016;375:2060-6. [Crossref] [PubMed]
- Schwemmer MA, Skomrock ND, Sederberg PB, Ting JE, Sharma G, Bockbrader MA, Friedenberg DA. Meeting brain-computer interface user performance expectations using a deep neural network decoding framework. Nat Med 2018;24:1669-76. [Crossref] [PubMed]
- Pan J, Xie Q, Qin P, Chen Y, He Y, Huang H, Wang F, Ni X, Cichocki A, Yu R, Li Y. Prognosis for patients with cognitive motor dissociation identified by brain-computer interface. Brain 2020;143:1177-89. [Crossref] [PubMed]
- Yue L, Xiao X, Xu M, Chen L, Wang Y, Jung TP, Ming D. A brain-computer interface based on high-frequency steady-state asymmetric visual evoked potentials Annu Int Conf IEEE Eng Med Biol Soc 2020;2020:3090-3. [Crossref] [PubMed]
- Hosni SM, Borgheai SB, McLinden J, Shahriari Y. An fNIRS-Based Motor Imagery BCI for ALS: A Subject-Specific Data-Driven Approach. IEEE Trans Neural Syst Rehabil Eng 2020;28:3063-73. [Crossref] [PubMed]
- Guy V, Soriani MH, Bruno M, Papadopoulo T, Desnuelle C, Clerc M. Brain computer interface with the P300 speller: Usability for disabled people with amyotrophic lateral sclerosis. Ann Phys Rehabil Med 2018;61:5-11. [Crossref] [PubMed]
- Chaudhary U, Chander BS, Ohry A, Jaramillo-Gonzalez A, Lulé D, Birbaumer N. Brain Computer Interfaces for Assisted Communication in Paralysis and Quality of Life. Int J Neural Syst 2021;31:2130003. [Crossref] [PubMed]
- Zhu D, Bieger J, Garcia Molina G, Aarts RM. A survey of stimuli methods used in SSVEP-based BCIs. Comput Intell Neurosci 2010;2010:702357. [Crossref] [PubMed]
- Yang C, Han X, Wang Y, Saab R, Gao S, Gao X. A Dynamic Window Recognition Algorithm for SSVEP-Based Brain-Computer Interfaces Using a Spatio-Temporal Equalizer. Int J Neural Syst 2018;28:1850028. [Crossref] [PubMed]
- Yuan P, Gao X, Allison B, Wang Y, Bin G, Gao S. A study of the existing problems of estimating the information transfer rate in online brain-computer interfaces. J Neural Eng 2013;10:026014. [Crossref] [PubMed]
- Martínez-Cagigal V, Thielen J, Santamaría-Vázquez E, Pérez-Velasco S, Desain P, Hornero R. Brain-computer interfaces based on code-modulated visual evoked potentials (c-VEP): a literature review. J Neural Eng 2021; [Crossref]