Tumor habitat-derived radiomics features in pretreatment CT scans for predicting concurrent chemoradiotherapy responses in nasopharyngeal carcinoma: a retrospective study
Introduction
Nasopharyngeal carcinoma (NPC) is among the most common malignant tumors of the head and neck. The 5-year local recurrence-free survival rate ranges from 83.0% to 91.8% (1,2). NPC is effectively treated with simultaneous chemoradiotherapy, which combines chemotherapy and radiotherapy to significantly enhance therapeutic efficacy and patient survival rates (3). However, recurrence and metastasis remain the primary causes of treatment failure in NPC (4). Consequently, exploring novel therapeutic approaches and biomarkers is crucial for enhancing patient outcomes.
Computed tomography (CT) is a powerful noninvasive tool for diagnosing and staging tumors. Radiomics offers a novel method for non-invasively characterizing the tumor environment, by capturing high-throughput image features related to the cancer treatment response (5). Radiomics has been proven valuable in grading, risk stratification, differential diagnosis, and prognosis prediction in NPC, highlighting its role in assessing tumor heterogeneity and predicting poor treatment response (6-8).
Tumor heterogeneity, a key feature of malignancy, indicates variability in the tumor microenvironment characteristics, as revealed by radiomics analysis (9). Therefore, tumor biomarkers that reflect solid tumor characteristics, disregarding intratumoral heterogeneity (ITH), exhibit limited value for treatment response prediction (10). Previous research has indicated that understanding ITH in NPC can help us develop personalized treatment strategies. This allows for tailored therapeutic approaches that consider the unique biological characteristics of individual tumors (11). However, the complexities surrounding ITH remain inadequately addressed, creating a gap in the current understanding of how these variations affect clinical outcomes.
The concept of habitat regions, regions within a tumor with similar voxels, highlights regions of tumor recurrence and treatment-induced changes. It has emerged as a critical area of focus in the study of NPC, as it is believed to significantly impact treatment efficacy and patient survival (12-14). This study was based on the assumption that habitats exhibiting analogous imaging characteristics share comparable tumor biology. Previous studies have overlooked ITH, thus limiting their predictive value. However, identifying tumor heterogeneity could pave the way for personalized treatment strategies tailored to the unique biological characteristics of individual tumors.
To address this gap in knowledge, our study introduces an advanced radiomics model integrating extensive feature selection, in-depth tumor microenvironment analysis, data fusion, and clinical application. Incorporating machine learning into this analysis improves the accuracy and reliability of the process of tumor characterization, enhancing prognostic capabilities. This approach aims to develop predictive models that refine prognostic assessments and inform clinical decision-making. Thus, this research has two main objectives: to characterize ITH in NPC using quantitative imaging metrics and to correlate these features with clinical treatment responses. By establishing a comprehensive understanding of the interplay between ITH and patient prognosis, this study aspires to contribute valuable insights that can ultimately optimize therapeutic strategies for NPC patients. We present this article in accordance with the TRIPOD reporting checklist (available at https://qims.amegroups.com/article/view/10.21037/qims-24-1642/rc).
Methods
Data sets
This retrospective study was approved by the institutional review committee of Shandong Cancer Hospital (No. 2024002445), and the need for written informed consent from the patients was waived. We comprehensively reviewed the medical records of each patient in Shandong Cancer Hospital’s electronic medical record system. Data sets included pretreatment CT scans from NPC patients who underwent simultaneous chemoradiotherapy between March 2012 and September 2022. According to the National Comprehensive Cancer Network (NCCN) guidelines, simultaneous chemoradiotherapy is often the preferred treatment method for NPC patients without distant metastasis. Cisplatin is a commonly used chemotherapy agent for NPC, and treatment typically involves 2 to 3 cycles. Radiation therapy is typically administered at a dose of 66 to 70 Gy in 2 Gy daily fractions, 5 days a week. This study examined the short-term treatment response in NPC patients 2 years post-chemoradiotherapy, focusing on local control, recurrence, and metastasis. The treatment response was defined according to the Response Evaluation Criteria in Solid Tumors (RECIST) criteria (15). The study was conducted in accordance with the Declaration of Helsinki (revised in 2013).
The inclusion criteria for participants were: (I) biopsy-confirmed malignant NPC; (II) availability of CT images within a month before treatment; and (III) recorded evaluation of curative effects post-treatment. Exclusion criteria were: (I) images with severe artifacts; and (II) tumors with distant metastasis at initial presentation.
The analysis of ITH in NPC involves the following steps: data collection, region of interest (ROI) segmentation, ITH evaluation, sub-region clustering, feature extraction and selection, and model development. Figure 1 illustrates how a systematic analytical framework enhances our understanding of the biological characteristics of NPC, improving clinical decision-making and treatment outcomes.
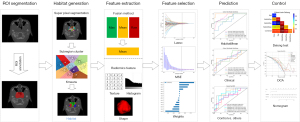
Image acquisition
All patients underwent both plain and contrast-enhanced CT scans using a Philips scanner (CT LightSpeed16, Philips, Amsterdam, Netherlands) with the following imaging parameters: tube voltage of 120 kV, tube current of 300 mA, thickness of 3 mm, and in-plane resolution of 0.97×0.97. All images were exported in the Digital Imaging and Communications in Medicine format for image feature extraction. According to standard protocols, during CT imaging acquisition, the images of each patient were reconstructed into three planes to generate three-dimensional information.
Data preprocessing
Our medical image analysis study employed two key preprocessing techniques to improve data reliability and consistency. The initial technique involved truncating pixel values to a designated intensity range between −200 and 400. The second method standardized voxel spacing across different volumes of interest to a uniform resolution of 1 mm × 1 mm × 1 mm using a fixed resolution resampling process. These critical steps were instrumental in facilitating precise image comparisons and substantially improving the robustness and accuracy of our analytical results.
Target region segmentation and habitat generation
The ROI selected encompassed malignant lesions that were clearly palpable or visually discernable. The three-dimensional tumor region, representing a volume created from multiple imaging slices, was manually delineated by two radiologists (with 8 and 10 years of experience) using MIM Maestro software (version 6.8.2 Cleveland, OH, USA). The number of slice images varied based on the tumor size and the scan layer thickness, usually requiring tens to hundreds of images. To minimize differences between observers, the final ROI was determined through the intersection of the results obtained by the two physicians. As shown in Figure 2, an algorithm was developed to delineate habitat regions within tumors using a four-stage process. The first step involved segmenting each tumor into 100 distinct subregions using a method called simple linear iterative clustering. Increasing the number of superpixel areas theoretically enhances division refinement but this significantly raises computational costs. Therefore, 100 superpixel areas were selected as the clustered regions in this study, representing an optimal trade-off between computational resources and performance.
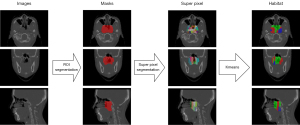
Next, we extracted 19 unique radiomics features (see Appendix 1) that primarily described the first-order and texture characteristics of the superpixel areas in each subregion. Similarly, while incorporating more features could result in the characterization being more refined, the computational costs would increase exponentially. The 19 features represent a compromise between computational capability and efficiency.
In the subsequent step, we applied K-means clustering to these features, enabling the classification of each subregion into distinct clusters. Finally, subregions sharing the same cluster identifiers were merged to form discrete habitat regions. This systematic approach markedly enhanced our ability to analyze the tumor microenvironment with greater precision and detail. As shown in Figure 2, the red areas in the second column represent the ROI regions that were segmented. The colored patches in the third column represent the superpixel segments obtained after superpixel segmentation. Each differently colored patch represents a superpixel region designated for aggregation. The different colors in the fourth column represent the outcomes of K-means clustering applied to these superpixel regions, with each color corresponding to a different habitat subregion. The specific process used to generate habitat regions is detailed in Appendix 2.
Feature extraction and selection
In our study, we organized features into three distinct groups for efficient extraction based on the following: (I) geometry, describing the three-dimensional shape of the tumor; (II) intensity, focusing on the first-order analysis of voxel intensity distribution within the tumor; and (III) texture, focusing on intensity patterns and spatial distributions using advanced second-order and higher-order analyses, including those involving the gray-level co-occurrence matrix. We conducted feature extraction using a pyRadiomics tool (version 3.0.1) following the Imaging Biomarker Standardization Initiative guidelines. A graphical depiction of the distribution of these 1,834 handcrafted feature groups is shown in Figure S1.
We used a systematic approach to feature selection and model building, applying statistical methods and machine learning algorithms to analyze tumor regions. We first normalized all features using Z-scores. Next, we conducted t-tests to determine the statistical significance of each feature, while retaining those with P values less than 0.05. To further refine our set of features, we evaluated repeatability by determining the Pearson’s correlation coefficient. In instances where feature pairs exhibited a high correlation (exceeding 0.9) was observed between, a greedy recursive deletion strategy was applied to retain only one feature from each highly correlated pair. For the final selection of features, we applied least absolute shrinkage and selection operator (LASSO) regression analysis to eliminate irrelevant features by reducing their coefficients to zero. The optimal regularization parameter (λ) for LASSO was identified through 10-fold cross-testing, ensuring a comprehensive feature selection process.
Model building
From the perspective of model construction, we aimed to predict the patient’s response to the treatment plan, a classification problem, rather than to describe disease progression, which involves continuous variables. Our study introduced four distinct models for tumor analysis: conventional radiomics (C-Rad), habitat radiomics (H-Rad), clinical, and combined models.
- C-Rad: after performing feature selection using LASSO regression, we applied the final set of features to different machine learning models. These models included the SVM, random forest, extra trees, extreme gradient boost (XGBoost), and light gradient boosting machine (LightGBM) models. The goal of using these diverse models was to construct a comprehensive classification model that leverages the unique advantages of each algorithm.
- H-Rad: habitat feature selection followed a methodology designed to maintain consistency within intra-radiomics models. We independently extracted features from specific habitat regions within each imaging subregion. However, as the number and distribution of subregions varied among patients, some regions lacked sufficient voxel counts for reliable feature extraction. To address this challenge, we implemented three specific strategies: extracting mean (HabitatMean) and maximum (HabitatMax) values for subregions with identical features, along with a feature pre-fusion approach termed HabitatRaw, which utilized characteristics from three distinct habitat areas. This multifaceted approach allowed for a more robust and comprehensive analysis of habitat features.
- Clinical model: our methodology involved univariate and stepwise multivariate analyses of all clinical features. Owing to the manageable number of features, each feature was incorporated into the construction of the clinical model, ensuring a thorough consideration of all potential clinical indicators. The clinical variables included in the analysis were age, sex, tumor-node-metastasis (TNM) staging, and tumor staging. The TNM system classifies tumors based on size (T), lymph node involvement (N), and metastasis (M). In this study, we used the latest American Joint Committee on Cancer (AJCC) 8th edition staging system for the TNM classification of tumors. The stage classifies tumors based on their extent and severity.
- Combined model: recognizing the significance of habitat regions in depicting the tumor microenvironment and the vital role of clinical data in diagnosis, we integrated insights from the habitat models with clinically relevant features. This integration involved a rigorous screening process that led to the formation of a combined model.
We employed receiver operating characteristic (ROC) curves to assess diagnostic accuracy. We also conducted calibration curve analysis and decision curve analysis (DCA) to evaluate the performance of the multi-classification model. To further validate the models’ performance, we transformed the three-class classification task into a binary one vs. others classification task and generated ROC curves to perform our analysis.
Statistical analysis
In our study, we performed statistical analysis to evaluate the clinical characteristics through various tests. We analyzed discrete and continuous variables using the χ2 test and independent sample analysis of variance (ANOVA), respectively. These analyses were conducted using the OnekeyAI platform (version 3.1.8) with Python software (version 3.7.12). Statsmodels version 0.13.2 was employed for statistical computations. Additionally, we implemented machine learning algorithms, including support vector machines (SVMs), using Scikit-learn (version 1.0.2).
Results
Patient characteristics
This study included 113 patients: 63 with local controls, 27 with recurrence, and 23 with distant metastasis. We randomly selected 30% of the participants from the training cohort to create an internal testing set. The refined model was then evaluated in the testing cohort to thoroughly assess performance and effectiveness. Detailed clinical characteristics of all the patients are provided in appendix available at https://cdn.amegroups.cn/static/public/qims-24-1642-1.xlsx. Table 1 summarizes the statistical data of all study participants.
Table 1
Characteristics | Train data set | Test data set | |||||||
---|---|---|---|---|---|---|---|---|---|
Control | Recurrence | Metastasis | P value | Control | Recurrence | Metastasis | P value | ||
Age (years) | 48.05±16.28 | 55.90±10.15 | 48.79±12.80 | 0.442 | 48.00±14.14 | 56.00±8.74 | 51.22±22.76 | 0.527 | |
Stage | 3.82±1.48 | 4.48±1.57 | 5.14±1.79 | 0.005 | 4.21±1.47 | 4.33±0.82 | 5.44±1.94 | 0.064 | |
Sex | 0.726 | 0.05 | |||||||
Female | 10 (22.73) | 3 (14.29) | 3 (21.43) | 8 (42.11) | 3 (50.00) | – | |||
Male | 34 (77.27) | 18 (85.71) | 11 (78.57) | 11 (57.89) | 3 (50.00) | 9 (100.00) | |||
T | 0.191 | 0.134 | |||||||
1 | 12 (27.27) | 5 (23.81) | 4 (28.57) | 5 (26.32) | – | – | |||
2 | 10 (22.73) | 4 (19.05) | 6 (42.86) | 5 (26.32) | – | 4 (44.44) | |||
3 | 17 (38.64) | 5 (23.81) | 2 (14.29) | 7 (36.84) | 5 (83.33) | 3 (33.33) | |||
4 | 5 (11.36) | 7 (33.33) | 2 (14.29) | 2 (10.53) | 1 (16.67) | 2 (22.22) | |||
N | <0.001 | 0.152 | |||||||
0 | 8 (18.18) | 9 (42.86) | 1 (7.14) | 6 (31.58) | 3 (50.00) | – | |||
1 | 14 (31.82) | 3 (14.29) | 2 (14.29) | 3 (15.79) | 1 (16.67) | 2 (22.22) | |||
2 | 18 (40.91) | 4 (19.05) | 2 (14.29) | 8 (42.11) | 2 (33.33) | 3 (33.33) | |||
3 | 4 (9.09) | 5 (23.81) | 9 (64.29) | 2 (10.53) | – | 4 (44.44) |
Data are presented as n (%) or mean ± standard deviation. Stage value: mean ± standard deviation in the C-Rad model. N, the involvement of regional lymph nodes; T, the size and extent of the primary tumor.
We extracted 1,834 distinct handcrafted radiomics features for each habitat area. First, we fused these features and then applied strict feature selection before using them for modeling. We used the LASSO method for feature selection with a logistic regression model. This approach played a crucial role in identifying non-zero coefficients, which were vital for calculating the radiomics score (Rad-score). A graphical representation of these coefficients and the mean standard error (MSE), derived from a 10-fold testing process, is shown in Figure S2.
The ExtraTrees model exhibited notable performance, with high area under the ROC curves (AUCs) of 0.969 and 0.894 in the training and testing cohorts, respectively, highlighting its effective discriminative ability. Other models, including SVM, RandomForest, XGBoost, and LightGBM, displayed varying degrees of effectiveness. While SVM and LightGBM showed strong performance in the training cohort, their results lacked consistency when applied to the test cohort. Overall, the ExtraTrees model was distinguished by its robust performance across both the training and testing cohorts. Table 2 presents the classification performance of different machine learning models, showcasing the unique strengths of each algorithm.
Table 2
Cohort | Model | AUC (95% CI) | Accuracy | Sensitivity | Specificity | PPV | NPV |
---|---|---|---|---|---|---|---|
Training | SVM | 0.963 (0.938–0.988) | 0.911 | 0.823 | 0.956 | 0.903 | 0.915 |
RandomForest | 0.890 (0.844–0.936) | 0.857 | 0.772 | 0.899 | 0.792 | 0.887 | |
ExtraTrees | 0.969 (0.948–0.991) | 0.878 | 0.671 | 0.981 | 0.946 | 0.856 | |
XGBoost | 0.936 (0.899–0.972) | 0.738 | 0.215 | 1.000 | 1.000 | 0.718 | |
LightGBM | 0.951 (0.924–0.978) | 0.886 | 0.684 | 0.987 | 0.964 | 0.862 | |
Testing | SVM | 0.887 (0.825–0.948) | 0.765 | 0.500 | 0.897 | 0.708 | 0.782 |
RandomForest | 0.819 (0.736–0.901) | 0.775 | 0.618 | 0.853 | 0.677 | 0.817 | |
ExtraTrees | 0.894 (0.835–0.954) | 0.794 | 0.441 | 0.971 | 0.882 | 0.776 | |
XGBoost | 0.806 (0.716–0.896) | 0.667 | 0.000 | 1.000 | 0.000 | 0.667 | |
LightGBM | 0.821 (0.741–0.901) | 0.745 | 0.382 | 0.926 | 0.722 | 0.750 |
AUC, area under the receiver operating characteristic curve; CI, confidence interval; C-Rad, conventional radiomics; LightGBM, light gradient boosting machine; NPV, negative prediction value; PPV, positive prediction value; SVM, support vector machine; XGBoost, extreme gradient boost.
Different number of clusters
Each ROI is divided into 100 superpixel areas, each characterized by 19 features, leading to data redundancy. Hence, we clustered these areas based on their features and selected the cluster number with the highest Calinski-Harabasz (CH) score. We validated the effectiveness of different clustering center numbers ranging from 2 to 10. As the number of clustering centers increased, the CH score increased initially and subsequently decreased. Its value was the highest when the number of clustering centers was 3. Therefore, we utilized three clustering centers as the optimal number of subregions for our habitat study. The clustering process and results are illustrated in Figure S3.
Our experimental findings indicate that the HabitatMean method demonstrated the best performance. Table 3 presents the micro-AUC results for habitat features calculated using the mean value.
Table 3
Cohort | Model | AUC (95% CI) | Accuracy | Sensitivity | Specificity | PPV | NPV |
---|---|---|---|---|---|---|---|
Training | Clinic | 0.903 (0.8370–0.9688) | 0.831 | 0.886 | 0.758 | 0.830 | 0.833 |
Rad | 0.918 (0.8483–0.9878) | 0.896 | 0.955 | 0.818 | 0.875 | 0.931 | |
HabitatRaw | 0.834 (0.7444–0.9236) | 0.766 | 0.727 | 0.818 | 0.842 | 0.692 | |
HabitatMax | 0.916 (0.8511–0.9808) | 0.857 | 0.864 | 0.848 | 0.884 | 0.824 | |
HabitatMean | 0.944 (0.8965–0.9905) | 0.883 | 0.955 | 0.788 | 0.857 | 0.929 | |
Combined | 0.961 (0.9240–0.9989) | 0.909 | 0.977 | 0.818 | 0.878 | 0.964 | |
Testing | Clinic | 0.684 (0.4962–0.8723) | 0.636 | 0.526 | 0.786 | 0.769 | 0.550 |
Rad | 0.733 (0.5494–0.9167) | 0.727 | 0.947 | 0.429 | 0.692 | 0.857 | |
HabitatRaw | 0.782 (0.6126–0.9513) | 0.727 | 0.684 | 0.786 | 0.812 | 0.647 | |
HabitatMax | 0.767 (0.5842–0.9496) | 0.758 | 0.842 | 0.643 | 0.762 | 0.750 | |
HabitatMean | 0.731 (0.5561–0.9063) | 0.667 | 0.632 | 0.714 | 0.750 | 0.588 | |
Combined | 0.861 (0.7288–0.9930) | 0.818 | 0.947 | 0.643 | 0.783 | 0.900 |
AUC, area under the receiver operating characteristic curve; CI, confidence interval; NPV, negative prediction value; PPV, positive prediction value; Rad, radiomics.
Clinical model
Our study conducted a detailed univariate analysis of clinical features, including age, sex, and tumor age. This process mainly involved calculating the odds ratio (OR) and corresponding P values for each feature, as shown in Table S1. These features were chosen to develop the nomogram due to their established relevance and importance in statistical analysis, as shown in Figure 3.
Control vs. others
Our study evaluated various models using ExtraTrees aggregation, revealing distinct performance levels measured by the AUC value. In the training cohort, the combined model demonstrated the highest AUC of 0.961, indicating superior predictive accuracy. The HabitatMean model also showed excellent performance, with an AUC of 0.944, closely followed by the Rad model and HabitatMax model, with AUCs of 0.918 and 0.916, respectively. The clinic model and HabitatRaw model showed moderate effectiveness, with AUCs of 0.903 and 0.834, respectively. In the testing cohort, the combined model again outperformed the other models, achieving a notably higher AUC of 0.861. The HabitatRaw model showed a relatively better performance with an AUC of 0.782, while the Rad and HabitatMax models displayed similar levels of effectiveness, with AUCs of 0.733 and 0.767, respectively. The clinic and HabitatMean models, however, demonstrated limited effectiveness in the testing cohort, with AUCs of 0.684 and 0.731, respectively. Overall, the high AUC in the combined model in both cohorts highlights its robustness and effective integration of features for predictive analysis. Table 3 displays the prediction performance of four distinct models in the training and testing cohorts.
Calibration curve analysis
The Hosmer-Lemeshow (HL) test is crucial for assessing the calibration of predictive models by comparing predicted probabilities with actual outcomes. Generally, a lower HL test statistic indicates better model calibration, implying that the model’s predictions align more closely with the observed results. In our study, the combined model demonstrated outstanding calibration performance in all cohorts (Figure 4). This is reflected in its low HL test statistics: 0.890 in the training cohort and 0.593 in the testing cohort. These values indicate a high degree of reliability in its predictions.
Clinical use
Figure 5 shows DCA curves for both the training and testing sets. The results indicate that our fusion model offers significant advantages according to the predicted probabilities. Furthermore, compared to other models, it demonstrates greater potential for delivering overall benefits.
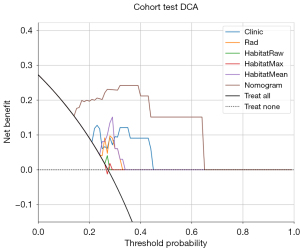
Discussion
In this study, we developed an innovative approach to address the complexities associated with medical image analysis. Our research introduces an advanced radiomics model that effectively integrates comprehensive feature selection, tumor microenvironment analysis, data integration, and direct clinical applicability. Detailed methodological descriptions of the research process and specific details are provided to ensure that this method can be applied to address other clinical issues, ensuring a high degree of reproducibility. Our approach features several key advancements. First, an in-depth analysis of the tumor microenvironment involves a detailed examination of various zones within the tumor. The predictive accuracy of our analysis was significantly enhanced by integrating insights from these zones. Shi et al. (16) developed a model for breast cancer patients that combined an index derived from pretreatment magnetic resonance imaging (MRI) imaging features measuring ITH, C-Rad scores, and clinicopathologic variables. That model effectively predicted pathological complete response (pCR) to neoadjuvant chemotherapy, achieving an AUC of 0.90 in training data and an AUC of 0.83 to 0.87 in external test datasets. Recently, Jiang et al. (17) found that deep learning radiomics techniques can accurately predict the tumor microenvironment status in gastric cancer, allowing for better monitoring and tracking of responses to cancer therapy. Feng et al. (18) reported that the CT radiomics model accurately predicts the macrotrabecular-massive subtype and could be used to investigate underlying immune infiltration patterns, with AUCs ranging from 0.74 to 0.84. Increasing evidence indicates that the tumor microenvironment (TME) significantly influences cancer biology, leading researchers to quantify the TME for identifying new prognostic biomarkers for targeted therapies in immunotherapy (19,20).
Secondly, our approach integrates clinical features, tumor habitat-related characteristics, and deep learning outcomes to create a user-friendly model. This model aims to enhance the precision of prognostic predictions and assist in clinical decision-making. Previous studies have used imaging features and clinicopathologic variables to construct models for predicting the treatment response in NPC. Kim et al. (6) integrated a multiparametric MRI signature and clinical variables, achieving a C-index of 0.74 in the testing cohort. Du et al. (7) developed a multi-task deep learning model (MTDLR) and evaluated its prognostic prediction capabilities in locally advanced NPC (LA-NPC) patients, achieving AUCs of 0.769 in the testing dataset. The habitat model developed in the current study achieved AUCs of 0.944 and 0.731 in the training and testing datasets, respectively. These results are comparable to those reported in previous studies. The integration of the clinical index into this model resulted in the highest performance among all testing models. It achieved AUCs of 0.961 and 0.861 in the training and testing data sets, respectively. Moreover, DCA demonstrated that the combined model provided a greater net benefit than the Rad, habitat, and clinical models across various potential thresholds in both data sets. This finding underscores the potential clinical utility of incorporating habitat information into prediction models.
Targeted studies on recurrence, metastasis, and control were conducted using a comparative research methodology that contrasts a single group against others. NPC is a radiosensitive epithelial malignancy characterized by the risk of both locoregional invasion and distant metastasis. It has the highest prevalence in certain populations (21). This approach enables a comprehensive comparison of model performance across these categories.
Several studies have shown a relationship between ITH and treatment responses. However, previously developed quantitative imaging analysis methods did not fully examine the relationship between C-Rad, H-Rad features, and clinical outcomes (22,23). For example, Wu et al. (10) characterized spatial heterogeneity in perfusion MRI by quantifying multiple spatially distinct tumor subregions segmented using four dynamic maps from dynamic contrast-enhanced MRI. However, the applicability of this approach to other imaging methods [such as CT and positron emission tomography (PET)], remains uncertain. In the current study, we implemented K-means clustering for habitat regions, making it applicable to similar research. H-Rad features, reflecting intratumor heterogeneity, have been proposed for developing prediction models for treatment responses. These models exhibited reasonable discriminatory ability and generalizability in the testing data sets (AUC, 0.861), comparable to the AUC ranges reported by both Zhang et al. (24) (0.77–0.85) and Peng et al. (25) (0.78–0.83).
Despite challenges associated with a minimal dataset, our study’s validity is supported by a clearly defined research question, high-quality data, advanced statistical methods, preliminary findings that pave the way for future research, result reproducibility, and real-world applicability. However, several notable limitations are associated with this study. First, the retrospective nature of the study may introduce bias due to the single-center population selection. Further prospective and multi-center studies need to investigate the quantitative assessment of NPC heterogeneity. Second, while we utilized a model established using CT images, images obtained using other modalities (MRI, PET) also provide important information. The incorporation of multimodal images in future studies is expected to further improve the prediction performance of the model. Finally, the inclusion of a limited set of clinical features in our study might have limited our model’s performance. Identifying additional, more effective clinical features could further enhance its predictive performance.
In conclusion, the model developed in this study, which integrated C-Rad, ITH, and clinical features, showed the best performance for predicting the treatment response to concurrent chemoradiotherapy in NPC patients. Future research should explore the underlying biological mechanisms of imaging-based methods for quantifying heterogeneity in NPC by incorporating multimodal data.
Conclusions
In conclusion, the investigation of ITH in NPC represents a promising avenue for future research, with the potential to transform clinical practice by facilitating personalized treatment approaches. By leveraging advanced imaging modalities and machine learning techniques, our study aims to provide a deeper understanding of the biological foundations of NPC and contribute to the development of more effective, patient-specific therapeutic interventions.
Acknowledgments
The authors thank the Onekey platform for its technical support.
Footnote
Reporting Checklist: The authors have completed the TRIPOD reporting checklist. Available at https://qims.amegroups.com/article/view/10.21037/qims-24-1642/rc
Funding: This work was supported by
Conflicts of Interest: All authors have completed the ICMJE uniform disclosure form (available at https://qims.amegroups.com/article/view/10.21037/qims-24-1642/coif). The authors have no conflicts of interest to declare.
Ethical Statement: The authors are accountable for all aspects of the work in ensuring that questions related to the accuracy or integrity of any part of the work are appropriately investigated and resolved. This retrospective study was approved by the institutional review committee of Shandong Cancer Hospital (No. 2024002445), and the need for written informed consent from the patients was waived. The study was conducted in accordance with the Declaration of Helsinki (revised in 2013).
Open Access Statement: This is an Open Access article distributed in accordance with the Creative Commons Attribution-NonCommercial-NoDerivs 4.0 International License (CC BY-NC-ND 4.0), which permits the non-commercial replication and distribution of the article with the strict proviso that no changes or edits are made and the original work is properly cited (including links to both the formal publication through the relevant DOI and the license). See: https://creativecommons.org/licenses/by-nc-nd/4.0/.
References
- Setton J, Han J, Kannarunimit D, Wuu YR, Rosenberg SA, DeSelm C, Wolden SL, Jillian Tsai C, McBride SM, Riaz N, Lee NY. Long-term patterns of relapse and survival following definitive intensity-modulated radiotherapy for non-endemic nasopharyngeal carcinoma. Oral Oncol 2016;53:67-73. [Crossref] [PubMed]
- Sun X, Su S, Chen C, Han F, Zhao C, Xiao W, Deng X, Huang S, Lin C, Lu T. Long-term outcomes of intensity-modulated radiotherapy for 868 patients with nasopharyngeal carcinoma: an analysis of survival and treatment toxicities. Radiother Oncol 2014;110:398-403. [Crossref] [PubMed]
- Chen YP, Chan ATC, Le QT, Blanchard P, Sun Y, Ma J. Nasopharyngeal carcinoma. Lancet 2019;394:64-80. [Crossref] [PubMed]
- Lee AW, Ma BB, Ng WT, Chan AT. Management of Nasopharyngeal Carcinoma: Current Practice and Future Perspective. J Clin Oncol 2015;33:3356-64. [Crossref] [PubMed]
- Guiot J, Vaidyanathan A, Deprez L, Zerka F, Danthine D, Frix AN, Lambin P, Bottari F, Tsoutzidis N, Miraglio B, Walsh S, Vos W, Hustinx R, Ferreira M, Lovinfosse P, Leijenaar RTH. A review in radiomics: Making personalized medicine a reality via routine imaging. Med Res Rev 2022;42:426-40. [Crossref] [PubMed]
- Kim MJ, Choi Y, Sung YE, Lee YS, Kim YS, Ahn KJ, Kim MS. Early risk-assessment of patients with nasopharyngeal carcinoma: the added prognostic value of MR-based radiomics. Transl Oncol 2021;14:101180. [Crossref] [PubMed]
- Du D, Feng H, Lv W, Ashrafinia S, Yuan Q, Wang Q, Yang W, Feng Q, Chen W, Rahmim A, Lu L. Machine Learning Methods for Optimal Radiomics-Based Differentiation Between Recurrence and Inflammation: Application to Nasopharyngeal Carcinoma Post-therapy PET/CT Images. Mol Imaging Biol 2020;22:730-8. [Crossref] [PubMed]
- Zhu C, Huang H, Liu X, Chen H, Jiang H, Liao C, Pang Q, Dang J, Liu P, Lu H. A Clinical-Radiomics Nomogram Based on Computed Tomography for Predicting Risk of Local Recurrence After Radiotherapy in Nasopharyngeal Carcinoma. Front Oncol 2021;11:637687. [Crossref] [PubMed]
- Duan W, Xiong B, Tian T, Zou X, He Z, Zhang L. Radiomics in Nasopharyngeal Carcinoma. Clin Med Insights Oncol 2022;16:11795549221079186. [Crossref] [PubMed]
- Wu J, Cao G, Sun X, Lee J, Rubin DL, Napel S, Kurian AW, Daniel BL, Li R. Intratumoral Spatial Heterogeneity at Perfusion MR Imaging Predicts Recurrence-free Survival in Locally Advanced Breast Cancer Treated with Neoadjuvant Chemotherapy. Radiology 2018;288:26-35. [Crossref] [PubMed]
- Lu B, Shi J, Cheng T, Wang C, Xu M, Sun P, Zhang X, Yang L, Li P, Wu H, Kuai X. Chemokine ligand 14 correlates with immune cell infiltration in the gastric cancer microenvironment in predicting unfavorable prognosis. Front Pharmacol 2024;15:1397656. [Crossref] [PubMed]
- Liu W, Wang W, Guo M, Zhang H. Tumor habitat and peritumoral region evolution-based imaging features to assess risk categorization of thymomas. Clin Radiol 2024;79:e1117-25. [Crossref] [PubMed]
- Ge W, Fan X, Zeng Y, Yang X, Zhou L, Zuo Z. Exploring habitats-based spatial distributions: improving predictions of lymphovascular invasion in invasive breast cancer. Acad Radiol 2024;31:4317-28. [Crossref] [PubMed]
- Su GH, Xiao Y, You C, Zheng RC, Zhao S, Sun SY, Zhou JY, Lin LY, Wang H, Shao ZM, Gu YJ, Jiang YZ. Radiogenomic-based multiomic analysis reveals imaging intratumor heterogeneity phenotypes and therapeutic targets. Sci Adv 2023;9:eadf0837. [Crossref] [PubMed]
- Eisenhauer EA, Therasse P, Bogaerts J, Schwartz LH, Sargent D, Ford R, Dancey J, Arbuck S, Gwyther S, Mooney M, Rubinstein L, Shankar L, Dodd L, Kaplan R, Lacombe D, Verweij J. New response evaluation criteria in solid tumours: revised RECIST guideline (version 1.1). Eur J Cancer 2009;45:228-47. [Crossref] [PubMed]
- Shi Z, Huang X, Cheng Z, Xu Z, Lin H, Liu C, Chen X, Liu C, Liang C, Lu C, Cui Y, Han C, Qu J, Shen J, Liu Z. MRI-based Quantification of Intratumoral Heterogeneity for Predicting Treatment Response to Neoadjuvant Chemotherapy in Breast Cancer. Radiology 2023;308:e222830. [Crossref] [PubMed]
- Jiang Y, Zhou K, Sun Z, Wang H, Xie J, Zhang T, Sang S, Islam MT, Wang JY, Chen C, Yuan Q, Xi S, Li T, Xu Y, Xiong W, Wang W, Li G, Li R. Non-invasive tumor microenvironment evaluation and treatment response prediction in gastric cancer using deep learning radiomics. Cell Rep Med 2023;4:101146. [Crossref] [PubMed]
- Feng Z, Li H, Liu Q, Duan J, Zhou W, Yu X, Chen Q, Liu Z, Wang W, Rong P. CT Radiomics to Predict Macrotrabecular-Massive Subtype and Immune Status in Hepatocellular Carcinoma. Radiology 2023;307:e221291. [Crossref] [PubMed]
- Jin MZ, Jin WL. The updated landscape of tumor microenvironment and drug repurposing. Signal Transduct Target Ther 2020;5:166. [Crossref] [PubMed]
- Takaki H, Cornelis F, Kako Y, Kobayashi K, Kamikonya N, Yamakado K. Thermal ablation and immunomodulation: From preclinical experiments to clinical trials. Diagn Interv Imaging 2017;98:651-9. [Crossref] [PubMed]
- de Martel C, Georges D, Bray F, Ferlay J, Clifford GM. Global burden of cancer attributable to infections in 2018: a worldwide incidence analysis. Lancet Glob Health 2020;8:e180-90. [Crossref] [PubMed]
- Dagogo-Jack I, Shaw AT. Tumour heterogeneity and resistance to cancer therapies. Nat Rev Clin Oncol 2018;15:81-94. [Crossref] [PubMed]
- Lüönd F, Tiede S, Christofori G. Breast cancer as an example of tumour heterogeneity and tumour cell plasticity during malignant progression. Br J Cancer 2021;125:164-75. [Crossref] [PubMed]
- Zhang B, He X, Ouyang F, Gu D, Dong Y, Zhang L, Mo X, Huang W, Tian J, Zhang S. Radiomic machine-learning classifiers for prognostic biomarkers of advanced nasopharyngeal carcinoma. Cancer Lett 2017;403:21-7. [Crossref] [PubMed]
- Peng L, Hong X, Yuan Q, Lu L, Wang Q, Chen W. Prediction of local recurrence and distant metastasis using radiomics analysis of pretreatment nasopharyngeal [18F]FDG PET/CT images. Ann Nucl Med 2021;35:458-68.