Qualitative and quantitative reproducibility of 3D MERGE and SNAP sequences for carotid vessel wall imaging across Siemens and Philips 3T scanners
Introduction
Stroke is the second leading cause of death worldwide (1), with carotid atherosclerosis contributing to approximately 10–20% of ischemic stroke (2). Two-dimensional (2D) multi-contrast vessel wall magnetic resonance imaging (MRI) has been widely used for the characterization of carotid vessel wall pathology, which is valuable for stroke risk stratification and treatment guidance (3-7). The reliability of 2D multi-contrast vessel wall MRI has been validated by a reproducibility test in a multicenter study on different MRI vendors (8).
Over the past decade, 3-dimensional (3D) vessel wall MRI sequences have emerged as new imaging tools for evaluating carotid atherosclerosis (9-11). Compared with the 2D vessel wall MRI, 3D vessel wall MRI can provide higher spatial resolution in the slice direction and, hence, improve the accuracy of measuring vessel wall morphology and plaque composition. 3D motion-sensitized driven equilibrium (MSDE) prepared rapid gradient echo (MERGE) (9) and simultaneous non-contrast angiography and plaque (SNAP) imaging (11) are two representative 3D vessel wall MRI sequences. Their effectiveness in visualizing and analyzing carotid plaque has been demonstrated in several studies (12-14): 3D MERGE provides superior delineation of vessel wall morphology (9) compared with 2D protocols, and SNAP offers a higher sensitivity in identifying intraplaque hemorrhage (IPH) than magnetization-prepared rapid gradient-echo imaging (MPRAGE) (15). Given that 3D MERGE and SNAP provide complementary roles in evaluating carotid atherosclerosis, they are suitable for serving as 3D vessel wall MRI protocols in multicenter and multivendor studies.
Despite these advantages, 3D MERGE and SNAP have only been available on Philips scanners. This limitation not only restricts their use in multicenter studies but also hinders their broader application in clinical practice. It is unknown whether implementations of 3D MERGE and SNAP on other scanner platforms will produce comparable image quality and quantitative measurements, such as vessel wall thickness. Extending 3D MERGE and SNAP to the other platforms with similar quality to that of the Philips platform could potentially facilitate multicenter studies.
In this study, we aim to evaluate the reproducibility of 3D MERGE and SNAP for carotid vessel wall imaging across Siemens and Philips—two widely used MRI platforms—on healthy volunteers. We first implemented these two sequences on the Siemens platform and then acquired carotid MRI data using matched parameters on the same six healthy volunteers. Image quality was assessed by experienced reviewers. An automated artificial intelligence framework, termed 3D localization, analysis, and thickness and tissue evaluation (LATTE) (16-18) was utilized to automatically process 3D MERGE imaging data to detect carotid bifurcation and segment lumen and outer wall, with subsequent manual corrections. Then, quantitative vessel wall morphological measurements were performed using a novel 3D thickness measurement using Laplacian method (19) and were compared between Philips and Siemens platforms. We present this article in accordance with the STROBE reporting checklist (available at https://qims.amegroups.com/article/view/10.21037/qims-24-2124/rc).
Methods
Sequence implementation
We programmed 3D MERGE and SNAP sequences on the Siemens platform following the literature, with the sequence diagrams shown in Figure S1. Briefly, 3D MERGE is an improved motion-sensitized driven-equilibrium (iMSDE) (20)-prepared 3D FLASH sequence, and SNAP is an inversion recovery sequence with two inversion times (TI). In 3D MERGE, the flowing blood is dephased by the iMSDE preparation, with two 180 refocusing RF pulses for reduced eddy current accumulation and thus improved image quality compared with MSDE; and FLASH acquisition with “Centric” k-space ordering is applied for optimal blood suppression by acquiring center k-space signals first. In SNAP, the imaging data are acquired at the first TI; while the phase reference data serving for the phase-sensitive inversion recovery (PSIR) reconstruction were acquired at the second TI. PSIR was implemented here as an online reconstruction pipeline on the Siemens scanner. Note that different fat suppression methods are used for these two sequences: 3D MERGE uses fat saturation while SNAP uses water excitation to match the original protocols implemented on Philips scanners (21).
Subjects
Six healthy volunteers aged between 22 and 67 years (one female and five males) and one carotid atherosclerotic patient (male, 70 years old) were included in this study. The six healthy volunteers participated in the MRI study at two different institutes: (I) University of Utah equipped with a Siemens scanner; and (II) University of Washington with a Philips scanner. The carotid atherosclerotic patient only participated in the MRI study at University of Utah.
The study was conducted in accordance with the Declaration of Helsinki (as revised in 2013). The study was approved by the institutional review boards (IRB) of the University of Utah (IRB_00162281) and University of Washington (No. STUDY00007076), and written consent was obtained from each participant.
Data acquisition
Carotid MRI data were acquired on a Siemens 3.0T MRI scanner (Prisma-fit, Erlangen, Germany) at University of Utah, and a Philips 3.0T MRI scanner (Ingenia CX, Best, The Netherlands) at University of Washington. No contrast was used for scans at both institutes.
For the Siemens MRI scans, a custom-designed, neck-shape-specific carotid coil (7 channels) (22) combined with a Siemens 20-channel head/neck coil was used. For the Philips MRI scans, a custom-designed 8-channel carotid coil (23) was used. Both 3D MERGE and SNAP were used for data acquisition on both vendors. Imaging parameters on both vendors were matched as best as possible, including resolution, echo time (TE), repetition time (TR), and TI (Table 1). The scan time on Philips was approximately 20% shorter due to elliptical sampling, which was not implemented on the sequences we programmed on Siemens but had a minor impact on the qualitative and quantitative comparison, except for slightly increased SNR (24). Before the 3D vessel wall MRI sequence, a quick 2-minute 2D time-of-flight (TOF) MRI sequence was used to obtain a reference for the positioning of 3D scans.
Table 1
Parameters | 3D MERGE (coronal) | SNAP (coronal) | |||
---|---|---|---|---|---|
Philips | Siemens | Philips | Siemens | ||
FOV (mm in each axis) | 250×160×42 | 250×160×42 | 160×160×32 | 160×160×32 | |
Acquired voxel (mm in each axis) | 0.8×0.8×0.8 | 0.82×0.82×0.82 | 0.8×0.8×0.8 | 0.83×0.83×0.83 | |
Interpolated voxel (mm in each axis) | 0.39×0.39×0.39 | 0.41×0.41×0.41 | 0.4×0.4×0.4 | 0.41×0.41×0.41 | |
Flip angle (degree) | 6 | 6 | 11/5 | 11/5 | |
TE/TR (ms) | 4.3/9.1 | 4.3/9.1 | 4.8/10 | 4.8/9.6 | |
TI (ms) | – | – | 500 | 500 | |
iMSDE preparation time (ms) | 22 | 22 | – | – | |
Number of segments | 22 | 22 | 98 | 98 | |
Number of averages | 2 | 2 | 2 | 2 | |
Scan time (min:sec)a | 3:36 | 4:35 | 5:17 | 6:26 |
a, Philips scan was ~20% shorter due to elliptical sampling. 3D MERGE, three dimensional motion-sensitized driven equilibrium prepared rapid gradient echo; FOV, field of view; iMSDE, improved motion-sensitized driven equilibrium; SNAP, simultaneous non-contrast angiography and plaque; TE, echo time; TI, inversion time; TR, repetition time.
In addition to human data acquisition, we conducted a phantom experiment to compare the capability of the setups in Siemens and Philips scanners, using ACR phantom and same coils and protocols as the human experiments.
Image processing and review
Qualitative analysis
Image quality scoring on healthy volunteer images was performed by two independent reviewers (two medical doctors with 2 years of experience in vessel wall analysis), who were blinded to the MRI parameters, vendors, and patient details, ensuring an unbiased assessment based purely on the quality of the images presented. They assigned a 4-point quality score (21,25) to each of the four predetermined locations [common carotid artery (CCA), bifurcation, internal carotid artery (ICA), and external carotid artery (ECA)] using the axial view images reformatted from the original acquisition view (i.e., coronal). The scoring system was as follows: 1= inadequate image quality (significant artifact or noise interference, unclear vessel wall boundaries), 2= adequate image quality (moderate artifact or noise interference, identifiable but less distinct vessel wall boundaries), 3= good image quality (minimal artifact or noise interference, clear and distinct vessel wall boundaries), 4= excellent image quality (no artifact or noise interference, sharp and distinct vessel wall boundaries). 3D MERGE and SNAP images were scored separately, and the independent image quality scores from both reviewers were averaged for each location and sequence.
In addition, SNAP images acquired on both healthy volunteers and the carotid atherosclerotic patient were visually inspected for IPH detection.
Vessel segmentation
The 3D MERGE images acquired from healthy volunteers on both vendors underwent automated processing using an in-house deep learning framework called 3D LATTE (17). This framework employs a three-step approach to process the black blood vessel wall MRI data (Figure S2). Initially, it detects the location of the right and left bifurcation slices. Subsequently, these identified slices are utilized to extract axial slices that cover the bifurcation region, as well as the distal and proximal carotid arteries, for further processing. In the next step, the carotid arteries are precisely localized through 3D segmentation of their lumens. Finally, the localization results were employed to extract 2D patches along the lumens of both left and right carotids, serving the purpose of final lumen and vessel wall segmentation using 2D convolutional neural networks. The segmentation results were then blindly reviewed and manually corrected by one trained reviewer, a medical doctor with 2 years of experience in vessel wall analysis, to ensure accuracy, using ITK-SNAP (www.itksnap.org) (26). Manual corrections were made, if necessary, to refine the boundaries of the lumen and vessel wall and improve the overall segmentation quality.
Quantitative analysis
A novel 3D thickness measurement approach using the Laplacian method (19) was applied to the final segmentation of 3D MERGE images acquired from healthy volunteers. In order to compare the quantitative measurements between Siemens and Philips at different artery locations, a bifurcation coordinate system was constructed by assigning 1D distance along the centerline from CCA to ICA as well as from CCA to ECA, with carotid bifurcation as the zero point (19). Metrics of arterial morphology, mean wall thickness, maximum wall thickness, normalized wall index (wall volume divided by total vessel volume), and normalized lumen index (lumen volume divided by total vessel volume) were calculated for each distance, each side of the arteries, and each volunteer. Then, location-specific morphology metrics were computed in 11 locations centered around the bifurcation, with each location containing a 7-mm distance (i.e., covering coordinates from −38.5 to 38.5 mm). Specifically, each metric was calculated as the average value along the 7 mm distance in each location.
Statistical analysis
To compare image quality between the two vendors, a t-test was used to examine if there was a significant difference between the qualitative scores rated by the reviewers. Since the image quality of each side of the artery was rated separately, the total number of samples was 12 (2 sides × 6 subjects). For SNAP, two young volunteers had strong flow artifacts due to large blood velocities (27), so they were excluded from the statistical analysis (i.e., the number of samples was 8). The difference was considered significant if P<0.05. In addition, quadratic weighted Cohen’s kappa analysis was used to test the inter-rater reliability.
Bland-Altman analysis and intra-class correlation coefficient (ICC) were used to assess the reproducibility of quantitative metrics measured from the images of two vendors, with the total number of samples being 132 (6 volunteers × 2 arteries × 11 locations) (28). In addition, the analysis was done in each artery region: CCA (coordinate from −31.5 to −10.5 mm, N=12), bifurcation (coordinate from from −10.5 to 10.5 mm, combining both ICA and ECA, N=24), ICA (coordinate from 10.5 to 31.5 mm, N=12), and ECA (coordinate from 10.5 to 31.5 mm, N=12).
Results
Phantom experiment
As shown in Figure 1, both 3D MERGE and SNAP have visually reasonable image qualities on both Philips and Siemens scanners, although they exhibit different uniformity patterns. SNR is measured in homogeneous ROIs on both the left and right side for each image, defined by dividing the ROI-averaged signal intensity by the standard deviation. Results show that SNR (averaging left and right) is similar in both sequences (Philips vs. Siemens): 21.4 vs. 21.8 in 3D MERGE; 22.75 vs. 20.6 in SNAP.
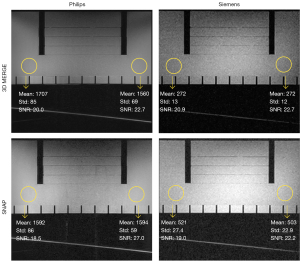
Qualitative analysis
The representative 3D MERGE and SNAP images obtained on Philips and Siemens are shown in Figures 2,3, respectively. There was no statistically significant difference (P>0.05) in the image quality scores of 3D MERGE and SNAP between Philips and Siemens scanners, except for 3D MERGE at the ECA region (P=0.01), indicating that 3D vessel wall MR images were generally comparable between the two vendors (shown in Figure 4). The Cohen’s kappa is 0.699 for 3D MERGE, and 0.677 for SNAP, both of which represent substantial agreement between two reviewers.
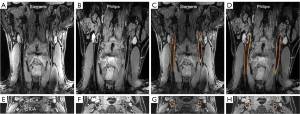
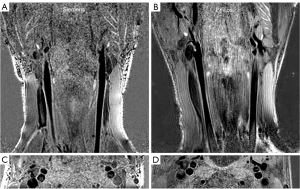
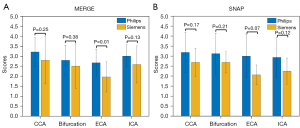
Quantitative analysis
The 3D LATTE framework successfully segmented the lumen and vessel wall from the 3D MERGE images, with manual corrections to fine-tune the segmentation (shown in Figure 2). The manual corrections were done in 7.1%±4.1% (mean ± standard deviation, averaged across all 6 volunteers) voxels of segmentation mask for Philips data and 12.1%±5.6% for Siemens data. This suggests that 3D LATTE has the potential to serve as a dedicated carotid vascular imaging tool across different MRI vendors.
The reproducibility test demonstrated that all the measured quantitative metrics exhibited good reproducibility (ICC >0.75) between the two MRI vendors, including mean wall thickness, maximum wall thickness, normalized wall index, and normalized lumen index (shown in Figure 5), with 1.96 standard deviations ranging from 15% to 22%. In CCA, all metrics have good reproducibility in the CCA (Figure S3); in bifurcation, mean wall thickness has good consistency and the other metrics have moderate consistency (ICC >0.5, Figure S4); in ICA, all metrics have moderate consistency (Figure S5); in ECA, normalized wall index and lumen index have moderate consistency, while mean wall thickness and maximum wall thickness have poor consistency (ICC <0.5, Figure S6).
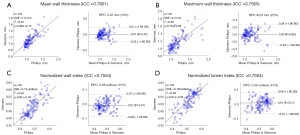
The cross-vendor percentage difference {i.e., (Philips − Siemens)/[(Philips + Siemens)/2]*100%} of quantitative metrics along the coordinate from CCA to ICA is shown in Figure 6, with the center being the bifurcation slice. In general, the average differences for all metrics are below 15% in all regions. Mean wall thickness and maximum wall thickness tend to have the highest difference around the bifurcation, likely due to the discrepancy in 3D thickness measurement caused by vessel complexity. Normalized wall index and lumen index tend to have highest difference in upper region of ICA, likely due to the smaller size of ICA compared with CCA. In addition, it is found that both vendors exhibit similar trend of metric distribution along the artery (Figure S7). Mean wall thickness and maximum wall thickness have the highest value around the bifurcation. The normalized wall index has the lowest value in the bifurcation and the highest value in the upper region of ICA, while normalized lumen index has the highest value in the bifurcation and the lowest value in the upper region of ICA.
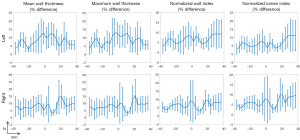
Plaque detection
The images acquired on the patient show that vessel wall and plaque structures are clearly visualized in 3D MERGE (Figure 7), and IPH on both left and right carotid arteries can be depicted by the hyperintensity signals in SNAP (Figure 7, red arrowheads). This is consistent with previous studies using Philips scanners.
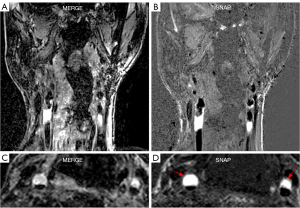
Discussion
While the superior performance of 3D vessel wall MRI has been shown for evaluating carotid atherosclerosis diseases, conventional 2D vessel wall MRI is still more widely used in clinical imaging scans. This is partly due to the lack of validated 3D vessel wall imaging sequences available on different MRI platforms. In this study, we implemented two representative 3D vessel wall MRI sequences on Siemens scanners, which originally were only available on Philips scanners, namely 3D MERGE, and SNAP. Then, we conducted a preliminary validation by comparing the qualitative image quality and quantitative morphological metrics acquired on the same six healthy volunteers with matched sequence parameters at two different vendors. Our results showed that both vendors can provide comparable image quality and consistent quantitative metrics on the same 6 subjects.
Because the noise characteristics of Siemens and Philips are different due to different receiver coils and post-processing methods, it is not feasible to compare the contrast-to-noise ratio (CNR) or signal-to-noise ratio (SNR) directly on Dicom images in humans. Instead, we did a qualitative comparison by letting two image reviewers rate the image quality using a 4-point rating system well established in this field (21). No significant differences were found when comparing the image quality scores of the two vendors except for 3D MERGE images in the ECA region, which are usually not considered a region of interest where pathology grows. However, the right carotid arteries of two volunteers exhibit very low image quality (average score <1.5) on Siemens data. In fact, we observed that, on average, 3D MERGE has a 15.7% lower image quality score, and SNAP has a 20.9% lower image quality score on Siemens compared to Philips. This was probably caused by higher signal intensities in regions close to the coil surface, either due to a sharper coil profile or due to suboptimal image nonuniformity correction at Siemens platform, which amplified the motion artifacts (Figure S8). Note that we have switched on the image uniformity correction techniques on both Siemens (“Prescan normalization”) and Philips (“Clear”) scanners. One way to mitigate this issue is to put water bags or towels to create space between the neck and the coil, and how to balance the SNR and image uniformity requires using this strategy requires further investigation. Despite this image quality mismatch, we demonstrated that the two sequences have sufficient image quality, allowing for reliable carotid atherosclerosis evaluation on both Siemens and Philips platforms (average image quality score >2.5), and they provide quantitative measurements with good consistency for the same volunteers.
The quantitative comparison was made by calculating the carotid vascular features, including mean wall thickness, maximum wall thickness, normalized wall index, and normalized lumen index, which have been widely used to evaluate the risk and progression of carotid atherosclerosis (29-31). Good reproducibility between Siemens and Philips data was found for all features when combining all regions of the artery. ECA region has poor reproducibility between Philips and Siemens scanners, probably due to the lower image quality in ECA on Siemens or the smaller artery size in ECA. The quantitative comparison was enabled by a novel 3D thickness measurement and bifurcation coordinate system (19), which can produce morphological measurements at different artery locations without requiring image registration between scans.
We recently proposed a semi-automatic carotid MR image processing tool, 3D LATTE (17,18), and we utilized it here to segment the lumen and vessel wall. Although 3D LATTE was trained only on Philips data, it showed robust performance in processing the Siemens data as well and significantly reduced the labor of manual segmentation. The 3D LATTE framework, combined with validated 3D carotid vessel wall MRI protocols, has the potential to serve as a dedicated carotid vascular imaging tool on different MRI vendors.
To the best of our knowledge, this is the first study to evaluate the 3D vessel wall MRI across two vendors on the same subjects. We implemented 3D MERGE and SNAP as they are two representative 3D vessel wall imaging sequences that provide complementary roles in evaluating carotid atherosclerosis (i.e., plaque burden evaluation and IPH detection). This study shows that the implementation of these 3D carotid MRI sequences is feasible with sufficient measurement capabilities. Given these findings, it is worth considering the evaluation of other 3D vessel wall sequences across different vendors in the future, including DANTE-Space (21), MATCH (22), and others.
One limitation of this study is that we have a limited number of participants (6 subjects), and only 1 female. Since these two scanners were in two different states, coordinating the travel of the same subjects from one institute to another was very challenging. To increase the statistical power, we included measurements in multiple locations in the carotid arteries when evaluating the consistency between quantitative measurements from two vendors. Since the quantitative measurements in different artery locations are equally important, it is meaningful to treat them as separate samples when evaluating the consistency. Moreover, we acquired images of one patient with carotid atherosclerosis, using the same protocol as for the healthy volunteers on a Siemens scanner. The images provided good visualization of vessel wall and plaque morphology, as well as detection of IPH. This is consistent with previous studies (9,11) that used the same protocols on Philips scanners. To fully validate the reproducibility of lesion detection and plaque component measurement on different vendors, the sequences should be validated by scanning the same patients across vendors. More healthy volunteers and patients will be included in a future long-term study.
Another limitation is that we only compared the sequences on Siemens and Philips platforms. While these results show that 3D MERGE and SNAP can be implemented successfully on a Siemens scanner, further efforts will be required to adapt these sequences for use on other vendors (GE, Canon, etc.).
Conclusions
In summary, through this pilot study, we did a double-vendor comparison study of 3D MERGE and SNAP, two representative carotid vessel wall MRI sequences on the same healthy volunteers for the first time. Qualitative and quantitative results show that the images acquired on Philips and Siemens have sufficient image quality, and they can provide consistent quantitative vessel morphology measurements, despite lower image quality in Siemens platforms, probably due to suboptimal implementation of coil or image processing. The findings of this study proved the possibility of establishing standardized 3D carotid vessel wall MRI protocols, paving the way for their future multicenter, multivendor research studies and clinical applications.
Acknowledgments
None.
Footnote
Reporting Checklist: The authors have completed the STROBE reporting checklist. Available at https://qims.amegroups.com/article/view/10.21037/qims-24-2124/rc
Funding: This study was supported by
Conflicts of Interest: All authors have completed the ICMJE uniform disclosure form (available at https://qims.amegroups.com/article/view/10.21037/qims-24-2124/coif). The authors have no conflicts of interest to declare.
Ethical Statement: The authors are accountable for all aspects of the work in ensuring that questions related to the accuracy or integrity of any part of the work are appropriately investigated and resolved. The study was conducted in accordance with the Declaration of Helsinki (as revised in 2013). The study was approved by the institutional review boards (IRB) of the University of Utah (IRB_00162281) and University of Washington (No. STUDY00007076), and written consent was obtained from each participant.
Open Access Statement: This is an Open Access article distributed in accordance with the Creative Commons Attribution-NonCommercial-NoDerivs 4.0 International License (CC BY-NC-ND 4.0), which permits the non-commercial replication and distribution of the article with the strict proviso that no changes or edits are made and the original work is properly cited (including links to both the formal publication through the relevant DOI and the license). See: https://creativecommons.org/licenses/by-nc-nd/4.0/.
References
- Martin SS, Aday AW, Almarzooq ZI, Anderson CAM, Arora P, Avery CL, et al. 2024 Heart Disease and Stroke Statistics: A Report of US and Global Data From the American Heart Association. Circulation 2024;149:e347-913. [Crossref] [PubMed]
- Cheng SF, Brown MM, Simister RJ, Richards T. Contemporary prevalence of carotid stenosis in patients presenting with ischaemic stroke. Br J Surg 2019;106:872-8. [Crossref] [PubMed]
- Yuan C, Mitsumori LM, Beach KW, Maravilla KR. Carotid atherosclerotic plaque: noninvasive MR characterization and identification of vulnerable lesions. Radiology 2001;221:285-99. [Crossref] [PubMed]
- Saam T, Hatsukami TS, Takaya N, Chu B, Underhill H, Kerwin WS, Cai J, Ferguson MS, Yuan C. The vulnerable, or high-risk, atherosclerotic plaque: noninvasive MR imaging for characterization and assessment. Radiology 2007;244:64-77.
- Kerwin WS, Hatsukami T, Yuan C, Zhao XQ. MRI of carotid atherosclerosis. AJR Am J Roentgenol 2013;200:W304-13. [Crossref] [PubMed]
- Saba L, Cau R, Murgia A, Nicolaides AN, Wintermark M, Castillo M, et al. Carotid Plaque-RADS: A Novel Stroke Risk Classification System. JACC Cardiovasc Imaging 2024;17:62-75. [Crossref] [PubMed]
- Canton G, Baylam Geleri D, Hippe DS, Sun J, Guo Y, Balu N, Chu B, Pimentel K, Akçiçek H, Yaman Akçiçek E, Tirschwell D, Tang G, Kohler T, Shibata D, Ferguson MS, Yuan C, Hatsukami TS. Pathophysiology of carotid atherosclerosis: Calcification, intraplaque haemorrhage and pulse pressure as key players. Eur J Radiol 2024;178:111647. [Crossref] [PubMed]
- Sun J, Zhao XQ, Balu N, Hippe DS, Hatsukami TS, Isquith DA, Yamada K, Neradilek MB, Cantón G, Xue Y, Fleg JL, Desvigne-Nickens P, Klimas MT, Padley RJ, Vassileva MT, Wyman BT, Yuan C. Carotid magnetic resonance imaging for monitoring atherosclerotic plaque progression: a multicenter reproducibility study. Int J Cardiovasc Imaging 2015;31:95-103. [Crossref] [PubMed]
- Balu N, Yarnykh VL, Chu B, Wang J, Hatsukami T, Yuan C. Carotid plaque assessment using fast 3D isotropic resolution black-blood MRI. Magn Reson Med 2011;65:627-37. [Crossref] [PubMed]
- Fan Z, Zhang Z, Chung YC, Weale P, Zuehlsdorff S, Carr J, Li D. Carotid arterial wall MRI at 3T using 3D variable-flip-angle turbo spin-echo (TSE) with flow-sensitive dephasing (FSD). J Magn Reson Imaging 2010;31:645-54. [Crossref] [PubMed]
- Wang J, Börnert P, Zhao H, Hippe DS, Zhao X, Balu N, Ferguson MS, Hatsukami TS, Xu J, Yuan C, Kerwin WS. Simultaneous noncontrast angiography and intraplaque hemorrhage (SNAP) imaging for carotid atherosclerotic disease evaluation. Magn Reson Med 2013;69:337-45. [Crossref] [PubMed]
- Li D, Zhao H, Chen X, Chen S, Qiao H, He L, Li R, Xu J, Yuan C, Zhao X. Identification of intraplaque haemorrhage in carotid artery by simultaneous non-contrast angiography and intraPlaque haemorrhage (SNAP) imaging: a magnetic resonance vessel wall imaging study. Eur Radiol 2018;28:1681-6. [Crossref] [PubMed]
- Murata K, Murata N, Chu B, Watase H, Hippe DS, Balu N, Sun J, Zhao X, Hatsukami TS, Yuan C. Characterization of Carotid Atherosclerotic Plaques Using 3-Dimensional MERGE Magnetic Resonance Imaging and Correlation With Stroke Risk Factors. Stroke 2020;51:475-80. [Crossref] [PubMed]
- Baylam Geleri D, Watase H, Chu B, Chen L, Zhao H, Zhao X, Hatsukami TS, Yuan C. Detection of Advanced Lesions of Atherosclerosis in Carotid Arteries Using 3-Dimensional Motion-Sensitized Driven-Equilibrium Prepared Rapid Gradient Echo (3D-MERGE) Magnetic Resonance Imaging as a Screening Tool. Stroke 2022;53:194-200. [Crossref] [PubMed]
- Chen S, Zhao H, Li J, Zhou Z, Li R, Balu N, Yuan C, Chen H, Zhao X. Evaluation of carotid atherosclerotic plaque surface characteristics utilizing simultaneous noncontrast angiography and intraplaque hemorrhage (SNAP) technique. J Magn Reson Imaging 2018;47:634-9. [Crossref] [PubMed]
- Chen L, Zhao H, Jiang H, Balu N, Geleri DB, Chu B, Watase H, Zhao X, Li R, Xu J, Hatsukami TS, Xu D, Hwang JN, Yuan C. Domain adaptive and fully automated carotid artery atherosclerotic lesion detection using an artificial intelligence approach (LATTE) on 3D MRI. Magn Reson Med 2021;86:1662-73. [Crossref] [PubMed]
- HashemizadehKolowri S, Nadin, Canton G, Balu N, Hatsukami T, Yuan C. Automated Localization of the Extracranial Carotid Artery in Black Blood Contrast MR Images Using a Deep Learning Approach. ISMRM & ISMRT Annual Meeting & Exhibition; 2023.
- HashemizadehKolowri S, Guo Y, Akcicek EY, Akcicek H, Ma X, Canton G, Balu N, Hatsukami T, Yuan C. Automated 3D Localization and Segmentation of Carotid Arteries in Black Blood Vessel Wall Imaging. 35th Annual International Conference of Society for MR Angiography; 2023.
- HashemizadehKolowri S. Akcicek EY, Akcicek H, Ma X, Ferguson MS, Balu N, Hatsukami TS, Yuan C. Efficient and Accurate 3D Thickness Measurement in Vessel Wall Imaging: Overcoming Limitations of 2D Approaches Using the Laplacian Method. J Cardiovasc Dev Dis 2024;11:249. [Crossref] [PubMed]
- Wang J, Yarnykh VL, Yuan C. Enhanced image quality in black-blood MRI using the improved motion-sensitized driven-equilibrium (iMSDE) sequence. J Magn Reson Imaging 2010;31:1256-63. [Crossref] [PubMed]
- Zhou Z, Li R, Zhao X, He L, Wang X, Wang J, Balu N, Yuan C. Evaluation of 3D multi-contrast joint intra- and extracranial vessel wall cardiovascular magnetic resonance. J Cardiovasc Magn Reson 2015;17:41. [Crossref] [PubMed]
- Beck MJ, Parker DL, Bolster BD Jr, Kim SE, McNally JS, Treiman GS, Hadley JR. Interchangeable neck shape-specific coils for a clinically realizable anterior neck phased array system. Magn Reson Med 2017;78:2460-8. [Crossref] [PubMed]
- Balu N, Yarnykh VL, Scholnick J, Chu B, Yuan C, Hayes C. Improvements in carotid plaque imaging using a new eight-element phased array coil at 3T. J Magn Reson Imaging 2009;30:1209-14. [Crossref] [PubMed]
- Koori N, Kamekawa H, Higuchi M, Fuse H, Miyakawa S, Yasue K, Kurata K. Influence of half Fourier and elliptical scanning (radial scan) on magnetic resonance images. J Magn Reson 2023;355:107560. [Crossref] [PubMed]
- Lu Y, Cao R, Jiao S, Li L, Liu C, Hu H, Ma Z, Jiang Y, Chen J. A novel method of carotid artery wall imaging: black-blood CT. Eur Radiol 2024;34:2407-15. [Crossref] [PubMed]
- Yushkevich PA, Piven J, Hazlett HC, Smith RG, Ho S, Gee JC, Gerig G. User-guided 3D active contour segmentation of anatomical structures: significantly improved efficiency and reliability. Neuroimage 2006;31:1116-28. [Crossref] [PubMed]
- Xiong Y, Zhang Z, He L, Ma Y, Han H, Zhao X, Guo H. Intracranial simultaneous noncontrast angiography and intraplaque hemorrhage (SNAP) MRA: Analyzation, optimization, and extension for dynamic MRA. Magn Reson Med 2019;82:1646-59. [Crossref] [PubMed]
- Klein R. Bland-Altman and Correlation Plot. Available online: https://www.mathworks.com/matlabcentral/fileexchange/45049-bland-altman-and-correlation-plot. MATLAB Central File Exchange. 2024.
- Underhill HR, Kerwin WS, Hatsukami TS, Yuan C. Automated measurement of mean wall thickness in the common carotid artery by MRI: a comparison to intima-media thickness by B-mode ultrasound. J Magn Reson Imaging 2006;24:379-87. [Crossref] [PubMed]
- Duivenvoorden R, de Groot E, Elsen BM, Laméris JS, van der Geest RJ, Stroes ES, Kastelein JJ, Nederveen AJ. In vivo quantification of carotid artery wall dimensions: 3.0-Tesla MRI versus B-mode ultrasound imaging. Circ Cardiovasc Imaging 2009;2:235-42. [Crossref] [PubMed]
- Cao X, Yang Q, Tang Y, Pan L, Lai M, Yu Z, Geng D, Zhang J. Normalized wall index, intraplaque hemorrhage and ulceration of carotid plaques correlate with the severity of ischemic stroke. Atherosclerosis 2020;315:138-44. [Crossref] [PubMed]