Deep learning network based on high-resolution magnetic resonance vessel wall imaging combined with attention mechanism for predicting stroke recurrence in patients with symptomatic intracranial atherosclerosis
Introduction
Stroke, a disease with high morbidity and mortality, poses a growing global healthcare burden due to aging populations (1). Intracranial atherosclerotic stenosis (ICAS) is prevalent worldwide, particularly in Asia, where it significantly elevates stroke recurrence risk (2). In Western countries, ICAS accounts for 10–15% of strokes, compared to 46.6% in Asia, highlighting regional differences. Therapeutic options for ICAS are limited, with extracranial-intracranial bypass surgery and intra-arterial stenting failing to achieve optimal results (3). Early and precise identification of high-risk ICAS patients, followed by timely pharmacological interventions, is crucial. Risk-stratified preventive treatment has reduced stroke recurrence over decades (4). Strengthening clinical research and optimizing patient management are essential for stroke prevention and outcome improvement (5).
Advancements in medical imaging, such as cranial computed tomography (CT), magnetic resonance imaging (MRI), magnetic resonance angiography (MRA), and computed tomography angiography (CTA), have improved stroke diagnosis by depicting lesions and assessing vessel localization and collateral circulation. Perfusion imaging techniques such as computed tomography perfusion (CTP) and perfusion-weighted imaging (PWI), enhance diagnostic efficiency by evaluating infarct cores and ischemic penumbra (6). However, current technologies have limitations in assessing critical plaques. High-resolution magnetic resonance vessel wall imaging (HR-VWI) has emerged as a vital tool for symptomatic ICAS, offering clear visualization of vessel walls and plaque characteristics, which are linked to stroke progression and prognosis (7,8). HR-VWI enables accurate identification of vulnerable plaques and high-risk patients, facilitating tailored treatment plans and improved outcomes (9,10).
The rapid development of artificial intelligence (AI), particularly deep learning (DL), brings new opportunities to medicine. DL automates feature extraction and pattern recognition from complex medical data through multi-layered neural networks (11). Among the various DL architectures, the Transformer model, with its self-attention mechanism, has emerged as a powerful tool for capturing long-range dependencies and global contextual information in data. This self-attention mechanism allows the model to dynamically adjust its focus, enhancing its ability to process large-scale images and integrate global information, which is particularly advantageous in medical imaging tasks. AI-assisted diagnostic systems based on DL are crucial, alleviating physician workload, enhancing diagnostic and treatment efficiency, and providing patients with more precise therapeutic strategies. Therefore, the present study aimed to leverage HR-VWI images in conjunction with DL, incorporating an attention mechanism to develop a classification network for predicting stroke recurrence in patients with symptomatic intracranial atherosclerotic stenosis (sICAS). Furthermore, the performance of this approach was evaluated by comparing it with traditional radiomics and plaque models. We present this article in accordance with the TRIPOD + AI reporting checklist (available at https://qims.amegroups.com/article/view/10.21037/qims-24-1723/rc).
Methods
Eligibility criteria and patients
A retrospective cohort of 363 patients from two centers was recruited, with 254 patients from Center 1 (The First Affiliated Hospital of Xinxiang Medical University), serving as the training set, and 109 patients from Center 2 (The Sixth People’s Hospital of Shanghai Jiao Tong University), constituting the test set. This study was conducted in accordance with the Declaration of Helsinki (as revised in 2013). This study was approved by the Ethics Committees of The First Affiliated Hospital of Xinxiang Medical University (No. EC-022-142) and The Sixth People’s Hospital of Shanghai Jiao Tong University (No. 2023-217). The requirement for individual consent was waived due to the retrospective nature of the analysis.
This retrospective study enrolled sICAS patients from two hospitals between January 2020 and December 2022 who underwent HR-VWI examination within one week after diagnosis. The inclusion criteria were as follows: (I) sICAS patients: defined according to the American Stroke Association guidelines as ICAS (12), with a stenosis rate of 50–99% that resulted in transient ischemic attack (TIA) or ischemic stroke. (II) HR-VWI examination performed within one week of sICAS diagnosis. (III) Age >18 years. (IV) Receiving conventional medical therapy without endovascular intervention. The exclusion criteria were as follows: (I) carotid stenosis >50% on imaging. (II) Other vascular diseases such as dissection or vasculitis. (III) Evidence of cardiac embolism: high-risk cardioembolic sources identified by transthoracic echocardiography (TTE) and/or transesophageal echocardiography (TEE), including plaques in the ascending aorta and aortic arch (thickness ≥4 mm), or the presence of right-to-left shunt detected by TEE contrast echocardiography, TTE contrast echocardiography, or contrast-enhanced transcranial Doppler ultrasound. (IV) Due to the small lesion volume of perforating artery and the poor consistency in regions of interest (ROIs) delineation, patients with perforating artery stenosis were excluded. (V) Poor image quality that impairs plaque evaluation or MRI contraindications. (VI) Missing follow-up information. Please refer to the Appendix 1 for specific inclusion and exclusion criteria.
Stroke recurrence was diagnosed by two neurologists with over 10 years of clinical experience. This study employed face-to-face follow-up (for stroke recurrence) and telephone follow-up (for non-recurrence), with a follow-up duration ranging from 18 to 24 months and a median follow-up time of 20 months. The diagnostic criteria for stroke recurrence included (13): (I) sudden onset of new focal neurological dysfunction lasting for more than 24 hours. (II) Focal neurological dysfunction lasting for less than 24 hours but confirmed as acute cerebral infarction by imaging. (III) Sudden neurological deterioration with an increase of ≥4 points on the National Institutes of Health Stroke Scale (NIHSS) score (14). The typical MRI images of recurrent and non-recurrent patients are shown in Figure 1.
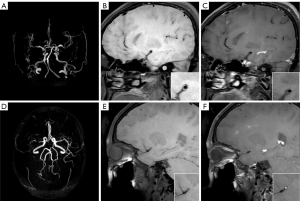
HR-VWI imaging method and image preprocessing
The study encompassed two centers, and all patients undergoing HR-VWI were scanned using either a Philips Ingenia Elition 3.0T (Philips Healthcare, Amsterdam, The Netherlands) or a Siemens MAGNETOM Vida MRI machine (Siemens, Erlangen, Germany). Detailed machine parameters are provided in the Tables S1,S2. To account for the impact of different devices, the collected images underwent the following standardized processing: (I) N4 bias field correction using the “SimpleITK” software package was applied to all images. (II) To achieve isotropic voxels, all images were resampled to a uniform voxel size of 0.75×0.75×0.75 mm3 using cubic b-spline interpolation. (III) To reduce inter-scanner differences in image acquisition, images were normalized to a final gray-scale intensity range of 0 to 600.
Definition and selection of culprit plaque
The construction of all radiomics and DL models is based on the specific “culprit plaque” identified for each patient enrolled in the study. The “culprit plaque” is rigorously defined as the atherosclerotic plaque located within the responsible vessel that directly corresponds to the neurological deficit symptoms, and is ipsilateral to the newly developed stroke lesion clearly visible on diffusion-weighted imaging (DWI) (15). In cases where multiple plaques are detected within the same vascular territory, the lesion causing the most significant stenosis, based on its impact on lumen narrowing, is selected for analysis.
Construction of radiomics model and plaque model
- ROI delineation: the ROI is outlined based on the T1-weighted enhanced sequence of HR-VWI, as this sequence enhances the contrast between the culprit plaque and surrounding normal vascular tissue, significantly facilitating the accuracy of three-dimensional (3D) volumes of interest (3D-VOIs) segmentation. The plaque boundaries are meticulously traced slice-by-slice, strictly excluding interference from normal vascular tissue to ensure the purity of the analysis region. This task is independently performed by two senior neuroradiology experts and reviewed by a physician with 10 years of experience in image diagnosis, utilizing ITK-SNAP 3.6.0 software (http://www.itksnap.org/pmwiki/pmwiki.php) to ensure consistency and accuracy.
- Radiomics feature extraction: utilizing the PyRadiomics 3.0.1 package (https://pypi.org/project/pyradiomics/) within the Python 3.6.7 environment (https://www.python.org/downloads/), a comprehensive set of radiomics features, including first-order statistics and various high-order texture features, are systematically extracted from the imaging data. These features encompass gray level size zone matrix (GLSZM), gray level co-occurrence matrix (GLCM), neighborhood gray tone difference matrix (NGTDM), gray level dependence matrix (GLDM), and gray level run length matrix (GLRLM).
- Radiomics feature selection and model construction: initially, all extracted features undergo z-score normalization to eliminate dimensional differences. Then, a feature selection process is implemented: (I) using the Mann-Whitney U test, statistically significant features (P<0.05) are identified, excluding irrelevant or redundant information. (II) The Pearson correlation coefficient is calculated to remove highly correlated features (r>0.95), reducing multicollinearity among features. (III) Least absolute shrinkage and selection operator (LASSO) combined with 10-fold cross-validation is applied to further optimize the feature set, ultimately determining the critical subset of features for model construction. This process ensures the predictive power, stability, and generalization ability of the model. Subsequently, we incorporated the selected features into the logistic regression machine learning model and evaluated the model performance on the training and test sets separately.
- Plaque model construction: in this study, a predictive model named “Plaque Model” is constructed, which integrates a series of qualitative and quantitative feature parameters within the culprit plaque that are closely related to stroke prognosis. These core parameters include the presence of intraplaque hemorrhage (IPH), enhancement grade, stenosis rate, normalized wall index (NWI), positive remodeling, and maximum wall thickness (MWT). To ensure assessment accuracy and reliability, two experienced senior neuroradiologists independently and meticulously evaluated and precisely calculated these plaque parameters following a standardized operational protocol (detailed in the Appendix 1). For quantitative data, the average of the two physicians’ results was adopted as the final data to minimize individual bias. For qualitative assessments, if there was a disagreement, both physicians were required to jointly review the imaging data and reach a consensus through thorough discussion, thereby ensuring the objectivity and consistency of the assessment results.
Network details
We devised an advanced architecture grounded on convolutional neural networks (CNNs), specifically utilizing ResNet50 and DenseNet169 as two distinct feature extraction networks, both trained on enhanced T1-weighted images from HR-VWI, which were independently trained in Center 1 (training set) and subsequently validated in Center 2 (test set). We visualized the last convolutional layer of two DL networks using Gradient weighted Class Activation Mapping (Grad-CAM), and found that the attention of the convolutional network was still somewhat insufficient (Figure S1). To further bolster the predictive prowess of our model, we integrated the attention mechanism from Transformers, enabling a profound fusion of the output features from both CNN models. We refer to this advanced integrated model as Transformer-convolutional neural network (Trans-CNN). This fusion aimed at precisely forecasting the risk of stroke recurrence in patients with sICAS (16). Figure 2 illustrates the schematic diagram of this hybrid network architecture. In the HR-VWI image processing stage, ResNet50 and DenseNet169 were carefully tuned into efficient feature encoders, removing their end classification structures and extracting rich and representative deep features in the final global average pooling layer. Subsequently, in order to reduce redundant information and optimize computational efficiency in the future, we will integrate principal component analysis (PCA) behind the global average pooling layer to output compressed important features. During the HR-VWI image processing phase, ResNet50 and DenseNet169 were meticulously adapted as efficient feature encoders by removing their top-level classification structures and retaining only the intermediate layers for feature representation, thereby extracting rich and representative deep features. The reduced features were concatenated along the channel dimension, forming a feature matrix encompassing multivariate information. To further enhance the efficacy of feature representation, we introduced a Transformer-based attention mechanism, as shown in Figure 2D. At its core, this mechanism divides the feature matrix into several sub-blocks and employs a multi-head self-attention mechanism to deeply interact and integrate information within these sub-blocks, yielding contextually enriched augmented feature maps. Then, the processed feature map was input into the classifier to output the model’s judgment results. The processed feature maps were then fed into a classifier. Figure 2B,2C depict the structural details of these two CNN models. Subsequently, PCA was applied to the features extracted from both CNN models for dimensionality reduction, mitigating redundant information and optimizing computational efficiency. The reduced features were concatenated along the channel dimension, forming a feature matrix encompassing multivariate information. To further enhance the efficacy of feature representation, we introduced a Transformer-based attention mechanism, as shown in Figure 2D. At its core, this mechanism divides the feature matrix into several sub-blocks and employs a multi-head self-attention mechanism to deeply interact and integrate information within these sub-blocks, yielding contextually enriched augmented feature maps. The processed feature maps were then fed into a classifier. Specifically, following a pooling layer, a multilayer perceptron (MLP) was utilized to decode the fused information, predicting the stroke recurrence status in sICAS patients. The relevant parameters for DL training are as follows: the network is implemented using Pytorch with a stochastic gradient descent (SGD) optimizer. The batch size is set to 2, the number of training epochs is 100, and the initial learning rate for the units is 0.001. The network has been implemented on an NVIDIA GeForce RTX 4060 Graphics Processing Unit (GPU) (Nvidia, Santa Clara, CA, USA).
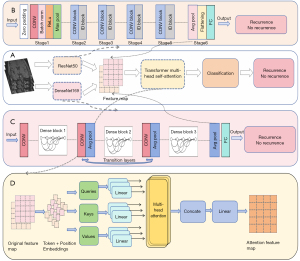
Performance and validation of the prediction model
The model’s performance was evaluated using the area under the curve (AUC) of the receiver operating characteristic (ROC) curve, sensitivity, and specificity (the calculation method of relevant indicators can be found in the Appendix 1). The DeLong test was applied to assess differences in ROC curves among various models. Finally, the decision curve analysis (DCA) curve was employed to evaluate the clinical decision-making value of the model. In order to verify the improvement of processing efficiency of DL models, we randomly selected images of 50 patients (30 in the training set and 20 in the test set), and used Trans-CNN model models and a team of radiologists to re-evaluate and record the time consumed by each patient. The team of radiologists consists of three experienced radiologists, and each doctor makes independent judgments and finally calculates the average of the three.
Statistical analysis
Normally distributed quantitative data were presented as mean ± standard deviation and comparisons between two groups were made using independent t-test. Non-normally distributed quantitative data were presented as median (interquartile range) [M (P25, P75)], and comparisons between two groups were made using the Mann-Whitney U test. Count data were presented as numbers and percentages, and comparisons between two groups were made using the χ2 test or Fisher’s exact test. P<0.05 was considered statistically significant.
Results
Basic information and plaque characteristics
The baseline data on plaque characteristics are presented in Table 1. Center 1 (training set) comprised a total of 254 patients, with 50 (19.7%) experiencing stroke recurrence and 204 (80.3%) remaining non-recurrent. Center 2 (test set) included 109 patients, among which 25 (22.9%) had stroke recurrence, and 84 (77.1%) did not. In the training set, statistically significant differences were observed between the recurrent and non-recurrent groups in terms of IPH (P=0.012) and MWT (P=0.001). For the test set, statistically significant differences were noted in the degree of stenosis (P=0.005), IPH (P=0.006), and enhancement level (P=0.009). For the baseline characteristics, in the test set, there was a statistically significant difference in whether patients with recurrent stroke and those without recurrence underwent lipid-lowering medications (P=0.005).
Table 1
Variables | Training set (n=254) | Test set (n=109) | |||||
---|---|---|---|---|---|---|---|
No recurrence (n=204) | Recurrence (n=50) |
P value | No recurrence (n=84) |
Recurrence (n=25) |
P value | ||
Male | 63 (30.9) | 19 (38.0) | 0.335 | 28 (33.3) | 7 (28.0) | 0.616 | |
Age (years) | 58.0 [51.0, 66.0] | 58.0 [50.0, 66.0] | 0.831 | 58.0 [51.0, 67.0] | 58.0 [47.0, 63.0] | 0.403 | |
BMI (kg/m2) | 24.6 [22.7, 26.8] | 24.8 [23.0, 28.1] | 0.296 | 25.0 [22.7, 27.6] | 25.4 [23.5, 28.3] | 0.652 | |
Smoking history | 85 (41.7) | 26 (52.0) | 0.187 | 31 (36.9) | 12 (48.0) | 0.319 | |
Drinking history | 57 (27.9) | 14 (28.0) | 0.993 | 33 (39.3) | 8 (32.0) | 0.509 | |
Hypertension | 113 (55.4) | 30 (60.0) | 0.556 | 57 (67.9) | 16 (64.0) | 0.719 | |
Diabetes | 51 (25.0) | 14 (28.0) | 0.663 | 27 (32.1) | 6 (24.0) | 0.437 | |
Stroke or TIA history | 86 (42.2) | 22 (44.0) | 0.813 | 30 (35.7) | 12 (48.0) | 0.268 | |
History of using lipid-lowering medications | 108 (52.9) | 20 (40.0) | 0.101 | 40 (47.6) | 4 (16.0) | 0.005 | |
Homocysteine | 16.6 [12.6, 21.9] | 15.2 [11.5, 20.3] | 0.274 | 16.5 [12.6, 21.6] | 18.9 [12.3, 20.7] | 0.857 | |
NIHSS | 1.0 [0.0, 3.0] | 1.0 [0.0, 3.0] | 0.767 | 1.0 [0.0, 3.0] | 1.0 [0.0, 4.0] | 0.930 | |
TC | 4.0 [3.3, 4.6] | 3.9 [3.2, 4.7] | 0.459 | 4.0 [3.4, 4.8] | 3.7 [3.2, 4.3] | 0.584 | |
TG | 1.2 [0.9, 1.8] | 1.1 [0.9, 1.7] | 0.998 | 1.3 [0.9, 1.7] | 1.4 [0.9, 1.6] | 0.843 | |
LDL | 2.3 [1.8, 2.8] | 2.3 [1.7, 2.9] | 0.481 | 2.3 [1.8, 2.8] | 2.2 [1.7, 2.9] | 0.977 | |
HDL | 1.1 [0.9, 1.3] | 1.1 [0.9, 1.3] | 0.920 | 1.1 [0.9, 1.2] | 1.0 [0.9, 1.2] | 0.179 | |
ApoA | 1.1 [1.0, 1.2] | 1.1 [1.0, 1.3] | 0.697 | 1.1 [1.0, 1.3] | 1.0 [1.0, 1.2] | 0.219 | |
ApoB | 0.8 [0.7, 1.0] | 0.8 [0.6, 1.0] | 0.579 | 0.9 [0.7, 1.0] | 0.9 [0.7, 0.9] | 0.727 | |
Degree of stenosis | 0.6 [0.4, 0.8] | 0.7 [0.4, 0.8] | 0.175 | 0.5 [0.4, 0.7] | 0.7 [0.6, 0.8] | 0.005 | |
NWI | 81.7 [71.9, 87.9] | 83.0 [71.7, 87.5] | 0.652 | 79.9 [73.0, 86.6] | 84.2 [79.0, 86.3] | 0.143 | |
IPH | 0.012 | 0.006 | |||||
No | 144 (70.6) | 26 (52.0) | 56 (66.7) | 9 (36.0) | |||
Yes | 60 (29.4) | 24 (48.0) | 28 (33.3) | 16 (64.0) | |||
Enhancement level | 0.124 | 0.009 | |||||
0 | 45 (22.1) | 5 (10.0) | 20 (23.8) | 1 (4.0) | |||
1 | 76 (37.3) | 19 (38.0) | 34 (40.5) | 7 (28.0) | |||
2 | 83 (40.7) | 26 (52.0) | 30 (35.7) | 17 (68.0) | |||
Positive remodeling | 0.067 | 0.112 | |||||
No | 123 (60.3) | 23 (46.0) | 52 (61.9) | 11 (44.0) | |||
Yes | 81 (39.7) | 27 (54.0) | 32 (38.1) | 14 (56.0) | |||
MWT | 1.4 [1.2, 1.7] | 1.7 [1.4, 1.9] | 0.001 | 1.4 [1.3, 1.8] | 1.6 [1.4, 1.8] | 0.296 |
Continuous variables are presented as median [interquartile range] or mean ± standard deviation; categorical variables are presented as number (%). History of using lipid-lowering medications, regular consumption of lipid-lowering drugs. ApoA, apolipoprotein A; ApoB, apolipoprotein B; BMI, body mass index; HDL, high-density lipoprotein; IPH, intraplaque hemorrhage; LDL, low-density lipoprotein; MWT, maximum wall thickness; NIHSS, National Institute of Health Stroke Scale; NWI, normal wall index; TC, total cholesterol; TG, triglyceride.
Predictive performance of the radiomics model and plaque model
All collected plaque parameters were incorporated into the plaque model, which achieved an AUC of 0.715 [95% confidence interval (CI): 0.629–0.797] with a sensitivity of 0.560 (95% CI: 0.402–0.932) and a specificity of 0.794 (95% CI: 0.377–0.921) in the training set. In the test set, the plaque model yielded an AUC of 0.771 (95% CI: 0.659–0.868), a sensitivity of 0.840 (95% CI: 0.646–0.967), and a specificity of 0.667 (95% CI: 0.554–0.825) (Table 2).
Table 2
Model | AUC (95% CI) | Sensitivity (95% CI) | Specificity (95% CI) | Accuracy (95% CI) |
---|---|---|---|---|
Training set | ||||
Trans-CNN | 0.951 (0.923–0.974) | 0.900 (0.836–1.000) | 0.882 (0.757–0.948) | 0.880 (0.797–0.937) |
Radiomics | 0.773 (0.698–0.836) | 0.860 (0.725–0.953) | 0.642 (0.547–0.758) | 0.698 (0.610–0.770) |
Densenet169 | 0.856 (0.777–0.921) | 0.700 (0.588–0.875) | 0.917 (0.760–0.975) | 0.865 (0.770–0.923) |
Resnet50 | 0.849 (0.764–0.917) | 0.760 (0.656–0.913) | 0.887 (0.697–0.943) | 0.860 (0.742–0.912) |
PlaqueModel | 0.715 (0.629–0.797) | 0.560 (0.402–0.932) | 0.794 (0.377–0.921) | 0.804 (0.752–0.846) |
Test set | ||||
Trans-CNN | 0.912 (0.839–0.969) | 0.880 (0.693–1.000) | 0.810 (0.690–0.976) | 0.858 (0.743–0.936) |
Radiomics | 0.698 (0.569–0.808) | 0.520 (0.410–0.981) | 0.845 (0.390–0.924) | 0.721 (0.500–0.853) |
Densenet169 | 0.780 (0.634–0.919) | 0.680 (0.550–0.938) | 0.917 (0.741–0.972) | 0.845 (0.752–0.927) |
Resnet50 | 0.780 (0.628–0.898) | 0.760 (0.561–0.905) | 0.869 (0.775–0.964) | 0.850 (0.761–0.917) |
PlaqueModel | 0.771 (0.659–0.868) | 0.840 (0.646–0.967) | 0.667 (0.554–0.825) | 0.767 (0.692–0.835) |
AUC, area under the curve; CI, confidence interval; CNN, convolutional neural network; sICAS, symptomatic intracranial atherosclerotic stenosis.
The radiomics model initially extracted 2,038 features. Following a rigorous feature selection process, including correlation coefficient filtering, U-test, Pearson coefficient elimination, and final LASSO regression (Figure 3A,3B), 33 features were incorporated into the radiomics model. In the training set (for detailed radiomic feature parameters, please refer to the Table S3), this model achieved an AUC of 0.773 (95% CI: 0.698–0.836), an accuracy of 0.698 (95% CI: 0.610–0.770), a sensitivity of 0.560 (95% CI: 0.402–0.932), and a specificity of 0.794 (95% CI: 0.377–0.921). In the test set, the radiomics model showed an AUC of 0.698 (95% CI: 0.569–0.808), an accuracy of 0.721 (95% CI: 0.500–0.853), a sensitivity of 0.520 (95% CI: 0.410–0.981), and a specificity of 0.845 (95% CI: 0.390–0.924) (Figure 4). In summary, neither the plaque model nor the radiomics model demonstrated exceptionally high performance in both the training and test sets, particularly with regard to sensitivity, indicating room for improvement.
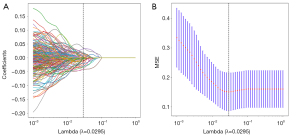
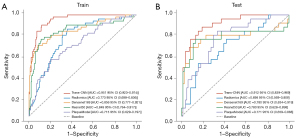
Predictive performance of the DL model and Trans-CNN model
This study employed two DL models, ResNet50 and DenseNet169, and utilized PCA to reduce the dimensionality of the DL features to a common level. Figure S2A,S2B illustrate the feature selection process during dimensionality reduction for both DL models, resulting in the selection of 27 features for DenseNet169 and 18 features for ResNet50. Both ResNet50 and DenseNet169 demonstrated satisfactory model performance in the training set, as detailed in Table 2 and Figure 4. In the test set, they achieved AUCs of 0.780 (95% CI: 0.628–0.898) and 0.780 (95% CI: 0.634–0.919), with accuracies of 0.850 (95% CI: 0.761–0.917) and 0.845 (95% CI: 0.752–0.927), respectively. The sensitivities were 0.760 (95% CI: 0.561–0.905) and 0.680 (95% CI: 0.550–0.938), whereas the specificities were 0.869 (95% CI: 0.775–0.964) and 0.917 (95% CI: 0.741–0.972), respectively. Both in the training and test sets, the DL models outperformed the radiomics model and the plaque model (DeLong test, P<0.05) (Tables 3,4).
Table 3
Model | Trans-CNN | Radiomics | Densenet169 | Resnet50 | PlaqueModel |
---|---|---|---|---|---|
Trans-CNN | – | 0.0 | 0.003 | 0.001 | 0.0 |
Radiomics | 0.0 | – | 0.04 | 0.05 | 0.248 |
Densenet169 | 0.003 | 0.04 | – | 0.837 | 0.006 |
Resnet50 | 0.001 | 0.05 | 0.837 | – | 0.013 |
PlaqueModel | 0.0 | 0.248 | 0.006 | 0.013 | – |
CNN, convolutional neural network.
Table 4
Name | Trans-CNN | Radiomics | Densenet169 | Resnet50 | PlaqueModel |
---|---|---|---|---|---|
Trans-CNN | – | 0.0 | 0.025 | 0.02 | 0.03 |
Radiomics | 0.0 | – | 0.288 | 0.258 | 0.371 |
Densenet169 | 0.025 | 0.288 | – | 0.988 | 0.926 |
Resnet50 | 0.02 | 0.258 | 0.988 | – | 0.93 |
PlaqueModel | 0.03 | 0.371 | 0.926 | 0.93 | – |
CNN, convolutional neural network.
The Trans-CNN model exhibited remarkable performance, integrating two CNN architectures with the Transformer attention mechanism into an advanced ensemble model. In both the training and test sets, the Trans-CNN model significantly surpassed the other four comparative models. Specifically, in the test set, it achieved an AUC of 0.912 (95% CI: 0.839–0.969), an accuracy of 0.858 (95% CI: 0.743–0.936), a sensitivity of 0.880 (95% CI: 0.693–1.000), and a specificity of 0.810 (95% CI: 0.690–0.976). Statistical analysis (DeLong test) confirmed that the Trans-CNN model demonstrated statistically significant differences in AUC compared to all other models in both the training and test sets (P<0.05) (Tables 3,4). Calibration curve analysis revealed good agreement between the predicted probabilities and observed outcomes for the Trans-CNN model in both sets, indicating high reliability of the model predictions. Furthermore, clinical DCA underscored the potential value of the Trans-CNN model in guiding clinical decision-making, as its curve characteristics suggested that the model provides useful information across different thresholds, facilitating more rational and scientific decision-making by clinicians (Figure 5). The evaluation time of the Trans-CNN model for each plaque was significantly shorter than that of the team of radiologists (P<0.001), as shown in the Table S4 and Figure S3.
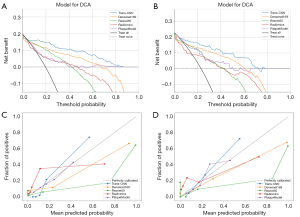
Discussion
The findings of this study demonstrate that the Trans-CNN model, constructed using HR-VWI to characterize culprit plaques, exhibits exceptional predictive capability in assessing the risk of recurrent stroke among patients with sICAS. This model innovatively incorporates the attention mechanism of the Transformer, enabling deep integration of output features from two CNN models. This strategy effectively enhances model performance, surpassing single radiomics models, plaque models, and traditional DL models in predictive accuracy.
sICAS-induced stroke is often accompanied by a high recurrence rate (17), which significantly elevates the risk of disability and mortality, thereby exacerbating the burden on the healthcare system. Although much research has focused on the degree of stenosis in the responsible vessels, real-world data reveal that patients with stenosis ≥70% have approximately twice the recurrence risk compared to those with stenosis <70% (18). However, with advancements in HR-VWI technology, stroke prognosis assessment has become increasingly precise, with culprit plaque characteristics emerging as a research hotspot. Studies have shown that plaque properties and quantitative parameters are closely related to stroke recurrence. Zhang et al. (10) found a significant correlation between plaque enhancement changes and recurrence (r=0.415, P<0.05), with multivariate regression indicating plaque enhancement as an independent risk factor [odds ratio (OR) =5.797; P<0.05]. Sun et al. (19) emphasized the importance of plaque burden, which was significantly higher in recurrent stroke patients compared to non-recurrent patients (P<0.05). Furthermore, IPH, a critical plaque characteristic, was confirmed by Yuan et al. (20) through Cox regression analysis to be significantly associated with ipsilateral stroke recurrence (OR =6.64; 95% CI: 1.23–35.8; P=0.028). Nevertheless, the plaque model based on qualitative and quantitative plaque data performed poorly in this study, with an AUC of 0.715 (95% CI: 0.629–0.797) and a sensitivity of 0.560 (95% CI: 0.402–0.932) in the training set. This low sensitivity limits the identification rate of positive patients, thereby diminishing its clinical value. This issue is attributed to the subjectivity of plaque assessment, which, even when performed by two experienced neuroradiologists, cannot fully eliminate inter-observer variability. Therefore, the integration of cutting-edge imaging techniques with innovative image analysis methods is crucial for individualized and precise assessment of culprit plaques.
Radiomics models represent an advanced analytical framework based on medical imaging, utilizing image processing and machine learning techniques to automatically extract a vast array of quantitative features from preprocessed images, encompassing shape, texture, intensity, wavelet transform coefficients, and more. These models aim to capture subtle image differences that are imperceptible to the naked eye, thereby compensating for the limitations of subjective interpretation (21,22). Tang et al. (23) pioneered the application of radiomics in analyzing intracranial culprit plaques in sICAS, with a model constructed based on 3D-T1-weighted imaging (T1WI)-Volume Isotropic Turbo Spin Echo Acquisition (VISTA)-enhanced images achieving AUCs of 0.790 (95% CI: 0.669–0.894) and 0.779 (95% CI: 0.620–0.853) in the training and test sets, respectively.
This study also constructed a radiomics model based on T1-weighted-enhanced images, revealing AUCs of 0.773 (95% CI: 0.698–0.836) and 0.698 (95% CI: 0.569–0.808) in the two evaluation groups, respectively, which were slightly inferior to Tang et al.’s findings and accompanied by lower sensitivities of 0.560 (95% CI: 0.402–0.932) and 0.520 (95% CI: 0.410–0.981). We speculate that the limited generalization ability of manually delineating 3D-VOIs may be one contributing factor. Additionally, despite image preprocessing, the reproducibility of radiomics features across different imaging protocols and devices cannot be overlooked (24).
DL, as a pivotal branch of machine learning, mimics the neural network mechanisms of the human brain, enabling automatic feature learning and extraction from vast amounts of data for complex pattern recognition and prediction. In medical image processing, DL reduces manual intervention, enhances diagnostic efficiency and accuracy, and demonstrates robust generalization capabilities, addressing images from diverse sources and qualities, thereby compensating for the limitations of manual assessment and radiomics techniques (25,26). Currently, DL in plaque research has primarily focused on the internal carotid artery. For instance, Fu et al. (27) demonstrated that a DL model based on head-neck CTA accurately classifies and predicts the severity of internal carotid artery plaques, achieving high agreement with radiologist diagnoses (κ =0.856; 95% CI: 0.832–0.886).
In this study, two CNN models, ResNet50 and DenseNet169, were employed to analyze plaques and predict stroke recurrence. Both models performed well, with AUCs of 0.780 in the test set (ResNet50: 95% CI: 0.628–0.898; DenseNet169: 95% CI: 0.634–0.919), and improved sensitivities (ResNet50: 0.760, 95% CI: 0.561–0.905; DenseNet169: 0.680, 95% CI: 0.550–0.938). However, the DeLong test did not reveal statistically significant differences compared to the radiomics and plaque models, suggesting limited gains. This may be attributed to CNNs’ difficulty in fully comprehending the hierarchical structure of feature spaces in complex scenarios. To overcome this limitation, our study innovatively fused the feature extraction capabilities of CNNs with the attention mechanism of the Transformer, resulting in the Trans-CNN model.
The Trans-CNN model exhibited exceptional performance in the test set, with an AUC of 0.912 (95% CI: 0.839–0.969), an accuracy of 0.858 (95% CI: 0.743–0.936), a sensitivity-boosted to 0.880 (95% CI: 0.693–1.000), and a specificity of 0.810 (95% CI: 0.690–0.976). The DeLong test confirmed the statistical significance of this AUC improvement. This remarkable leap in performance is attributed to the introduction of the attention mechanism, which mimics human selective attention behavior, dynamically adjusting data focus, and effectively capturing long-range dependencies and complex structures through global correlation modeling of sequence elements. This is particularly advantageous in processing large-scale images and integrating global information (28,29).
Despite adopting a multicenter design, this study faces several critical limitations: Firstly, the overall sample size is relatively limited, necessitating future prospective, larger-scale, multicenter data collection to validate the classifier’s generalizability in a broader population. Secondly, to enhance predictive accuracy, there is an urgent need to integrate multi-modal imaging data for comprehensive analysis. Thirdly, although standardized preprocessing of imaging data was performed, inconsistencies in imaging equipment, acquisition parameters, and post-processing workstations among different centers may affect the comparability of results, thus requiring further evaluation of classifier performance in an independent test set.
Conclusions
In summary, this study successfully constructed two CNN DL models based on HR-VWI of culprit plaques—ResNet50 and DenseNet169—both of which effectively predict the risk of stroke recurrence in patients with sICAS. Furthermore, when these CNN models serve as feature extractors and are combined with the attention mechanism of Transformer to form the advanced Trans-CNN model, even superior performance is demonstrated. This classifier holds promise as an essential tool for aiding in individualized diagnosis and timely clinical intervention for sICAS patients, with vast potential applications.
Acknowledgments
None.
Footnote
Reporting Checklist: The authors have completed the TRIPOD + AI reporting checklist. Available at https://qims.amegroups.com/article/view/10.21037/qims-24-1723/rc
Funding: This study was supported by
Conflicts of Interest: All authors have completed the ICMJE uniform disclosure form (available at https://qims.amegroups.com/article/view/10.21037/qims-24-1723/coif). The authors have no conflicts of interest to declare.
Ethical Statement: The authors are accountable for all aspects of the work in ensuring that questions related to the accuracy or integrity of any part of the work are appropriately investigated and resolved. This study was conducted in accordance with the Declaration of Helsinki (as revised in 2013). This study was approved by Ethics Committees of The First Affiliated Hospital of Xinxiang Medical University (No. EC-022-142) and The Sixth People’s Hospital of Shanghai Jiao Tong University (No. 2023-217). The requirement for individual consent was waived due to the retrospective nature of the analysis.
Open Access Statement: This is an Open Access article distributed in accordance with the Creative Commons Attribution-NonCommercial-NoDerivs 4.0 International License (CC BY-NC-ND 4.0), which permits the non-commercial replication and distribution of the article with the strict proviso that no changes or edits are made and the original work is properly cited (including links to both the formal publication through the relevant DOI and the license). See: https://creativecommons.org/licenses/by-nc-nd/4.0/.
References
- Hou C, Lan J, Lin Y, Song H, Wang Y, Zhao W, et al. Chronic remote ischaemic conditioning in patients with symptomatic intracranial atherosclerotic stenosis (the RICA trial): a multicentre, randomised, double-blind sham-controlled trial in China. Lancet Neurol 2022;21:1089-98. [Crossref] [PubMed]
- Gutierrez J, Turan TN, Hoh BL, Chimowitz MI. Intracranial atherosclerotic stenosis: risk factors, diagnosis, and treatment. Lancet Neurol 2022;21:355-68. [Crossref] [PubMed]
- Gao P, Wang T, Wang D, Liebeskind DS, Shi H, Li T, Zhao Z, Cai Y, Wu W, He W, Yu J, Zheng B, Wang H, Wu Y, Dmytriw AA, Krings T, Derdeyn CP, Jiao LCASSISS Trial Investigators. Effect of Stenting Plus Medical Therapy vs Medical Therapy Alone on Risk of Stroke and Death in Patients With Symptomatic Intracranial Stenosis: The CASSISS Randomized Clinical Trial. JAMA 2022;328:534-42. [Crossref] [PubMed]
- Hong KS, Yegiaian S, Lee M, Lee J, Saver JL. Declining stroke and vascular event recurrence rates in secondary prevention trials over the past 50 years and consequences for current trial design. Circulation 2011;123:2111-9. [Crossref] [PubMed]
- Chimowitz MI, Lynn MJ, Derdeyn CP, Turan TN, Fiorella D, Lane BF, et al. Stenting versus aggressive medical therapy for intracranial arterial stenosis. N Engl J Med 2011;365:993-1003. [Crossref] [PubMed]
- Czap AL, Sheth SA. Overview of Imaging Modalities in Stroke. Neurology 2021;97:S42-51. [Crossref] [PubMed]
- Sui B, Gao P, Lin Y, Jing L, Qin H. Distribution and features of middle cerebral artery atherosclerotic plaques in symptomatic patients: a 3.0 T high-resolution MRI study. Neurol Res 2015;37:391-6. [Crossref] [PubMed]
- Turan TN, Rumboldt Z, Granholm AC, Columbo L, Welsh CT, Lopes-Virella MF, Spampinato MV, Brown TR. Intracranial atherosclerosis: correlation between in-vivo 3T high resolution MRI and pathology. Atherosclerosis 2014;237:460-3. [Crossref] [PubMed]
- Kopczak A, Schindler A, Sepp D, Bayer-Karpinska A, Malik R, Koch ML, Zeller J, Strecker C, Janowitz D, Wollenweber FA, Hempel JM, Boeckh-Behrens T, Cyran CC, Helck A, Harloff A, Ziemann U, Poli S, Poppert H, Saam T, Dichgans M. Complicated Carotid Artery Plaques and Risk of Recurrent Ischemic Stroke or TIA. J Am Coll Cardiol 2022;79:2189-99. [Crossref] [PubMed]
- Zhang X, Chen L, Li S, Shi Z, Tian X, Peng W, Chen S, Zhan Q, Liu Q, Lu J. Enhancement Characteristics of Middle Cerebral Arterial Atherosclerotic Plaques Over Time and Their Correlation With Stroke Recurrence. J Magn Reson Imaging 2021;53:953-62. [Crossref] [PubMed]
- Krittanawong C, Johnson KW, Rosenson RS, Wang Z, Aydar M, Baber U, Min JK, Tang WHW, Halperin JL, Narayan SM. Deep learning for cardiovascular medicine: a practical primer. Eur Heart J 2019;40:2058-73. [Crossref] [PubMed]
- Turan TN, Zaidat OO, Gronseth GS, Chimowitz MI, Culebras A, Furlan AJ, Goldstein LB, Gonzalez NR, Latorre JG, Messé SR, Nguyen TN, Sangha RS, Schneck MJ, Singhal AB, Wechsler LR, Rabinstein AA, Dolan O'Brien M, Silsbee H, Fletcher JJ. Stroke Prevention in Symptomatic Large Artery Intracranial Atherosclerosis Practice Advisory: Report of the AAN Guideline Subcommittee. Neurology 2022;98:486-98. [Crossref] [PubMed]
- Coull AJ, Rothwell PM. Underestimation of the early risk of recurrent stroke: evidence of the need for a standard definition. Stroke 2004;35:1925-9. [Crossref] [PubMed]
- Kwah LK, Diong J. National Institutes of Health Stroke Scale (NIHSS). J Physiother 2014;60:61. [Crossref] [PubMed]
- Leng X, Wong KS, Liebeskind DS. Evaluating intracranial atherosclerosis rather than intracranial stenosis. Stroke 2014;45:645-51. [Crossref] [PubMed]
- Zhang Y, Liu C, Liu M, Liu T, Lin H, Huang CB, Ning L. Attention is all you need: utilizing attention in AI-enabled drug discovery. Brief Bioinform 2023;25:bbad467. [Crossref] [PubMed]
- Wang Y, Zhao X, Liu L, Soo YO, Pu Y, Pan Y, Wang Y, Zou X, Leung TW, Cai Y, Bai Q, Wu Y, Wang C, Pan X, Luo B, Wong KSCICAS Study Group. Prevalence and outcomes of symptomatic intracranial large artery stenoses and occlusions in China: the Chinese Intracranial Atherosclerosis (CICAS) Study. Stroke 2014;45:663-9. [Crossref] [PubMed]
- Prabhakaran S, Liebeskind DS, Cotsonis G, Nizam A, Feldmann E, Sangha RS, Campo-Bustillo I, Romano JG. MYRIAD Investigators. Predictors of Early Infarct Recurrence in Patients With Symptomatic Intracranial Atherosclerotic Disease. Stroke 2021;52:1961-6. [Crossref] [PubMed]
- Sun B, Wang L, Li X, Zhang J, Zhang J, Liu X, Wu H, Mossa-Basha M, Xu J, Zhao B, Zhao H, Zhou Y, Zhu C. Intracranial Atherosclerotic Plaque Characteristics and Burden Associated With Recurrent Acute Stroke: A 3D Quantitative Vessel Wall MRI Study. Front Aging Neurosci 2021;13:706544. [Crossref] [PubMed]
- Yuan W, Liu X, Yan Z, Wu B, Lu B, Chen B, Tian D, Du A, Li L, Liu C, Liu G, Gong T, Shi Z, Feng F, Liu C, Meng Y, Lin Q, Li M, Xu WH. Association between high-resolution magnetic resonance vessel wall imaging characteristics and recurrent stroke in patients with intracranial atherosclerotic steno-occlusive disease: A prospective multicenter study. Int J Stroke 2024;19:569-76. [Crossref] [PubMed]
- Yip SS, Aerts HJ. Applications and limitations of radiomics. Phys Med Biol 2016;61:R150-66. [Crossref] [PubMed]
- Chen Q, Zhang L, Liu S, You J, Chen L, Jin Z, Zhang S, Zhang B. Radiomics in precision medicine for gastric cancer: opportunities and challenges. Eur Radiol 2022;32:5852-68. [Crossref] [PubMed]
- Tang M, Gao J, Ma N, Yan X, Zhang X, Hu J, Zhuo Z, Shi X, Li L, Lei X, Zhang X. Radiomics Nomogram for Predicting Stroke Recurrence in Symptomatic Intracranial Atherosclerotic Stenosis. Front Neurosci 2022;16:851353. [Crossref] [PubMed]
- Meyer M, Ronald J, Vernuccio F, Nelson RC, Ramirez-Giraldo JC, Solomon J, Patel BN, Samei E, Marin D. Reproducibility of CT Radiomic Features within the Same Patient: Influence of Radiation Dose and CT Reconstruction Settings. Radiology 2019;293:583-91. [Crossref] [PubMed]
- Lee SB, Cho YJ, Hong Y, Jeong D, Lee J, Kim SH, Lee S, Choi YH. Deep Learning-Based Image Conversion Improves the Reproducibility of Computed Tomography Radiomics Features: A Phantom Study. Invest Radiol 2022;57:308-17. [Crossref] [PubMed]
- Yaqub M, Jinchao F, Arshid K, Ahmed S, Zhang W, Nawaz MZ, Mahmood T. Deep Learning-Based Image Reconstruction for Different Medical Imaging Modalities. Comput Math Methods Med 2022;2022:8750648. [Crossref] [PubMed]
- Fu F, Shan Y, Yang G, Zheng C, Zhang M, Rong D, Wang X, Lu J. Deep Learning for Head and Neck CT Angiography: Stenosis and Plaque Classification. Radiology 2023;307:e220996. [Crossref] [PubMed]
- Fan X, Feng X, Dong Y, Hou H. COVID-19 CT image recognition algorithm based on transformer and CNN. Displays 2022;72:102150. [Crossref] [PubMed]
- Papanastasiou G, Dikaios N, Huang J, Wang C, Yang G. Is Attention all You Need in Medical Image Analysis? A Review. IEEE J Biomed Health Inform 2024;28:1398-411. [Crossref] [PubMed]